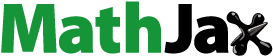
ABSTRACT
Although previous studies have tried to estimate the impact of innovation on employment growth at the firm level in developed economies, very few studies have investigated this phenomenon in contexts of developing countries with high unemployment rates. This study builds on an existing multi-product approach to develop an econometric model, which analyses the relationship between innovation and employment growth rate at firm level. The analysis of the data indicates that sales growth of new to firm and new to market products have a positive (increasing) effect on employment growth for manufacturing but not the services sectors. On the other hand, overall, process innovations have a negative (decreasing) effect on employment growth, for both the manufacturing and service sectors. The study contributes to the scarce empirical literature in sub-Saharan Africa, by assessing the impact of innovation on employment at firm level using innovation data from CIS-type surveys.
1. Introduction and background
Innovation has been recognised in both academic and industrial research as an important factor that enables firm performance, competitive advantage and growth. On a macro level, innovation has been shown to positively impact the economic development of countries (Aghion et al., Citation2009). Through the development of new products and services, as well as the significant improvement of existing products or services, innovation allows firms to grow through various innovation outcomes. These include financial outcomes (e.g. increased revenue, or improved profitability), strategic and marking outcomes (e.g. entering new markets, or increase in market share), product outcomes (e.g. increase in range of goods) and process outcomes (e.g. development of new services).
More significant for this paper, previous studies have shown that innovation can influence employment growth at the firm level (Brouwer et al., Citation1993; Blechinger et al., Citation1998; Harrison et al., Citation2014; Calvino, Citation2019). However, as a result of recent rapid technological advancements such as machine learning and artificial intelligence, innovation may have unintended consequences including further increasing unemployment levels. This is a particular concern in the context of developing countries with already high unemployment rates. As such, it is important to investigate the link between innovation and employment at the firm level in developing country contexts with high unemployment rates (Vivarelli, Citation2014). Such investigation can assist with understanding how and why different types of innovation are more likely to have negative effects on employment (Calvino & Virgillito, Citation2018). Moreover, understanding the link between innovation and employment growth at firm level in such contexts can help to ascertain how innovations aimed at saving cost (through automation, for example) can impact positively or negatively on employment growth at firm level (Vivarelli, Citation2014; Ugur et al., Citation2018). An investigation that explores the direct and indirect effects of different types of innovation is thus potentially of value, to avoid the unintended consequences of further increasing unemployment levels.
The objectives of this study are therefore twofold: (1) to investigate the effects of innovation on employment in contexts of upper-middle-income countries with high unemployment rates and (2) to analyse and explain the direct and indirect effects of different types of innovations activities on employment growth. These objectives are motivated by the need to promote innovation and to alleviate high unemployment levels in contexts of upper- middle-income countries with high unemployment. Thus, the research question guiding this study is formulated as
What are the effects of innovation activities on employment growth in contexts of upper-middle-income countries with high unemployment rates?
This paper uses the growth of market novelties, which is used less frequently in the literature. It sheds light on this approach and makes a methodological contribution by using the instrumental variables (IV) method. The use of the IV method solves a methodological problem, namely the violation of one of the assumptions of OLS regression approach that the explanatory variables are uncorrelated with the error term.
The outline of the paper is as follows. First, the section to follow presents a summary of the literature. This section discusses previous studies on the topic and builds up to the theoretical framework. Second, the theoretical foundation and model development section informs the development of the model used in the study. Third, the empirical context in which this study is situated is presented. Fourth, a section that discusses the dataset used in the study is presented. This section also presents descriptive statistics of the key variables in the model. Fifth, the estimation procedure used in fitting the model to South African data is described. The econometric results are presented in the sixth section. The final section presents the conclusions and recommendations of the study regarding the relationship between innovation and employment growth.
2. Literature review
Innovation is considered an important driving force for economic growth in developing countries (Aubert, Citation2005). Early studies have reported a relationship between innovation and employment (Chennells & Van Reenen, Citation2002; Spiezia & Vivarelli, Citation2002). The review of literature has shown that the impact of innovation on employment can be studied from three different levels: firm, sector and national levels. Further analysis of literature indicates that there are numerous research gaps that still need to be investigated on the impact of innovation on employment growth (Pianta, Citation2003; Bryson et al., Citation2012).
A critique of the literature on the link between innovation and employment revealed a number of factors that may influence this relationship (Becheikh et al., Citation2006; Lööf & Heshmati, Citation2006; Djellal & Gallouj, Citation2007). These factors include country-specific dynamics such as the labour market and a wide range of institutional factors such as government policies on innovation. Moreover, early studies that investigated the link between innovation and employment have mostly used R&D-based indicators to quantify the impact of innovation on employment (Boyer, Citation1988). However, there are problems associated with using R&D indicators to link innovation to employment. According to Peters (Citation2008), R&D-based indicators tend to underestimate innovation activities in small and medium enterprises as well as in service sector firms.
