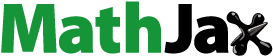
Abstract
This paper establishes a posterior convergence rate theorem for general Markov chains. Our approach is based on the Hausdorff α-entropy introduced by Xing (Electronic Journal of Statistics 2:848–62, 2008) and Xing and Ranneby (Journal of Statistical Planning and Inference 139 (7):2479–89, 2009). As an application we illustrate our results on a non linear autoregressive model.
1. Introduction
The aim of this paper is to study the asymptotic behavior of posterior distributions based on observations which arise from Markov chains. Let be a Markov chain with transition density
and initial density
with respect to some σ-finite measure μ on a measurable space
We assume that the function
and the 2-variable function
are measurable for all parameters θ in the parameter set
So the joint distribution
of
has a density given by
relative to the product measure
where the parameter θ does not depend on the size of
Denote by θ0 the true parameter generating the observations
Note that any semimetric
on the product space of the initial densities and the transition densities induces naturally a semimetric
on Θ when the mapping
is one-to-one, which is assumed in the paper. Given a prior Π on Θ, the posterior distribution
is a random probability measure given by
for each measurable subset B in Θ, where
stands for the likelihood ratio.
Recall that the posterior distribution is said to be convergent almost surely at a rate at least
if there exists r > 0 such that
Posterior consistency is an important issue in Bayesian analysis. Much works were concerned with the asymptotic behavior of posterior distributions for independent and identically distributed observations, see, for instance, Barron, Schervish, and Wasserman (Citation1999), Shen and Wasserman (Citation2001), Ghosal and van der Vaart (Citation2007), Walker, Lijoi, and Prunster (Citation2007), Walker (Citation2003), Walker (Citation2004), Xing and Ranneby (Citation2009), Xing (Citation2011a) and Xing (Citation2011b). An old well-known approach is based on the existence of uniformly consistent tests. In this paper we use an integration condition together with the Hausdorff α-entropy to study convergence rates of posteriors when the observations are not independent and identically distributed. The integration condition and the Hausdorff α-entropy have an advantage in applications, because they both are prior-dependent. The Hausdorff α-entropy condition was introduced in Xing (Citation2008) and Xing and Ranneby (Citation2009) and it is weaker than the metric entropy condition. By means of the integration condition and the Hausdorff α-entropy, we establish a posterior convergence rate theorem for general Markov chains. As applications we discuss the posterior rate of convergence for the non linear autoregressive model.
The layout of this paper is as follows. In Sec. 2 we present a prior-dependent integration inequality and show one type of general posterior convergence rate theorem for Markov chains. In Sec. 3 we illustrate our result by finding a posterior convergence rate for non linear autoregression model. The technical proofs are collected in the Appendix.
2. A convergence rate theorem for Markov chains
In this section we introduce a prior-dependent integration condition to consistency of posterior distributions. Together with the Hausdorff α-entropy, the integration condition plays a central roll in study of the Bayesian convergence rate.
Recall that the Hausdorff α-entropy for a subset
is the logarithm of the minimal sum of αth power of prior masses of balls of d-radius
needed to cover
see Xing (Citation2008) and Xing and Ranneby (Citation2009) for the details of the Hausdorff α-entropy. For simplicity of notations, we define the Hausdorff α-constant
of any subset
of Θ. Observe that
depends on the prior Π. It was proved in Xing and Ranneby (Citation2009) that the inequality
holds for any
where
denotes the minimal number of balls of d-radius
needed to cover
We shall adopt the following Hellinger type semimetrics.
Denote
By means of the metric
Ghosal and van der Vaart (Citation2007, Theorem 5) gave an in-probability posterior convergence rate theorem for stationary α-mixing Markov chains. Since calculation of the α-mixing coefficients is generally not easy and many processes are neither mixing nor stationary, it seems worth to develop a posterior convergence rate theorem for Markov chains which may be neither stationary nor α-mixing. Now we present an almost sure assertion in this direction. Our result is based on the following prior-dependent integration condition.
