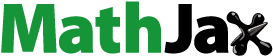
ABSTRACT
We investigate the impact of household air pollution on household health outcomes using the Uganda National Household Survey (2017) and employ a probit model with selection. Results reveal that exposure to household air pollution is significantly associated with cough and fever incidences among household members, with the young ones being more vulnerable. Also, low education level of household head, cooking indoors in a living house, and poverty significantly increases chances of contracting cough and fever. Also, findings show that having a separate indoor built kitchen, high education, being male, residing in urban areas and staying in the northern and western regions significantly reduce the likelihood of contracting cough and fever. Thus, strategies for behavioural interventions such as promoting outdoor cooking and raising awareness of the health hazards associated with household air pollution, good household planning and adoption of clean fuel could be a promising path for policy makers.
1. Introduction
The use of biomass based fuels leads to release of many harmful pollutants and inhalation of these pollutants has led to household members to contract diseases such as respiratory diseases, chronic obstructive pulmonary disease (COPD), lung cancer, asthma, breathing difficulties and wheezing, reduced lung function, stinging eyes, sinus problems, and low birth-weight babies among household members (Smith & Mehta, Citation2003; WHO, Citation2006; Tasleem et al., Citation2007; Kleimola et al., Citation2015). Globally, 93% of all children live in environments with air pollution levels above the WHO guidelines (WHO, Citation2018). In 2016, a total of 2.45 billion people (33.7% of the global population) were exposed to household air pollution and Africa had over 90% of its populations exposed to household air pollution from the use of solid fuels. Household air pollution was responsible for 2.6 million deaths and is ranked 8th in risk factors for early death (WHO, Citation2017). Although representing 4.7% of all deaths globally, household air pollution exposure accounts for substantially greater percentages of the worldwide mortality due to ischaemic heart disease, stroke, lung cancer (Global Health Observatory, Citation2018; WHO, Citation2018). Also, Lelieveld et al. (Citation2015) show that outdoor pollution contributes to 3.3 million premature deaths worldwide per year and it is estimated that the number of deaths will double by 2050 if the issue remains unattended. On the other hand, in 2015, around 4.5 million people died prematurely from diseases attributed to air pollution, including 237,000 children under the age of five from respiratory infections (WHO, Citation2016a). In comparison, 87,000 children died from HIV/AIDS, 525,000 from diarrhoea and 312,000 from malaria in the same year.
Sub-Saharan Africa (SSA) has the highest exposure to air pollution due to widespread use of polluting biomass fuels and technologies by about 80% of its population for basic daily needs, such as cooking, heating and lighting (WHO, Citation2007, Citation2009, Citation2014; Kleimola et al., Citation2015). Consequently there is a high child death (10%) in SSA due to air pollution. Thus, exposure to biomass smoke is a very serious issue and can be considered as a major public health hazard and globally millions of people who use biomass fuels are at a high risk. Other pollution exposure diseases include tuberculosis, perennial mortality, low birth weights and eye irritation and contract (Kleimola et al., Citation2015). Perceiving the harm that these pollutants cause to human beings upon prolonged exposure to these pollutants are named as ‘silent killers’ (WHO, Citation2006; Lelieveld et al., Citation2015) as they slowly damage the health over a long period of time.
Despite the great strides the government of Uganda has made in its attempt to meet the targets set in the National Development Plan II and the Millennium Development Goals by 2030, huge challenges still remain in terms of social-economic indicators. With 63% of an estimated 16,000 child death attributed to lower respiratory infections are from household air pollution (WHO, Citation2012), this means that Uganda cannot attain the Sustainable Development Goal 3 (SDG3), which aims at reducing illness and morbidity from hazardous chemicals and air pollution (UN, Citation2016). However, there is consensus that clean energy is one of the most important means to improve the quality of life and that increased access to affordable and reliable clean energy is an integral component of social and economic development, as it leads to a better use of the available resources and to realise potential development both at individual and societal levels, reduces poverty, and promotes human health and independence (Bernard, Citation2012).
Kampa & Castanas (Citation2007) and Kalpana et al. (2006) show that HAP exposure at individual level may be mostly directly influenced by the surrounding environment such as type of fuel and room dimensions in which the fireplace is placed. However, many factors such as income, climate conditions, cooking habits and family size may indirectly influence the type of fuels/stove (Parikh, Citation2011; World Bank, Citation2014; WHO, Citation2016a), and therefore contribute to individual HAP exposure indirectly. Therefore, determinants of exposure to pollution can be described by classifying them broadly into ‘proximal’ determinants that are directly in the pathway and ‘distal’ determinants that contribute to differences in exposure through their effects on the systems that each of the proximal determinants may represent (Kalpana et al., 2006; Bansal et al., Citation2014).
