Abstract
An analysis of health provider choice together with a model of implicit rationing in healthcare is presented using Mozambique as a case study. We make use of the Mozambican Household Surveys on Living Conditions 2002/3 and 2008/9. Updated and sometimes contrasting results with respect to similar analyses on older Mozambican data are obtained. In particular, we find that the role of income is more important in 2002/3 and in 2008/9 than it was in 1996/7. In consequence, we further expand the analysis by studying how socioeconomic disparities among other factors influence implicit rationing in healthcare. When using the 2008/9 survey only, moderate evidence that some sort of implicit healthcare rationing is in place is found; that is, some people do not seek care because they foresee they will not be granted quality healthcare access or treatment.
Keywords:
JEL classification:
1. Introduction
The study of health-related behaviour in developing countries is gaining further interest in economics because of its implications in economic activity and outcomes. Health status, and consequently health services' availability and quality, are strictly linked to well-being and productivity. Moreover, understanding the determinants of healthcare demand and use is particularly helpful for guiding public interventions in the health sector. In this article an analysis of health provider choice together with a model of implicit rationing in healthcare is presented, using Mozambique as a case study. We want to understand: first, which are the most influential characteristics associated with seeking care from different healthcare providers; second, whether ill individuals who do not seek care do so for reasons that are more related to unobservable characteristics/social norms that are not registered in the survey questionnaire.
Concerning healthcare provider choice, we present updated and sometimes contrasting results with respect to the ones described in Lindelow (Citation2005) based on the 1996/7 household survey. The analysis of rationing in healthcare access and treatment contained in Section 5 instead is the first attempt to our knowledge of modelling and analysing the importance of perceived barriers to health access in Mozambique, which we claim can be fruitfully applied to similar countries and contexts.
Studying health-related behaviour and the existence of perceived barriers in healthcare access is primarily important: like several other developing countries with analogous characteristics, Mozambique is expanding the supply of healthcare facilities to improve healthcare access while granting better service quality. This effort is often embedded in the context of broader poverty reduction strategies, and improving health access for the poor is particularly stressed in some of these strategies. Nonetheless, demand issues cannot be ignored in such contexts, and more effective results can be obtained if individuals' choices concerning preventive and curative healthcare are explored. Understanding the determinants of healthcare utilisation is then extremely useful for public health policies.
On top of this, health-seeking behaviour is greatly influenced by characteristics that are seldom registered in non-specific household surveys. Akin et al. (Citation1986) have already pointed out that it is often difficult for the researcher to understand why some facilities or services are used while others are not. Perceived quality characteristics or barriers to access of the different facilities are rarely registered, and the complex set of social norms and traditions that shape healthcare interactions is mostly unobservable. Personal preferences of the health personnel as well as social judgements about status, gender, and other characteristics may play an important role in biasing the provision of care. In some cases, this may also prevent specific categories of individuals from seeking care.
This complex interaction of discretionary decisions made by health personnel on the basis of such norms and judgements as to the amount and type/quality of resources to be made available to healthcare seekers – given limited resources – is generally defined as ‘implicit rationing’ (Mechanic, Citation1997). For example, when fees for healthcare services are nominal or nil but the system is resource poor – as is generally the case in sub-Saharan Africa, and in particular in Mozambique – the allocation of these services is often unequal and regressive (Stierle et al., Citation1999; Kapiriri & Martin, Citation2007), mostly favouring the urban areas and the elites, who find queue-avoiding mechanisms (Pinho, Citation2008; Rosen et al., Citation2010). Studying the implicit rationing mechanisms at work in healthcare is then fundamental to further understand what does not work and what might work better in the process of healthcare provision and use. Concerning the analysis of health provider choices in Mozambique, our analysis finds that the role of income is more important in 2002/3 and in 2008/9 than it was in 1996/7 (Lindelow, Citation2005). In consequence we further expand the analysis by studying how socioeconomic disparities influence implicit rationing in healthcare.
Our study especially investigates the possibility that some people, because of their limited ability to pay or perception about their possibility to receive quality healthcare access or treatment, may not seek care in the first place. Hence, a probability of accessibility to medical services is obtained, with special emphasis on non-price determinants of healthcare allocation and use. Previous modelling only considers the probability to seek treatment from a certain provider given personal, household, and community characteristics. In order to fully identify the rationing mechanisms at work, the probability of receiving good treatment, conditional on seeking care, is also modelled here. As the literature on queue jumping and rationing illustrates, for those who cannot jump the queue, getting to the chosen healthcare provider does not ensure receiving the desired treatment. This is modelled by means of a probability of receiving satisfying treatment that depends primarily on economic status, education, and distance to the health provider. Using the 2008.9 survey only, moderate evidence is found that some sort of healthcare rationing is in place.
The model used in the first part of the article is a standard random utility model with dichotomous choice between care and self-care (Gertler & Van Der Gaag, Citation1990) as the dependent variable, which is estimated with a probit. For the polytomous choice of the healthcare provider, a more flexible model is used (Dow, Citation1996; Lindelow, Citation2005), and estimated by means of a multinomial logit and an ordered probit model.
Two cross-sectional surveys, the Mozambican Household Survey on Living Conditions (Inquérito aos Agregados Familiares sobre Orçamento Familiar [IAF]) 2002/3 and the Survey on Living Conditions (Inquérito sobre Orçamento Familiar [IOF]) 2008/9, are employed. These surveys are richer and more detailed compared with those implemented in 1996/7, yielding more robust results and deeper insights into households' health-related behaviour. Section 2 presents the theoretical model used for the analysis of both the binary choice care/no care and of the multinomial/ordered choice of the provider. The datasets used are presented in Section 3, and Section 4 introduces the econometric specification and discusses the results. In Section 5 the problem of implicit rationing is introduced while a possible strategy to devise such rationing is discussed. Section 6 concludes discussing policy implications and further research.
