Abstract
Water managers lack practical and readily available tools to inform them about what impact price changes (or changes in other drivers of water use) will have on demand – and therefore revenue – over both the short and long term. This paper examines how the concept of demand elasticity can be used to model changes in annual aggregate water use in response to future changes in major demand drivers including water and electricity prices, average income, population, level of business activity and climate. It does so by describing a pilot investigation completed in York Region in Southern Ontario, where a range of assumptions about price elasticities were used to calculate the rate of growth for water demand over a 40-year period. This investigation was deliberately exploratory and the findings can only be considered indicative and preliminary. However, with further development, the modelling approach described could provide an additional tool to help water managers understand changes in demand, and communities make the transition to a conservation-oriented water pricing system.
Les gestionnaires de l’eau n’ont pas suffisamment d’outils pratiques et facilement accessibles à leur disposition pour pouvoir déterminer quelle sera l’incidence des fluctuations de prix (ou fluctuations liées à d’autres facteurs entourant l’utilisation de l’eau) sur la demande et, par conséquent, sur les revenus, aussi bien à court terme qu’à long terme. La présente communication examine la façon dont le concept de l’élasticité de la demande peut servir à modéliser les changements touchant l’utilisation globale annuelle de l’eau en réponse aux changements futurs liés aux principaux facteurs entourant la demande, notamment les prix de l’eau et de l’électricité, le revenu moyen, la population, le niveau d’activité commerciale et le climat. Dans cette optique, la présente communication décrit une étude pilote menée dans la région de York dans le sud de l’Ontario, où une gamme d’hypothèses à propos de l’élasticité-prix a servi à calculer le taux de croissance de la demande en eau sur une période de 40 ans. Cette étude était délibérément de nature exploratoire et les résultats peuvent uniquement être considérés comme étant indicatifs et préliminaires. Cependant, moyennant un développement plus poussé, l’approche de modélisation décrite pourrait fournir un outil supplémentaire qui aiderait les gestionnaires de l’eau à comprendre les fluctuations de la demande et les collectivités à entreprendre la transition vers un système de tarification de l’eau axé sur la conservation.
Introduction
Municipal water managers need to understand future use trends for the purpose of anticipating growth and for infrastructure planning. There are different methods of projecting demand. Past research has focused on complex forecasting approaches. This includes both simple and intricate statistical models and data-intensive end-use models, which typically rely on predicting, quantifying and aggregating the consumption of various end uses at the individual household and business level.
At the same time, water managers also have reasons to be interested in the impacts of different levels of and approaches to services pricing – ensuring that they collect enough revenue to pay for the operation of the system and necessary future capital works chief among them. However, they may also be interested in the potential impact of price on consumption, and thus its value as a tool to promote conservation, referred to here and elsewhere as conservation oriented water pricing (see Brandes et al. Citation2010).
Unfortunately, water managers in general do not have sufficient or readily available tools to inform them about what impact price changes (or changes in other drivers of use) will have on demand – and therefore revenue – over both the short and long term. This creates yet another barrier to pursuing a course of price reform.
One option that is well understood by economists, but rarely applied practically, is to rely on the concept of demand elasticity. This is a tool for measuring the responsiveness of a function to changes in parameters in a relative way.
The purpose of this paper is to apply estimated demand relationships to carry out scenario analysis depicting paths of water use growth under differing assumptions regarding future changes in major drivers including water and electricity prices, average income, population, level of business activity and climate. It does so by describing a preliminary investigation completed in York Region in Southern Ontario, where a range of assumptions about price elasticities were employed to calculate the rate of growth for consumption over a 40-year period.
If the role played by specific drivers of water use, such as price, can be better understood, then managers in local governments and utilities will be in a better position to advise on potential measures, policies and programs to promote efficiency and conservation. Importantly for this research, we also suggest that scenario building based on demand elasticity also offers a novel and simple way to contemplate future community demand, offering a complement, if not a substitute, to the more labour- and data-intensive forecasting methods already available.