With respect to studies that have used innovation-based indicators, existing debates in the literature point to multiple ways in which product and process innovations can generate or decimate employment (Harrison et al., Citation2014). Although the literature suggests a positive relationship between innovation and employment, the majority of these studies analysed the link between innovation and employment in contexts where unemployment levels are low (Rodrıguez-Pose & Crescenzi, Citation2008). In addition, given that most technological innovations typically occur in higher income economies or developed countries, Mitra & Jha (Citation2015) argue that these innovations ‘ … are made to suit these economies and their factor endowments … ’. Furthermore, in these advanced economies, new technological innovations are mostly geared towards increasing profits by reducing labour (Blechinger et al., Citation1998; Griffith et al., Citation2006; Dachs & Peters, Citation2014).
This study differs from previous studies in two main ways: first, it analyses and tests if the relationship between innovation and employment observed in developed/industrialised countries with low unemployment rates holds in upper-middle-income countries with high unemployment rates, using non-R&D based indicators. Second, the study examines the effects of innovations aimed at saving costs on employment, using a large sample disaggregated by different sectors.
3. Theoretical foundation and model development
Peters (Citation2008) notes that, theoretically, there are several ways through which innovation can positively impact (by creating new jobs) and negatively impact (by destroying existing jobs) on employment. It is argued that process innovations that are aimed at saving costs (rationalisation innovations) are expected to have a negative impact on employment growth. This is primarily because there will be fewer resources (in particular labour) required to produce outputs. This is referred to as the productivity effect. However, in the long-run, this may have a positive impact on employment growth, given that cost reductions may be transferred to output prices to improve production output. This is referred to as the price effect.
With respect to product innovations, employment growth may be impacted positively by a high demand for new products, irrespective of whether the product is new to the firm or new to the market. This is referred to as the demand effect. However, the relationship between the old and new products may also impact positively or negatively on employment growth (Hou et al., Citation2019). If the demand for both old and new products is high, logically, employment growth should increase and vice versa. Peters further argues that if there is an inverse relationship between old and new products, then it is not clear what the impact on employment growth is. This is because a number of factors may play a positive or negative role. These factors include, for example, the level of novelty of the new product and the lifecycle of the product or the sector (Mairesse & Wu, Citation2019). According to the product lifecycle theory, for new products that have not yet reached stage four and five of their lifecycle (i.e. saturation and decline respectively), the demand for the new product will remain high (Vernon, Citation1979, Citation1992).
The model used for the evaluation of the impact of innovation on employment in this paper is an adaptation of the model developed by Peters (Citation2004, Citation2008). This model was built from the multi-product model developed by Jaumandreu (Citation2003) and Harrison et al. (Citation2014), using specific information provided by Community Innovation Surveys (CIS) data to analyse effects of innovation activities on employment at the firm-level. The multi-product model is based on the production function from economic theory that relates output to capital, labour and material inputs. The applied empirical model establishes a theoretical link between employment growth and output in terms of sales growth of innovative products (Peters, Citation2004, Citation2008). The model by Jaumandreu (Citation2003) is based on transforming the economic model into an econometric model, and taking into account that efficiency gains are likely to be different between process innovators and non-process innovators. This leads to the model in Equation (1) below.(1)
(1) where l : employment growth rate; α0 : (negative) average efficiency growth for non-process innovators; α1 : average efficiency growth for process innovators; d : dummy variable indicating process innovators; y1 : real output growth due to old products; y2 : real output growth due to new products; ε : error term, with E(ε |y1, y2) = 0.
Key to Equation (1) is that non-process innovators can achieve efficiency gains due to, for example, exogenous technological change, learning or spill-over effects. One problem with estimating the model in Equation (1), however, is that we do not observe real output growth, but nominal sales growth. Therefore, Jaumandreu (Citation2003) derived Equation (1) in nominal variables to get the following estimating equation:(2)
(2) where g1 = y1 + π1 : nominal rate of sales growth of old products; g2 = y2 + π2
y2 : sales ratio of old to new products; π1 : price growth of old products; π2 : ratio of price difference between new and old products to the price of the old product; υ = –π1 – βπ2
y2 + ε : error term.
