ABSTRACT
Brain-Computer Interface (BCI) connects the human brain with computers and electronic devices. The signals from human brain are processed using several deep-learning techniques to convert them into a comprehensible form. Among these techniques, the convolutional neural network (CNN) model has excellent performance in BCI recognition. However, the existing CNN model is prone to over-fitting and has limitations with accuracy. The model complexity must be increased to achieve better accuracy. To address these concerns, a novel hybrid R-CNN model for BCI thought recognition is proposed in this work. The convolution layer of CNN and the Long short-term memory (LSTM) layer of recurrent neural network (RNN) is utilized for this purpose. A batch normalization layer is also instigated to reduce over-fitting. Further, a rectified linear unit (ReLU) is engaged to speed up training under as low as five epochs, along with a custom optimizer which optimizes some default values within the optimizer. Experiments are performed with BCI datasets of two different file sizes with different records. The first dataset size is 6.5 MB having 60684 records with three classes, and the second dataset size is 10.1 MB having 94119 records with five classes. Consequently, the proposed hybrid model exhibits a higher average accuracy of 95% for 6.5 MB file size and 98% for 10.1 MB file size, which is superior compared to the accuracy of existing deep learning models. Furthermore, the efficiency of the proposed novel hybrid R-CNN model is evaluated with some other performance measures such as F1-score, recall and precision.
Disclosure statement
No potential conflict of interest was reported by the author(s).
Additional information
Notes on contributors
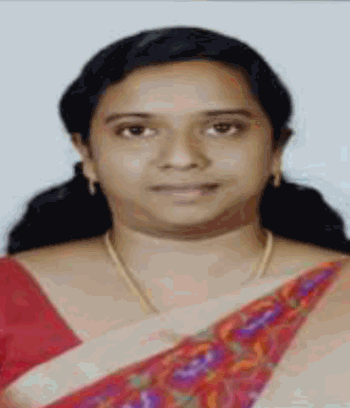
Janeera D.A
Janeera DA is an assistant professor in the Department of Electronics and Communication Engineering at Sri Krishna College of Engineering and Technology, Coimbatore. She has completed her BE in electronics and communication engineering from Karunya University in 2011 and ME in applied electronics from Sri Krishna College of Engineering and Technology during 2014. She is currently pursuing her PhD in brain-computer interface under Anna University, Chennai. She has over seven years of teaching experience and three yrs of research experience. She has published many research papers in several national and international journals and conferences. She has also authored a book on VLSI Design. Her key research areas include BCI, VLSI and neural networks.
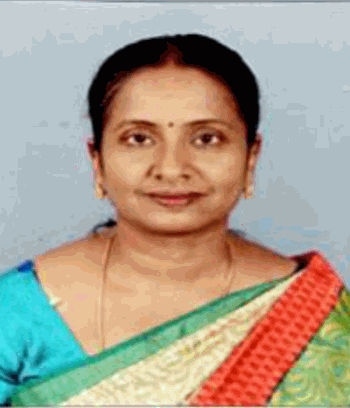
S. Sasipriya
S Sasipriya has completed her UG in 1994 from Bharathiar University and subsequently PG from Anna University. She received her PhD in the area of wireless communication in the year 2012. She has to her credits 52 paper publications in various international conferences / journals. She has published three patents and is the author of two book chapters. She has organized many sponsored conferences and workshops. She is currently guiding 10 research scholars under information and communication engineering and four scholars have successfully completed under her guideship. Her area of research includes interference cancellation, cognitive radio, signal integrity and MIMO. She is a member of ISTE, IEEE and IETE. She has delivered guest lectures at various institutions under Anna University Sponsored FDP Programme and AICTE sponsored Summer/Winter School. At present, she is working as professor in the Department of ECE in Sri Krishna College of Engineering and Technology. Email: [email protected]