Abstract
As the world’s population grows, the agricultural sectors are destined to increase crop production and security. Improving crop yield by using advanced technologies gives remarkable growth to the economy of the country. Agriculture provides over 20% of India's GDP. Using machine learning algorithms, the crop yield can be predicted which is useful to the farmers to plan the cultivation beforehand. In this work, various machine learning (ML) algorithms are applied to predict the yield of ‘rice and sorghum (jowar)’ and a novel weighted feature approach with a combination of Support Vector Machine (SVM) and Random Forest (RF) is proposed for two Indian seasons. RF is used to select training data at random, and the learning rate approach from deep learning concepts is implemented to add random weights to each parameter; the SVM model is then trained using the weighted training data. The best weights are again applied for the whole data to implement the SVM and RF algorithms. The weighted feature hybrid model is compared with SVM, RF, Decision tree, Naive Bayes, and k-Nearest Neighbor algorithms. RF-based regression method is also implemented and its ability to predict the crop yield has been discussed based on its performance metrics. The results show that the proposed weighted feature hybrid SVM-RF model gives the best accuracy of 90% when compared with the traditional algorithms. Also, the performances of various ML algorithms for crop yield prediction are analysed and cross-validation of the models is performed and compared, which improved the accuracy by 8-10%.
ACKNOWLEDGEMENTS
We thank the Telangana state development planning society for providing the required data set.
DISCLOSURE STATEMENT
No potential conflict of interest was reported by the author(s).
Additional information
Notes on contributors
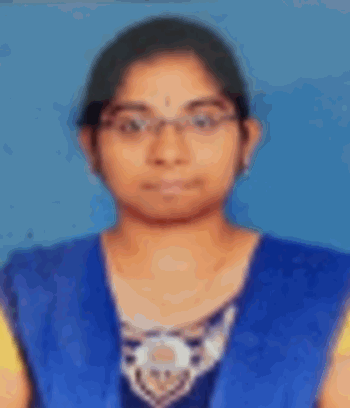
S. Pavani
S Pavani is currently pursuing her PhD under the guidance of Augusta Sophy Beulet P at Vellore Institute of Technology, Chennai, India. She received her bachelor's and master's degrees from JNTU Hyderabad University. Her research interests are VLSI design, data science, machine learning, deep learning and hardware implementation. Email: [email protected]
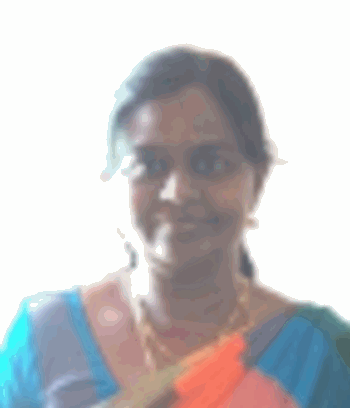
Augusta Sophy Beulet P.
P Augusta Sophy Beulet, being a lecturer for more than 25 years, has developed a passion for teaching engineering subjects and guiding projects. The passion has naturally been extended for developing the students too. She has handled more than 20 different subjects in electronics and communication engineering and guided more than 50 projects at UG and PG levels. She has published more than 30 research papers in international conferences and Journals. She did her research work in the field of VLSI signal processing at Anna University, in 2015. She received her ME degree from Anna University and finished her BE degree at the Government College of Engineering, Tirunelveli. Now she is working as professor at VIT University, Chennai. Corresponding author. Email: [email protected]