Throughout this paper the notation means
for some positive constant C which is universal or fixed in the proof. Write
if
and
Denote
which is the integral of the non negative function f with power α relative to the measure P on
Proposition 1.
Suppose that there exist a μ-integrable function r(y) and constants with
such that
and
for all
and
. Let
and
. Then the inequality
holds for all n,
and
, where
Therefore we have
Theorem 1.
Suppose that all assumptions of Proposition 1 hold and suppose that for all large n and some fixed constant
. Suppose that there exist
and a sequence of subsets Θn on Θ such that
for all large j, n, and
Then there exists b > 0 such that for each large r and all large n,
By choosing and
we can easily get
Corollary 1.
Suppose that there exist a μ-integrable function r(y) and constants such that
and
for all
and
. Suppose that
for all large n and some fixed constant
. Suppose that there exist
with
and
and a sequence of subsets Θn on Θ such that for all large j and n,
3. Non linear autoregression
In this section we discuss an application of our theorems. By means of Corollary 1, we improve on the posterior rate of convergence for the non linear autoregressive model in Ghosal and van der Vaart (Citation2007).
We observe of a time series
given by
where
are i.i.d. random variables with the standard normal distribution and the unknown regression function f is in the space
which consists of all functions f with
for some fixed positive constant M. Let
be the density of X0 relative to the Lebesgue measure
on
So
can be considered as a Markov chain generated by the transition density
with
and the initial density
Since
is a strictly positive continuous function tending to zero as
there exist two constants
depending only on M such that
for all
and
Assume that there exists a constant N > 0 such that the set of initial densities of the Markov chain satisfies
for all initial densities
and
For instance, all of the initial densities with
satisfy
and hence form a set with the requirement. Define a measure
in
and a norm
on
Assume that the true regression function
belongs to the Lipschitz continuous space LipM, which consists of all functions f on
satisfying
for all
where L is a fixed positive constant. When the Markov chain is stationary, Ghosal and van der Vaart (Citation2007, Section 7.4) constructed a prior on the regression functions and obtained the in-probability posterior convergence rate
which is the minimax rate times the logarithmic factor
In the following we shall apply Corollary 1 to get the posterior convergence rate
in the almost sure sense for a general Markov chain defined as above.
First, we note that for any
where the last inequality follows from the elementary inequality
Hence for some small constant
we have that
for all large n. Similarly,
hold for all
with
Hence Corollary 1 works well for the metric
We also need some basic facts on approximation of Lipschitz continuous functions by means of step functions. Given a finite interval and a positive integer Kn, we make the partition
with
for
Write
The space of step functions relative to the partition is the set of functions
such that h is identically equal to some constant on each Ik for
more precisely,
for some
where
denotes the indicator function of Ik. Denote by
the function on
which is equal to
on
and vanish outside
Hence
and
where
Let Π be the prior on
which is induced by the map
such that all the coordinates βk of β are chosen to be i.i.d. random variables with the uniform distribution on
Hence the support
of Π consists of all such functions
Take
and
with
Then
Write
for
Since
we have that
and
From the triangle inequality and the inequality
for all x > 0, it follows that for all
and for all large n,
Thus for all large j and n, we have
Note that the Euclidean volume of the Kn-dimensional ellipsoid
is equal to
times the Euclidean volume of the “unit” Kn-dimensional ellipsoid
So the last quotient does not exceed
which is less than
for any given
and all large j. Hence we have obtained condition (ii) of Corollary 1. Similarly, for all large j and n, we have
which, by Lemma 4.1 in Pollard (Citation1990), is less than
for some constant
and therefore condition (i) of Corollary 1 holds for any given
References
- Barron, A., M. Schervish, and L. Wasserman. 1999. The consistency of posterior distributions in nonparametric problems. The Annals of Statistics 27 (2):536–61. doi: 10.1214/aos/1018031206.
- Ghosal, S., and A. W. van der Vaart. 2007. Convergence rates of posterior distributions for noniid observations. The Annals of Statistics 35 (1):192–223. doi:10.1214/009053606000001172.
