Abstract
This paper describes a new regional ice analysis system developed at Environment Canada. It is primarily designed to satisfy the requirements for planning of marine transportation and other marine operations in ice-infested waters around North America, including Canada's two Arctic Metareas; regional sea-ice model initialization; and the needs of regional numerical weather prediction models. A three-dimensional variational approach (3D-Var) is used to assimilate various types of observations. In this first version, only analyses of ice concentration are produced at approximately 5 km resolution using a 6 h persistence forecast from the previous analysis as the background state. The assimilated observations are sea-ice concentrations from two sources of passive microwave satellite data, Advanced Microwave Scanning Radiometer-Earth observing system (AMSR-E) and Special Sensor Microwave/Imager (SSM/I), and manually derived ice charts from the Canadian Ice Service (CIS). Objective verification scores computed from independent data are used to evaluate the accuracy of the analyses in comparison with other available sources of ice information. This comparison demonstrates that the new analyses have consistently more accurate ice extent compared with the currently operational global sea-ice analyses at the Canadian Meteorological Centre. It also shows that the early morning analysis can provide marine transportation clients with a valuable update to the most recently available CIS daily ice chart.
RÉSUMÉ [Traduit par la rédaction] Cet article décrit un nouveau système d'analyse régionale des glaces mis au point à Environnement Canada. Il est principalement conçu pour répondre aux exigences de la planification du transport maritime et autres opérations maritimes dans les eaux infestées de glace entourant l'Amérique du Nord, y compris les deux régions météorologiques arctiques du Canada, pour l'initialisation du modèle régional de glaces de mer et pour les besoins des modèles régionaux de prévision météorologique numérique. Nous utilisons une approche variationnelle tridimensionnelle (3D-VAR) pour assimiler différents types d'observations. Dans cette première version, seules des analyses de la concentration de la glace sont produites avec une résolution d'environ 5 km à l'aide d'une prévision de 6 h basée sur la persistance à partir de l'analyse précédente utilisée comme l'ébauche. Les observations assimilées sont les concentrations de la glace de mer de deux sources satellitaires de données hyperfréquences passives — le radiomètre à balayage hyperfréquences de pointe du Système d'observation de la Terre (AMSR-E) et le capteur hyperfréquences spécialisé/imageur (SSM/I) — et les cartes des glaces élaborées manuellement du Service canadien des glaces (SCG). Nous utilisons des indices de vérification objectifs calculés à partir de données indépendantes pour évaluer l'exactitude des analyses par comparaison avec d'autres sources d'information sur les glaces disponibles. Il ressort que les nouvelles analyses ont constamment des étendues de glaces plus exactes que les analyses globales opérationnelles courantes des glaces de mer employées au Centre météorologique canadien. Cela montre aussi que l'analyse préliminaire du matin peut fournir aux clients du transport maritime une mise à jour valable de la plus récente carte quotidienne des glaces du SCG.
1 Introduction
Sea-ice analyses are prepared for a variety of purposes including marine transportation planning, sea-ice model initialization, specification of the boundary conditions for numerical weather prediction (NWP) models and climate monitoring. In Canada, analyses to assist in the planning of marine transportation and other marine activities are currently produced by a highly manual and time-consuming process involving expert analysis of a wide variety of satellite, aircraft and in situ observations. Such manual analyses are produced operationally in near real-time by the Canadian Ice Service (CIS) and include the ice concentration and thickness distribution (Carrieres, Greenan, Prinsenberg, & Peterson, Citation1996). The application of numerical sea-ice models usually also requires ice concentration and ice thickness distribution to specify the initial conditions and for this reason may be initialized using manual analysis products (e.g., Pellerin et al., Citation2004), though their limited spatial and temporal coverage may represent a challenge. Atmospheric models used for NWP require ice information as a lower boundary condition for computing the radiative, thermal and humidity fluxes. For this purpose, the ice concentration and mean thickness are usually sufficient.
Automated sea-ice analyses have a fairly long history dating back to the launch of the first satellite-borne passive microwave sensors in the 1970s. The main application of these early analyses was climate monitoring. Automated sea-ice analyses for NWP also rely heavily on passive microwave satellite sensors because they provide the required global daily coverage. Because of a lack of sufficient reliable observations, ice thickness for NWP must often be derived from other sources, such as a climatology obtained from observations or model outputs.
Automated sea-ice analyses for marine operational applications, such as shipping and off-shore hydrocarbon extraction, are nearly non-existent. The European Organisation for the Exploitation of Meteorological Satellites (EUMETSAT) Ocean and Sea Ice Satellite Applications Facility (OSI SAF) provides routine sea-ice products including ice extent, concentration and drift, based mainly on passive microwave satellite data (OSI SAF, Citation2011). These products are of interest to the marine community but are too coarse in spatial resolution for most operational applications. Newer products that use visible and infrared observations from weather satellites are starting to approach the resolution required for marine operations although their use is limited by the presence of clouds and (except for those relying exclusively on thermal infrared channels) lack of sunlight.