Also, household income and education can be expected to affect family size and type of housing that in turn affect biomass fuel quantities or the number of rooms and/or location of the kitchen. In addition, household layout in terms of living space and the kitchen, household ventilation, time-activity profiles of individual household members and cooking practices and fuels used have been shown to influence pollution levels and individual exposure to them (Kampa & Castanas, Citation2007; WHO, Citation2016a; Joon et al., Citation2009). Cultural habits and residential status may influence cooking practices which in turn may affect duration of cooking or the quantity of fuel used (WHO, Citation2016a). Uganda like other developing countries with low gross domestic product per capita, experience great gender inequalities and variation in terms of income, education, access to healthcare, social position and cooking practices (GoU, Citation2010; WHO, Citation2016b; UBoS, Citation2017). Joon et al. (Citation2009) note fuel cost, availability, occupational risks and service quality potentially affect household pollution exposures especially of vulnerable groups, such as the poor, women and children.
Other factors at play that increase HAP such as exposure to tobacco smoke in the household may also contribute to an extra serious health consequences such as acute chronic respiratory illness and death (Charoenca et al., Citation2013; Sigaud et al., Citation2016). Also, household garbage disposal through burning is a big cause of smoke in the household environment that contributes to HAP and associated risk. Also, macroeconomic factors (fuel prices and their variability) and infrastructure (reliability of supply of any specific form of energy) influence the choice of household energy sources and energy use behaviours (Foster et al., Citation2000; Kampa & Castanas, Citation2007). Also, households’ own generated HAP from use of biomass fuels, households share pollution from other people’s fuel use, burning of solid waste, traffic, and industrial emissions, as much as or more than their own exposure in own residents.
About 83% of Uganda’s 37 million people (Worldometers, Citation2018) live in rural household, most of whom depend on solid fuels for the bulk of their household’s energy needs. In Uganda, most people still use rudimentary technology of cooking/heating/lighting which requires one to use biomass fuels like dried and baked animal waste, wood, dried twigs etc as inputs into a three stone traditional stove and keeps blowing air into the stove until the fire catches. Notably, as the biomass burns, smoke is emitted continuously and the person attending to the cooking/heating/lighting fireplace must continue to stay in the same place/premises as is required to over the fire as they adjust the wood, waste content, and twigs (UBoS, Citation2017). Household members are exposure to biomass emission/air pollution because, even though the fire catches below the cooking dish, it needs to be fanned frequently to regulate the fire and monitor the burning process lest the cooking/heating/lighting is done (Valentina, Citation2008; Nabulo & Cole, Citation2011). Besides, if the fireplace is located within residential premises, this exposes all household members to smoke emitted from the fireplace (Nabulo & Cole, Citation2011). However, in energy poor countries like Uganda, most women and little children always hang around the fireplace taking care of the household cooking/heating/lighting and this exposes women and children to a high risk of HAP related diseases. In Uganda, pollution related diseases including upper and lower respiratory account for the second highest incidence of reported health issues after malaria in Uganda (GoU, Citation2010; Nabulo & Cole, Citation2011; UBoS, Citation2017).
Household air pollution (HAP) remains a major public health concern in low and middle-income countries, although clean energy is a commodity that is vital for the existence of modern life (Eakins, Citation2013; IEA, Citation2014) and constitutes one of the most aspects of human life. Thus, this article provides answers to the following pertinent questions. First, controlling for the household socioeconomic characteristics, does household air pollution affect household health outcomes in Uganda? If so, is there discernible difference for using different types of fuels? Second, is there any difference in the effect of household air pollution on household health between rural and urban areas? This implies that understanding the effect of HAP per se on household health outcomes is not enough, the effect of the differential fuel types can provide more meaningful insights. We hypothesise that household air pollution negatives influence household health outcomes in terms of fever and cough incidences.
There have been few studies from Uganda investigating the association between HAP and the health of young children, with most limited to a particular health outcome such as low birth weight, under-five mortality, indoor pollution and respiratory illness and factors that determine choice of fuel sources (Mwaura et al., Citation2014; Lwiza et al., Citation2017). Previous studies used ordinary least squares techniques and probit to estimate factors that determine choice of energy sources, under-five mortality rates among. This study improves on the previous work on Uganda by employing sample selection probit model, taking into account the role of potential environmental and behavioural factors that might affect the level of exposure to HAP from cooking, heating and lighting fuels. It is important to consider the issue of sample selection bias in this type of study, unless all households included use same types of fuels and experience unilaterally the same sickness. Whether household members can fall sick due to HAP depends on an individual’s self-selection, that is, whether any given household uses polluting fuels for cooking, heating and lighting in a given household environment. Generally, sample selection arises if unobservable factors such as household’s preference influence the selection process for the type of fuel used and the subsequent exposure to HAP. Thus, we identify households with members who fell sick either using polluting fuels or non-polluting fuels. The results show that household cooking practices and more so where the cooking is done inside on open fire has a large effect on health outcomes and explains a large part of health outcomes in the rural and urban areas, such that rural residents are more likely to fall sick in households using open fire cooking and this affects women more greatly. The effect of background factors such residence, education level and sex of the parents, type of tenure, age of the child and gender have health improving effects for the household members.
