Abstract
Since the volume of the medical data is increasing due to the presence of vast amount of features, the conventional rule mining technique is not competent to handle the data and to perform precise diagnosis. For instance, this paper intends to implement the improved rule mining technique to overcome the above mentioned limitations. The model comes out with two main contribution stages (i) Using Map Reducing Framework (ii) Classification. Initially, the input medical data is given to map reduce framework. Here, Multi-linear Principal Component Analysis (MPCA) is used for reducing the given bulk data. Then, the reduced data is given to the classification process, where it classifies the disease with high accuracy. For this, this paper uses Support Vector Machine (SVM) classifier. After the completion of implementation, the proposed model compares its performance over other conventional methods like Principal Component Analysis- NN (PCA-NN), Independent Component Analysis- NN (ICA-NN), MPCA-NN, PCA-SVM and ICA-SVM, respectively in terms of performance measures like accuracy, specificity, sensitivity, precision, False Positive Rate (FPR), False Negative Rate (FNR), Net Present Value (NPV), False Discovery Rate (FDR), F1Score, and Mathews Correlation Coefficient (MCC), and the superiority of proposed model is proven over other methods.
Additional information
Notes on contributors
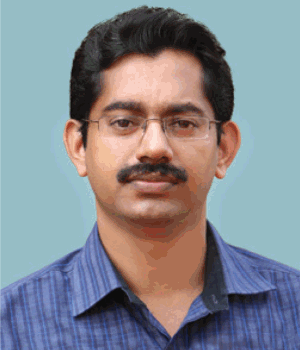
Juby Mathew
Juby Mathew is currently working as research scholar in the School of Computer Sciences, Mahatma Gandhi University Kottayam, Kerala. Corresponding author. Email: [email protected]
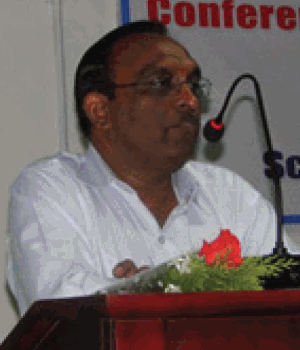
R. Vijaya Kumar
R. Vijaya Kumar is currently working as professor in the School of Computer Sciences, Mahatma Gandhi University, Kottayam, Kerala. Email: [email protected]