ABSTRACT
It is very challenging to work with low resource languages pairs as monolingual and parallel dataset do not exist or exist in a very small amount. Furthermore, there is a lack of digitization of the available written resources. This work provides a comparison and analysis of the neural machine translation system for low resource definitely endangered, Kangri (ISO 639-3xnr) language using unsupervised and semi supervised methods. For this a shared encoder with back translation machine translation system for both unsupervised and semi-supervised learning techniques and a language model with denoising autoencoder that uses fully unsupervised learning technique has been used. Kangri which is an Indo-Aryan language has Devanagari () script same as Hindi. The translation task is further complicated by the fact that Kangri is a morphologically rich language, and it does not have well defined linguistic rules. To remove out of vocabulary problem we have used different technique and in finally, we have provided the comparison of results by taking the different evaluation metrics which shows that semi supervised translation with semi supervised cross lingual word embedding has highest score as compared to other translation models.
ACKNOWLEDGEMENTS
We thank Dr. Karam Singh, Director of Himachal Academy of Arts Culture and Languages, Shimla, Himachal Pradesh, India for their efforts in arranging workshops to collect datasets and to provide annotators. We also thank to all the authors of various books specially Praytoosh Guleri, Gautam Vayidhit. We also thank all the language translators writers, Bhupinder Singh Bhupi, Rajiv kumar Trigarti, Aman Kumar Vishva, Bharti kudailiya, Bhupinder Singh Bhupi, Deepak kulavi, Om parkash Prabhakar, Vijay Puri, Pratibha Sharma, Hari Krishan Murari, Navin Haldwani, Sanju Pal, Suresh Lata Awasthi, Shakti Singh Rana, Shelly Kiran, Vandana Rana, Vinod Bhavuk, Sonia Dutt, Virender Sharma, Gopal Sharma, Naveen, Manoj Kumar, Durgesh Nandan, Bhagat ram, Kushal, Tej Kumar.
DISCLOSURE STATEMENT
No potential conflict of interest was reported by the author(s).
Additional information
Notes on contributors
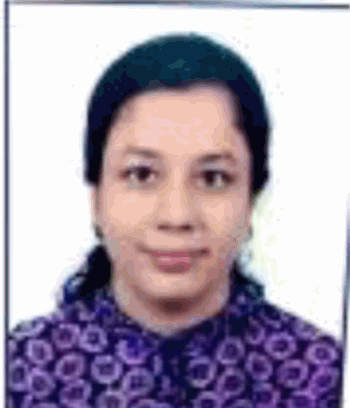
Shweta Chauhan
Shweta Chauhan pursued her BTech (Hons) in ECE, MTech in VLSI design automation and technique from NIT Hamirpur. She is pursuing her PhD in neural machine translation and evaluation for morphologically rich languages and low resource languages from the National Institute of Technology, Hamirpur (Himachal Pradesh).
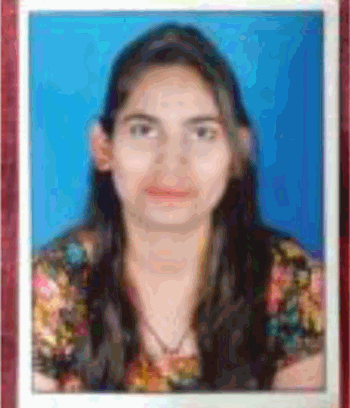
Shefali Saxena
Shefali Saxena is pursuing her PhD in natural language processing, statistical machine translation, low resource languages from the National Institute of Technology, Hamirpur, Himachal Pradesh. Email: [email protected]
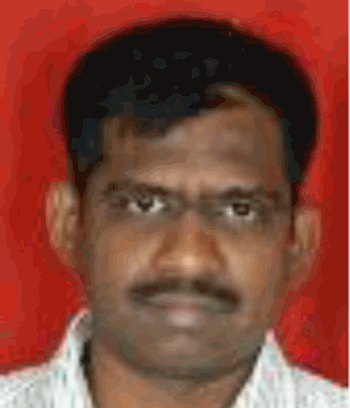
Philemon Daniel
Philemon Daniel, PhD in electronics and communication ngineering (NIT Hamirpur), MTech in VLSI design (VIT Vellore), BE in electronics and Communication Engineering (Bharathidasan University). Daniel has over 13 years of teaching experience at NIT Hamirpur as assistant professor. Email: [email protected]