The new regional ice analysis system described here represents the analysis component of the Canadian Regional Ice Prediction System (RIPS). It is primarily intended to satisfy the requirements for planning of marine transportation and other marine operations in ice-infested waters around North America, including Canada's two Arctic Metareas; regional sea-ice model initialization; and for the requirements of regional NWP models. The strategy for developing this initial version of the system has been, as much as possible, to apply existing techniques and public domain research, including the three-dimensional variational data assimilation approach (3D-Var) upon which the system is based. A global configuration of the same system is also being developed for global NWP and other global applications.
Data assimilation techniques previously used in atmospheric and oceanographic applications have also recently been applied to the objective analysis of sea-ice data. Several aspects of the system described in this paper are similar to the 3D-Var approach evaluated by Caya, Buehner, and Carrieres (Citation2010) in the context of initializing a coupled ice-ocean model for the region off the east coast of Canada. Several earlier studies focused on the assimilation of ice motion estimated from processing sequential satellite images. In the studies of Meier, Maslanik, and Fowler (Citation2000) and Meier and Maslanik (Citation2003) optimal interpolation was used to assimilate ice motion estimated from Special Sensor Microwave/Imager (SSM/I) data to initialize a stand-alone ice model for the Arctic. The assimilation improved the agreement of the modelled ice motion with buoy observations and also substantially altered the average ice thickness in some regions. Similarly, using a coupled ice-ocean model of the Arctic Ocean, Zhang, Thomas, Rothrock, Lindsay, and Yu (Citation2003) assimilated ice motion from buoy motion and SSM/I imagery using optimal interpolation. They showed that the assimilation improved both ice motion and thickness. Rollenhagen, Timmermann, Janjić, Schröter, and Danilov (Citation2009) used an ensemble-based sequential data assimilation method to assimilate ice drift observations. This resulted in realistic corrections made to the ice concentration and thickness fields as a result of the multivariate covariances obtained from using ensemble-based error covariances. Using the simpler approach of nudging and a model similar to Zhang et al.’s (Citation2003), Lindsay and Zhang (Citation2006) assimilated ice concentration observations and, in a separate step, also assimilated ice motion using optimal interpolation while adjusting the ice thickness to minimize changes in ice mass. They found that, relative to observations, the ice extent and the simulated ice draft were both significantly improved by this assimilation procedure. Stark, Ridley, Martin, and Hines (Citation2008) assimilated ice concentration and motion from satellite data in addition to a full set of ocean data using an optimal interpolation scheme and a global coupled ice-ocean model. Although they adjusted ocean salinity in response to changes made to the ice mass, the ocean temperature was not directly modified by the assimilation of ice data. They obtained significant improvements for either ice concentration or ice motion from assimilating observations of either concentration or motion alone. Posey et al. (Citation2011) described the Naval Research Laboratory Arctic Cap Nowcast/Forecast System that uses 3D-Var to assimilate numerous sources of oceanographic observations in addition to ice concentration derived from passive microwave satellite data to initialize a coupled ice-ocean model. However, the ice concentration analysis is only used to initialize the subsequent forecast when the model ice concentration is below 40%, thus allowing the model to evolve unconstrained within the interior of the ice pack. Lisæter, Rosanova, and Evensen (Citation2003) used an ensemble Kalman filter (EnKF) applied to a coupled ice-ocean model of the Arctic Ocean to assimilate ice concentration derived from SSM/I. They demonstrated the complex spatial and multivariate covariances of the short-term forecast (background) error that were estimated from the ensemble. In a subsequent study, Lisæter, Evensen, and Laxon (Citation2007) assimilated synthetic ice thickness data using the EnKF and a coupled ice-ocean model resulting in a significant impact on both sea ice and ocean variables with the ice thickness being improved. Scott, Buehner, Caya, and Carrieres (Citation2012) used a simple radiative transfer model within a 3D-Var assimilation system to assimilate passive microwave brightness temperature observations directly to estimate sea-ice concentration and several other geophysical variables related to the brightness temperature observed by the satellite-borne sensor. They found that direct assimilation of brightness temperatures produces ice concentration analyses that are in slightly better agreement with independent observations than when assimilating an ice concentration retrieval obtained from the same brightness temperature data.
The outline of this paper is as follows. Section 2 provides a description of the overall system configuration and the data assimilation techniques utilized in RIPS. Section 3 describes the observations assimilated in RIPS as well as the quality control applied to these observations. Section 4 provides the results from a detailed objective and subjective evaluation of the system over a one-year period. Section 5 gives some details concerning the implementation of the system at the Canadian Meteorological Centre (CMC), and in Section 6 a summary and some conclusions are given.
2 System description
a General System Configuration
The analysis domain () of RIPS covers all the ice-infested waters surrounding North America, extending from west of Bering Strait to east of Greenland and from south of the Great Lakes to just beyond the North Pole. The projection is a rotated latitude-longitude grid with 0.045° resolution in both directions (approximately 5 km resolution near the numerical equator) and 1640 × 1080 horizontal grid points. One of the poles of the rotated grid is located at geographical longitude 100°W and the numerical equator intersects this longitude at 64°N. The numerical equator is located near the centre of the domain to obtain a horizontal resolution (in terms of physical distance) that is as uniform as possible. Grid points for which the land fraction field (shown in ) is greater than 20% are treated as land and, therefore, not included in the ice analysis.
