Abstract
Plant diseases are a significant threat to global food security since they directly affect the quality of crops, leading to a decline in agricultural productivity. Several researchers have employed crop-specific deep learning models based on convolutional neural networks (CNN) to identify plant diseases with better accuracy and faster implementation. However, the use of crop-specific models is unreasonable considering the resource-constrained devices and digital literacy rate of farmers. This work proposes a single light-weight CNN model for disease identification in three major crops, namely, Corn, Rice, and Wheat. The proposed model uses convolution layers of variable sizes at the same level to accurately detect the diseases with various sizes of the infected area. The experimentation results reveal that the proposed model outperforms several benchmark CNN models, namely, VGG16, VGG19, ResNet50, ResNet152, ResNet50V2, ResNet152V2, MobileNetV2, DenseNet121, DenseNet201, InceptionV3, and Xception, to achieve an accuracy of 84.4% while using just 387,340 parameters. Moreover, the proposed model validates its efficacy as a multi-functional tool by classifying healthy and infected categories of each crop individually, obtaining accuracies of 99.74%, 82.67%, and 97.5% for Corn, Rice, and Wheat, respectively. The better performance values and light-weight nature of the proposed model make it a viable choice for real-time crop disease detection, even in resource-constrained environments.
DISCLOSURE STATEMENT
No potential conflict of interest was reported by the author(s).
Additional information
Notes on contributors
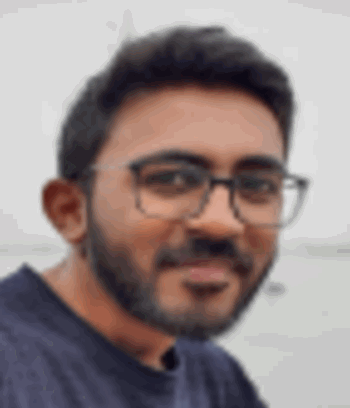
Sahil Verma
Sahil Verma received his BE degree in information technology from Birla Institute of Technology, Mesra in 2017. He received the MTech in computer science and engineering from the National Institute of Technology, Patna in 2020. Currently, he is pursuing PhD in the Department of Computer Science and Engineering, National Institute of Technology (NIT) Patna. His current research area includes machine learning, deep learning, and nature-inspired optimization techniques. Corresponding author. Email: [email protected]
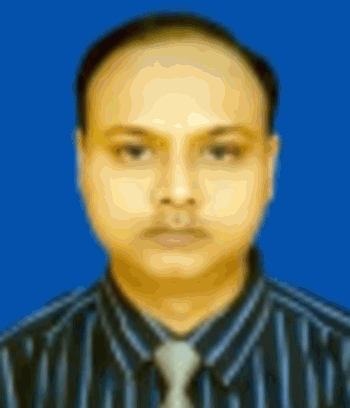
Prabhat Kumar
Prabhat Kumar is a professor in the Department of Computer Science and Engineering at the National Institute of Technology Patna, India. He is a member of the International Federation for Information Processing (IFIP) Working Group (WG) 6.11: “Communication Aspects of the E-World” and a member of NWG-13 (National Working Group 13) corresponding to ITU-T Study Group 13 “Future Networks, with focus on IMT-2020, cloud computing, and trusted network infrastructures”. He is also the former state student coordinator of Bihar for the Computer Society of India. He holds a PhD in computer science and MTech in information technology. He is a senior member of IEEE, a professional member of ACM, a life member of CSI, the International Association of Engineers (IAENG), the Indian Society for Technical Education (ISTE) and a global member of the Internet Society. His research area includes wireless sensor networks, the internet of things, data analytics, software engineering, e-governance, etc. Email: [email protected]
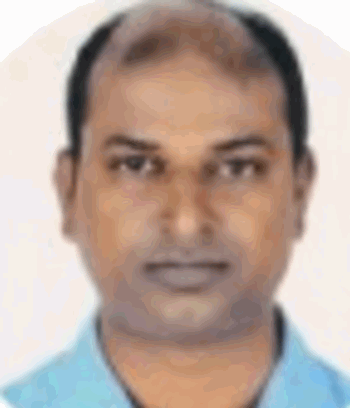
Jyoti Prakash Singh
Jyoti Prakash Singh is an associate professor in the Department of Computer science and Engineering at the National Institute of Technology, Patna. He has coauthored seven books in the area of C programming, data structures, operating systems, and ad hoc networks. Apart from this, he has around 25 international journal publications and more than 40 international conference proceedings. His research interests include text mining, deep learning, social network, and information security. He is the associate editor of the International Journal of Electronic Government Research. Email: [email protected]