As noted by Jaumandreu (Citation2003), the relationship in Equation (2) implies endogeneity as well as identification problems for the estimation. The identification problem arises because we do not observe the firm-level prices which implies that we also do not observe the sales growth of old products, π1, included in the error term. As a result, we cannot identify the gross employment effect of productivity (efficiency) gains but only the net employment effect, which has been accounted for through indirect price effects. To overcome this problem, Jaumandreu (Citation2003) suggested using the disaggregated sector averages, µ1, which results in replacing the left-hand side of Equation (2) with l1 – (g1 − µ1). The endogeneity problem occurs because, by definition, g2 is correlated with the error term, υ. This is associated with a reverse-causality problem due to the fact that, theoretically, g2 has a causal effect on g1, and conversely g1 also has a causal effect on g2. In other words, sales growth of unchanged or not significantly modified products can have an effect on sales growth of new products, and vice versa. Using the Ordinary Least Squares (OLS) regression method to estimate the equation would lead to biased and inconsistent estimates of the coefficients. A solution to this problem is to assume that E(π2 |y2) = 0 (Jaumandreu, Citation2003; Peters, Citation2004; Harrison et al., Citation2005; Peters, Citation2008, Harrison et al., Citation2008, Citation2014). This way, E(π2 y2) = 0 and y2 is uncorrelated with π2 y2. This boils down to the assumption that π2 is mean independent of y2 and implies that the relative price difference between new and old products is not a function of the relative output growth of the new products. This assumption seems reasonable, as the price of the new product may be dependent on many other unrelated factors, including quality improvements, substitutability among the goods, demand shifters, market power and so on (Peters, Citation2008). This allows us to look for the suitable instruments that are correlated with y2 but uncorrelated with the error term. This implies the use of the Instrumental Variables (IV) regression technique.
In the present study, the IV method entails substituting g2 with a variable correlated to g2, but not correlated with the error term, υ. Suitable variables for such substitution as well as the choices made for the estimation in this paper are discussed in the estimation procedure section of the paper.
A shortfall with the model developed by Jaumandreu (Citation2003) is that it measures the effect of the sales growth (on the function of labour and output from old products) from the two novelties of new products as a single combined effect (i.e. new to the market and new to the firm). The implication is that the impacts of these novelties are indistinguishable. Also, the model does not account for impacts attributable to process innovations. To address these limitations, Peters (Citation2004, Citation2008) extended the model to: (1) separate the impacts of the sales growth of new products into new to market and new to firm novelties and (2) account for both rationalisation process innovations as well as other process innovations. Rationalisation innovations refer to process innovations intended to reduce production costs. Equation (3) below illustrates this model.(3)
(3)
where Pc : dummy indicating rationalisation process innovations; Pnc : dummy indicating other process innovations; g2m : sales growth generated by market novelties; g2f : sales growth generated by firm novelties.
Peters (Citation2004, Citation2008) controlled for non-innovation factors in Equation (3) by removing firms affected by such factors from their sample. Examples include firms that closed part of the enterprise.
In this paper, we control for such firm characteristics and economic behaviour by including them in the model, as illustrated in Equation (4) below. One advantage of this approach is that it allows the model to determine the statistical significance or lack thereof of each control factor, while retaining all the sample observations.(4)
(4)
Ci denotes the control variables, characteristics and economic behaviour variables of the firm. These variables are described in detail under the empirical estimation procedure in a later section of this paper.
4. Empirical context: the case of South Africa
One of South Africa’s biggest problems over the last ten years has been its poor economic growth. The overall Gross Domestic Product (GDP) has been on the decline year on year (World Bank, Citation2018). Poor economic growth has been accompanied by high levels of unemployment. The World Bank estimates the average unemployment rate for countries classified in the upper-middle-income countries to be 6 percent (Yu, Citation2017; World Bank, Citation2018).
South Africa’s unemployment rateFootnote1 stood at 26 percent in 2016 and 27 percent in 2017 (STATS SA, Citation2017; World Bank, Citation2018). In contrast to the unemployment rate in one of its closest neighbours (also classified in the same upper-middle-income bracket), Botswana, stands at 18 percent (Yu, Citation2017). Furthermore, South Africa has the highest unemployment rate among all OECD countries (OECD, Citation2018).
To address this problem, the South African government has developed and adopted a number of policies geared towards reducing rising unemployment. One such policy is the National Development Plan (South African Government, Citation2012) which presents ‘ … a long-term strategy to increase employment and broaden opportunities through education, vocational training and work experience, public employment programmes … ’. One of its highly ambitious goals is to reduce the rising unemployment to 6 percent by 2030. Another important policy, the New Growth Path adopted in 2011, proposed to reduce unemployment to 15 percent by the end of 2020. However, despite these policies, unemployment has continued its rising trend. Understanding the effect of innovation on employment is therefore particularly significant in such a context.
5. Dataset and descriptive statistics
The analysis uses data extracted from the South African Business Innovation Survey 2010–2012 database (HSRC INNOV Citation2010–Citation2012). This dataset allows for an evaluation of the impact of different types of product innovations (new to market or new to firm) and process innovations (intended to reduce production costs or other process innovations) that may influence a firm’s employment level. The survey design is based on the guidelines of the Organisation for Economic Co-operation and Development (OECD) Oslo Manual (OECD/Eurostat) (Citation2005) and more specifically the methodological recommendations for round four of the Community Innovation Survey (CIS 4) of 2006 (EuroStat, Citation2006) provided by Eurostat, the Statistical Office of the European Commission.