The article has four main parts. First, it provides background including discussion of some of the current forecasting methods in use and some of the challenges with them. This section also discusses the concept of demand elasticity and proposes that using this might provide an alternative approach. Finally, background on the York Region case study context is provided. Second, it sets out the methodology. Third, it provides results. Finally, the last section discusses both the potential and the limitations of this method for the future, opportunities for additional research and insights into the implications for conservation-oriented water pricing.
Background
Forecasting future water demand
Water managers and practitioners forecast water use for three main reasons:
Strategic forecast: this is the forecast with the longest and broadest perspective. Importantly, this level of forecast can investigate the impacts of structural and technological changes to the economy as well as the impacts of major policy changes. This is the level at which forecasting is completed in the analysis in this paper.
Investment or tactical forecast: this is a more detailed appraisal usually divided by user group and used for medium-term investment decisions.
Operational forecast: this is a very short-term and detailed analysis of alternate facets of water demands that is typically conducted by municipal and regional water suppliers. For example, this may involve looking at the expected magnitude of peak loads and other seasonal patterns in water consumption.
In the method of forecasting that has dominated historically, total future demand is predicted as the product of expected population growth and a fixed per-capita water use coefficient. It is assumed that as population increases, residential water demand could be expected to increase at the same rate, based on the assumption that each person requires a specific volume of water. This implies a population elasticity of aggregate residential water demand of 1.0.
This method can be refined by disaggregating total water use: first by user classes and, subsequently, by area (for example, different water-use patterns across neighbourhoods) and time period (for example, summer versus winter use). The fundamental forecasting method remains the same, however, with unit water-use coefficients being multiplied by the projected growth in a particular user group in a specific location.
Development of the Municipal and Industrial Needs (MAIN) model in the 1980s represented a significant advance in forecasting methods. It employs the disaggregated factor forecast approach in which total urban water use is disaggregated into major urban sectors (residential, commercial/institutional, industrial, system loss, etc.). These sectors, in turn, are broken down into hundreds of categories. For each category, water use is estimated for summer and winter seasons and during maximum day (Dziegielewski Citation1996). Since then, numerous other approaches to end-use modelling have been developed (for further discussion see Worthington and Hoffman Citation2008; Rathnayaka et al. Citation2011).
In recent years, researchers have explored alternative approaches to computing water demand forecasts (provided by highly disaggregated end-use models) by employing sophisticated statistical and mathematical techniques (Billings and Jones Citation2008). These include estimated autoregressive integrated moving average (ARIMA) time series models (Jain and Ormsbee Citation2002) and artificial neural network models (Ghiassi et al. Citation2008).
The major advantages of these approaches are that they can accurately represent the time series properties of historical data and are amenable to testing alternative hypotheses regarding the data. The principal shortcomings include their complexity (and the resulting difficulty to explain them to community members), the relatively high level of aggregation at which the forecasts are usually conducted, their data hunger and the challenge of using them for policy analysis and scenario building.
Elasticity of demand as an alternative way to model future water consumption
One alternative to the traditional approaches and to the newer, more technically complex computer modelling versions is to ground projections in the economic concept of demand elasticity. As noted above, this is a tool for measuring the responsiveness of a function to changes in parameters in a relative way. Specifically, it refers to the ratio of the percent change in one variable to the percent change in another variable.
Some products typically have large price elasticities because they are discretionary purchases or they have many substitutes. On the other hand, some commodities have quite small elasticities because they are very important to consumers and have few substitutes. Not surprisingly, water is usually considered to fall into the second category of commodities (see Dalhuisen et al. Citation2003 for more on the price elasticity of residential water demand).
However, price is just one driver of demand. Water use in households and businesses is driven by various forces. Past economic research and experience have identified the factors in Table as the most important for influencing water use for each sector (Renzetti Citation2002; Worthington and Hoffman Citation2008).