Our results reveal the compelling need to know health risks associated with the use of the biomass fuels, so that with proper use, major implementations of effective preventive measures and new technology may reasonably provide the intended benefits for improved household health and overall household environmental conditions. Thus, addressing the key health risks related to HAP and its reduction will provide critical new information that can inform large scale implementation programmes to encourage use of clean fuels by households living in energy poverty, such that diseases are prevented, a healthier lifestyle is promoted, and increase household productivity and overall economic growth in the country. Essentially, our findings will help policy makers better understand behaviour of the energy poor households regarding fuel choice for domestic use and guide them in designing policies to ensure sustainable and efficient supply of clean energy in the country.
Section two reviews the selected literature. The data and empirical strategy are described in section three. Section four presents and discusses the study findings, while section five presents the concluding remarks.
2. Selected literature
There is a large theoretical and empirical knowledge on the relationship between household air pollution and human health risk. Evidence shows that burning biomass fuels such as firewood\grass\shrubs\animal dung and charcoal produces poisonous smoke which is a major cause of acute chronic respiratory diseases, burning of eyes, eye disease, low birthweight in the new born and infant mortality and death worldwide (Janke et al., Citation2009; Kashima et al., Citation2010; Ezeh et al., Citation2014). The socioeconomic characteristics (mother’s and father’ education, gender of household head, wealth status, and mother’s and father’s employment) determine the type of fuel the household uses and this influence the level of household pollution (Wichmann & Voyi, Citation2006; Joon et al., Citation2009; Rehfuess et al., Citation2009; Kashima et al., Citation2010; Ezeh et al., Citation2014; Garland et al., Citation2015; Naz et al., Citation2015, Citation2016). In addition, cultural factors (traditions and race) that allow family members to sit around the fire for long, keep women responsible for cooking, location of kitchen in the household, type of food eaten most etc determine how long cooking takes and who is more vulnerable to household pollution (Ezeh et al., Citation2014; Perlroth & Castelo Branco, Citation2017). In addition, geographical location of the household (urban or rural, mountainous or valleys or cold or warm region) influence type of fuel and length of time fuel burning takes place, which determine the degree of exposure to pollution (Chay & Greenstone, Citation2003; Kleimola et al., Citation2015). Furthermore, evidence shows that energy poverty is one cause of use of highly pollutant biomass fuels-firewood, twigs/grass and animal dung that cause great risk and permanent damage to human life and greater risk of death (Khandker et al., Citation2012; Ezeh et al., Citation2014; Kleimola et al., Citation2015; Perlroth & Castelo Branco, Citation2017). Poverty influence choice of fuel to use (Ekholm et al., Citation2010) and many people are developing countries are trapped in energy poverty that has serious health effects (Baines et al., Citation2011). In Uganda, about 91% of the total energy consumption is generated through biomass, consisting of firewood (78.6%), charcoal (5.6%) and crop residues (4.7%). Biomass fuel use goes up to 93% in rural areas and is 58% in urban areas (UBoS, Citation2017).
3. Data and empirical strategy
3.1. Data
The data for the analysis were drawn from Uganda National Household Survey (UNHS), which provides information at the individual, household, and community levels (UBoS, Citation2017). The household module provides information on socioeconomic indicators including residence (urban or rural), household wealth index (very poor, poor, none-poor rich and very rich), parents’ education levels, mothers and fathers working status, smoking status of parents, floor material of household (cement/carpet, or earth/sand), and wall material of household (cement/brick or non-cement/non-brick), household size, age of household members, sex and relationship to the household head, economic indicators (assets, income and expenditure), health status and energy used. Specifically, respondents were asked what type of fuels they use for cooking, heating and lighting. Also, respondents were asked whether a household member fell sick in the last 6 month or 30 days, the symptoms diagnosed, the type of sick and whether one sought medical treatment from a private or government health facility. In addition, the survey collected information on the household’s environment conditions such as location of household’s kitchen (located in the house, outdoor, or in a separate building), and the form of garbage disposal by the household (pit, garden, commercial, burning and use of waste bags). The survey covered 74,613 households with 23,560 individuals. This sample is large enough to provide reliable estimates at individual-level that can be used to draw meaningful conclusions and recommendations.
In this article, household air pollution sickness is measured by incidence of reported health outcomes-fever and cough. The types of fuels used for cooking, heating and lighting fuels were the main exposure variable in this study. For the empirical analysis, these fuels were first grouped into two broad categories on the basis of exposure to HAP, that is ‘clean fuels’ (electricity, liquid petroleum gas (LPG), natural gas and biogas), and polluting fuels (kerosene, charcoal, wood, straw/shrubs/grass and animal dung). Kerosene was categorised in the polluting fuels group in this study as some previous studies on HAP have reported kerosene as a polluting fuel and have shown significant association between respiratory illness and use of kerosene fuel in the household.