2. Theoretical model
Following relevant works on provider choice and health-related behaviour in developing countries (Mwabu, Citation1984; Akin et al., Citation1986, Citation1995; Dor & Van Der Gaag, Citation1993; Ellis et al., Citation1994; Glick et al., Citation2000; Sahn et al., Citation2003; Ssewanyana et al., Citation2004; Lindelow, Citation2005), the decision to seek care is analysed by means of a random utility model. This model is used to study the dichotomous decision to seek care or not, the dichotomous decision to seek formal care or not, and the polytomous decision about the health provider where to seek care. The random utility model seems particularly suitable for a developing country framework where more sophisticated models meet practical difficulties in their application (Gertler et al., Citation1987; Gertler & Van Der Gaag, Citation1990).
2.1 The choice to seek care or not and the choice to seek care from a certain provider
For the dichotomous case about seeking care or not, we take the expected utility of seeking or not seeking care (i.e. choosing self-care), respectively equal to Uc and Usc. These depend on expected health status when seeking or not seeking care, Hc and Hsc, and on consumption of goods other than health, Cc and Csc:
Uj depends on expected health status when seeking care from provider j, Hj, and on consumption of goods other than health, Cj. Again, Cj is modelled as residual consumption after the decision to seek care from provider j. Hj will depend on individual, household and community characteristics, while Cj can be derived from the budget constraint as:
Each individual will maximise Uj:
Moreover, since the healthcare alternatives here identified can be broadly ordered from worst to best, we also estimate an ordered probit model. The alternatives were ranked as follows: 1, self-care; 2, TMP; 3, health post; 4, health centre; 5, hospital and other formal providers.
3. Data
The datasets used are the Household Survey on Living Conditions IAF 2002/3 and IOF 2008/9, which are nationally representative household surveys conducted by the National Institute of Statistics (INE). The 2002/3 survey (IAF 2002/3) was conducted from July 2002 to June 2003, while the 2008/9 survey (IOF 2008/9) was conducted from August 2008 to September 2009. The surveys have a stratified structure with three steps of selection: selection of the primary sampling units; selection of the enumeration areas within the primary sampling units; and selection of the households within the enumeration areas.
Twelve households were selected in each rural area and nine in each urban area. Twenty-one strata were constructed, one for each urban/rural sample of the 11 provinces (the province of Maputo city does not have a rural area). The final sample size of the IAF 2002/3 amounts to 44 083 individuals in 8727 households: 4020 urban households and 4707 rural households. The IOF 2008/9 instead has a sample size of 51 177 individuals in 10 832 households, divided into 5223 urban households and 5609 rural households. Both surveys are representative at the national, regional (North, Centre, South), provincial and urban/rural level. Sampling weights are provided in the survey dataset.
The surveys include information on general characteristics of the individuals and of the households, home consumption, transfers and gifts, as well as on daily, monthly, and durable goods final consumption expenditures. Community surveys are also available, but only for rural areas, containing information on community characteristics. Supplementary information for the IAF 2002/3 can be found in Ministry of Planning and Finance (Citation2004) and in INE (Citation2004), while for the IOF 2008/9 it can be found in INE (Citation2010).
With regards to health-related issues, the IOF 2008/9 has a more detailed health section, but both surveys contain information on permanent illnesses, on past episodes of sickness, on the choice of healthcare providers, and relative satisfaction with the treatment. Moreover, in the community questionnaires there is comprehensive information on distances to different healthcare providers.
4. Econometric model, variables and estimation results
Starting from the model outlined in Section 1, we use different econometric specifications when studying the dichotomous case of care/self-care and the polytomous case of healthcare provider choice. In the dichotomous case, a simple linear equation is used, following among others Mwabu (Citation1984) and Akin et al. (Citation1986, Citation1995). From Equation (1) we thus specify:
For the polytomous choice problem, instead, the empirical model is extended with the inclusion of cross-price terms in each provider's equation.Footnote2 In this case, a multinomial logit model and an ordered probit model are used in order to assess the probability to seek care from each provider.Footnote3 The multinomial logit model is a valid alternative provided that the independence of irrelevant alternatives assumption holds; otherwise, other specifications should be used. In what follows the independence of irrelevant alternatives assumption is tested by means of a Hausman test to make sure that estimates are reliable. All econometric estimations are corrected for the stratified survey design and weighted according to the sampling weights provided in order to ensure representativeness.
4.1 Variables used and results
In the econometric estimation of the models outlined in Sections 1 and 3, the dependent variable is first the dichotomous variable care/self-care and then a polytomous variable that has as possible outcomes the different healthcare provider alternatives.Footnote4 The latter variable is treated as non-ordered in the multinomial logit estimation and as ordered in the ordered probit estimation, as described in Section 2.
For independent variables, several individual, family, and community characteristics are considered. As price variables, only the time price is considered since the official fees are either very low or unevenly paid by the population throughout the country (Lindelow et al., Citation2004). The opportunity cost of time that is employed is the median – computed for each community – of per-capita hourly consumption: this is not as a good measure of opportunity cost as is wage, but for many developing countries, and in particular for Mozambique, data on wages are either missing or non-existent. This is mainly due to the fact that few people work in the formal sector. In contrast, hourly consumption, although suffering from possible endogeneity problems, is available for the whole sample.
