Abstract
Preliminary design flood flows have been derived for 24,000 locations throughout Canada on rivers with catchments larger than 400 km2. In the absence of any published consistent method for flood estimation covering Canada, a flood frequency analysis of instantaneous and daily annual maximum flows at 1664 gauging stations has been regionalized using geostatistical methods. The methodology accounts, in a generalized way, for unusual catchments including arid areas in the Prairie Provinces, the influence of extensive lakes, and (implicitly) for the effects of snowmelt. It does not attempt to represent other more local effects such as flow regulation and diversion. Cross-validation has shown that the results exhibit little bias and at half of all locations the predicted results are within ± 25% of those calculated from the flood peak data, for floods with annual exceedance probabilities of both 1 in 2 and 1 in 20. This broad-scale analysis, which was performed as part of a flood mapping study, helps to pave the way for the development of a unified procedure for flood frequency analysis across Canada, which forms part of the FloodNet research initiative.
Des débits fluviaux préliminaires ont été calculés à 24 000 endroits sur l’ensemble du Canada pour les bassins versants mesurant plus de 400 km2. En l’absence d’une méthode cohérente pour l’estimation du risque inondation au Canada, une analyse des débits instantanés et journaliers maximums annuels a été réalisée à 1664 stations de jaugeage en utilisant des méthodes géostatistiques. D’une manière générale, cette méthode prend en compte les bassins hydrographiques particuliers : incluant les zones arides dans les provinces des Prairies, l’influence des grandes étendues d’eau et (implicitement) les conséquences de la fonte des neiges. Elle n’a pas pour but de représenter les effets locaux tels que les systèmes de régulation ou de prévention des crues. La validation croisée a révélé que les résultats comportent quelques biais et que, pour la moitié des zones d’étude, les résultats calculés à partir des débits maximaux prévisionnels pour les périodes de retours de 2 et 20 ans diffèrent de ± 25%. Cette étude à grande échelle menée dans un contexte de cartographie de l’aléa inondation, ouvre la voix au développement d’une procédure commune pour l’étude des inondations sur l’ensemble du territoire Canadien dans le cadre du programme de recherche FloodNet.
Introduction
This paper describes a broad-scale approach to the estimation of design flood flows throughout Canada for the development of an indicative flood hazard map. There are no published Canada-wide methods for flood estimation, partly because flood management is largely carried out at the provincial level. This contrasts with the situation in several other countries where flood management authorities or professional bodies have for many years led the development and updating of officially sanctioned flood hydrology methods (see for example United States Department of Agriculture [USDA] Citation1986; Institute of Hydrology Citation1999; Engineers Australia Citation2015). The situation in Canada is expected to change as a result of the Natural Sciences and Engineering Research Council’s FloodNet research network (Natural Sciences and Engineering Research Council of Canada [NSERC] Citation2015), which includes a project aiming to develop a unified procedure for flood frequency analysis (FFA) across Canada and provide estimates of flood and extreme rainfall quantiles for many locations across Canada.
The development of such comprehensive methodologies for flood estimation has typically required major research studies taking several years to complete. Results from the FloodNet research aimed at developing a flood estimation procedure are not expected until around 2019. In contrast, recent publications have demonstrated the potential for rapid development of simplified hydrological methods using readily available data sets at a continental or even global scale. Smith et al. (Citation2015) carried out regional FFA at the global scale using annual maximum flows from 703 gauges from the Global Runoff Data Centre. Beck et al. (Citation2015) produced global maps of streamflow characteristics by relating flow data from several thousand gauged catchments to climate and physical catchment characteristics. Arheimer et al. (Citation2011) describe the HYdrological Predictions for the Environment (HYPE) model that has subsequently been applied for continental-scale modelling of runoff, including an Arctic model domain that includes most of the area of Canada, and was calibrated using data from 1349 flow gauges (Swedish Meteorological and Hydrological Institute [SMHI] Citation2015).
The method described below, developed and implemented within a short time period, is intended as a preliminary step towards a comprehensive approach to estimating design floods for any location in Canada. Local data and other sources of information were used where possible to develop and adjust national-scale approaches. This is vital given Canada’s large diversity in climate, topography, geology and other physical characteristics.
The objective, dictated by the coverage of the hydraulic methods used to generate the flood map, was to estimate design peak flood flows for a variety of exceedance probabilities at around 24,000 points along Canadian rivers with a catchment area greater than 400 km2. Flood maps for the majority of rivers were produced using a method based on normal depth calculations and a two-dimensional (2D) flood-spreading algorithm (not described here), intended for rapid modelling of continental-scale flood risk. As this requires only a peak flow input, there was no need to determine a shape for the flood hydrograph.
Some hydraulic modelling for urban areas with better quality digital terrain data applied the 2D hydraulic model JFlow (CitationCrossley et al. 2010), which requires a flood hydrograph. Common practice is to calculate design flood hydrographs by rainfall-runoff modelling, although alternative approaches are available in which hydrographs are defined from analysis of the durations of normalized observed hydrographs (O’Connor et al. Citation2014), by fitting mathematical functions to observed hydrographs (Pramanik et al. Citation2010; O’Connor et al. Citation2014) or by analysis of flood volume and duration data (a comprehensive review is given by Gräler et al. Citation2013, and a Canadian example by Javelle et al. Citation2002). For the purpose of the present study, the shapes of design flood hydrographs were represented using a simplified approach based on the estimation of catchment response time, not discussed further in this paper.
Flooding on smaller catchments and pluvial flooding from direct surface runoff (including snowmelt) was modelled using a direct rainfall method, also not covered in this paper.
Design floods were required for annual probabilities of exceedance ranging from 1 in 20 to 1 in 1500, equivalent to return periods of 20 to 1500 years.
General approach
The flow chart in Figure summarizes the steps involved in the analysis.
Design flows were estimated from statistical analysis of flood peak data. This has the significant advantage of avoiding any need to model catchment processes (such as snowmelt) or to consider the nature of the relationship between design rainfall and design flow. While considerable progress has been made in hydrological modelling based on distributed databases, challenges remain in applying this type of modelling framework at the spatial scale undertaken herein. Sampson et al. (Citation2015) outline some of the issues with identifying flood hazards over a large spatial scale using hydrological models, highlighting in particular the need to calibrate a model for locations where suitable calibration data are lacking.