3.2. Empirical strategy
The association between exposure to household pollution that is caused by poisonous smoke from cooking, heating and lighting fuels and the disease incidence among household members was investigated using a two-stage analysis. First, the descriptive evidence of household members’ exposure to smoke from the different fuel sources is undertaken using both graphical and statistical mean difference to show the difference in exposures due to the different fuels. Secondly, the empirical probit model with sample selection is estimated to establish the causal effect associated to the different covariates included in the model and the associated marginal effects that indicate the likelihood of getting a given disease given that someone fell sick in a given household are reported.
The empirical binary probit with sample selection and two stages probit (Heckman, Citation1974, Citation1979; Buyinza et al., Citation2018) is generally motivated by reference to latent (or unobservable) dependent variable. The model is expressed as a linear function of a set of explanatory variables as in the following expression:
(1)
(1)
From Equation (1), denotes health status of HAP related sickness binary dependent variable coded as ‘1’ if a household member suffered from HAP related sickness and ‘0’ otherwise.
is a set of explanatory variables including a constant for individual
and β is a column vector of unknown parameters to be estimated, while
is assumed to be normally distributed error term, such that,
.
Basically, the values of the latent dependent variable are measured on the real line and in this study it reflects the underlying propensity that a household member suffered from HAP related sickness or not. In this case, a threshold is assumed that is used to delineate whether an individual in a given household suffered from HAP related sickness such that
or not for
. Thus, the probability that household member
suffered from HAP related sickness occurs can be linked to the latent dependent variables by the following expression:
(2)
(2)
From Equation (2), is the dichotomous realisation of the latent dependent variable that coded as ‘1’ when a household member suffered from HAP related sickness and ‘0’ otherwise. In addition,
denotes the cumulative distribution function operator for the standard normal distribution in which it is expressed as
.
From Equation (1), given that if
and
are normally distributed with mean zero and constant variance, then assume that
and
, this implies that estimates of Equation (1) using simple probit will yield biased results. Hence, in order to obtain unbiased results, Equation (1) is estimated as a probit model with a sample selection. In our empirical specification,
when member in a given household fell sick from HAP related sickness (fever or cough) only and
when no member contracted an HAP related sickness. Thus, selection Equation (2) gives the likelihood that an individual
from given household fell sick from HAP related sickness. We assume that a household member’s sickness from the HAP related sickness is influenced by household’s socioeconomic characteristics, environment and the HAP. Therefore, estimation of the outcome Equation (1) gives the factors that affects the health status of a household member in terms of HAP related sickness (fever or cough).
The parameters are estimated using conventional nonlinear optimisation algorithm. The efficient score tests suggested by are undertaken to assess the reported specifications in terms of homoscedastic errors, and a normal distribution of the generalised residuals. In addition, the appropriate functional form and/or omitted variables are tested using RESET framework. Also, weights are used to ensure robust estimates. The omitted variables or miss-specified functional form are proxied by the predicted standardised probit from the original probit regression model raised to the powers of two, three and four.
The estimated probit coefficients can be interpreted by reference to their effect on the standardised probit index but it is generally more convenient to translate them into marginal and impact effects.
From Equation (1), the dependent variable is the probability that a household member in a given household fell sick from HAP related sickness (fever or cough) and, on conditional illness of the individual member, the probability of falling sick from Hap related sickness is computed for the individual household member who fell sick from HAP related sickness defined by the outcome equation. The marginal effects for the outcome equation are reported. The estimated marginal effects will show whether each independent variable causes either an increase of decrease in the likelihood of contracting HAP related sickness on condition that a household member in a given household fell sick.
4. Results
4.1. Descriptive results
presents household’s fuel sources used by education level in both rural and urban areas. In Panel (a), its observed that use of electricity greatly vary by education level in both rural and urban areas with households headed by individuals with postsecondary education using electricity more than for any other education levels. In panel (b), use of paraffin/kerosene by households is also skewed towards households headed by individuals with post-secondary education, but paraffin use is more pronounced among urban dwellers. In panel (c), use of charcoal is more pronounced among urban dwellers (81%) among households headed by individuals with postsecondary education. As expected, panel (d) shows that use of firewood fuel is more dominant among rural dwellers and is high as 98% in rural areas and 66% in urban areas among households headed by no education.
represents the household fuel sources by household wealth index and area of residence. Panel (a) indicates that electricity fuel is mainly used by rich urban residents, while very few rural households use electricity source of fuel. Panel (b) shows that urban and the richer household use paraffin as household fuels. In panel (c), use of charcoal is mainly used by urban households than rural households and more by the rich households. In panel (d) poorest rural households dominantly use firewood/grass/animal dung as source of household fuel.
represents households’ fuel sources by tenure in rural and urban areas. Panel (a) indicates that electricity is mainly used by urban residents those in given and rented households, although few rural households use electricity in rented and given houses. In panel (b), we observe that urban households staying in given and rented houses use paraffin as household fuels more than their rural counterparts. Panel (c) shows that more urban households use charcoal than their rural counterparts. Interestingly, panel (d) shows that use of firewood fuels by rural households is dominant by households staying in owned houses.