Individual income is measured by (real) per-capita daily consumption (household consumption divided by household size). This is the measure of income that is also used in official analyses of poverty carried out by the Mozambican government. A composite measure of consumption that takes into account daily and monthly expenditures, durable goods use and house rent, self-consumption, and in-kind earnings is used. All of these different sources of consumption are averaged out on a daily basis, providing a good measure of economic status for Mozambican households. This measure of income also takes into account the inflation occurred during the implementation of the surveys, the different values of the Metical – the Mozambican currency – over different periods of the year, and spatial differences in price levels among different provinces and areas (rural/urban).Footnote5
The information on illness episodes, decision to seek care and where, problems experienced during the medical consultation and time needed to reach the closest health facility is also used as a key variable. The other variables in the regressions include standard controls: individual characteristics such as age, gender, ability to read and write; family characteristics such as the household head education level, household size, and household members per room; and community characteristics such as accessibility through public transport and conditions of the main road, and which healthcare provider is present and how far it is from the community.Footnote6 Descriptive statistics for the variables used are presented in , while descriptive statistics for healthcare facility utilisation are shown in .
Table 1: Descriptive statistics
Table 2: Healthcare facility utilisation (%)
4.2 Seeking care or not – Probit results
In the marginal effects of the probit results for the dichotomous decision to seek care or not are introduced. Socioeconomic status – expressed by the logarithm of per-capita daily consumption – is strongly associated with seeking care. Computed marginal effects show an increase of about 9% and 7% in the probability of seeking care respectively for 2002/3 and 2008/9.Footnote7
Table 3: Probit – Seek care or not (marginal effects)
Such results contrast with previous findings by Lindelow (Citation2005) on Mozambican data for 1996/7, but are in line with the literature on healthcare seeking (Dor et al., Citation1987; Sahn et al., Citation2003; Ssewanyana et al., Citation2004). This may be due to differences in the sample used – urban and rural households in the present article versus only rural households in Lindelow (Citation2005) – or it might reflect a change in the behaviour of Mozambican society between 1996/7 and 2002/3.Footnote8
Higher level of education is also associated with a greater probability of seeking care: this is reflected by the coefficient on the ability to read and write, which appears to be more important than formal educational training in 2002/3, and by the education level of the household head in 2008/9. Moreover, greater distance to the closest healthcare provider is negatively associated with the decision to seek care. This effect is in line with existing literature (Lindelow, Citation2005); coefficients are highly significant in 2002/3 and only at 10% significance level in 2008/9. Coefficient directions are as expected.
4.3 Choosing where to seek care – Multinomial logit results
The following analysis deals with the choice of healthcare provider, as outlined in Section 1. In 2002/3 socioeconomic status has a positive and significant effect for health centres and hospitals, strongly negative and significant for self-care. Moreover, ability to read and write maintains the expected positive sign for health centre, hospital, and other formal providers, while it is negative for self-care. In addition, if the household head has eight or more years of education, this plausibly decreases the probability to seek care from a TMP. Concerning distance, a negative effect is observed for the health post and the health centre alternatives, while a significant positive effect is displayed for self-care or traditional care. Individuals from rural areas are also less likely to seek care from hospitals.
In 2008/9 socioeconomic status has the expected positive sign only for the health centre and other formal provider alternatives, but maintains the negative sign for the self-care alternative. A higher level of household head education positively influences the choice of seeking care from a health centre, a hospital, and other formal providers, and it is negatively associated with seeking self-care or traditional care. Finally, living in a rural area or in an inaccessible community is negatively associated with seeking care from hospital, other formal providers, and a health centre.
Interestingly, distance to health facilities seemed to be more important in 2002/3 than in 2008/9. In 2002/3 the need to build more health facilities was still of primary importance. In 2008/9 the increased availability of health providers probably made distance less important in the process of seeking care, while other factors maintained its importance. Marginal effects for 2002/3 and 2008/9 are computed and displayed in and respectively.
Table 4: Multinomial logit – Where to seek care (marginal effects, 2002/3)
Another observation concerns a change in health-seeking behaviour between 2002/3 and 2008/9. In , looking at the percentage of ill people seeking care from a traditional medical practitioner in 2002/3 shows that this figure is 10 percentage points higher than the same figure in 2008/9 (about 14% of ill individuals in 2002/3 and 4% of ill individuals in 2008/9). Despite only the last consultation being registered in the survey, this suggests that people are becoming more prone to seek care from formal providers.Footnote9 This is probably influenced by the increase in the number of rural health providers that occurred between the two dates. A graphical analysis of marginal effects (for relevant variables) is found in , while a few robustness checks are presented in Appendix B.
4.4 Choosing where to seek care: Ordered probit results
As introduced in Section 2, when analysing the choice of different healthcare facilities, a viable alternative is to treat them as if they were ordered. A plausible ranking – from worst to best – of the different choice is used in this case: 1, self-care; 2, TMP; 3, health post; 4, health centre; 5, hospital and other formal providers. In this case the analysis can be conducted using the ordered probit model, which is simpler than the multinomial logit and requires fewer assumptions. The analysis conducted using the ordered probit model mostly confirms and reinforces the results obtained from the multinomial logit model. As shown by and , it also yields more robust results. Both for 2002/3 and 2008/9 the effect of income, education/ability to read and write, distance to health facilities, and the effect of living in rural areas emerge much more clearly than in the multinomial logit analysis. In particular, higher income and education/ability to read and write positively and significantly influence the probability of choosing ‘better’ alternatives – health centre, hospital and other formal providers – and negatively influence the probability of choosing ‘worse’ ones – self-care and TMP.Footnote10 Conversely, the opposite signs are observed for the coefficients of living in rural areas/inaccessible communities or living far from health facilities ( and ). Again, distance to health facilities seemed to be more important in 2002/3 than in 2008/9.
