Abstract
Accurate state of charge (SOC) estimation is considered as the main barrier to adopt Lithium-ion battery-based electric vehicles as alternatives to conventional internal combustion engine vehicles. There are several SOC estimation techniques recommended by many researchers still; they are all not accurate. This paper presents a robust and accurate SOC estimation battery model developed using an independently recurrent neural network (IndRNN). The proposed SOC estimation battery model is developed by training IndRNN constructively under various experimental datasets collected from Lithium Nickel Cobalt Aluminium Oxide battery cell at different ambient temperatures. Without any prior knowledge about battery internals, the proposed battery model successfully characterizes the non-linear behaviour of the battery effectively. Furthermore, its performance is proved by comparing with similar RNN architecture such as gated recurrent unit (GRU) and long short-term memory (LSTM). The attained results demonstrate that an IndRNN outperformed both GRU and LSTM in terms of accuracy under different electric vehicle drive cycle with minimal root mean square error of 0.7633% and mean absolute error of 0.6389% for diverse temperature conditions.
Abbreviations: ANN: artificial neural network; BMS: battery management system; BPNN: back-propagation neural network; DFS: deep feature selection; EV: electric vehicle; FL: fuzzy logic; FNN: feedforward neural network; GA: genetic algorithm; GRU: gated recurrent unit; HWFET: highway fuel economy test; ICE: internal combustion engine; IndRNN: independently recurrent neural network; LA92: Los Angeles 92; LiB: lithium-ion battery; LSTM: long short-term memory; MAE: mean absolute error; MAX: maximum error; RNN: recurrent neural network; RMSE: root mean square error; ReLU: rectified linear unit; SOC: state of charge; SOH: state of health; SVM: support vector machine; US06: supplemental federal test procedure; UDDS: urban dynamometer driving schedule.
Additional information
Notes on contributors
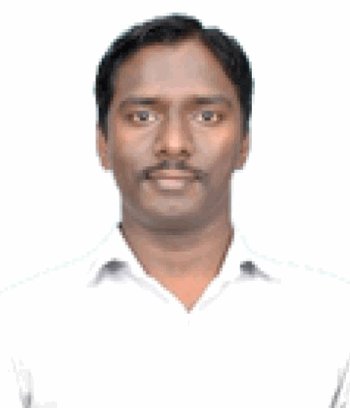
Prakash Venugopal
Prakash Venugopal received the BE degree in electronics and communication engineering from Srinivasa Institute of Engineering & Technology, Chennai, Tamil Nadu, India in 2006, MTech from College Of Engineering, Guindy, Anna University in 2010. He has more than 10 years of experience in academic teaching and currently pursuing a PhD degree in EVs. He is currently working as assistant professor in School of Electronics Engineering, Vellore Institute of Technology, Chennai. His research interest includes EV, realtime operating systems, deep learning Email: [email protected]
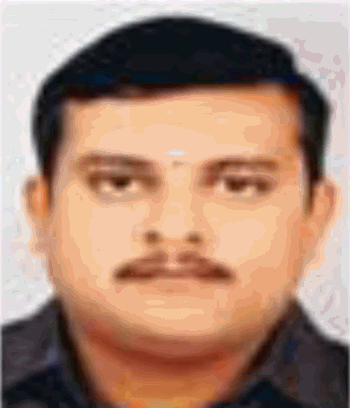
Vigneswaran T
Vigneswaran T received the graduate degree in electrical and electronics engineering from Bharathidhasan University Tiruchirappalli, Tamil Nadu, India in 2000, M.E (VLSI Design) from College of Engineering, Guindy, Anna University in 2003 and the PhD degree in low power VLSI design from SRM University, India in 2009. In 2003, he joined as lecturer in the Department of ECE, SRM University. His research interests include different aspects of low power VLSI circuit design and high-speed algorithm and architecture development. He is a Member of Indian Science Congress. Presently, he is working as a professor, School of Electronics Engineering, Vellore Institute of Technology, Chennai. Email: [email protected]
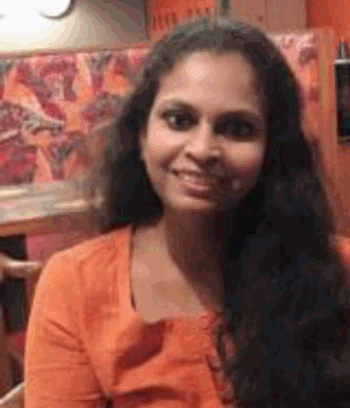
Sofana Reka S
Sofana Reka S is presently working as assistant professor senior in School of Electronics Engineering at Vellore Institute of Technology Chennai. She has been working in the area of smart grid, embedded systems and machine learning. She is an associate editor of IEEE Access journal. Published papers in various international journals. She is a reviewer of many SCI journals. Email: [email protected]