The survey questionnaire is directly comparable with the core questionnaire for round 4 of the OECD Community Innovation Survey (CIS 4). Sampling was informed by the structure of the Business Register of Statistics South Africa, from which a stratified random sample by sector (determined according to the Standard Industrial Classification (SIC) system) and size of the business enterprise (based on turnover) was drawn. Determining enterprise size classes on the basis of turnover is a departure from the CIS guidelines, which recommend that the enterprise size classes be based on the number of employees so that the survey can target only enterprises that have ten or more employees. However, the Business Register of Statistics South Africa that was available at the time of sample design did not enable size stratification by number of employees. Nonetheless, an effort was made to sample enterprises to link turnover size cut-offs to employment via a schedule prescribed in the National Small Business Amendment Act, 2003 (No. 26 of 2003). The results presented here are based on data from the realised samples of enterprises that responded as follows:
328 manufacturing (222 innovating and 106 non-innovating); and
379 services (218 innovating and 161 non-innovating), with the following break-down:
179 wholesale and retail trade (82 innovating and 97 non-innovating);
93 transport, storage and communication (57 innovating and 36 non-innovating);
33 financial intermediation (24 innovating and 9 non-innovating);
36 computer and related activities (28 innovating and 8 non-innovating);
12 research and development (11 innovating and 1 non-innovating);
21 architectural and engineering (14 innovating and 7 non-innovating);
5 technical testing and analysis (2 innovating and 3 non-innovating).
Therefore, the data analysis involved filtering the innovating enterprises first and then analysing the innovation information provided by these enterprises accordingly.
The descriptive statistics of key variables in the model are summarised in . The results show the following: (1) there was higher employment growth for innovators than non-innovators, except in the Financial Intermediation sector. (2) For innovators: (a) sales growth of old products (g1) exceeded sales growth of each of new to market (g2m) and new to firm products (g2f), and (b) sales growth of new to firm products (g2f) exceeded sales growth of new to market products (g2m).
Table 1. Descriptive statistics [Mean (Standard deviation)] for employment growth and sales growth of innovative and non-innovative firms, 2010–2012
6. Estimation procedure
As mentioned in Section 3, the model section, the variables and the BIS 2010–2012 data that were used to estimate the model in Equation (4) are based on the CIS questionnaire. provides the definitions of these variables, in a way that indicates how they are computed from the CIS data.
Table 2. Definitions of variables used in the empirical model.
As noted in Section 6, and captured in above, the employment effect of product innovation is evaluated through two terms, g2m and g2f for sales growth of new to market and new to firm novelties, respectively. Similarly, the effect of process innovation is measured through two terms, Pc and Pcn, for rationalisation (cost-reduction) process innovations and other process innovations, respectively. The use of two separate terms for each type of innovation (product and process) was introduced by Peters (Citation2004). This method is an extension of an earlier model used by Jaumandreu (Citation2003), which had a single combined effect for both new to market and new to firm product innovations and single combined effect for all process innovations. In the case of process innovation, it would be useful to have a further breakdown of Pc and Pcn by process only innovators, and product and process innovators, to provide four categories (terms in the model) for process innovations. These are: (1) process only innovators with rationalisation innovations (rationalisation 1), (2) process only innovators with other process innovations (other 1), (3) product and process innovators with rationalisation innovations (rationalisation 2) and (4) product and process innovators with other process innovations (other 2) (). Such a breakdown is important since, by definition, process only innovators would have applied process innovations on old products (introduced at the beginning of the reference period, 2010–2012), while product and process innovators would have applied process innovations on old products as well as new products (introduced during the reference period).
Figure 1. Venn Diagram: Different types of product & process innovators (Source: Authors’ own illustration).
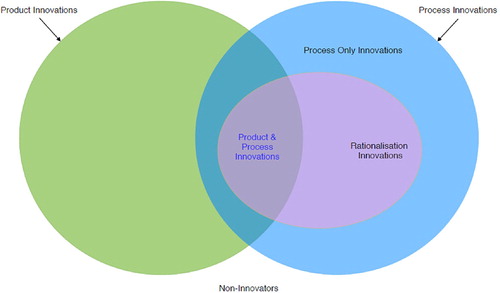
As depicted by the model, the employment effects of process innovations are dependent on what types of processes are under consideration (e.g. rationalisation innovations and other process innovations). A further breakdown of the types of process innovations is illustrated in above. As mentioned above, the model in Equation (2) has an endogeneity problem. Using OLS to estimate it would produce inconsistent parameter estimates. Therefore, the final model used in this paper is estimated by applying the IV method. This method comprises substituting the independent variable(s) that cause(s) endogeneity in the model with a different variable which is correlated with the variable causing endogeneity, but uncorrelated with the error term. In the model used in this paper, two variables can potentially cause endogeneity. These are g2m and g2f. Peters (2003, Citation2004) noted that sales growth of product innovations is likely to be correlated with the following factors, with the variables in parentheses identified as potential instruments in the empirical analysis to measure these factors:
Innovation input (R&D intensity: ratio of R&D expenditure to sales or innovation)
Intensity: ratio of innovation expenditure to sales;
Effects of product innovations (range: expansion of range of goods, quality: quality improvements or market: increase in market shares);
Degree of product novelty (market novelty share: share of sales due to market novelties);
Appropriability conditions (patents: firms which applied for at least one patent in 2010–2012);
Technological capabilities (continuous R&D: continuous engagement in intramural R&D activities);
Competitiveness (export intensity: ratio of exports to sales or export growth rate: growth rate of exports during 2010–2012);
Integration of customers in the innovation process (clients: customers have a high importance as an innovation source).