Table 5: Multinomial logit – Where to seek care (marginal effects, 2008/9)
Table 6: Ordered probit – Where to seek care (marginal effects, 2002/3)
Table 7: Ordered probit – Where to seek care (marginal effects, 2008/9)
5. Implicit rationing in healthcare access
In Mozambique, the vast majority of healthcare providers are public and fees are set very low with many exemptions for children under-five and other categories (Lindelow et al., Citation2004). However, especially in rural areas, it is not easy to provide healthcare service that is good and accessible to all. Lack of medicine, lack of trained staff, long waiting times, and requests for informal payments have been frequently reported as existing problems (Newman et al., Citation1998; INE, Citation2004; Posse & Baltussen, Citation2009). In such circumstances it is likely that some ill individuals do not receive a full or successful treatment.
Given the above, there are at least two types of possible rationing mechanisms in place. The first, and more obvious, is the one due to objective obstacles to healthcare access; for example, occurring on a first-come first-served basis. The other is more subtle and is due to the fact that personal characteristics of the ill may also affect the way in which healthcare is provided; that is, what was described as ‘implicit rationing’ in Section 1. In particular, social status and distance (cultural and physical distance) may contribute to influence health access and care.
This section is thus devoted to the analysis of implicit rationing in healthcare, and we particularly focus on whether some people do not seek care because they foresee they will not be granted quality healthcare access or treatment.
This is not new to qualitative anthropological and economic studies on healthcare access in different countries (Ahmed et al., Citation2000; Khan et al., Citation2000; Griffiths & Stephenson, Citation2001; Baltussen et al., Citation2002; Ager & Pepper, Citation2005; Goins et al., Citation2005; Baltussen & Ye, Citation2006; Chibwana et al., Citation2009) and was confirmed by repeated discussions had with patients, nurses, missionaries, and non-governmental organisation professionals in Mozambique. Ill individuals try to seek care from healthcare providers, but sometimes they do not receive the treatment they expect. As a consequence of this they no longer seek care from such providers. Nonetheless, we experienced that expectations about access and quality of treatment were not the same among different kind of people. In particular, poorer people, people living far away from the chosen health provider, and people with lower social status all had much worse expectations about their possibility to be granted quality healthcare access and treatment. Richer people or people of higher social status seemed more able to find queue avoiding mechanisms and to receive good treatment. If one knows that for his personal characteristics he is more likely to have problems in access to healthcare providers, he will probably decide to seek care only when it is strictly necessary. Many people we interacted with clearly took the risk of ‘bad’ treatment into account. Hence, they thought twice before walking many hours to the healthcare provider or paying for public transport and wasting a workday. This was also confirmed by people who had experienced sickness episodes but did not seek care at all or did not seek formal care. In what follows, only two quotes are presented, which summarise most of the answers in informal interviews at different health providers in the districts of Erati and Monapo, Northern Mozambique.
I went to the hospital to get drugs for leprosy, they told me to come back on the next day. When I went on the next day, they told me: ‘Come next week’. I am not going anymore. Every time I go, I have to walk many hours and waste a working day. (A poor woman)
When they go to the hospital with me, then the nurse finds the medicines; when they go on their own, he says there are no medicines. (A missionary)
The model is then extended to the case when receiving good treatment is not certain, but it happens with probability pi. pi is modelled as the individual probability of receiving a good treatment and depends on education, income, demographic characteristics, area of residence, distance to the facility, and other controls already presented in Section 4. The distance to the facility can be interpreted as transport costs faced by the ill and/or as an additional indicator of her poverty status, which implies that if one lives far away from a health facility, then the probability of seeking care from the facility is lower, and in turn the probability of receiving good treatment is lower. On the other hand, a positive effect coming from higher education and income is expected.
If the expected health status, Hj, depends on pi, then we have that:
We assume that each provider has a certain quality that is based on objective characteristics of the provider.Footnote11 Other conditions being equal, the fact that having a good treatment is not certain increases the probability of choosing self-care. Indeed, if an ill individual is not certain that seeking care from a health provider would make a difference with self-care (because he expects that for his characteristics he will not be granted a quality access or treatment), then this increases the probability of choosing self-care.
In the health section of the surveys' questionnaire, people were asked to answer whether they experienced problems in access or treatment and which problem they had. They could choose among lack of hygiene, long waiting time, lack of qualified staff, too expensive, lack of medicines, unsuccessful treatment, corruption, and other problems.Footnote12 People who were ill but did not seek care were instead asked to provide a reason why they did so. The available options were: not necessary, too expensive, too far away, lack of means of transportation,Footnote13 and other reasons. In our analysis we try to understand what is behind the ‘other’ reasons and whether this answer hides an implicit rationing in healthcare.
For example, it results that most of the people who were ill and did not seek care, and who answered that they did so for ‘other reasons’, were living in rural areas (about 80%, as opposed to a national average of about 70%). Moreover, these people have a mean per-capita consumption that is lower than mean per-capita consumption for the entire population (17.5 Mt against 21.5 Mt), and is also lower than mean per-capita consumption for the entire population of ill people. This might suggest that this is a subgroup of particularly disadvantaged people who perceive that for their characteristics they can experience problems in healthcare access or treatment.
