Abstract
The non-orthogonal multiple-access (NOMA) technique affords massive user scaling, industrial use for mission-critical communication, and multi-user shared in a single resource block. Because of these merits, it is a scheme that future technologies will prefer over orthogonal multiple access (OMA). This research study focuses on a comparative analysis of several power allocation algorithms (PAA) to identify the PAA that is most suited for the “MIMO-NOMA” technology. The investigation is done using two different methods. First, an analysis is conducted to take into account direct approaches, followed by PAA and the Deep Neural Network (DNN) and the Depth Limited Search Algorithm (DLSA). Both techniques are tested considering two-user and multi-user scenarios in a MIMO-NOMA network. Optimum power allocation (OPA) to the weaker user terminal is a complex task in a real environment; moreover, the appropriate distribution of access point (AP) source power is constrained by the successive interference cancellation (SIC) technique. The above issues can be resolved with the help of the Deep Neural Network (DNN) and DLSA, where the stronger signal user gets minimal power and the poorer signal user gets optimal power. Based on the DLSA, the “DNN-MIMO-NOMA” system helps user terminals to receive their signals more accurately and without noise. For the successful implementation of SIC, the DLSA combines MIMO-NOMA with deep learning. The location of the user terminal is predicted by the DLS and depending on where the user terminal is located; optimal power allocation is done. The simulation results show that the PAA, combining DNN and the DLSA, outperforms traditional power allocation technique.
Disclosure statement
No potential conflict of interest was reported by the author(s).
Additional information
Notes on contributors
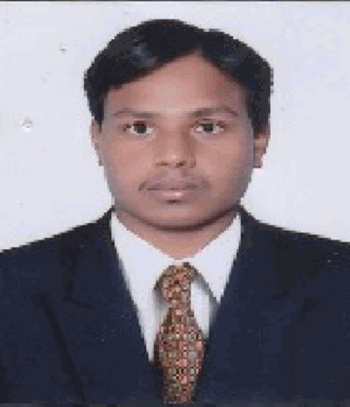
Ravi
Mancharla Ravi received the BTech and MTech degrees in electronics and communication engineering from Jawaharlal Nehru Technological University, Hyderabad, India in 2012 and 2014, respectively. He is currently pursuing PhD at the Department of Electronics and Communication Engineering, National Institute of Technology, Arunachal Pradesh, India. His areas of interest are NOMA networks, deep learning, the internet of things, cellular and space communications, massive MIMO networks, and machine learning.
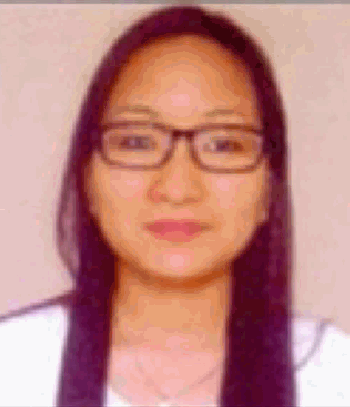
Y. Bulo
Yaka Bulo is an assistant professor at the National Institute of Technology Arunachal Pradesh in the Department of Electronics and Communication Engineering. She received the BTech degree in electronics & telecommunication engineering from Shivaji University, Maharashtra, India in 2010, MTech in information technology from North Eastern Regional Institute of Science and Technology (NERIST), Nirjuli, Arunachal Pradesh, India 2012 and PhD in electronics and communication engineering from National Institute of Technology, Arunachal Pradesh, India in 2017. Her areas of research interest are wireless sensor networks, error control techniques, 5G technology, NOMA and hybrid automatic repeat request (HARQ) protocols. Email: [email protected]