The analysis makes no distinction between floods caused by rainfall, snowmelt, rain on snow or indeed any other combination of processes. This is a common approach (e.g. as suggested in Watt Citation1989) which avoids the considerable difficulties associated with assigning flood-generating mechanisms (e.g. Collins et al. Citation2014) and attempting to fit separate distributions to split samples. An index flood approach was adopted, with regional FFA techniques being applied to generalize the method and allow the estimation of design floods for ungauged basins.
Although there are various existing methods for regional FFA in Canadian provinces (Glaves and Waylen Citation1997; Rollings Citation1999), there is no Canada-wide approach that is used in current practice. Some provinces do not have current regional methods available. For these reasons, a simplified regional FFA method was developed.
Development of flood peak data set
Flood peak data for several thousand gauging stations were obtained from the Water Survey of Canada’s hydrological database HYDAT, aiming to identify flow records that have acceptable quality of measurement of flood flows, sufficient length for FFA, and are thought to be suitable for FFA.
There are 3367 stations in HYDAT that have instantaneous annual maximum (AMAX) flows available. 2328 of these have at least 10 AMAX values, which was imposed as a minimum record length for the estimation of the index flood. A more stringent minimum length of 20 years was set for the estimation of flood growth curves, as discussed later. Analysis is restricted to annual maximum data, as opposed to peaks over threshold (POT) data, since POT data were not available, and a very large amount of work would have been required to identify appropriate thresholds and extract independent peaks for such a large number of gauging stations.
HYDAT also contains an alternative set of AMAX flows, calculated from daily mean flow data. There are 3153 gauges with at least 10 years of data of this type. Some of these records overlap completely with those with instantaneous AMAX data, but there are other gauges that provide daily data and no instantaneous data, or that have longer daily records than instantaneous records. A total of 1454 gauges have daily AMAX records that are at least 10 years longer than their instantaneous AMAX records. This is a potentially valuable additional source of data.
However, on rapidly responding catchments the peak flow calculated from daily mean data can be significantly lower than the instantaneous peak flow. Daily data from such catchments was excluded from the analysis. This was accomplished by checking for consistency between alternative estimates of the median annual maximum flood, QMED. The following sets of daily gauges were included, where they provide at least 10 years more annual maximum flows than available from instantaneous data:
(1) | Gauges on catchments between 200 and 10,000 km2 where estimates of QMED from daily data and from instantaneous data, calculated only for years with both data types available, agree to within 5%. There are 135 such gauges. They have been used to provide longer flood peak records than those available from instantaneous data; | ||||
(2) | All 287 gauges on catchments larger than 10,000 km2. On such catchments, flood response can be expected to be gradual enough to allow reliable approximation of the peak flow using daily data. This can be seen in Figure , where there are few catchments of this size for which the two types of flow data result in differences in QMED exceeding 5–10%. Most of these 287 gauges do not have AMAX flows from instantaneous data available. |
Figure 2. Comparison of median annual flood estimated from daily and instantaneous annual maximum flows.
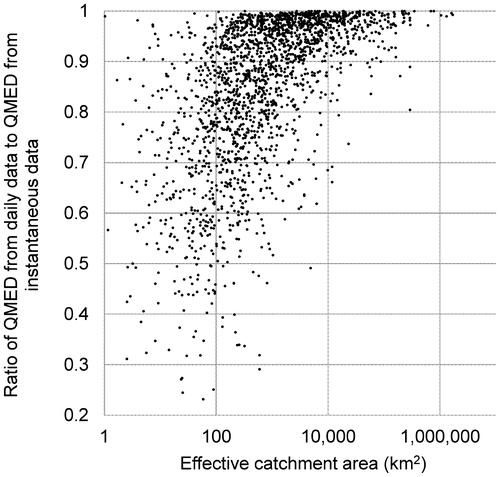
The final number of gauges analyzed was smaller than the numbers quoted above, because some gauges were removed during the process of checks described below, and others were excluded as they were on small catchments.
Figure shows the locations of gauges providing suitable flood peak data (including those on smaller catchments). It can be seen that the inclusion of daily data fills in a large gap in the coverage of instantaneous gauges in Quebec. Although there is a reasonable number of gauges north of 60°, there are hardly any in the Arctic Archipelago.
The following checks on the data set were performed:
(1) | Stations with significant numbers of zero AMAX flows were investigated. Those where more than half of the AMAX values are zero were removed from the analysis, to avoid zero values for the median, QMED. Most such gauges are in the southern Prairie Provinces, and zero AMAX values appear to correspond generally with drought years. It was necessary to ensure that the fitting of extreme value distributions could cope with zeros, as discussed below. | ||||
(2) | Stations with particularly low or high ratios of maximum flow (QMAX) to QMED were investigated. Some stations with extremely high ratios are found in southeastern Alberta, in an arid area where most AMAX are zero or very close to zero. The highest ratio of QMAX/QMED is 908, and there are seven others with ratios over 50. Most were removed on the basis that QMED is probably not accurately estimated given the difficulties of measuring very low flows in significant-sized river channels. QMED is probably not a useful index flood for these highly unusual catchments, and it has been suggested that the 1 in 5 or 1 in 10 annual probability floods are more appropriate indices for arid regions (Farquharson et al. Citation1992; Engineers Australia Citation2015). | ||||
(3) | Stations on rivers obviously affected by flow diversions or on branch channels were generally removed from the analysis. There are major flow diversions in parts of Canada, built for purposes including hydro-electric generation, irrigation and flood management. Examples include the diversion of the upper Churchill River into the Nelson River, the Portage la Prairie diversion upstream of Winnipeg, diversions affecting La Grande River as part of the James Bay hydro scheme and numerous diversions in the Prairies for irrigation schemes. It is unrealistic to expect a national-scale study to account adequately for the effects of flow diversions. The estimated design flows on rivers subject to flow diversions are therefore highly uncertain. | ||||
(4) | A sample of stations on other rivers classed as regulated was investigated. Most showed apparent regular fluctuations in flow due presumably to hydropower releases, but there were few with evident significant effects on peak flow, so most were retained in the analysis. Thirty percent of rivers with AMAX flows are classed as regulated. They were treated the same as non-regulated rivers, but the results of the analysis were subsequently examined to check whether regulated rivers have any statistically significant differences from otherwise similar non-regulated rivers. | ||||
(5) | Stations with missing catchment areas, for example those located on outlet channels from lakes with multiple outlets, were generally removed. | ||||
(6) | Stations with very large residuals when predicting QMED by regression on effective drainage area were investigated in some detail. Extreme over-prediction of QMED was found at some stations in the southern Prairie Provinces. Improved values for effective drainage areas were obtained for these stations. |
After the checks listed above, the data set included 2417 gauging stations. Of these, 1664 are on catchments larger than 200 km2. The analysis focused solely on these gauges to remove the influence of very small catchments, given that results were needed primarily for catchments larger than 400 km2.