Table 1. Factors that influence water demand.
The key to the type of modelling proposed here is to examine the likely impacts on aggregate water use in a community when major drivers of demand are changed.
Consider the following example. Suppose, for simplicity, that the only factor influencing the growth of residential water demand is population change (actually, this is frequently assumed in water planning studies). If population is assumed to grow over the near future at an annual rate of 2%, and if it is assumed that the elasticity of water use with respect to population is 0.8, then residential water use would be projected to grow annually at 0.8 × 2% = 1.6%. What makes things more complicated is that there is good reason to believe that a variety of factors influence residential and non-residential water demand. As a result, as many of these influential factors as possible must be considered if there is to be a reasonable forecast of growth in water demand. When there are multiple demand drivers (income, population, prices, water agency conservation programs, etc.), then each must be considered, and the cumulative effect on demand must be calculated by summing the individual effects. Thus, two types of information are needed for each demand driver: the expected rate of growth in the future, and the sensitivity (or elasticity) of water demands to each driver.
Exploratory case study in York Region
To test the hypothesis that applying elasticities of demand offers an alternative and potentially practical approach to modelling future water demand, an exploratory case study was conducted in partnership with the Regional Municipality of York. York Region provides wholesale supply of drinking water to nine area municipalities in Southern Ontario: Aurora, East Gwillimbury, Georgina, Township of King, Markham, Newmarket, Richmond Hill, Vaughan and Whitchurch-Stouffville.
This project was completed as a component of the Region’s Long-Term Water Conservation Strategy (York Region Citation2011). This broad and comprehensive plan identifies policies, measures and programs to achieve water efficiency goals over a 40-year horizon to 2051. Its intent is to position York Region as a national leader in water conservation.
By national standards, the municipalities within York Region are already quite well positioned with respect to conservation-oriented pricing. Virtually all residential dwellings and businesses that are connected to the potable water supply are individually metered (barring residents and businesses in multi-unit buildings) and all nine municipalities charge their customers based on the volume of water they consume. At the time of the study, residential water use in the Region was 252 L per capita per day (York Region Citation2011). This figure is comparable to both Canadian and Ontario averages (Environment Canada Citation2011). Comparing York Region with elsewhere in Southern Ontario and across Canada, the data indicate that combined prices for water and wastewater services are not the highest in the region, but are generally above the Canadian national average (see Econnics et al. Citation2011).
In 2008, York Regional Council approved a series of rate changes for both water and wastewater, including a 10% increase for 2009–2011. This follows from other historical rate increases, which were passed on by local governments at the retail level. However, over the past several decades, while residential water prices appear to have just kept up with inflation, non-residential prices appear to have actually fallen in real terms. When the effects of inflation have been netted out of price changes, the rate of growth in non-residential prices is actually negative (–0.55% per year).
There is an important relationship between population growth and future water use. York Region’s population is one of the fastest growing and most diverse in all of Canada (York Region Citation2007). As of 2009, the Region’s population was estimated to be 1,032,605, with a water-serviced population of 993,109. The total population is projected to reach 1.5 million people by 2031, and over 1.8 million by 2051, with virtually all new growth to receive water through municipal supply (see Figure ).
Figure 1. Projected York Region population growth to 2051. Based on York Region (Citation2010).
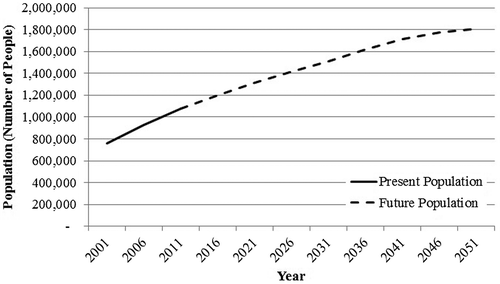
Methodology
The methodology used for this work is fully documented in a report prepared for York Region in 2011 (Econnics et al. Citation2011). This section provides a summary, but the reader wanting more detail is referred to the full report which can be found online (see the references below for details).