4.2. Empirical results
presents the marginal effects from a two stage Heckman probit model controlling for the household characteristics and household environment for the variant of estimated model of HAP on household health outcomes. Two of the four model estimated are based on classification of household fuel into clean (electricity and gas) and polluting fuels (paraffin, firewood, charcoal, grass and animal dung) for both cough and fever. The second set of models estimated are based on a wide classification of household fuels (electricity, paraffin, charcoal and firewood). Overall, the Wald test of independent equations rejects the null hypothesis (H0: ρ = 0), validating the estimated model specification. Second, the Chi-square test for the estimated models could not be rejected that the selected variable are significant.
Table 1. Marginal effects for household sickness from HAP related sickness (cough and fever).
column 3 results show that the marginal effect for using polluting fuel is positive and statistically significant at the conventional levels. The findings indicate that using polluting fuels increases the chances of individual members in that household to suffer from cough by 2% compared to their counterparts in households using non-polluting fuels. Also, columns 2 and 4 show that using firewood/grass/animal dung fuels increase chances of contracting cough by 5% and fever by 7% among household members compared to individuals in households using electricity. However, , column 4 results show that using paraffin significantly reduces the likelihood of getting fever among households by about 13%. Our results are consistent with previous findings (Ezzati & Kammen, Citation2001; Ezeh et al., Citation2014; Kleimola et al., Citation2015) who find that using biomass fuels causes various pollution related diseases to the household members.
In addition, the type of household’ kitchen used is a significant factors in exposure to biomass pollution and the subsequent effect on health of the household members. Our results show that with highly significant estimates, cooking in an indoor separate built kitchen is associated with a decrease in suffering from cough by 2% and between 1% and 5% for fever compared to cooking outdoors. But cooking inside the living house anywhere increases the likelihood of suffering from fever between 4% and 6% compared to those living in households that cook from outdoor. This finding is line with other authors (Smith & Mehta, Citation2003; Gajate-Garrido, Citation2013). This could be attributed to the poor house ventilation and high exposure to pollution for longer hours by household members.
Results in , columns 1 and 2 show that household members are less likely to contract cough by 2% in households headed by persons with postsecondary education compared counterparts living in households headed by a person with no education. In column 3 the results show that having postsecondary education reduces contracting fever by 8% and in column 4 the results show that living in households headed by individuals with primary, secondary and postsecondary education reduces the probability of contracting fever by 3%, 5% and 8% respectively, compared to individuals living in households headed by individuals with no education. This finding shows the importance of education for the socioeconomic development at individual and national levels as noted by previous studies (Graff et al., Citation2011; Smith et al., Citation2011).
Previous studies (Smith et al., Citation2011; Sigaud et al., Citation2016; Perlroth & Castelo Branco, Citation2017) show that age of an individual is an important determinant of exposure to the risk and effect of Hap on one’s health. Results () column 1 indicates that lower ages increase the probability of contracting HAP related sicknesses. The results show that household members aged 0–4 years and 5–12 years are more likely to contract cough by 15% and 16% and fever by 5% and 19% compared to members aged 36 and above. For individual members aged 6–12 years, the marginal effects show a likelihood to contract cough between 4% and 5% and fever by 4% compared to members aged 36 years and above. However, members aged 13–24 and 25–35 years show a significant reduction in the probability of contracting cough by 2% and 3%, respectively. Also, the marginal effects show that members aged 13–24 and 25–35 years reduce the probability of contracting fever by 9% and 4% respectively, compared to counterparts aged 36 years and above.
In addition, the marginal effects show that being male has a significant negative impact on contracting HAP sickness and this reduces the probability of a male member contracting cough by 2% and fever by 6% compared to their female counterparts. This finding is in line with findings of previous studies (Po et al., Citation2011; Naz et al., Citation2015), who note that women are more vulnerable to HAP as they do most of the domestic chores of lighting fire, cooking and house warming and usually continue to live with persistent illness that makes them weak, less productive and make them live a useless life especially in rural areas.
Evidence by Janke et al. (Citation2009) and Jetter & Kariher (Citation2009) show that economic status of the household significantly influences the probability of exposure to HAP sicknesses in Uganda. columns 1 and 2 show that living in poor and very poor households increases the probability of contracting cough by 3% compared to counterparts living in very rich households. Also, the marginal effect in columns 3 and 4 show that living in a non-poor, poor and very poor household increases the probability of contracting fever by 4%, between 4% and 7%, and between 3% and 4%, respectively compared to counterparts living in very rich households. This finding is in line with previous studies (Mwaura et al., Citation2014) who show that energy ladder use from polluting to clean energy as one’s income increase.
Interestingly, the marginal effects on household size provide a new finding as opposed to the reviewed literature that has documented an increasing effect of household size on HAP exposure. The interpretation of this finding is that, in households with many individuals, there is a likelihood of division of labour that implicitly might reduce on one’s time of exposure to biomass fuel pollution. This consequently might reduce on the chances of contracting HAP related sickness.