Figure illustrates the distribution of record lengths used in the analysis.
Determination of catchment areas
Total (gross) catchment areas are available in HYDAT for nearly all gauges. Several hundred gauges have different gross and effective catchment areas. At most of them the effective area is more than 5% smaller than the gross. The significance of effective catchment areas was investigated in some detail. The effective drainage area is defined as the portion of a drainage basin that is expected to contribute runoff during a flood of annual exceedance probability of 1 in 2 (Godwin and Martin Citation1975). Figure indicates the importance of accounting for effective catchment areas in estimation of QMED from catchment properties: QMED is overestimated on all catchments where the effective area is much smaller if it is predicted from gross area.
Figure 5. QMED plotted against gross catchment area, highlighting catchments with a large difference between effective and gross areas.
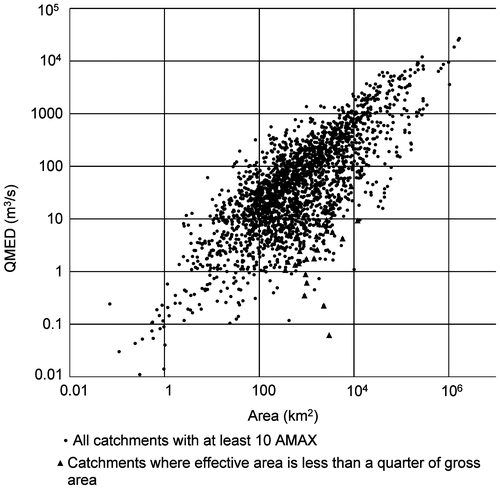
On some catchments, no runoff may occur from non-contributing (ineffective) portions even during more extreme flood events. However, in general one would expect the contributing area to increase with flood magnitude, hence expecting low values of QMED and steep flood growth curves on catchments with a large proportion of non-contributing drainage area. This is reflected in the AMAX data: for the catchments where effective area is less than a quarter of gross, the (geometric) mean ratio of the maximum to the median annual maximum flow is 8.96. On the full set of catchments with at least 10 AMAX, the corresponding ratio is 3.04. Guidelines on extreme flood estimation suggest leaning toward the gross catchment area when estimating the probable maximum flood (Alberta Transportation Citation2004).
Improved values of effective drainage area were obtained from the Agriculture and Agri-food Canada (AAFC) Watersheds Project (Agriculture and Agri-food Canada Citation2013). The ratio of AAFC to HYDAT effective areas ranges from 0.28 to 3.14. There are some gauges for which effective areas are provided by AAFC but are not in HYDAT. These effective areas are as small as 0.05 times the HYDAT gross areas. Adopting the effective area rather than the gross area for gauged catchments should greatly help the regression of QMED on catchment area.
For ungauged catchments for which design flows are required, effective areas were derived by calculating topographic catchment boundaries from a composite digital terrain model (DTM) with a 30m grid resolution, using the SCALGO terrain processing software (SCALGO ApS Citation2013), and then removing non-contributing drainage areas using a digital map layer from the AAFC Watersheds Project.
Estimation of the index flood
Overview of approach
The index flood was chosen to be QMED, which has an annual exceedance probability of 1 in 2, i.e. 0.5. The median has several advantages over the mean, a principal one being its robustness in the presence of outliers.
QMED was estimated directly as the median of the AMAX flows at suitable gauging stations. To generalize the calculation to ungauged locations, QMED was estimated using a two-step process:
(1) | Development of a regression model; | ||||
(2) | Application of adjustment factors to account for regional and local variations in climate, soils, geology and other factors not included in the regression. |
Rather than attempting to develop a complex multiple regression model with numerous potential explanatory variables, QMED was estimated by regression on just two variables: effective catchment area (AREA) and an index of lake attenuation (FARL, defined below).
The logic for choosing these two variables is that they can both take very different values even for nearby stations/catchments. It is consistent with earlier work in Newfoundland (Rollings Citation1999) and Ontario and Quebec (Groupe de research en hydrologie statistique [GREHYS] Citation1996) – although neither of those studies was faced with the sort of extreme variation in climatic characteristics that a Canada-wide study faces. Rollings (Citation1999) found that lake attenuation was the most influential factor on design flows after basin area. Many other variables that are expected to influence QMED are spatially coherent: examples include climate, altitude, slope, distance inland, geology, soils and vegetation. There is also correlation between many of these variables.
For these reasons, and to avoid the need to seek out additional data sets and calculate catchment-average values of descriptor variables, the influence of these and other variables was accounted for implicitly by spatial interpolation of the residuals from the regression. Interpolation of the residuals between gauging station sites was intended to derive local adjustment factors for QMED, thus combining catchment attributes and spatial proximity in a process known as georegression (Faulkner and Prudhomme Citation1998; Merz and Blöschl Citation2005). It is recognized that in data-sparse areas this approach cannot be expected to properly represent the influence of any local variations in rainfall, elevation, gradient, etc. The interpolation of the residuals is described below.
