Abstract
The popularity of decision tree forest is due to its superior performance and accuracy. The accuracy of the decision tree forest algorithm depends upon the base learner and its diversification. As far as the literature is concerned, a large number of academia’s and researchers proposed various methods which are mostly based on pre-filtering and post-filtering of the decision tree forest technique. In this research work, a novel technique is proposed for increasing the mixture of individual decision tree present in the forest, which will improvise the final precision. In the proposed method, throughout the training, each tree of the forest is being trained to use dissimilar sets of rotation spaces which are linked together to an elevated space at the parent node. After linking each rotation space, the search for the good split is done within the elevated space. The decision of selecting a rotation technique for all the succeeding nodes depends upon the placement of a good split. Conventional equity market forecasting methods are mostly based on historical data and it is used to predict the upcoming pattern. As internet information is growing in an exponential manner, few authors have proposed work based on financial news and technical indicators for improving the prediction on the equity market. In this research work, heterogeneous information from various sources like social media, world market performance, global news, financial news and historical data have been considered for improving the prediction of Indian market indices. The performance of the proposed technique is evaluated on the Indian stock market indices with significant accuracy.
DISCLOSURE STATEMENT
No potential conflict of interest was reported by the author(s).
Additional information
Funding
Notes on contributors
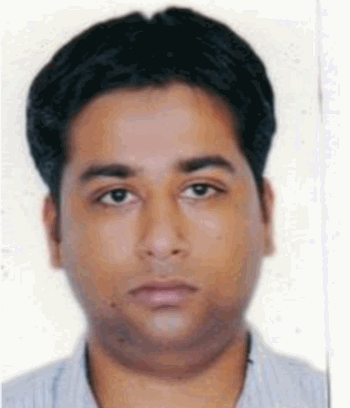
Lalit Agrawal
Lalit Agrawal received his BE degree in information technology from Shri Ramdeobaba College of Engineering and Management, Nagpur, India, in 2002 and postgraduate degree from Birla Institute of Technology and Science in 2013. He is currently pursing PhD degree in Shri Ramdeobaba College of Engineering and Management, Nagpur. His areas of interest include Internet of Things and data analytics. Agrawal has published several papers in reputed journals.
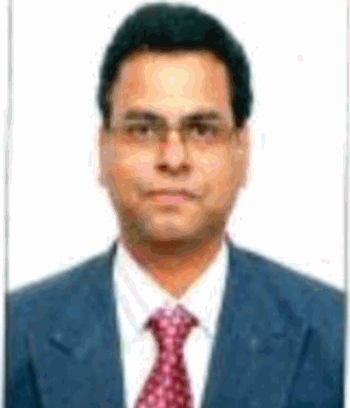
Dattatraya Adane
Dattatraya Adane is MTech in computer science and engineering from IIT Guwahati and received his PhD in computer science and engineering from VNIT, Nagpur. He is currently working as Professor, Information Technology Department, Shri Ramdeobaba College of Engineering and Management, Nagpur (MS), India. He has over 28 years of experience in teaching and research with over 45 publications in reputed journals. His areas of interest are information and network security, internet of things and data analytics. He is also a lifemember of ISTE and the Institution of Engineers, India. Email: [email protected].