Descriptions of the instruments and their methods of computation are presented in .
Table 3. Descriptions of the instruments and their methods of computation
However, the BIS 2010–2012 used for this paper did not collect data on exports, and the variable on share of sales due to market novelties may well be endogenous to g1, while the variables associated with intramural R&D may be endogenous to employment growth. Therefore, these variables were excluded from further consideration as instruments in this paper, leaving only the variables in to be tested as instruments in the empirical analysis.
Before testing the strength and validity of the instruments, a test of whether or not g2m and g2f are truly endogenous to g1 was conducted for both manufacturing and services firms. The test for manufacturing firms produced Durbin and Wu-Hausman scores, 15.91 and 7.95, with very low p values, 0.0003 and 0.0005, respectively. Similarly, the Durbin and Wu-Hausman scores, 6.66 and 3.16, for services firms had sufficiently low p values, 0.03 and 0.04, respectively, to reject the null hypotheses that g2m and g2f are exogenous. Therefore, we were correct in treating these variables as endogenous. Upon performing the Sargan test for over-identification in the model, the p-values for both the Sargan and Basmann scores came up high for manufacturing firms, 0.25 and 0.27, respectively, which suggests that the instruments are indeed valid and the model is correctly specified. Similarly, the p-values, 0.94 and 0.95 for the Sargan and Basmann scores respectively, were high for services firms, suggesting that the instruments are valid and the model is correctly specified.
7. Econometric results
The empirical results reveal a significant relationship between firm-level employment and product and process innovations, in the manufacturing sector and in three selected services sectors. The services sectors are: (1) wholesale and retail trade, (2) transport, storage and communication, (3) financial intermediation, (4) computer and related activities, (5) research and development, (6) architectural and engineering, and (7) technical testing and analysis. presents the results on the effects of innovation on employment growth for South African manufacturing firms for the period 2010–2012, while presents the results on the effects of innovation on employment growth for South African firms in selected services sectors for the same period.
Table 4. Effects of Innovation on Employment for South African Manufacturing Firms, 2010–2012.
Table 5. Effects of Innovation on Employment for South African Services Firms, 2010–2012.
In the regressions on selected services, the OLS model estimates a significant effect for sales growth of new to market products, while the instrumented regressions do not. An explanation for this is that in the OLS model, there is an additional indirect effect of sales growth of the market products via the error term, since the term for sales growth of new to market products is endogenous to sales growth of unchanged or marginally improved products, i.e. correlated with the error term.
One of the main results is that product innovations in manufacturing firms have a significant positive effect on employment in the sense that higher sales growth rates from product innovations, both new to the market and new to the firm, correspond to higher employment growth rates. However, for services firms, there were no significant effects due to new product novelties. For manufacturing firms, this result is similar to a finding by Peters (Citation2004, Citation2008) based on an analysis of German firms, that new jobs are not only created in firms with novelties (new to the market), but also in firms which successfully pursue product imitation strategies (only new to the firm), with the impact being larger for manufacturing than service firms.
A previous result by Falk (Citation1999) is partly in contrast with this result, indicating product innovations new only to firm do not have a significant impact on employment demand, while those new to market do. Jaumandreu (Citation2003) also found sales growth of new products to be positively associated with employment growth for Spanish firms.
The empirical analysis shows that process innovations were responsible for a reduction in employment in the period 2010–2012 in both the manufacturing and services sectors. Peters (Citation2004) found that such a reduction had occurred in the period 1998–2000 in German manufacturing, but not in the service sector. This author explained this by the way manufacturing displacement effects outweigh compensation effects, resulting in a negative employment growth effect. Service firms tend to react more aggressively and pass on to prices only the productivity gains due to innovations. The fact that the South African service firms also experienced negative employment growth in the period 2010–2012 suggests that either they did not pass on to prices just the innovation productivity gains, or did not pass them on fast enough to have a net positive growth on employment.