Concerning the estimation strategy, a three-step procedure is followed:
Step 1 – a probit-with-selection (heckprobit) model is used to assess the probability of experiencing problems in access or treatment, provided that one seeks care. The list of explanatory variables includes all of the previously introduced covariates. Furthermore, for 2008/9 also available is a variable that registers the number of primary health units – health centres and health posts – per person in the district. This has been constructed from the annex to the National Inventory of Health Infrastructures, Services and Resources Citation2007, provided by the Mozambican Ministry of Health and National Institute of Health.Footnote14 In principle, all of the variables that affect the probability of seeking care also influence the probability of experiencing problems in access or treatment. However, as evidenced in Wooldridge (Citation2002), among others, Heckman-type models are better identified with exclusion restrictions similar to instrumental variables. Among the independent variables, it was chosen to exclude from the second tier of the model the number of days in which the individual was ill, as this is likely to affect the probability of seeking care relatively more than the probability of experiencing problems in access or treatment. However, since this variable is continuous only for 2008/9 – and continuous variables work better as instruments in the estimation procedure – implicit rationing in healthcare was only analysed for this year. Indeed, the number of days the individual was ill seems to work well in the model. It is highly significant in the selection equation and results in evidence for a selection issue ().
Step 2 – after the estimation, the conditional probability of experiencing problems in access or treatment, Pr(problems = 1|seek care = 1), is predicted for the whole sample of sick people, and also for those who did not seek care for various reasons. This provides a measure that we interpret as potential rationing.
Step 3 – we try to answer the following question: is there potential rationing in the choice of seeking care? That is to say, the people who were ill but did not seek care for ‘other’ reasons,Footnote15 are the same as those who had a medium-high probability of experiencing problems in access or treatment? Evidence on this issue is that the percentage of ill people who did not seek care for ‘other’ reasons is not negligible (about 25% of the ill who did not seek care in 2002/3, about 15% in 2008/9), and indeed it is generally associated with a medium-high probability of experiencing problems in access or treatment.
Table 8: Probit with selection – experiencing problems conditional on seeking care, with marginal effects
5.1 Estimation of the conditional probability and rationing
In the results for the probit-with-selection estimation, with marginal effects, are shown, while in the imputed rationing probability for ill people who did not seek care for ‘other’ reasons is presented. The results in confirm expectations about the sign of most regressors. In particular, rural people and people living far away from a healthcare provider are more likely to experience problems in access or treatment. Moreover, socioeconomic status significantly affects the probability of experiencing problems in access or treatment. A unit increase in (log) consumption decreases the probability to experience problems by about 4%. Education only seems to affect the probability of seeking care but is not significantly associated with the probability of experiencing problems in access or treatment.
Table 9: Imputed probability of experiencing problems for ill people who did not seek care for ‘other’ reasons, 2008/9 (%)
The (log) number of primary health units divided by the population of the district also displays the expected sign in the selection equation.
As introduced, the number of days the individual was ill is positive and significant in the selection equation, and it results that there is evidence for a selection issue. The parameter ρ is negative and significantly different from zero. As a consequence, the lambda term (ρ·σ) has a negative sign, which suggests that the error terms in the two equations are negatively correlated. Hence, (unobserved) factors that make care-seeking (selection) more likely tend to be associated with a lower probability of experiencing problems in healthcare access or treatment. Those who report having experienced problems are relatively less likely to seek care.
After computing the conditional probability of experiencing problems in access or treatment given care seeking, it was checked whether such probability correctly predicts the behaviour of ill people who actually sought care and did or did not experience problems. shows that the computed probability correctly predicts the behaviour of about 60% of the sample in 2008/9 (bold data in the table). The columns display the percentage of people experiencing problems or not, while the rows show there is the percentage of people having a conditional probability lower or higher than the distribution mean.
Table 10: Predicted and actual behaviour of ill people, 2008/9 (%)
In , instead, the distribution of the conditional probability of experiencing problems for people who were ill and sought care and did not actually experience problems (grey), or experienced problems (black), is plotted. It is possible to notice that the black distribution lies on the right of the grey distribution, indicating that the constructed probability discriminates, at least in part, between the two groups. Moreover, results from the Wald test show that the means of the two distributions are different at the 1% confidence level (F = 394.1).
Figure 2: Conditional probability of experiencing problems, 2008/9
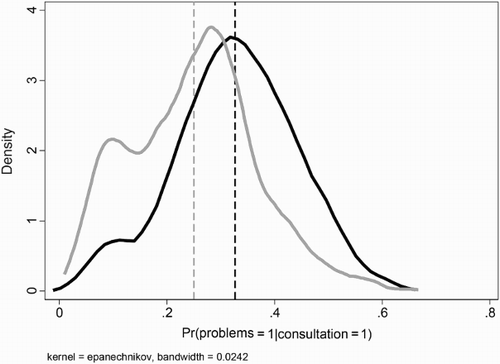
From it is also possible to see that a cumulative percentage of 75% of ill individuals not seeking care for ‘other’ reasons have an imputed probability of experiencing problems in access or treatment greater than 20%. And that, 39% of such individuals have an imputed probability greater than 30%. Moreover, it results that 49% of ill individuals not seeking care for ‘other’ reasons have an imputed probability greater than the distribution mean (corresponding to about 27%).
