ABSTRACT
Diseases is an unusual circumstance that affects single or more parts of a human’s body. Because of lifestyle and patrimonial, different kinds of disease are increasing day by day. Among all those diseases, heart disease turns out to be the most common disease and the impact of this ailment is dangerous than all other diseases. In this paper, we compared a number of computational intelligence techniques for the prediction of coronary artery heart disease. Seven computational intelligence techniques named as Logistic Regression (LR), Support Vector Machine (SVM), Deep Neural Network (DNN), Decision Tree (DT), Naïve Bayes (NB), Random Forest (RF), and K-Nearest Neighbor (K-NN) were applied and a comparative study was drawn. The performance of each technique was evaluated using Statlog and Cleveland heart disease dataset which are retrieved from the UCI machine learning repository database with several evaluation techniques. From the study, it can be carried out that the highest accuracy of 98.15% obtained by deep neural network with sensitivity and precision 98.67% and 98.01% respectively. The outcomes of the study were compared with the outcomes of the state of the art focusing on heart disease prediction that outperforms the previous study.
ACKNOWLEDGEMENT
The authors would like to acknowledge the colleagues and friends who made valuable comments on the study that enhanced the quality of the research.
Additional information
Notes on contributors
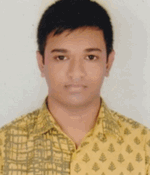
Safial Islam Ayon
Safial Islam Ayon received BSc degree from Computer Science and Engineering (CSE) Department at the Khulna University of Engineering and Technology (KUET), Bangladesh in 2019. He is currently a lecturer of Computer Science Engineering Department at the Bangladesh Army University of Engineering & Technology (BAUET), Natore. His research interests focus on deep neural network, machine learning, embedded systems, and swarm intelligence. Email: [email protected]
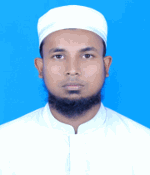
Md. Milon Islam
Md Milon Islam received BSc and MSc degree in computer science engineering (CSE) from the Khulna University of Engineering & Technology (KUET), Khulna, Bangladesh in 2016 and 2019, respectively. He joined as a lecturer at the Department of CSE, KUET in 2017. His research interests include computer vision, embedded system, machine learning and to solve real-life problems with the concept of computer science.
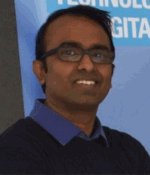
Md. Rahat Hossain
Md Rahat Hossain is a dedicated and articulate lecturer at CQUniversity, Australia with extensive teaching and learning experience across different areas of information and communication technology (ICT). He moved to Rockhampton, Australia from Bangladesh in March 2010 and completed his PhD in computational intelligence in 2013 at CQUniversity. Prior to his current academic position, Rahat acquired more than seven years of learning and teaching experience in the Department of Computer Science and Information Technology at Islamic University of Technology, Bangladesh. He achieved the “Student Voice Commendation Award for Distance Educator of the year 2018, 2017 & 2016” and obtained a place in the 2018 Early Career Researcher (ECR) Program at CQUniversity. Rahat possesses record of ability to conduct high-quality research that is reflected in the authorship of high-quality publications and other research outputs in the areas of ICT. He is currently working on an article about “Renewable Energy”. Email: [email protected]