Furthermore, the analysis shows that manufacturing and service firms with both new products and new processes experience negative employment growth, while firms that introduced only new process innovations experience positive employment growth. This result is the opposite of the finding of Peters (Citation2004, Citation2008) that German manufacturing firms which introduced only process innovations in the period 1998–2000 experienced negative employment growth, but not firms that introduced product and process innovations. This could be explained by South African firms employing additional personnel to implement new process innovations on only old products (as process only innovators would) rather than both old and new products (as product and process innovators would). Moreover, some important differences are revealed by comparing the manufacturing and service sectors in terms of the employment effects of rationalisation process (intended for cost-reduction) versus other process innovations. For process only innovators, firms in both sectors experience positive employment growth from other process innovations (not intended for cost-reduction). However, firms with rationalisation process innovations (intended for cost-reduction) experienced positive employment growth for the manufacturing sector and negative for the service sector (Crespi et al., Citation2019). On the other hand, for product and process innovators, firms in both sectors experience negative employment growth from other process innovations. However, firms with rationalisation process innovations only experience negative employment growth in the manufacturing sector, while this is not the case in the service sector. These results confirm that, as concluded by Peters (Citation2004, Citation2008), different innovation strategies lead to different price behaviour. Peters (Citation2004, Citation2008) further noted that there was no evidence of a clear robust effect (positive or negative) of process innovations on employment. The outcome may well depend on the firm’s economic activity.
In terms of the non-innovation factors for which the assessment of the employment effect of innovation controlled based on the empirical model, manufacturing firms that established subsidiaries in other African countries experienced a reduction in their employment growth (Avenyo et al., Citation2019). This could be explained by the fact that, by opening subsidiaries elsewhere on the continent, jobs could be transferred there, resulting in a negative effect on employment growth locally. Manufacturing start-ups experience an increase in their firm-level employment growth. This could be expected as start-ups, by definition, have to grow and such growth includes employment. Service firms that are part of a larger group experience a negative employment growth. Becoming part of a larger group during the reference period, 2010–2012, could mean establishing a subsidiary or subsidiaries in other African countries or outside of Africa or merging or taking over other enterprises. The net result of this undertaking on the firm could be a reduction in employment.
8. Conclusion and recommendation
Using the approach proposed by Jaumandreu (Citation2003) and extended by Peters (Citation2004, Citation2008), this paper has analysed the relationship between employment growth rates and innovation output in terms of sales growth generated by new products and process innovations at firm-level. The extension by Peters (Citation2004, Citation2008) considers the fact that employment effects are expected to behave according to innovation type. Therefore, the extension distinguishes between (1) two different types of product innovations (new to market and new to firm) and (2) two different types of process innovation (rationalisation intended for cost-reduction and other). While Peters (Citation2004, Citation2008) controls for firm characteristics by removing from the sample firms that are affected by those factors, this paper controls for firm characteristics and economic behaviour by including them in the model, to be able to control for their effects, while limiting the loss of observations in the analysis.
The empirical results show that both types of product innovations (new to market and new to firm) have a positive effect (increase) on employment for manufacturing but not the services sectors. On the other hand, overall, process innovations have a negative effect (decrease) on employment growth, for both the manufacturing and service sectors. However, although manufacturing firms are expected to experience a negative employment effect from process innovations, service firms are expected to experience a positive effect (Peters, 2003, Citation2004). This is because displacement effects outweigh compensation effects in the manufacturing sector, resulting in a negative employment growth rate.
Service firms tend to react more aggressively, passing on to prices only the productivity gains due to innovations (Peters, 2003, Citation2004). This is either not the case at all or not occurring fast enough for a positive employment growth rate to be realised in South African service firms.The employment effect of process innovation differs between process only innovators, and product and process innovators. For both manufacturing and service firms, process only innovators have a positive employment growth rate effect, while product and process innovators experience a negative employment growth effect from process innovations. In this regard, of note is that process only innovators would have applied their process innovations on only ‘old’ products, as they would have not introduced new products, while product and process innovators would have applied their process innovations on both ‘old’ and new products. Some of these process innovations would be rationalisation (cost-reduction) innovations that would have probably compelled the firm to shed some jobs. The outcome of the results associated with the employment effect of process innovations is that there is no straight-forward conclusion regarding the employment effect of rationalisation (cost-reduction) process innovations compared to other process innovations (Peters, Citation2008). For process only innovators, rationalisation innovations have a positive employment growth effect. However, other process innovations have a positive employment growth effect for manufacturing firms but the opposite (negative effect) for service firms.
The implication of the results is that, to avert the negative growth in employment due to process (including rationalisation) innovations, firms in both the manufacturing and service sectors should pass on to prices only the efficiency gains due to these innovations. For product and process innovators, rationalisation innovations have a negative employment growth effect (Mairesse, & Mohnen, Citation2010; D’Attoma, & Pacei, Citation2018). However, other process innovations have a negative employment growth for manufacturing firms but no significant effect for service firms.
The results of the study presented in this paper have the following implications for the upper-middle-income countries with high unemployment rates: With modern technologies (e.g. automations, robotics, artificial intelligence), the trend of (short-term) loss of jobs due to process innovations is expected to continue for both the manufacturing and service sectors. This raises policy questions across a wide range of government departments (ministries), including labour, science and technology, trade and industry, higher education and training and even home affairs (responsible for skilled immigration). The questions arise: Do upper-middle-income countries (e.g. South Africa) have the necessary skills for the jobs created due to product and process innovations? What types of jobs are lost due to process innovations, and are these jobs for which these countries have skills shortages, requiring training or skills importation? With a high unemployment rate and in the context of companies seeking to increase their shareholder value, how can governments in these countries work closely with firms and unions to strike and maintain a reasonable balance between the need to stay innovative (and hence competitive) and the need to retain and create new jobs?