These findings are interpreted as supporting the hypothesis that some sort of implicit rationing exists. Some people are induced not to seek care at all because they fear that after reaching the healthcare provider their economic and travel efforts could be wasted because of problems in access or treatment. Moreover, it seems that the probability of experiencing problems in access or treatment is not equally distributed in the population, but that poorer people and people that are more distant from health providers are more disadvantaged.
6. Conclusions and policy implications
In this article we examined the issues of healthcare seeking and implicit rationing in Mozambique. The first part of the article analysed which are the individual, household and community characteristics that most affect the dichotomous choice of seeking care or not, the choice of seeking care from a formal healthcare provider provided that one seeks care, and finally the polytomous/ordered choice of where to seek care among different providers. Nationally representative cross-sectional data from the Mozambican Household Survey on Living Conditions IAF 2002/3 and IOF 2008/9 were used. We highlighted the role of socioeconomic indicators together with education and distance variables as important determinants for the choice to seek care. Moreover, gender and education variables also seemed to play a role in the decision about the choice to seek care from formal providers. With regards to the choice among different healthcare providers, the evidence on the explicative power of different variables was mixed when the analysis was conducted using the multinomial logit model; it was more robust when the ordered probit model was alternatively used. The fact that the percentage of people seeking care from informal providers such as the traditional medical practitioner declined between 2002/3 and 2008/9 was also emphasised.
In the second part of the article, an analysis of whether some sort of implicit healthcare rationing is in place in Mozambique was carried out. To this aim, an imputed conditional probability of experiencing problems in access or treatment was constructed, on the basis of the explicative variables previously introduced, but excluding the number of days the individual was ill and using it as an instrument in the probit-with-selection model. Next, whether people who were ill but did not seek care for ‘other’ reasons had an imputed probability of experiencing problems high enough to dissuade them from seeking care was studied. It was found that the percentage of people showing an imputed probability of experiencing problems higher than 20 to 30% – out of the sample of people not seeking care for ‘other’ reasons – was not negligible. Our interpretation is that some form of implicit healthcare rationing exists, against poorer people and people that are more distant from health providers.
Concerning policy recommendations, the results of the study indicate that health policy should take the demand side of the healthcare utilisation process into greater consideration. For example, even though fees are set very low and there are exemptions, the fact that poorer people face relatively bigger obstacles in their access to healthcare needs to be tackled by public health policy-makers in a more compelling way. As shown in the second part of the article, a great deal of improvement could be achieved by addressing perceived social barriers and preventing health personnel from carrying out implicit rationing practises.
Also, the increased supply of healthcare providers between 2002/3 and 2008/9 reduced the importance of distance as a determinant of health provider choice. However, individuals from rural/inaccessible communities still seek healthcare sensibly less than their urban counterparts. This suggests that increasing health consciousness among non-urban communities together with an improvement in the quality of rural health facilities should be encouraged.
In general, our study shows that problems related to the quality of healthcare need to be given greater attention by policy-makers. Further research on this topic should investigate more thoroughly the reasons why people do not seek care. Future surveys should include more detailed questions regarding the quality of healthcare facilities, also considering that a non-negligible percentage of people answered they experienced ‘other’ problems. Moreover, the indication of the specific healthcare facility chosen when ill could better inform policy-makers on the quality of different providers. Further multidisciplinary researches involving anthropology and psychology could also better disentangle the implicit rationing process and its consequences on health-seeking behaviour.
Acknowledgements
The author is grateful to Kenneth Simler, Channing Arndt and Valentina Gil for providing help with data collection and analysis; to Maureen Mackintosh, Rebecca Hanlin and Cecilia Navarra for useful comments on preliminary drafts; and to the Comboni Missionaries of Alua (Mozambique) for providing hospitality and very valuable insights on the Mozambican reality. The author is especially grateful to the Università degli Studi di Siena for the support provided, and to Francesca Bettio for extremely helpful supervision and support.
Notes
2Dow (Citation1996) provides justifications for this and for other potential extensions.
3Again, details on the reparametrisation and on the possibility to estimate such a model by means of a multinomial logit model can be found in Lindelow (Citation2005:450–3).
4We do not take into account the whole range of possible options since some of them are chosen by very few people. So we put into one group all the other formal providers others than health post, health centre, hospital and TMP.
5Thirteen regional poverty lines have been computed for Mozambique, in order to take into account the differences in price levels in the various provinces and rural/urban areas (Simler et al., Citation2004).
6The distance variables are used both alone and in interaction with the shadow price of time to obtain the prices of the different providers. These are expressed respectively by dummies in 2002/3 and by a continuous variable in 2008/9. Some of the community-level variables are only available for rural communities. Values for urban areas are imputed on the basis of information available through other sources.
7Both the Wald and Likelihood Ratio test reject dropping the expenditure-related variable. The difference in the coefficients for the logarithm of per-capita daily expenditures for 2002/3 and 2008/9 is not statistically significant.
8In the sense that perhaps the better-off have developed a greater attention to healthcare in these years.
9See also Appendix A.
10Interestingly, it seems that the coefficients for the variables of interest for the health post alternative change their sign between 2002-3 and 2008-9. Indicating that perhaps health posts were among the ‘good’ alternatives in 2002--3 while they turned to be less good alternatives in 2008-9, given the increased availability of better equipped health facilities (health centres and hospitals/other formal providers).
11In this feature our model differs from the one in Dor et al. (Citation1987), which instead models providers' quality as depending on individual characteristics.
12We exclude the ‘too expensive’ problem, because of possible sources of endogeneity.
13This option was only available in the IOF 2008/9.