Index of lake attenuation
Previous studies in Canada have developed various indices of the influence of lakes on flood peaks, including the surface areas of lakes and swamps (GREHYS Citation1996), the proportion of catchment area controlled by lakes and swamps (GREHYS, Citation1996; Rollings Citation1999) and a lake attenuation factor (LAF), which is an additive index of factors calculated for individual lakes, accounting for the area of each lake, the area draining to each lake and the total catchment area (Rollings Citation1999).
An index similar to LAF but multiplicative rather than additive was applied for the present study. It is denoted FARL, flood attenuation by reservoirs and lakes. It was first developed for the UK Flood estimation handbook (FEH; Institute of Hydrology Citation1999, vol. 5) and has also been utilized for flood estimation in Ireland (Mills et al. Citation2014).
FARL is defined as the product of an attenuation index α for each lake or reservoir in a catchment:
(1)
The index for an individual lake is defined as follows:
(2)
where r is the relative size of the lake to its subcatchment, i.e.
(3)
and w is a weight that reflects the importance of the lake in terms of the flood behaviour at the catchment scale:
(4)
(Institute of Hydrology Citation1999, vol. 5).
A data set of lake extents was obtained from the National Scale Frameworks data sets (Natural Resources Canada Citation2015). Lakes with a surface area of less than 20 km2 (5% of the minimum catchment area for which design flows were required) were excluded, as were some rivers, estuaries and islands that were found in the data set. This process reduced the number of water features in the data set from 128,633 to 2469. The variables required for the calculation of FARL were calculated using SCALGO and ArcGIS. The most difficult step was to work out which lakes lie within the catchment of each gauging station and each ungauged location. There is no comprehensive set of digital catchment boundaries currently available for Canadian gauging stations. Boundaries were derived using SCALGO, a preliminary step being to snap gauging stations to the closest flow path derived from the DTM. The DTM flow paths form a synthetic drainage network in vector format, generally but not always corresponding to the position of real watercourses. Adjustments were necessary for some catchments, for example for gauges near confluences, where the closest flow path may represent a tributary rather than the main river whose flow the gauge is measuring. Given the national scale of the approach developed herein, it was not possible to investigate and correct all discrepancies in catchment boundaries, and so some FARL values for gauges are likely to be erroneous. Nevertheless, 83% of the derived catchment boundaries had areas within 20% of the published area. Note that all calculations requiring a value of catchment area for gauges were based on the published areas and not on the outputs from SCALGO, which were used only to identify which lakes fell within each catchment.
Regression for QMED
Equation Equation5(5) was fitted to the 1664 gauges selected for analysis, using least squares in the logarithmic domain:
(5)
R2 is 0.58 and both variables are significant at the 0.001 probability level. The standard error of the fitted model measured on the log scale is 1.225, resulting in a factorial standard error (FSE) of 3.40.
Equation Equation5(5) has only a limited ability to explain the variance in QMED. The R2 is relatively low, and the FSE relatively high, compared with other regression models for QMED that cover smaller geographical areas and include many more candidate variables. For example, the UK FEH currently uses a regression model with R2 0.94 and FSE 1.43 (Environment Agency Citation2008). This discrepancy in performance should not be a surprise in light of the wide variety of climatic and geographical characteristics across Canada, most of which were not considered in developing the above equation. The equation should not be applied without accounting for the spatial variation in residuals, as described below.
The exponent for the AREA term, 0.817, is similar to that which has been found in other studies. Glaves and Waylen (Citation1997) found 0.74 for southern Ontario. In the UK, the FEH equation has an exponent of 0.85.
Residuals
Residuals shown in Figure are differences between the natural logs of predicted and observed QMED, plotted at the centroid of each gauged catchment. High values indicate under-estimation of QMED.
Figure 6. Residuals in the QMED regression equation plotted at the centroid of each gauged catchment.
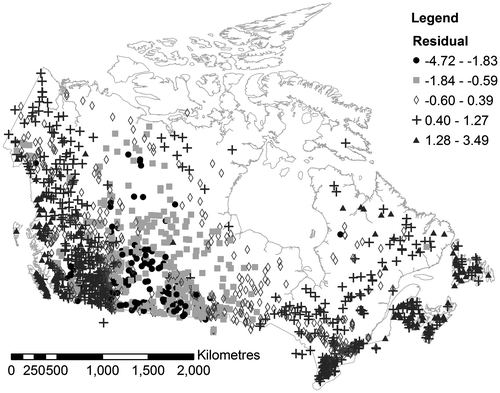
A large degree of spatial coherence can be seen in the residuals. As expected, prediction of QMED from AREA and FARL results in under-estimation in the Maritime Provinces and around the Rocky Mountains, where precipitation is higher than elsewhere. There is also substantial under-estimation in southern Ontario and in parts of the far north. QMED is over-estimated in the more arid interior, particularly in the southern Prairies, and also in low-lying parts of the Fraser River catchment in southern British Columbia, which lies in the rain shadow of the coastal mountain ranges.
The mean residual for non-regulated rivers is +0.19 and the mean for regulated rivers is −0.34. A possible explanation is that the regression results in larger errors on regulated rivers, with a tendency to over-estimate QMED. However, to interpret this finding properly it is important to realize that the subset of regulated catchments is not representative of the entire set of gauged catchments. For example, regulated catchments tend to be larger, with a mean area over six times that of non-regulated catchments. It is, however, reasonable to expect that the regression will be less reliable where river flow is artificially influenced.
The residuals were interpolated, using ordinary kriging, to a grid, with 12 surrounding data points used to estimate the value at each grid square. For the purpose of interpolation, each residual was located at the centroid of the catchment corresponding to the gauging station, rather than at the site of the gauging station. The centroid is a more appropriate reference point since the peak flow data recorded at a gauge reflect the geographical characteristics of the upstream catchment. For larger catchments the centroids can be located a long way from the gauge, just as the bulk of the runoff can be derived from headwater areas distant from the gauging point.
The grid of interpolated residuals permits the identification of an adjustment factor for QMED at the location of the centroid of every ungauged catchment.