Further studies like this need to be conducted using future business innovation surveys to confirm the trends for South Africa. Comparative studies with other countries in Africa and the global South would provide further insight into the employment effects of innovation in upper-middle-income countries.
Disclosure statement
No potential conflict of interest was reported by the author(s).
Notes
1 Based on the strict definition of unemployment, i.e. only includes those actively looking for work.
References
- Aghion, P, David, PA & Foray, D, 2009. Science, technology and innovation for economic growth: linking policy research and practice in ‘STIG Systems’. Research Policy 38(4), 681–93. doi: 10.1016/j.respol.2009.01.016
- Aubert, J-E, 2005. Promoting innovation in developing countries: A conceptual framework. The World Bank Policy Research Working Paper, (3554).
- Avenyo, EK, Konte, M & Mohnen, P, 2019. The employment impact of product innovations in sub-Saharan Africa: Firm-level evidence. Research Policy 48(9), 103806. doi: 10.1016/j.respol.2019.103806
- Becheikh, N, Landry, R & Amara, N, 2006. Lessons from innovation empirical studies in the manufacturing sector: A systematic review of the literature from 1993–2003. Technovation 26(5–6), 644–64. doi: 10.1016/j.technovation.2005.06.016
- Blechinger, D, Kleinknecht, A, Licht, G & Pfeiffer, F, 1998. The impact of innovation on employment in Europe-an analysis using cis data. ZEW-Dokumentation, 98–02, Mannheim.
- Boyer, R, 1988, December. Assessing the impact of R&D on employment: Puzzle or consensus?. International Conference on New Technology: Its Impacts on Labor Markets and the Employment System, December (pp. 5–7), Berlin.
- Brouwer, E, Kleinknecht, A & Reijnen, JO, 1993. Employment growth and innovation at the firm level. Journal of Evolutionary Economics 3(2), 153–9. doi: 10.1007/BF01213832
- Bryson, J, Rubalcaba, L & Strom, P, 2012. Services, innovation, employment and organisation: Research gaps and challenges for the next decade. The Service Industries Journal 32(4), 641–55. doi: 10.1080/02642069.2011.596531
- Calvino, F, 2019. Technological innovation and the distribution of employment growth: A firm-level analysis. Industrial and Corporate Change 28(1), 177–202. doi: 10.1093/icc/dty003
- Calvino, F & Virgillito, ME, 2018. The innovation-employment nexus: A critical survey of theory and empirics. Journal of Economic Surveys 32(1), 83–117. doi: 10.1111/joes.12190
- Chennells, L, & Van Reenen, J, 2002. Technical change and the structure of employment and wages: A survey of the microeconometric evidence. Productivity, Inequality and the Digital Economy, MIT Press, Cambridge, MA, 175-223.
- Crespi, G, Tacsir, E & Pereira, M, 2019. Effects of innovation on employment in Latin America. Industrial and Corporate Change 28(1), 139–59. doi: 10.1093/icc/dty062
- Dachs, B & Peters, B, 2014. Innovation, employment growth, and foreign ownership of firms: A European perspective. Research Policy 43(1), 214–32. doi: 10.1016/j.respol.2013.08.001
- D’Attoma, I & Pacei, S, 2018. Evaluating the effects of product innovation on the performance of European firms by using the generalised propensity score. German Economic Review 19(1), 94–112. doi: 10.1111/geer.12122
- Djellal, F & Gallouj, F, 2007. Innovation and employment effects in services: A review of the literature and an agenda for research. The Service Industries Journal 27(3), 193–214. doi: 10.1080/02642060701206959
- EuroStat, 2006. Community Innovation Survey (CIS). http://ec.europa.eu/eurostat/web/microdata/community-innovation-survey.
- Falk, M, 1999. Technological innovations and the expected demand for skilled labour at the firm level (Tech. Rep.). ZEW Discussion Papers.
- Griffith, R, Huergo, E, Mairesse, J & Peters, B, 2006. Innovation and productivity across four European countries. Oxford Review of Economic Policy 22(4), 483–98. doi: 10.1093/oxrep/grj028
- Harrison, R, Jaumandreu, J, Mairesse, J & Peters, B, 2005. Does Innovation Stimulate Employment? A Firm-Level Analysis Using Comparable Micro Data on Four European Countries. MPRA Discussion Paper 1245. Munich.
- Harrison, R, Jaumandreu, J, Mairesse, J & Peters, B, 2008. Does Innovation Stimulate Employment? A Firm-level Analysis Using Comparable Microdata from Four European Countries. NBER Working Papers, 14216. Cambridge MA: NBER.