Furthermore, residence of household has a positive significant effect on the probability of contracting fever. The results show that staying in rented houses would increase the probability of contracting fever by 3%, compared to counterparts living in given free houses. Also, the marginal effect shows that living with smoking household members increases the probability of contracting cough by 4% and fever by 10% compared to counterparts living with non-smoking members. This finding is in line with authors (Charoenca et al., Citation2013; Sigaud et al., Citation2016). In addition, the results show that sharing toilets with other households increase the probability of contracting fever between 3% and 5%, compared to members living in households that do not share toilet facilities. This finding is in line with Mishra et al. (Citation2005) who note that overall household environment is pertinent to human health status. In addition, the results show that households that dispose garbage through burning increase the probability of household members contracting cough between 3% and 4% compared to those in households where garbage is disposed through pit, gardens and commercially. This finding is in line with previous authors (Perlroth & Castelo, Citation2017; Kampa et al., Citation2007) who note that overall household environment is important for good health of the household.
Also, marginal effect results show that living in urban area reduces the chances of contracting cough by between 1% and 2% and fever by 4% compared to counterparts living in rural areas. This means that Ugandan urban dwellers have a greater access and use of less pollutant fuels (electricity, gas and paraffin) (UBoS, Citation2017), which than their rural counterparts. In addition, the marginal effects reveal that living in the Eastern region significantly increases the probability of contracting fever by between 7% and 12% compared to counterpart living in the Central region. However, the marginal effects show that living in the Northern region reduces the probability of contracting cough by 1% and fever by 2% compared to counterparts living in the Central region. Also, the results show that living in the Western region reduces chances of contracting cough by 5%, and fever by between 9% and 21% compared to counterparts living in the Central region. This finding is in line with previous findings by Chay & Greenstone (Citation2003) who find that climatological variations have an impact on HAP exposure.
Also, the marital status of household head has a significant effect on HAP related sicknesses among household members. The marginal effects indicate that living in households headed by married and divorced individuals increase chances of contracting fever by 6% and 9% respectively, compared to individuals living in households of the never married heads. This could be attributed to the large family size that is associated with married holds and the divorced families.
displays a visual depiction of the relationship between estimates in changes in probabilities of contracting cough and fever for age 0–4, 5–12, 13–24, 25–35 and 36 and above for both female and male household members. indicates a change in the probability of cough and fever with a unit change in age in alternative scenarios of using clean and polluting fuels after holding all other covariates constant. Panels I and II indicate that probabilities of contracting cough and fever expectedly decline with increasing age. However, the risk to contract cough and fever is relatively higher for the lower age 0–4 and 5–12 years. The distributions also indicate that as the age of the child advances, the gap between the probabilities of contracting cough and fever for inhabiting household members is lower among male than their female counterparts. Panel II, based on the fever incidences enables us to see more clearly that using polluting fuels plays a more significant role in predicting fever infections among female and male household members.
5. Conclusion
The article used data drawn from Uganda National Household Survey (2016/2017) to examine the impact of HAP on health outcome of household members in Uganda taking into account the socioeconomic and demographic characteristics of the household. The results reveal that exposure to household air pollution due to use of polluting fuels is significantly associated with cough and fever incidences among household members, with members aged 0–4 years and 5–12 years more vulnerable compared to those aged 13 years and above. Also, low education level of household head, cooking inside the living house, smoking, sharing toilets among households and burning garbage and poverty among households significantly increase the probability of contracting HAP diseases. On the other hand, results show that having a separate indoor built kitchen, high education, being male, residing in urban areas and staying in the Northern and Western regions significantly reduce the likelihood of contracting HAP related diseases.
The findings suggest that efforts for household behavioural interventions, such as promoting outdoor cooking and use of clean fuels, can have a substantial impact on health hazards. Given Uganda’s climate and weather variabilities, not everyone can cook from outside the whole year due to unfavourable seasonal conditions and insecurity issues. Such constraints should be taken into account by government when formulating policy, targeting behavioural interventions in vulnerable communities where there is scope for change from indoor to outdoor cooking to minimise airborne related diseases. Second, our results show the importance for the need to raise awareness of the detrimental effects of smoke emission on households’ health and the benefits of staying away from cooking/heating/fire places, particularly the mothers and the young children who are always in charge of these domestic choirs as opposed to their male counterparts in the household. With measures to step up distribution of clean fuels, in the meantime, remedial effort should concentrate on promoting household behavioural change, with the extreme case of outdoor cooking being an important component. More importantly, a combination of measures targeting clean fuels adoption and low-cost health knowledge interventions may be a more promising path to curb airborne related diseases.
Disclosure statement
No potential conflict of interest was reported by the author(s).
References
- Baines, P, Fill, C, & Page Kelly, 2011. Marketing 2nd edn. US: Oxford University Press.