Flood growth curves
Fitting at individual sites
Flood growth curves were fitted to annual maximum flows using the method of L-moments (Hosking and Wallis Citation1997). The method of analysis assumes that annual maximum flows at a given gauge are independent and identically distributed. The assumption of independence may not hold for large river basins with considerable storage (in lakes, etc.), which can exhibit serial dependence between annual maxima (as discussed, for example, for the St Lawrence River by Klemeš Citation2011). The assumption of identical distribution will not hold for rivers where AMAX flows are non-stationary, whether due to climate change, land-use change or other human intervention. Tan and Gan (Citation2015) found that abrupt changes are more common than gradual trends in Canadian AMAX data, partly because many rivers began to be regulated in the twentieth century. They recommended non-stationary FFA for future work.
Choice of extreme value distribution
Previous studies have investigated a range of extreme value distributions for fitting to annual maximum flows in Canada. The log-Pearson III (LP3) distribution has been recommended for use in Canada, the USA and Australia. Guidelines for Alberta (Alberta Transportation Citation2001) quote such recommendations from US agencies but state that this choice has not met with general acceptance in Canada and that the log normal (LN), log normal III (LN3), extreme value type I (EV1) and LP3 distributions are used in various parts of Canada. The guidelines recommend, for single-site FFA, that the set of candidate distributions should at least include LN, EV1 and LP3.
A Newfoundland study (Rollings Citation1999) used the generalized extreme value (GEV) and LN3 and found that LN3 fitted better on 68% of watersheds. Glaves and Waylen (Citation1997) found that the GEV was a good fit in Southern Ontario, fitted using L-moments. The GEV was found to be a better fit than other three-parameter distributions for regional analysis in Ontario and Quebec (GREHYS, Citation1996).
For the present study, four candidate distributions were considered: the GEV, generalized logistic (GLO), generalized normal (GNO), which has the same distribution as the LN3 with slight parameter modifications, and Pearson type III (PE3). All have three parameters, although the first parameter is not needed when expressing the distribution as a growth curve, as QMED substitutes for it. For simplicity it is desirable to choose a single distribution for all of Canada, although the choice of distribution was tested using regional subsets of gauges, in recognition of the possibility that different distributions may be more appropriate in different regions, for example to account for variations in flood-generating processes.
The goodness of fit of the distributions was assessed using L-moment ratio diagrams (Hosking and Wallis Citation1997). Sample L-moments were calculated from each annual maximum series and compared with theoretical combinations of L-moments for various distributions.
Figure compares the sample L-skew and L-kurtosis for every gauge in the data set. Three-parameter distributions plot as a line, as can be seen. It can be seen that the GEV and GNO distributions both provide a near-exact match to the mean of the sample L-moments. This might be expected from averaging sample L-moments from such a large and heterogeneous region, although similar results were found for most individual provinces, the exceptions being Prince Edward Island, with only 14 gauges, and Saskatchewan, where the GNO fitted better than the GEV. The GLO appears to have rather higher L-kurtosis than the majority of stations.
Figure 7. L-moment ratios for all gauging stations in Canada with at least 20 years of flood peak data. Each circle represents a gauging station.
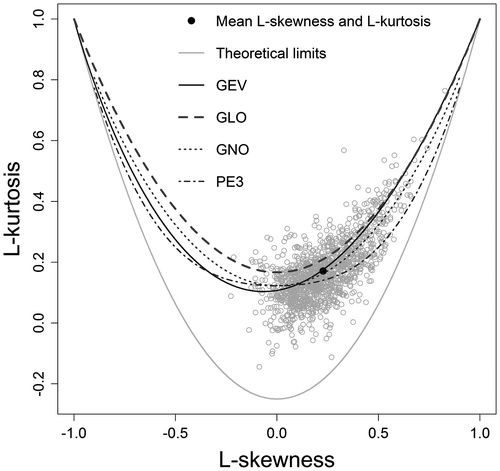
A limitation of this approach to judge goodness of fit is that it is subjective. Any future work could consider more objective measures such as the goodness of fit statistic developed by Hosking and Wallis (Citation1997).
On the basis of these results and findings from previous studies, the GEV distribution was selected for all of Canada. It was applied in the form of a growth curve standardized by QMED, the formula for which can be found in volume 3 of Institute of Hydrology (Citation1999).
Coping with zero AMAX flows
A conditional probability method was implemented to exclude the influence of AMAX flows of zero, which occur from time to time on very dry catchments, particularly in the southern parts of the Prairie Provinces.
The general approach is as described in Alberta Transportation (Citation2001). It involves first excluding the zero-flow years from the series and fitting a conditional probability distribution, G(x), to the remainder – that is, conditional on the stream not being dry for the year. The probability of an AMAX flow equalling zero, p0, is then estimated as the proportion of zero-flow years in the original record. Finally the unconditional probability distribution F(x) is calculated from the conditional probability distribution G(x) using the formula:
(6)
This conditional probability method was applied throughout all of Canada, although in the vast majority of areas p0 = 0, in which case the conditional and unconditional distributions are equal. The probability p0 was treated as an additional parameter, with its value estimated from AMAX data at every gauging station, and interpolated on to a grid as for the L-moments described below. This enables the estimation of p0 at all ungauged sites. The highest values are found in the southern Prairies, where p0 takes values up to 0.09.
Regionalization
Regional FFA typically involves developing a method for grouping gauging stations, either geographically or according to their catchment properties, and analyzing homogeneous groups in order to obtain pooled flood-growth curves.
To fulfil the aims of the present study, a simplified approach was applied in which sample L-moments for gauged catchments were mapped and interpolated to grids using the method of ordinary kriging in which the value of the variable at each grid point is calculated as a weighted average of the surrounding observations, with greater weight allocated to nearby data points and to those in directions where there are few other data points. A maximum of 12 surrounding data points was considered for the averaging process at each grid point.
The interpolation approach is equivalent to regional FFA using a region-of-influence method (cf. Burn Citation1990) with regions, i.e. groups of gauging stations, selected on the basis of geographical proximity between catchments. However, no attempt was made to test alternative ways of grouping stations or to evaluate the homogeneity of the groups.