As a comparative starting point, the study first looked at a more conventional statistical extrapolation of the observed trend in York Region’s residential water output between 1980 and 2008. Using work carried out by Sampson Ndah (Citation2010), this analysis uses the ARIMA technique. It bases its projection only on the observed trends in the volume of water produced, and extrapolates these into the future. It does not use information on the role played by changes in population, income, prices or any other demand driver. The procedure is statistically quite sophisticated. However, this method may be considered “naïve” because it does not seek to explain the projected changes or relate them to their underlying causes. Nonetheless, it is based on a statistical technique that is widely used in the water-planning field and provides a useful comparison to the results in the modelling described later in the report. As shown in Figure below, this “naïve” projection estimates that York Region water demand will grow by over 80% by 2050.
Figure 2. Autoregressive integrated moving average (ARIMA)-based projection of water demand growth in York Region. Based on Ndah (Citation2010).
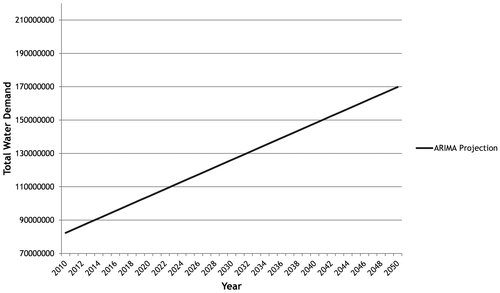
Importantly, note that the ARIMA model included only residential demand, so the starting point for 2008 differs significantly from what is found in our alternative projections provided below (which include both residential and non-residential). Nevertheless, this allows for a useful point of contrast (e.g. by looking at the slopes of the respective curves).
With this comparative starting point in mind, attention turned to using demand elasticities as an alternative approach to modelling.
The modelling begins by dividing total water use in the region into residential and non-residential water use. This is because different drivers influence each sector. For example, past research has shown that climate strongly influences residential water use (primarily in the summer months) but has relatively less influence over most non-residential water uses. Thus, it was assumed that households will continue to consume about 60% of the total water output of York Region and non-residential customers will consume the remainder (based on data from Environment Canada Citation2009).
The estimated change in total water use in each sector is calculated by separating the effects of the demand drivers into two components: (1) the projected change in the driver over the period 2011 to 2050, and (2) the expected sensitivity of residential or non-residential water use to each driver (captured in the demand elasticity).
The next step involved gathering the best available data on how drivers of water demand may change in the future. This required surveying the economic research literature to find values (or ranges of values) for the demand elasticities for each of the drivers (income, climate, prices, etc.). As much as possible, elasticity values were taken from case studies that were similar to the features of York Region.
After taking into account what data were available (and, thus, how many demand drivers could be included in the model), total residential water demand in York Region was assumed to depend on the following: population, income, water and electricity prices, and climate (temperature and precipitation). Total non-residential water demand in York Region is assumed to depend on level of economic activity and prices (water and electricity). A full explanation of the research and elasticity values used can be found Econnics et al. (Citation2011).
An important omission from these factors is the role of conservation programs, which have been shown to influence residential water demands in various jurisdictions (for a more general discussion see Brandes et al. Citation2006; Maddaus and Maddaus Citation2006). However, it is quite difficult to incorporate the effects of these types of programs into the type of model used here. There are also challenges related to the overlap in the results that are achieved by pricing and conservation programs. Thus, the projections listed here must be interpreted as assuming that there are no future programs of this sort (except raising the price of water). This is an area where this methodology can be improved.
The rate of growth for water demand was calculated using low, medium and high elasticity values. This was done in order to see how the projections in each scenario vary according to the assumed elasticity values. However, for ease of reading, only results using the medium elasticity values are provided here. Results for high and low values can be found in Econnics et al. (Citation2011).
