Abstract
Unified Parkinson’s disease rating scale (UPDRS) is a popular tool in the clinical set up for the evaluation of Parkinson's disease (PD) symptoms and severity. However, the physical examination aspect and expertise requirements of the UPDRS assessment makes it subjective, expensive, and inconvenient. Thus, we have investigated the performance of vocal features from three sustained phonetic tasks (/a/, /u/, /m/) in objectively evaluating the motor UPDRS score which will help in the remote monitoring of PD motor symptoms. 26 PD patients (mean age = 72) and 22 control subjects (mean age = 66.91) volunteered the study. The voices were collected from: 1. PD patients in both off and on states of Levodopa medication and 2. control subjects. The Least Absolute Shrinkage and Selection Operator (LASSO) feature selection algorithm was applied to rank the voice features. Regression models using support vector machines (SVM), random forest (RF) and AdaBoost were employed for the objective evaluation of the motor UPDRS score. The parameters mean squared error (MSE), root mean squared error (RMSE), mean absolute error (MAE) and R2 were employed to assess the performance of the models. The average motor UPDRS score for PD off-state, on-state and controls were 27.31, 20.42 and 2.63, respectively. We observed a better objective estimation of UPDRS score in all the models when using the features from /m/ compared to that from /a/ and /u/. Our study assures the possibility of objective evaluation of motor UPDRS using the vocal features from sustained phonetic tasks in PD patients under Levodopa medication off and on-state..
Acknowledgements
Authors would like to acknowledge the fellowship provided under the Scheme for Promotion of Academic and Research Collaboration (SPARC) project. Authors also like to acknowledge Dr Peter Kempster, Dr Dinesh Kumar, Dr Sanjay Raghav, Dr Jenifer K. Nagao and Kitty Wong for helping us gather volunteers, collect their clinical data and for the continual support and encouragement throughout the study.
Additional information
Funding
Notes on contributors
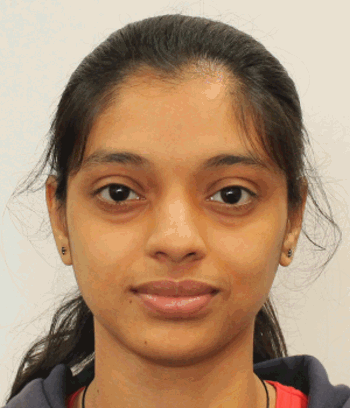
Rekha Viswanathan
Rekha Viswanathan received her PhD in electrical and electronics engineering from RMIT University, Australia. She is currently an early career researcher at RMIT University. Her areas of interest are speech data analysis, machine learning and signal processing. Email: [email protected]
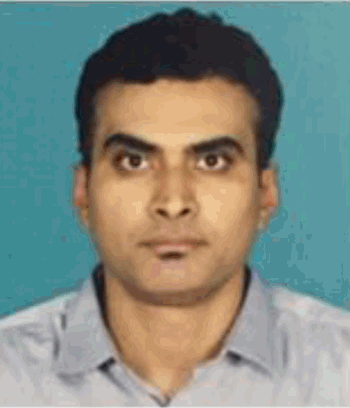
Sridhar P. Arjunan
Sridhar P Arjunan received his PhD in electronics and biomedical from RMIT University, Australia. He is currently in research position at Department of Electronics and Instrumentation Engineering, SRM Institute of Science and Technology. He has published over 100 research articles. His areas of interest are machine learning, wearable sensors, biomedical instrumentation & signal processing multispectral analysis.