The approach applied is similar to that applied by Glaves and Waylen (Citation1997) who investigated the relationship between GEV parameters and catchment area in Ontario, finding a slight increase in the GEV shape parameter with increasing area. They plotted maps of GEV parameters and found that geographic trends were more significant than those associated with catchment size. Spatial variations were thought to be related to the relative importance of snowmelt. Similarly, Merz and Blöschl (Citation2005) found spatial proximity to be a significantly better predictor of regional flood frequencies in Austria than catchment attributes, and developed a method based on kriging that combines spatial proximity and catchment attributes, accounting for record length.
In order to calculate statistics suitable for the creation of flood growth curves, L-moments were expressed as ratios, i.e. t2 = L-CV/L-mean where CV denotes coefficient of variation and t3 = L-skewness/L-mean. It was found that there was some correlation between the L-moment ratios and catchment area, with the largest catchments not showing the high values of L-CV and lower L-skewness that can be found on small to medium catchments. This effect has been reported elsewhere (e.g. Kjeldsen et al. Citation2008; Smith et al. Citation2015) and the likely explanation is the fact that larger catchments tend not to be subject to catchment-wide precipitation that can give rise to extreme flood flows.
Figures and plot L-moment ratios at the centroids of gauged catchments. For the FFA, only the 1302 gauging stations with at least 20 years of data were considered, in order to ensure an acceptably robust estimate of the L-moment ratios, particularly t3. The choice of 20 years as a minimum record period is consistent with the criterion applied by Javelle et al. (Citation2002).
The spatial distribution of the two L-moment ratios is similar, and can be seen to generally follow that of the QMED residuals. The highest L-CV and skewness values, indicating steep and concave flood growth curves, are found in the Prairies, where QMED is lower than expected from the regression. This is as expected from the nature of the climate in this area: generally dry conditions but occasional floods that are much more severe than the average, resulting from occasional extreme meteorological conditions. Similar results, i.e. steeper flood frequency curves in dry regions, were found on a global scale by CitationSmith et al. (2015).
There appears to be rather less spatial consistency in the distribution of L-skewness, with moderately high values seen at a large number of gauges in British Columbia, the North and the Atlantic provinces, as well as across the Prairies. This may be a genuine effect, but it could also be influenced by sampling variability given the error inherent in estimation of L-skewness from records as short as 20 years. The L-skewness ratio takes small positive values for most gauges (0.08 to 0.20), indicating flood growth curves with little curvature (the GEV distribution is bounded above for L-skewness < 0.17).
As for the interpolation of QMED residuals, the estimated L-moments at each ungauged location were calculated as a weighted average of L-moments at gauging stations whose catchment centroids are close to that of the ungauged catchment.
Validation checks and uncertainty of the results
Validation
Because design flows are by their very nature statistical estimates rather than quantities that it is possible to measure, it is not possible to validate them in the usual sense of comparing predictions with observations. However, an indication of the performance of the methodology can be obtained by comparing the results of the procedure for estimation of design flows for ungauged catchments with estimates made directly from flood peak data. Confidence limits for the latter were constructed using Monte Carlo simulation. For the 1 in 20 annual exceedance probability, the 90% confidence interval, averaged over all gauges used in the analysis, ranged from 0.79 to 1.23 times the best estimate of the GEV quantile. This indicates that, as expected, the design flows can be treated with reasonably high confidence where the annual exceedance probability of interest is not too low in comparison with the length of the flood peak record.
A process of leave-one-out cross-validation was applied to assess the results at ungauged sites, in which each gauging station was treated as an ungauged site, with QMED estimated by regression and adjusted using a residual interpolated by kriging from surrounding gauged sites, ignoring data at the site of interest. Similarly, the parameters needed for estimating the growth curve were interpolated from surrounding sites. Since each individual gauge has a negligible impact on the regression for QMED, there was no need to carry out leave-one-out cross-validation to remove the effect of each gauge on the regression model for QMED.
Design flows estimated directly from the flood peak data were compared with those predicted using the ungauged catchment method, for all gauging stations with at least 20 years of data. Results were compared for annual exceedance probabilities 1 in 2 and 1 in 20. Figures and show the results, distinguishing regulated and non-regulated rivers.
Summary statistics are given in Table and explained and discussed below. The bias is expressed as a percentage where negative values indicate that the predicted flow is less than that calculated from the flood peak data. The averaging is carried out using a geometric mean in this case. Taking all gauges together, there is a slight negative bias (2–3%) in the results. Surprisingly, there is more of a bias in non-regulated rivers than in regulated rivers. As already noted, in examining the performance of the method on regulated catchments it is important to consider the possibility that catchments that are regulated tend to differ from unregulated catchments: for example, they tend to be larger and to exhibit greater attenuation due to reservoirs and lakes. It is possible that differences in the results between regulated and non-regulated catchments are explainable to some extent by differences in the characteristics or location of the two sets of catchments.
Table 1. Summary of cross-validation results for ungauged catchments.
RMSE is the root mean square error, and is calculated on a log scale, i.e. from comparing the natural logarithm of the results. The RMSE decreases slightly for the 1 in 20 annual probability flood in comparison with the 1 in 2. Although this may appear counterintuitive, it should be remembered that this test takes no account of the uncertainty in the fitted flood frequency distributions, which will inevitably increase as the exceedance probability diminishes. As might be expected, the results for regulated rivers show larger RMSE than non-regulated rivers. It can be seen from Figures and that the largest outliers in the results are on regulated rivers. The RMSE for non-regulated rivers is very similar for the two exceedance probabilities, whereas RMSE decreases slightly with reducing probability for regulated rivers. A tentative explanation is that the effects of regulation are less significant on larger floods, although the effect is very weak.
The final two columns indicate the range of ratios of predicted to observed results. The 95% range is the range that encompasses 95% of the gauges in the sample, i.e. only 5% of gauges have predictions that fall outside these limits. The ratios are calculated as predicted flow divided by the direct estimate from the flood peak data. It is encouraging that 95% of gauges have predicted results that fall within the range of 3 times smaller or larger than the results obtained directly from the flood peak data for the 1 in 20 annual probability flood. This indicates that gross over- or under-predictions are rare. For half of the gauging stations the predicted results are within ± 25% of those calculated from the flood peak data, for both the 1 in 2 and the 1 in 20 annual probability floods.