Next, a number of different scenarios were developed and the rate of growth of total community water demand was calculated. The various projections differ in their assumptions regarding the rates of growth of the demand drivers, again based on the best readily available data. The individual demand drivers’ percentage rate of change was calculated for each scenario and multiplied by its respective elasticity. Then, the projected rate of change of water demand was calculated as the summation of the (weighted) rates of change of the individual drivers.
The projections of aggregate residential and non-residential water use were carried out for the period 2010 to 2050. This was calculated as the sum of projected residential and non-residential water use during each year. The year 2050 was selected as the time horizon for the model simply because this aligned with York Region’s broader long-term conservation planning process. It should be recognized, however, that the reliability of assumptions about water use and elasticities diminishes as the planning horizon moves into the future, particularly with a very distant one such as that used for this project.
It is important to note that for any demand driver measured in dollars (water prices, electricity prices, average household income, etc.), the effects of inflation have been netted out of any projections. Thus, in the base case scenario described below, past changes in water and electricity prices are measured net of inflation.
Assumed elasticity values at the medium level are listed in Table .
Table 2. Assumed medium-level demand elasticity values.
Results
While the number of scenarios that could be modeled with this approach is virtually limitless, the description is limited to the results of four different scenarios below. Not surprisingly, the results for community water consumption vary greatly depending on the inputs.
Scenario #1: No real price increase (NP)
This simple demand model bases its projection only on growth in demand drivers that are outside of the control of governments in York Region. Thus, it is assumed that growth in residential water use is driven only by changes in population, income and climate. Growth in non-residential water use is driven only by the level of commercial activity. All prices are assumed constant in real terms, and thus do not influence water use. The NP results are broadly similar to the projection from the ARIMA statistical model, at least in order of magnitude (noting again that our projections contain both residential and non-residential demand, whereas the ARIMA results depicted in Figure contain only residential). Specifically, the combined effects of growth in population, average household incomes, regional economic activity and climate lead to increases in total water demands of 35.0% over the next 40 years, assuming medium elasticity values for all parameters.
Scenario #2: Base case (BAC)
This projection adds price changes to the NP model. In this case, recent historical trends in price change are followed for all demand drivers in order to construct projections. The nature and source of the projected price changes should be noted. It is assumed that local water or electricity supply agencies are not embarking on new efforts to raise prices faster than they have in the past. Instead, it is assumed that there are no new significant policy-related changes in water or electricity prices. These simply continue to rise based on recent trends, with real water prices increasing by 0.38% annually.
In this scenario, the percentage change in total water demand over the period is projected to be 32.4% over the next 40 years, assuming medium elasticity values for all parameters. Comparing BAC to NP, it can be seen that growth of future water demand is curbed by a small amount (from 35.0% to 32.4%) by simply carrying forward recent trends in changes to water and electricity prices.
Scenario #3: Medium water price increases (MW)
This projection is the same as the aggressive water price increases (AW) scenario (described next) except that water prices rise by a more moderate rate. That is, they are assumed to grow at the rate found in the BAC scenario plus an additional 1.5% per year (i.e. at an inflation-adjusted rate of 1.88% per year rather than 0.38%). Electricity prices are assumed to rise at the previously assumed slower rates found in the BAC projection. Assuming all demand drivers except the price of water follow BAC trends, this reduces projected water demand growth somewhat in the region. Not surprisingly, the resulting rates of water demand growth are approximately halfway between the rates seen in the BAC and AW scenarios. The percentage change in total water demand over the period is projected to be 19.2% over the next 40 years, assuming medium elasticity values for all parameters.
Scenario #4: Aggressive water price increases (AW)
This projection is the same as BAC except that water prices are assumed to rise “aggressively.” Specifically, real water prices are assumed to rise annually at the BAC rate (0.38%) plus 3.0%, or 3.38% annually. Electricity prices are assumed to rise at the previously assumed slower rates found in the BAC projection. Assuming all demand drivers except the prices of water follow BAC trends, and that residential and non-residential prices of water follow an aggressive growth rate, comes close to eliminating projected water demand growth in York Region, despite population increase. The percentage change in total water demand over the period is projected to be 6.0% over the next 40 years, assuming medium elasticity values for all parameters. Including the effects of compounding, this involves a significant increase in average water price at the end of the period, so this scenario should probably be considered an illustrative outer “goalpost” or extreme rather than a proposal for an actual annual price increase.