- Harrison, R, Jaumandreu, J, Mairesse, J & Peters, B, 2014. Does innovation stimulate employment? A firm-level analysis using comparable micro-data from four European countries. International Journal of Industrial Organization 35, 29–43. doi: 10.1016/j.ijindorg.2014.06.001
- Human Sciences Research Council, South African National Innovation Survey (INNOV) 2010–2012: All provinces. [Data set]. INNOV 2010–2012. Version 1.0. Pretoria South Africa: Human Sciences Research Council, Department of Science and Technology [producers] 2012. Human Sciences Research Council [distributor] 2017. https://doi.org/10.14749/1487667112.
- Hou, J, Huang, C, Licht, G, Mairesse, J, Mohnen, P, Mulkay, B, … Zhen, F, 2019. Does innovation stimulate employment? Evidence from China, France, Germany, and the Netherlands. Industrial and Corporate Change 28(1), 109–21. doi: 10.1093/icc/dty065
- Jaumandreu, J, 2003. Does innovation spur employment? A firm-level analysis using Spanish cis data. Universidad Carlos III de Madrid 17(4), 813–39.
- Lööf, H & Heshmati, A, 2006. On the relationship between innovation and performance: A sensitivity analysis. Economics of Innovation and New Technology 15(4–5), 317–44. doi: 10.1080/10438590500512810
- Mairesse, J & Wu, Y, 2019. Impacts of innovation, export, and other factors on firm employment growth in Chinese manufacturing industries. Industrial and Corporate Change 28(1), 123–38. doi: 10.1093/icc/dty066
- Mairesse, J, & Mohnen, P, 2010. Using innovation surveys for econometric analysis. In B H Hall & N Rosenberg (Eds.). Handbook of the economics of innovation (pp. 1129–1155). Amsterdam, Holland: Elsevier. https://doi.org/10.1016/S0169-7218(10)02010-1
- Mitra, A & Jha, AK, 2015. Innovation and employment: A firm level study of Indian industries. Eurasian Business Review 5, 45–71. https://doi.org/10.1007/s40821-015-0015-3
- OECD, 2018. Unemployment rate (indicator). https://doi.org/10.1787/997c8750-en. https://data.oecd.org/unemp/unemployment-rate.htm. Accessed 5 September 2018.
- OECD, Eurostat, 2005. Oslo manual: Proposed guidelines for collecting and interpreting technological innovation data. OECD Publishing, Paris.
- Peters, B, 2004. Employment effects of different innovation activities: Microeconometric evidence. ZEW Discussion Paper 04-73, Mannheim.
- Peters, B, 2008. Innovation and firm performance: An empirical investigation for German firms (Vol. 38). Springer Science & Business Media.
- Pianta, M, 2003. Innovation and employment. In The Oxford handbook of innovation.
- Rodrıguez-Pose, A & Crescenzi, R, 2008. Research and development, spillovers, innovation systems, and the genesis of regional growth in Europe. Regional Studies 42(1), 51–67. doi: 10.1080/00343400701654186
- South African Government, 2012. National Development Plan 2030 (n.d.). https://www.gov.za/issues/national-development-plan-2030.
- Spiezia, V & Vivarelli, M, 2002. Innovation and employment: A critical survey. In N Greenan, Y L’Horty & J Mairesse (Eds.), Productivity, inequality and the digital economy: A Transatlantic Perspective. MIT Press, Cambridge, MA, 101–31.
- STATS SA, 2017. Quarterly employment statistics. http://www.statssa.gov.za/?p=10530.
- Ugur, M, Awaworyi Churchill, S & Solomon, E, 2018. Technological innovation and employment in derived labour demand models: A hierarchical meta-regression analysis. Journal of Economic Surveys 32(1), 50–82. doi: 10.1111/joes.12187
- Vernon, R, 1979. The product cycle hypothesis in a new international environment. Oxford Bulletin of Economics and Statistics 41(4), 255–67. doi: 10.1111/j.1468-0084.1979.mp41004002.x
- Vernon, R, 1992. International investment and international trade in the product cycle. In John M. Letiche (Ed.), International economic policies and their theoretical foundations. second edition (pp. 415–35). Elsevier. https://doi.org/10.1016/B978-0-12-444281-8.50024-6.
- Vivarelli, M, 2014. Innovation, employment and skills in advanced and developing countries: A survey of economic literature. Journal of Economic Issues 48(1), 123–54. doi: 10.2753/JEI0021-3624480106
- World Bank, 2018. Data Bank Microdata Data Catalog: Upper middle income. https://data.worldbank.org/income-level/upper-middle-income. Accessed 15 April 2018
- Yu, D, 2017, September. The lesser known and scarier facts about unemployment in South Africa. https://mg.co.za/article/2017-09-04-the-lesser-known-and-scarier-facts-about-unemployment-in-South-Africa. Accessed 4 September 2017.