The performance of the method for many areas of interest is expected to be better than indicated by the results of the above tests, since many areas of flood risk are expected to be in urban areas on major rivers where there are gauging stations. When the results from the generalized method are compared with those obtained directly from the flood peak data at each gauge, without leaving the gauge out of the interpolation process, the RMSE for the 1 in 20 annual probability flood reduces from 0.535 to 0.456 and the 95% range of ratios narrows from 0.33–2.96 to 0.40–2.82.
Discussion of uncertainty
Even at locations where high-quality river flow data are available and it is possible to carry out an in-depth study focused on the particular catchment, design flood flows are uncertain – all the more so for low probabilities. The present study has used a highly generalized method to cover the whole of Canada, which inevitably increases the uncertainty in the results.
The uncertainty will vary with catchment type and location. For example, it is larger for regulated catchments and is also expected to be large for catchments that are unusual in other ways, for example urbanized. Examination of the cross-validation results shows no obvious relationship between uncertainty and catchment size.
There is potential for reducing the uncertainty without needing to carry out detailed localized studies; some avenues worth exploring are suggested below.
Conclusions
This paper presents a technique that can be used to develop design flood estimates applicable to all watercourses in Canada. The approach demonstrates the potential for rapid development of methods for prediction in ungauged basins, using readily available data sets, and drawing on other sources of information to account for local conditions as far as possible. The work can be viewed as an initial step in the development of a more comprehensive flood estimation manual for Canada, such as is envisioned as part of the FloodNet research program.
The following observations are made with respect to future developments of the methodology:
• | There is potential to extract more information from the daily flow maximum database, which often has considerably more years of record. One approach is to treat daily readings as censored data, i.e. using the knowledge that the instantaneous peak is at least as high as the daily reading (Micevski et al. Citation2005). Alternative approaches include regression and piecewise scaling (Ding et al. Citation2014). | ||||
• | Non-stationary FFA (e.g. the approach of Tan and Gan Citation2015) may be beneficial for some gauges (see also publications by O’Brien and Burn Citation2014; Yu et al. Citation2015); alternatively, records with significant non-stationarity could be removed from the analysis. Additionally, the projected effects of climate change on flood flows should be considered if the flood estimates are to be used for applications which need to be future-proof such as the design of infrastructure or flood defences. | ||||
• | The calculation of FARL values for gauged catchments could be improved by addressing discrepancies in catchment boundaries. | ||||
• | It would be worth investigating ways of improving the regional analysis, comparing the performance of alternative methods including a region of influence approach, interpolation in a higher dimensional space (for example with catchment area as a third dimension) and alternative kriging methods that account for uncertainty in the estimated L-moments, such as kriging with uncertain data (Merz and Blöschl Citation2005). | ||||
• | An alternative approach to flood frequency estimation in arid areas would be worth considering, in particular when considering low-probability floods in catchments dominated by non-contributing areas. Publications by Shook and Pomeroy (Citation2011) and MacCulloch and Whitfield (Citation2012) illustrate the complex flood response of arid prairie areas with extensive depressions. It may be that a peaks-over-threshold technique is more appropriate, as adopted for arid areas of Australia (Engineers Australia Citation2015). | ||||
• | Where areas of significant flood risk are affected by rivers with flow diversions or other major artificial influences, local specific studies will be required to properly account for the effects of these influences. |
Acknowledgements
The work described in this paper was funded by the JBA Group and the Insurance Bureau of Canada.
References
- Agriculture and Agri-food Canada. 2013. ISO 19131 AAFC Watersheds Project – 2013 – Data product specification. http://www.agr.gc.ca/atlas/supportdocument_documentdesupport/aafcWatersheds2013/en/ISO_19131_AAFC_Watersheds_Project_2013_Data_Product_Specification.pdf (accessed March, 2015).
- Alberta Transportation. 2001. Guidelines on flood frequency analysis. Alberta Transportation, Transportation and Civil Engineering Division, Civil Projects Branch.Alberta
- Alberta Transportation. 2004. Guidelines on extreme flood analysis. Alberta Transportation, Transportation and Civil Engineering Division, Civil Projects Branch. Alberta
- Arheimer, B., J. Dahné, G. Lindström, L. Marklund, and J. Strömqvist. 2011. Multi-variable evaluation of an integrated model system covering Sweden (S-HYPE). In Conceptual and modelling studies of integrated groundwater, surface water, and ecological systems, Proceedings of Symposium H01 held during IUGG2011 in Melbourne, Australia, July 2011, 145–150, ed. Corinna Abesser, Gunnar Nützmann, Mary Hill, Günter Blöschl and Elango Lakshmanan. IAHS Publication 345, Wallingford, UK.
- Beck, H. E., A. de Roo, and A. I. J. M. van Dijk. 2015. Global maps of streamflow characteristics based on observations from several thousand catchments. Journal of Hydrometeorology 16: 1478–1501. doi:10.1175/JHM-D-14-0155.1.
- Burn, D. H. 1990. Evaluation of regional frequency analysis with a region of influence approach. Water Resources Research 26: 2257–2265.
- Collins, M. J., J. P. Kirk, J. Pettit, A. T. DeGaetano, M. S. McCown, T. C. Peterson, T. N. Means, and X. Zhang. 2014. Annual floods in New England (USA) and Atlantic Canada: Synoptic climatology and generating mechanisms. Physical Geography 35: 195–219.
- Crossley, A., R. Lamb, and S. Waller. 2010. Fast solution of the shallow water equations using GPU technology. In Proceedings of the British Hydrological Society third international symposium, managing consequences of a changing global environment. British Hydrological Society, London, UK.
- Ding, J., U. Haberlandt, and J. Dietrich. 2014. Estimation of the instantaneous peak flow from maximum daily flow: A comparison of three methods. Hydrology Research 46 (5): doi:10.2166/nh.2014.085.