15We exclude people who declared they did not seek care because it was not necessary, because it was too expensive or because the healthcare provider was too distant, only keeping those who declared they did not seek care for ‘other’ reasons. People could give more than one answer.
References
- Ager, A & Pepper, K, 2005. Patterns of health service utilization and perceptions of needs and services in rural Orissa. Health Policy and Planning 20(3), 176–84. doi: 10.1093/heapol/czi021
- Ahmed, SM, Adams, AM, Chowdhury, M & Bhuiya, A, 2000. Gender, socioeconomic development and health-seeking behaviour in Bangladesh. Social Science and Medicine 51(3), 361–71. doi: 10.1016/S0277-9536(99)00461-X
- Akin, J, Griffin C, Guilkey, DK & Popkin, BM, 1986. The demand for primary health care services in the Bicol region of the Philippines. Economic Development and Cultural Change 34(4), 755–82. doi: 10.1086/451558
- Akin, J, Guilkey, D & HazelDenton, E, 1995. Quality of services and demand for health care in Nigeria: A multinomial probit estimation. Social Science & Medicine 40(11), 1527–37. doi: 10.1016/0277-9536(94)00274-W
- Baltussen, R & Ye, Y, 2006. Quality of care of modern health services as perceived by users and non-users in Burkina Faso. International Journal for Quality in Health Care 18(1), 30–4. doi: 10.1093/intqhc/mzi079
- Baltussen, R, Ye, Y, Haddad, S & Sauerborn, R, 2002. Perceived quality of care of primary health care services in Burkina Faso. Health Policy and Planning 17(1), 42–8. doi: 10.1093/heapol/17.1.42
- Chibwana, AI, Mathanga, DP, Chinkhumba, J & Campbell Jr, CH, 2009. Socio-cultural predictors of health-seeking behaviour for febrile under-five children in Mwanza-Neno district, Malawi. Malaria Journal 8(219), 1–8.
- Dor, A, Gertler, P & Van Der Gaag, J, 1987. Non-price rationing and the choice of medical care providers in rural Côte d'Ivoire. Journal of Health Economics 6(4), 291–304. doi: 10.1016/0167-6296(87)90017-8
- Dor, A & Van Der Gaag, J, 1993. Quality rationing and the demand of adults for medical care in rural Cote d'Ivoire. In Mills, A and Lee, K (Eds.), Health Economics Research in Developing Countries. Oxford University Press, Oxford, pp. 291–304.
- Dow, W, 1996. Unconditional Demand for Health Care in Côte d'Ivoire: Does Selection on Health Status Matter? Living Standards Measurement Study Working Paper No. 127, The World Bank, Washington, DC.
- Ellis, R., McInnes, D & Stephenson, E, 1994. Inpatient and outpatient health care demand in Cairo, Egypt. Health Economics 3(3), 183–200. doi: 10.1002/hec.4730030307
- Gertler, P, Locay, L & Sanderson, W, 1987. Are user fees regressive? The welfare implications of health care financing proposals in Peru. Journal of Econometrics 36(1–2), 67–88. doi: 10.1016/0304-4076(87)90044-3
- Gertler, P & Van Der Gaag, J, 1990. The Willingness to Pay for Medical Care: Evidence from Two Developing Countries. Johns Hopkins University Press, Baltimore, MD.
- Glick, P, Razafindravonona, J & Randretsa, I, 2000. Education and health services in Madagascar: Utilization patterns and demand determinants. Cornell Food and Nutrition Policy Program Working Paper No. 107, Ithaca, NY.
- Goins, R, Williams, K, Carter, M, Spencer, S and Solovieva, T, 2005. Perceived barriers to health care access among rural older adults: a qualitative study. The Journal of Rural Health 21(3), 206–13. doi: 10.1111/j.1748-0361.2005.tb00084.x
- Griffiths, P & Stephenson, R, 2001. Understanding users’ perspectives of barriers to maternal health care use in Maharashtra, India. Journal of Biosocial Science 33(3), 339–59. doi: 10.1017/S002193200100339X
- INE (National Institute of Statistics), 2004. Inquérito Nacional aos Agregados Familiares Sobre Orçamento Familiar 2002/3. http://www.ine.gov.mz/pt/ResourceCenter/DownloadFile?id=973 Accessed 27 February 2014.
- INE (National Institute of Statistics), 2010. Inquérito Sobre Orçamento Familiar, 2008/09 – Quadros Básicos. http://www.ine.gov.mz/pt/ResourceCenter/DownloadFile?id=967 Accessed 27 February 2014.
- Kapiriri, L & Martin, D, 2007. Bedside rationing by health practitioners: a case study in a Ugandan hospital. Medical Decision Making 27(1), 44–52. doi: 10.1177/0272989X06297397
- Khan, A, Walley, J, Newell, J & Imdad, N, 2000. Tuberculosis in Pakistan: Socio-cultural constraints and opportunities in treatment. Social Science & Medicine 50(2), 247–54. doi: 10.1016/S0277-9536(99)00279-8
- Lindelow, M, 2005. The utilisation of curative healthcare in Mozambique: Does income matter? Journal of African Economies 14(3), 435–82. doi: 10.1093/jae/eji015
- Lindelow, M, Ward, P, Zorzi, N & World Bank Africa Regional Office Human Development Department, 2004. Primary Health Care in Mozambique: Service Delivery in a Complex Hierarchy. Africa Region Human Development Working Paper No. 69, The World Bank, Washington, DC.
