Abstract
Stacked generalization-based heterogeneous ensemble methods combine the prediction of multiple classifiers to improve overall classification performance. Several stacking methods are available in the literature, but the criteria to select the number and type of classifiers are missing. This work analyzes the performance of stacked generalization-based ensemble machine learning methods for high-dimensional datasets. Also, the impact of the classifier selection for the first level () of the stacked generalization method has been studied. Based on that, the criteria for selecting classifiers at the first level of the stacked generalization method are proposed. So, six stacked generalization approaches are presented and have been analyzed for the thirty high-dimensional datasets. The experiments and results indicate that the performance of stacking strategies based on proposed selection criteria performs better. Also, a comparative study about the choice of homogeneous ensemble classifiers in stacked generalization concerning the use of basic classifiers and fusion of basic with homogeneous ensemble methods has been made. It has been observed that the use of only homogeneous ensemble classifiers is not beneficial in the stacked generalization methods. Also, the performance of stacked generalization based on basic classifiers or a combination of homogeneous ensemble methods with basic classifiers is better than homogeneous ensemble methods. The proposed stacking approach based on a combination of the basic and ensemble classifiers has improved the accuracy 0.72% to 8.46%. The impact of removing redundant and non-relevant features on the proposed stacking approaches has been evaluated.
DATA AVAILABILITY STATEMENT
The data that support the findings of this study are available in the ASU feature selection repository [https://jundongl.github.io/scikit-feature/datasets.html],OpenML [https://www.openml.org], and GitHub [https://github.com/ramhiser/datamicroarray].
Disclosure statement
No potential conflict of interest was reported by the author(s).
Additional information
Notes on contributors
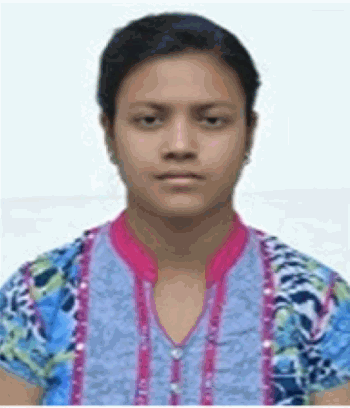
Suvita Rani Sharma
Suvita Rani Sharma received the Master of Technology degree in computer science and engineering from Sant Longowal Institute of Engineering and Technology, Longowal, India. Now, she is pursuing a PhD in computer science and engineering from the same institute. Her research interests include machine learning, feature selection, and metaheuristic optimization techniques. Email: [email protected]
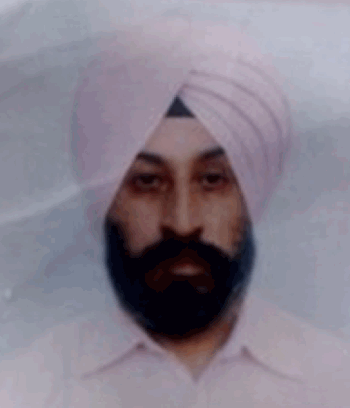
Birmohan Singh
Birmohan Singh is working as a professor in the Department of Computer Science and Engineering, Sant Longowal Institute of Engineering and Technology, Longowal, India. His research interests include signal processing, image processing, machine learning, and metaheuristic optimization techniques.
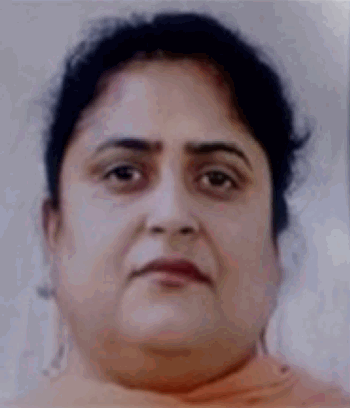
Manpreet Kaur
Manpreet Kaur is a professor in the Department of Electrical and Instrumentation Engineering, Sant Longowal Institute of Engineering and Technology, Longowal, India. Her research interests include biomedical signal processing, image processing, and machine learning. Email: [email protected]