- Pollard, D. 1990. Empirical processes: Theory and applications. Hayward, CA: IMS.
- Shen, X., and L. Wasserman. 2001. Rates of convergence of posterior distributions. The Annals of Statistics 29 (3):687–714. doi:10.1214/aos/1009210686.
- Walker, S. 2003. On sufficient conditions for Bayesian consistency. Biometrika 90 (2):482–488. doi:10.1093/biomet/90.2.482.
- Walker, S. 2004. New approaches to Bayesian consistency. The Annals of Statistics 32 (5):2028–43. doi:10.1214/009053604000000409.
- Walker, S., A. Lijoi, and I. Prunster. 2007. On rates of convergence for posterior distributions in infinite-dimensional models. The Annals of Statistics 35 (2):738–46. doi:10.1214/009053606000001361.
- Xing, Y. 2008. On adaptive Bayesian inference. Electronic Journal of Statistics 2:848–62.
- Xing, Y. 2011a. Rates of posterior convergence for iid observations. Communications in Statistics - Theory and Methods 39 (19):3389–98. doi:10.1080/03610920903177389.
- Xing, Y. 2011b. Convergence rates of nonparametric posterior distributions. Journal of Statistical Planning and Inference 141 (11):3382–90. doi:10.1016/j.jspi.2010.10.009.
- Xing, Y., and B. Ranneby. 2009. Sufficient conditions for Bayesian consistency. Journal of Statistical Planning and Inference 139 (7):2479–89. doi:10.1016/j.jspi.2008.11.008.
Appendix
Proof of Proposition 1.
Our proof is mainly based on some elementary inequalities such as Jensen’ inequality and Hölder’s inequality. It is no restriction to assume that is an even number. Take non empty disjoint subsets
of Θ such that
and d-diameters of all Bj do not exceed
Then by the inequality
for all
we get
we shall use the notations
and
for
For simplicity we also let
stand for the parameter of the corresponding integral means. Then the last maximum is equal to
which, by Hölder’s inequality, is less than
Take
for each j. From Jensen’s inequality and the assumption
it turns out that
Thus,
and
Write
Take an non negative integer m with
From Hölder’s inequality it turns out that for each j and k,
which, by repeating the above procedure m more times, does not exceed
Thus we get
Hence we have
Repeating the same argument k − 1 times one can get that
Similarly, we have
Hence we have proved the required inequality and the proof of Proposition 1 is complete. □
To prove Theorem 1 we need the following inequality.
Lemma 1.
If there exists a constant such that
for all
and
, then the inequality
holds for all n,
and c > 0.
Proof of Lemma 1.
Without loss of generality, we may assume that From Jensen’s inequality and Chebyshev’s inequality it follows that
So it suffices to prove that
for all
We assume without loss of generality that n is an even number, say
Write
From Hölder’s inequality it then turns out that
Hence by Fubini’s theorem we get that
is equal to
where by the proof of Lemma 1 in Xing (Citation2011b) we have
Thus, we have obtained that
Repeating the same argument k − 1 times and using
one can get
Similarly, we can get that
Therefore
for all
and the proof of Lemma 1 is complete. □
Proof of Theorem 1.
Take a constant c such that Hence
and hence
By Lemma 1 and the first Borel-Cantelli lemma, we get that for almost all
the inequality
holds for all large n. But
Hence, for large n we have
which, by the assumption of Theorem 1, implies that
if the constant b is small enough.
On the other hand, let and let
be the largest integer less than or equal to the constant r. Then, by the inequality
for all
and
we get
which, by Proposition 1 and the inequality assumption of Theorem 1, does not exceed
where the next last inequality holds for all large r and the last inequality holds for all large n. Since the last exponent of n is strictly less than −1 if r is large enough, we obtain that
if the constant r is large enough. Hence, by the first Borel-Cantelli lemma we obtain that for almost all
if n is large enough. The proof of Theorem 1 is completed. □