- Bansal, M, Saini, RP & Katod, DK, 2014. Addressing the burden of disease attributed to air pollution in India: The need to integrate across household and ambient air pollution exposure. Environmental Health Perspective 122(2), 16–44.
- Bernard, T, 2012. Impact analysis of rural electrification projects in sub-Saharan Africa. The World Bank Research Observer 27(1), 33–51.
- Buyinza, F, Tibaingana, A & Mutenyo, J, 2018. Factors affecting access to formal credit by micro and small enterprises in Uganda. Athens Journal of Business & Economics 4(4), 405–24.
- Charoenca, N, Kungskulniti, N, Tipayamongkholgul, M, Sujirarat, D, Lohchindarat, S, Mock, J & Hamann, SL, 2013. Determining the burden of secondhand smoke exposure on the respiratory health of Thai children. Tobacco Induced Diseases 11, 7.
- Chay, KY & Greenstone, M, 2003. The impact of Air pollution on infant mortality: evidence from Geographic variation in pollution Shocks Induced by a Recession. The Quarterly Journal of Economics 118(3), 1121–67.
- Eakins, J, 2013. An analysis of the determinants of household energy expenditures: Empirical evidence from the Irish household budget survey. PhD Thesis. University of Surrey, UK.
- Ekholm, T, Krey, V, Pachauri, S, & Riahi, K, 2010. Determinants of household energy consumption in India. Energy Policy 38, 5696–5707.
- Ezeh, OK, Agho, KE, Dibley, MJ, Hall, JJ & Page, AN, 2014. The effect of solid fuel use on child mortality in Nigeria: Evidence from the 2013 cross-sectional household survey. Environmental Health 13, 1–10.
- Ezzati, M & Kammen, D, 2001. Indoor air pollution from biomass combustion and acute respiratory infections in Kenya: An exposure-response study. The Lancet 358, 619–24.
- Foster, V, Tre, J & Wodon, Q, 2000. Energy prices, energy efficiency, and fuel poverty, Latin America and Caribbean regional studies programme. The World Bank, Washington, D.C.
- Gajate-Garrido, G, 2013. The impact of indoor air pollution on the incidence of life threatening respiratory illnesses: Evidence from young children in Peru. The Journal of Development Studies 49, 500–15.
- Garland, RM, Matooane, M, & Mary-Jane, B, 2015. Regional Projections of Extreme Apparent Temperature Days in Africa and the Related Potential Risk to Human Health. International Journal of Environmental Research and Public Health 12(10), 12577–12604.
- Global Health Observatory (GHO) data, 2018. Household air pollution, burden of disease. World Health Organization, Geneva.
- GoU, 2010. National development Plan 2010/11–2014/15. Government of Uganda, Kampala, Uganda.
- Graff, Z, Joshua, S & Mathew, JN, 2011. The impact of pollution on worker productivity. NBER Working Paper #17004.
- Heckman, J, 1974. The common structure of statistical models of truncation, sample selection and limited dependent variable and simple estimator for such models. Annals of Economic and Social Measurement 5, 475–92.
- IEA, 2014. Renewable energy medium-term market report. IEA (2014). Available from: https://www.iea.org/Textbase/npsum/MTrenew2014sum.pdf.
- Heckman, JJ, 1979. Sample selection bias as a specification error. Econometrica 47(1), 153–61.
- Janke, K, Propper, C & Henderson, J, 2009. Do current levels of air pollution kill? The impact of air pollution on population mortality in England. Health Economics 18(9), 1031–55.
- Jetter, JJ & Kariher, P, 2009. Solid-fuel household cook stoves: Characterization of performance and emissions. Biomass and Bioenergy 33, 294–305.
- Joon, V, Chandra, A & Bhattacharya, M, 2009. Household energy consumption pattern and socio-cultural dimensions associated with it: A case study of rural Haryana, India. Biomass and Bioenergy 33, 1509–12.
- Kampa, M, & Castanas, E, 2007. Human health effects of air pollution. Environ. Pollut 1–6.
- Khandker, SR, Barnes, DF, & Samad, HA, Rubaba, A, & Banrnes, DF, 2012. Who benefits most from rural electrification? Evidence in India. Policy research Working Paper Series 6095, The World Bank.
- Kleimola, LB, Patel, AB, Borkar, JA & Hibberd, PL, 2015. Consequences of household air pollution on child survival: evidence from demographic and health surveys in 47 countries. International Journal of Occupational and Environmental Health 21, 294–302.
- Kashima, S, Yorifuji, T, Tsuda, T, Ibrahim, J, 2010. Effects of traffic-related outdoor air pollution on respiratory illness and mortality in children, taking into account indoor air pollution, in Indonesia. Journal of Occupational and Environmental Medicine 52, 340–345.
- Lelieveld, J, Evans, JS, Fnais, M, Giannadaki, D & Pozzer, A, 2015. The contribution of outdoor air pollution sources to premature mortality on a global scale. Nature 525(7569), 367–71.