- Mechanic, D, 1997. Muddling through elegantly: finding the proper balance in rationing. Health Affairs 16(5), 83–92. doi: 10.1377/hlthaff.16.5.83
- Ministry of Health & National Institute of Health, 2007. National Inventory of Health Infrastructures, Services and Resources, Mozambique 2007 (Inventario Nacional de Infraestruturas de Saude, Serviços e Recursos, Moçambique 2007) http://www.misau.gov.mz/pt/misau/dpc_direccao_de_nacional_planificacao_e_cooperacao/departamento_de_informacao_para_a_saude_e_monitoria_e_avaliacao/documentos_chave_do_sistema_de_informacao_para_a_saude/sam_inventario_nacional_de_infra_estruturas_de_saude_2007 Accessed 30 August 2011.
- MPF (Ministry of Planning and Finance), 2004. Poverty and Well-Being in Mozambique: The Second National Assessment (2002–2003). National Directorate of Planning and Budget, Ministry of Planning and Finance, Economic Research Bureau, Ministry of Planning and Finance, International Food Policy Research Institute, Purdue University, Maputo, Mozambique.
- Mwabu, G, 1984. Conditional Logit Analysis of Household Choice of Medical Treatments in Rural Villages in Kenya. Nairobi: Kenyatta University College.
- Newman, R, Gloyd, S, Nyangezi, J, Machobo, F & Muiser, J, 1998. Satisfaction with outpatient health care services in Manica Province, Mozambique. Health Policy and Planning 13(2), 174–80. doi: 10.1093/heapol/13.2.174
- Pinho, M, 2008. Racionamento dos cuidados de saúde: problemática inerente. Cadernos de Saúde Pública 24(3), 690–5. doi: 10.1590/S0102-311X2008000300023
- Posse, M & Baltussen, R, 2009. Barriers to access to antiretroviral treatment in Mozambique, as perceived by patients and health workers in urban and rural settings. AIDS Patient Care and STDs 23(10), 867–75. doi: 10.1089/apc.2009.0050
- Rosen, S, Sanne, I, Collier, A & Simon, J, 2010. Rationing antiretroviral therapy for HIV/AIDS in Africa: Efficiency, equity, and reality. Health and Development Discussion Paper No. 4. Center for International Health and Development, Boston University, Boston, MA.
- Sahn, D, Younger, S & Genicot, G, 2003. The demand for health care services in rural Tanzania. Oxford Bulletin of Economics and Statistics 65(2), 241–60. doi: 10.1111/1468-0084.t01-2-00046
- Simler, K, Mukherjee, S, Dava, G & Datt, G, 2004. Rebuilding after war: Micro-level determinants of poverty reduction in Mozambique. International Food Policy Research Institute Research Report 132, International Food Policy Research Institute, Washington, DC.
- Ssewanyana, S, Nabyonga, J, Kasirye, I & Lawson, D, 2004. Demand for health care services in Uganda: Implications for poverty reduction. EPRC Research Paper, Economic Policy Research Centre, Makerere University, Kampala, Uganda.
- Stierle, F, Kaddar, M, Tchicaya, A & Schmidt-Ehry, B, 1999. Indigence and access to health care in sub-Saharan Africa. The International Journal of Health Planning and Management 14(2), 81–105. doi: 10.1002/(SICI)1099-1751(199904/06)14:2<81::AID-HPM543>3.0.CO;2-P
- Wooldridge, JM, 2002. Econometric Analysis of Cross Section and Panel Data. The MIT Press, Cambridge, MA.
Appendix A: Seeking formal care or not – Probit-with-selection results
A slightly different issue related to the choice of seeking care is the choice of seeking care from a formal provider (i.e. excluding self-care and TMPs). In order to study this problem, a probit-with-selection model, checking for the probability to seek formal care provided that one seeks care, is run first. (While for 2002/3 there is evidence of a selection bias, the same is unattested for 2008/9, for which reason in this second case a simple probit model was run.) In both cases, estimation results (not shown) are very similar to the ones presented in Section 4.2. Some differences are found for 2002/3, where the household head education and female dummies have a statistically significant positive coefficient. That individuals from more educated backgrounds are more likely to seek care from formal healthcare providers is a plausible and well-established result. On the other hand, the fact that ill female individuals seek more formal care is an interesting finding that deserves to be investigated more in depth. (A possible explanation comes from standard utility model frameworks such as Acton's, where individuals with higher opportunity costs of time – generally adult males – demand less medical care.) One account of this finding depends certainly on the fact that women are more likely to seek formal sector care for pre-natal and birthing care, which typically represents a large proportion of all health visits in poor countries.
Appendix B: Robustness checks
This appendix describes a few robustness checks performed on our base model. A first robustness check consists of running the same multinomial logit regressions but using a constructed measure of wage as the shadow price of time. In particular, information about wages in the household questionnaire is used to compute the median wage for each province. Given that complete information about wages was only present in the IOF 2008/9, this robustness check is only undertaken for this year. What emerges from this check (not shown) is that the coefficient of the income variable is still significant for the health post, health centre, and other formal provider alternatives. While the coefficients for education and distance are only significant for the health post alternative, ability to read and write is found to influence the choice of health centre and other formal providers.
In another robustness check, an alternative specification of income, namely consumption per adult equivalent, is used instead of consumption per capita. The former takes into account possible economies of scale within the household. For 2008/9, results (not shown) say that income remains significant for the choice of seeking care from health post, health centre and other formal providers. Also, the coefficients for education and distance are only significant for the health post alternative, whereas ability to read and write seems to influence the choice of health centre, and other formal providers.