Summary
Summary results in terms of annual and total percent change for the four main scenarios are set out in Table below, all assuming medium elasticity values. Projected growth in total water demand is summarized in Figure .
Table 3. Results for all scenarios assuming medium elasticity levels.
Discussion and conclusion
The scenario-building approach presented above provides a novel and alternative way for water managers to consider future water demand in their communities. Importantly, it provides a means to investigate the potential impacts of factors that are rarely looked at with current modelling approaches, notably the prices of water services and energy.
This modelling approach also enables experimentation with different approaches to conservation-oriented pricing, which is emerging as an increasingly important tool to help control demand. Water managers need more practical tools to understand the impacts that price changes will have on revenue, water demand and communities. This approach is a potential way forward. Additionally, it has the advantage of offering a means for water managers to communicate with elected officials and community members about the likely impact of price changes as a demand management measure.
Despite the potential, the reader is advised to keep a few caveats in mind. First, the estimates of elasticity used in this analysis are just that – estimates taken from research literature or case studies of places similar to York Region. It would be preferable to compute elasticities directly from data drawn from York Region (this was done recently by Bithas and Stoforos Citation2006, in their forecasts of water demands in Athens, Greece). Unfortunately, problems with data availability as well as time and resource constraints precluded this approach. Second, and importantly, elasticities will tend to change over time, for example due to changing technology and cultural preferences. Future changes in demand elasticities may have a significant impact on the demand estimates derived. This would argue for any water agency to regularly review its demand forecasts in light of new information and revised elasticity estimates. Related to this point is the challenge of including energy prices in the models of water demand. There are very few studies that estimate the impacts of energy prices on residential water demands. We believe ours to be the first in Canada to address this. Given the paucity of studies, this is an area that requires further study, and any agency including energy prices in its model should carry out sensitivity analysis on the assumed elasticity of water demand with respect to energy prices. Third, no quantitative analysis has been completed on how to deal with possible distributional impacts on the community from water price changes (e.g. on low-income people), nor have any financial impacts on water service provider revenue been quantified. Finally, and of most significance, at this point we have not provided confidence intervals on the accuracy of the projections in our scenarios (with the comment that the same limitation characterizes virtually all of the other more established forecasting techniques already routinely used by water managers to make infrastructure planning decisions). A straightforward way to address this issue is to carry out a sensitivity analysis by examining the impacts of alternative assumed elasticity estimates on projected demand growth.
In sum, the quantitative analysis provided here is intentionally basic and serves as a demonstration project to illustrate the potential impact that changes in water price or other variables might have on community water demand. The findings should not be taken as conclusive. Further investigation using appropriate locally sensitive data to develop a clear local price elasticity of demand would be required to forecast the impact that price changes have with greater accuracy.
This investigation was deliberately exploratory, and the findings should be considered indicative and preliminary only. However, the analysis does indicate a practical path towards new tools that can help communities make the transition to a conservation-oriented water pricing model.
Acknowledgements
The authors wish to acknowledge the editorial and technical assistance of Laura Brandes, Communications Director for the POLIS Water Sustainability Project. We also acknowledge the work of Ms. Yang Tang, a graduate student in the Department of Economics at Brock University under the supervision of Professor Renzetti, who completed economic literature research into values for the demand elasticities.
References
- Billings, R. B., and C. V. Jones. 2008. Forecasting urban water demand. Denver, CO: American Water Works Association.