- Lwiza, F, Mugisha, J, Walekhwa, PN, Smith, J & Balana, B, 2017. Dis-adoption of household biogas technologies in Central Uganda. Energy for Sustainable Development 37, 124–32. http://www.sciencedirect.com/science/article/pii/S0973082617300145.
- Mishra, V, Retherford, RD & Smith, KR, 2005. Cooking and Tobacco Smoke as Risk Factors for Still Birth. International Journal of Environmental Health Research 15(6), 397–410.
- Mwaura, F, Okoboi, G & Ahabwe, G, 2014. Determinants of households choice of cooking energy in Uganda. Economic Policy Research Centre, Research Paper Series N.114.
- Nabulo, G & Cole, D, 2011. Uganda: Environmental health concerns. In Encyclopedia of environmental health, 439–51
- Naz, S, Page, A & Agho, KE, 2015. Household air pollution and under-five mortality in Bangladesh (2004–2011). International Journal of Environmental Research and Public Health 12, 12847–62.
- Naz, S, Page, A, & Agho, KE, 2016. Household air pollution and under-five mortality in India (1992-2006). Environ Health 15(15), 54.
- Parikh, J, 2011. Hardships and health impacts on women due to traditional cooking fuels: A case study of Himachal Pradesh, India. Energy Policy 39(12), 7587–94.
- Perlroth, NH & Castelo Branco, CW, 2017. Current knowledge of environmental exposure in children during the sensitive developmental periods. Jornal de Pediatria 93, 17–27.
- Po, JY, FitzGerald, JM & Carlsten, C, 2011. Respiratory disease associated with solid biomass fuel exposure in rural women and children: Systematic review and meta-analysis. Thorax 66(3), 232–9.
- Rehfuess, EA, Tzala, L, Best, N, Briggs, DJ, & Joffe, M, 2009. Solid fuel use and cooking practices as a major risk factor for ALRI mortality among African children. Journal of Epidemiol Community Health. Nov 1;63, 887–892.
- Sigaud, C, Castanheira, A & Costa, P, 2016. Association between second-hand smoking in the home and respiratory morbidity in preschool children. Revista da Escola de Enfermagem da USP 50, 562–8.
- Smith, KR, McCracken, JP & Weber, MW, 2011. Effect of reduction in household air pollution on childhood pneumonia in Guatemala (RESPIRE): A randomised controlled trial. The Lancet 378, 1717–26.
- Smith, KR & Mehta, S, 2003. The burden of disease from indoor air pollution in developing countries: Comparison of estimates. International Journal of Hygiene and Environmental Health 206, 279–89.
- Tasleem, A, Zahoor, U, Mir, HK, & Nazli, R. 2007. Chronic bronchitis in women using solid biomass fuel in rural Peshawar, Pakistan. Chest 6(2529), 1472–1476.
- UBoS, 2017. Uganda national household survey. UBoS, Kampala, Uganda.
- UN, 2016. Sustainable development goals. United Nations, New York. http://www.un.org/sustainabledevelopment/ Accessed 3 May 2016.
- Valentina, G, 2008. Impact of appropriate cooking stove technologies on rural women. International Journal of Innovative Research in Technology & Science 2(5), 66–73.
- WHO, 2006. Fuel for life: Household energy and health. WHO, Geneva. 40.
- WHO, 2007. Indoor air pollution-national burden of diseases due to indoor air pollution. WHO, Geneva. http://www.who.int/indoorair/health_impacts/burden_national/en/ Accessed 16 August.
- WHO, 2012. Handbook for integrated vector management. Geneva: World Health Organization. http://apps.who.int/iris/bitstream/10665/44768/1/9789241502801_eng.pdf?ua=1
- WHO, 2009. Quantifying environmental health impacts: Global estimates of diseases caused by the environmental risks, Gevena. www.who.int/quantifying_ehimpacts/global/globalair2004/en/index.html.
- WHO, 2014. Burden of disease from household air pollution for 2012. World Health Organization, Geneva, August 2016.
- WHO, 2016a. Global estimates of burden of disease caused by the environment and occupational risks. World Health Organization. http://www.who.int/quantifying_ehimpacts/global/en/.
- WHO, 2016b. Global health observatory data repository: Population using solid fuels. http://apps.who.int/gho/data/view.main.1701?lang=en.
- WHO, 2017. WHO indoor air quality guidelines: household fuel combustion. WHO, pp. Who.int.
- WHO, 2018. Burden of disease from the joint effects of household and ambient air pollution for 2016. Summary of results. World Health Organization, Geneva.
- Wichmann, J, & Voyi KVV, 2006. Influence of cooking and heating fuel use on 1–59 month old mortality in South Africa. Mater Child Health J. 10, 553–561.
- World Bank, 2014. Household cooking fuel choice and adoption of improved cookstoves in developing countries. A Review Sunil Malla Govinda R Timilsina.
- Worldometers, 2018. World population statistics. Worldometer. www.Worldometers.info.