- Engineers Australia. 2015. Peak discharge estimation. Book 3, Australian Rainfall & Runoff. Draft, March. The Institution of Engineers, Australia, Barton, ACT.
- Environment Agency. 2008. The improved FEH statistical method. Environment Agency R&D Report SC050050/TR. Bristol: Environment Agency.
- Farquharson, F. A. K., J. R. Meigh, and J. V. Sutcliffe. 1992. Regional flood frequency analysis in arid and semi-arid areas. Journal of Hydrology 138: 487–501.
- Faulkner, D. S., and C. Prudhomme. 1998. Mapping an index of extreme rainfall across the UK. Hydrology and Earth System Sciences 2: 183–194.
- Glaves, R., and P. R. Waylen. 1997. Regional flood frequency analysis in Southern Ontario using L-moments. The Canadian Geographer 41: 178–193.
- Godwin, R. B., and F. R. J. Martin. 1975. Calculation of gross and effective drainage areas for the Prairie Provinces. In Canadian Hydrology Symposium – 1975 Proceedings. Winnipeg.
- Gräler, B., M. J. van den Berg, S. Vandenberghe, A. Petroselli, S. Grimaldi, B. De Baets, and N. E. C. Verhoest. 2013. Multivariate return periods in hydrology: A critical and practical review focusing on synthetic design hydrograph estimation. Hydrology and Earth System Sciences 17: 1281–1296.
- Groupe de research en hydrologie statistique (GREHYS). 1996. Presentation and review of some methods for regional flood frequency analysis. Journal of Hydrology 186: 63–84.
- Hosking, J. R. M., and J. R. Wallis. 1997. Regional frequency analysis: An approach based on L-moments. Cambridge: Cambridge University Press.
- Institute of Hydrology. 1999. Flood estimation handbook. 5 vols. Wallingford: Institute of Hydrology.
- Javelle, P., T. B. M. J. Ouarda, M. Lang, B. Bobée, G. Galéa, and J. M. Grésillon. 2002. Development of regional flood-duration-frequency curves based on the index-flood method. Journal of Hydrology 258: 249–259.
- Kjeldsen, T. R., D. A. Jones, and A. C. Bayliss. 2008. Improving the FEH statistical procedures for flood frequency estimation. Report SC050050. Bristol: Environment Agency.
- Klemeš, V. 2011. Common sense and other heresies. In Selected papers on hydrology and water resource engineering, ed. C. David Sellars. Cambridge: Canadian Water Resources Association.
- MacCulloch, G., and P. H. H. Whitfield. 2012. Towards a stream classification system for the Canadian Prairie Provinces. Canadian Water Resources Journal 37(4): 311–322.
- Merz, R., and G. Blöschl. 2005. Flood frequency regionalisation – spatial proximity vs. catchment attributes. Journal of Hydrology 302: 283–306.
- Micevski, T., G. Kuczera, and S. W. Franks. 2005. Flood frequency censoring errors associated with daily-read flood observations. Water Resources Research 41: W07002. doi:10.1029/2004WR003881.
- Mills, P., O. Nicholson, and D. Reed. 2014. Physical catchment descriptors. Volume IV, Flood studies update technical research report. Dublin: Office of Public Works.
- Natural Resources Canada. 2015. Free data – GeoGratis. http://geogratis.gc.ca (accessed March, 2015).
- Natural Sciences and Engineering Research Council of Canada (NSERC). 2015. Floodnet – NSERC Network – Enhanced flood forecasting and management capacity in Canada. http://www.nsercfloodnet.ca/ (accessed June, 2015).
- O'Brien, N. L., and D. H. Burn. 2014. A nonstationary index-flood technique for estimating extreme quantiles for annual maximum streamflow. Journal of Hydrology 519: 2040–2048.
- O’Connor, K., M. Goswami, and D. Faulkner. 2014. Hydrograph analysis. Volume III, Flood studies update technical research report. Dublin: Office of Public Works.
- Pramanik, N., R. K. Panda, and D. Sen. 2010. Development of design flood hydrographs using probability density functions. Hydrological Processes 24: 415–428.
- Rollings, K. 1999. Regional flood frequency analysis for the island of Newfoundland. St. John's: Hydrologic Modelling Section, Water Resources Management Division, Department of Environment and Labour, Government of Newfoundland and Labrador.
- Sampson, C. C., A. M. Smith, P. B. Bates, J. C. Neal, L. Alfieri, and J. E. Freer. 2015. A high-resolution global flood hazard model. Water Resources Research 51 (7358–7381): 2015W. doi:10.1002/R016954.
- SCALGO ApS. 2013. SCALGO hydrology documentation. Release 1.4.0.
- Shook, K. R., and J. W. Pomeroy. 2011. Memory effects of depressional storage in northern Prairie hydrology. Hydrological Processes 25: 3890–3898.
- Smith, A., C. Sampson, and P. Bates. 2015. Regional flood frequency analysis at the global scale. Water Resources Research 51: 539–553. doi:10.1002/2014WR015814.
- Swedish Meteorological and Hydrological Institute (SMHI). 2015. Large-scale multi-basin modelling and comparative hydrology. http://www.smhi.se/en/research/research-departments/hydrology/hype-model-systems-eng-1.21603 (accessed July, 2015).
- Tan, X., and T. Y. Gan. 2015. Nonstationary analysis of annual maximum streamflow of Canada. Journal of Climate 28: 1788–1805.
- United States Department of Agriculture (USDA). 1986. Urban hydrology for small watersheds. Technical Release 55, Natural Resources Conservation Service, Conservation Engineering Division, USDA. http://www.nrcs.usda.gov/Internet/FSE_DOCUMENTS/stelprdb1044171.pdf
- Watt, W. E. ed. 1989. Hydrology of floods in Canada: A guide to planning and design. Ottawa: Associate Committee on Hydrology, National Research Council Canada..
- Yu, X., T. A. Cohn, and J. R. Stedinger. 2015. Flood frequency analysis in the context of climate change. In World environmental and water resources congress ed. Karen Karvazy and Veronica Webster, 2376–2385. Reston: American Society of Civil Engineers.