- Bithas, K., and C. Stoforos. 2006. Estimating urban residential water demand determinants and forecasting water demand for Athens Metropolitan Area, 2000–2010. South-Eastern Europe Journal of Economics 1: 47–59.
- Brandes, O., T. Maas, and E. Reynolds. 2006. Thinking beyond pipes and pumps: Top 10 ways communities can save water and money. Victoria, BC: POLIS Project on Ecological Governance, University of Victoria.
- Brandes, O., S. Renzetti, and K. Stinchcombe. 2010. Worth every penny: A primer on conservation-oriented water pricing. Victoria, BC: POLIS Project on Ecological Governance, University of Victoria.
- Dalhuisen, J. M., R. Florax, H. de Groot, and P. Nijkamp. 2003. Price and income elasticities of residential water demand: A meta-analysis. Land Economics 79(2): 292.
- Dupont, D., and S. Renzetti. 2001. The role of water in manufacturing. Environmental and Resource Economics 18(4): 411–432.
- Dziegielewski, B. 1996. Long-term forecasting of urban water demands. In Marginal cost rate design and wholesale water markets: Advances in the economics of environmental resources, Vol. 1, ed. D. Hall, 33–47. Greenwich, CT: JAI Press.
- Econnics, S. Renzetti, and POLIS Water Sustainability Project. 2011. Conservation-oriented water pricing analysis for the Regional Municipality of York’s Long-Term Water Conservation Strategy: Phase One report. Prepared for York Region, Victoria BC.
- Environment Canada. 2009. Municipal water and wastewater survey: Municipal water use 2006, summary tables. Ottawa, ON.
- Environment Canada. 2011. 2011 Municipal water use report: Municipal water use 2009 statistics. Ottawa, ON.
- Ghiassi, M., D. K. Zimbra, and H. Saidane. 2008. Urban water demand forecasting with a dynamic artificial neural network model. Journal of Water Resources Planning and Management 134: 138.
- Hansen, L. G. 1996. Water and energy price impacts on residential water demand in Copenhagen. Land Economics 72(1): 66–79.
- Jain, A., and L. E. Ormsbee. 2002. Short-term water demand forecast modelling techniques: Conventional methods versus AI. Journal of the American Water Works Association 94(7): 64–72.
- Maddaus, W. O., and L. A. Maddaus. 2006. Water conservation programs: A planning manual. AWWA Manual of Water Supply Practice – M52. Denver, CO: American Water Works Association.
- Malla, P., and C. Gopalakrishnan. 1999. The economics of urban water demand: The case of industrial and commercial water use in Hawaii. International Journal of Water Resources Development 15(3): 367–374.
- Ndah, S. 2010. Forecasting water demand for the Province of Ontario. Masters of Business Economics graduating essay, Department of Economics, Brock University, St. Catherines, ON.
- Rathnayaka, K., H. Malano, S. Maheepala, B. Nawarathna, B. George, and M. Arora. 2011. Review of residential urban water end-use modelling. Paper presented at the 19th International Congress on Modelling and Simulation, Perth, Australia, December 12–16. http://mssanz.org.au/modsim2011 ( accessed December, 2012).
- Renzetti, S. 2002. The economics of water demands. Norwell, MT: Kluwer Academic Press.
- Williams, M., and B. Suh. 1986. The demand for urban water by customer class. Applied Economics 18: 1275–1289.
- Worthington, A., and M. Hoffman. 2008. An empirical survey of residential water demand modelling. Journal of Economics Surveys 22(5): 842–871.
- York Region. 2007. An economic profile of York Region: Population growth and labour force. Newmarket, ON. http://www.york.ca/Business/Economic±Profile/economic_profile_chapter3.htm ( accessed December, 2011).
- York Region. 2010. York Region population projection, MS Excel file YORK-#2388105-v1-Population_Projections, provided by York Region staff. Newmarket, ON.
- York Region. 2011. The Regional Municipality of York long-term water conservation strategy. http://openwater.ca/Reports.aspx ( accessed December, 2011).