Fig. 1 The land-sea mask of the Regional Ice Prediction System. Note the colour bar indicates the fraction of land in each analysis grid cell in units of tenths.
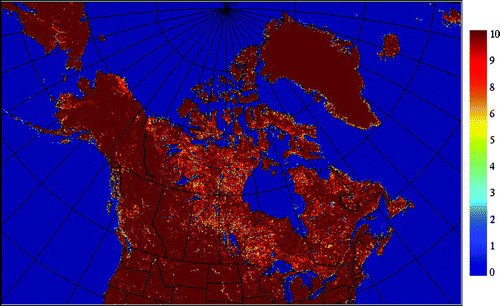
For the current version of RIPS only ice concentration is estimated. No information on the ice thickness or other ice-related variables is considered. The assimilation algorithm is known as 3D-Var with first guess at the appropriate time (FGAT). All observations within the assimilation time window are considered when producing the analysis at the centre of the window. In principle, the background state is interpolated in time to the time of each observation when computing the difference between the observation and background state (also referred to as the innovation). In the current system, however, no sea-ice model is employed, instead the previous analysis is used as the background state and, therefore, the background state does not vary in time within the assimilation window. Four analyses (valid at 0000, 0600, 1200 and 1800 utc) are produced each day. The length of the assimilation window is six hours, centred on the valid time of each analysis.
Following the 3D-Var approach, the gridded sea-ice concentration analysis is obtained by minimizing the following pre-conditioned and incremental form of the cost function:
Note that in Eq. (1) the inverse of is required, but only the square-root of
is needed. The observation errors are assumed uncorrelated, yielding a diagonal matrix
which is trivial to invert. This assumption is also made for many implementations of the variational data assimilation approach for NWP (e.g., Gauthier, Charette, Fillion, Koclas, & Laroche, Citation1999). Though computationally convenient, this may not be a valid assumption for the observations assimilated here because retrievals of ice concentration from both satellite data and manual ice analyses likely have errors with complex spatial correlations caused by the processes and approximations used to compute the ice concentration values. Further study is required to estimate these correlations and to evaluate the impact of neglecting them. More detail regarding the background-error covariances is provided in the next section.
b Background-Error Covariances
The background-error covariances are an essential aspect of any data assimilation system. They partially govern the level of influence the observations have on the analysis relative to the background state and how the information is spread both spatially and to other analysis variables that may not be directly related to the observations (though in the current version of RIPS there is only one analysis variable). A simple parameterized form of the background-error covariance matrix for ice concentration is used with covariance parameters assumed to be stationary in time and horizontally homogeneous. The horizontal background-error correlations are incorporated in the system using a diffusion operator (Weaver & Courtier, Citation2001). The background-error standard deviation for the total ice concentration is set to 0.093 and the background-error horizontal correlation length scale is set to 10 km. Several aspects of the implementation of the background-error covariances and the overall 3D-Var system are described in further detail in the study by Caya et al. (Citation2010), including the calculation of the error standard deviation that was obtained using an EnKF. Because this earlier study used a coupled ice-ocean model and a different spatial domain, the value of the standard deviation is likely not optimal for RIPS.
c Adjustments to the Analysis
It was found necessary to apply additional adjustments to the sea-ice concentration analysis to eliminate artifacts introduced by the assimilation procedure. The assumption that errors are Gaussian (normally distributed) and the use of a simple parameterized form for the background-error covariances can lead to unrealistic values for the ice concentration analysis, especially near the ice edge. Consequently, all ice concentration values above one are set to one and all negative values are set to zero. Another procedure is aimed at removing spurious sea ice over warm water where the likelihood of ice being present is negligible. The presence of ice in such areas of the analysis is most often a result of spurious non-zero ice concentration retrievals obtained from passive microwave data (discussed in the next section). To reduce this problem, the sea-ice concentration analysis is set to zero at all grid points where the sea surface temperature (SST) is greater than 4°C. The SST is obtained from the operational SST analysis system at CMC (Brasnett, Citation1997).
3 Sea-ice observational data
The observations assimilated are total ice concentration values derived from a variety of sources. In this section each of these is described. The observation-error standard deviations for each were obtained empirically by performing a limited number of data assimilation experiments with different standard deviation values and also by using, as a guide, a comparison of the predicted innovation variance (i.e., the diagonal elements of H
H
T +
) with the computed innovation variance (i.e., the variance of y − H[x
b
]) for areas near the ice edge.
a Passive Microwave Data: AMSR-E and SSM/I
Observations from two passive microwave sensors are assimilated: the Advanced Microwave Scanning Radiometer-Earth observing system (AMSR-E) aboard the Aqua satellite and SSM/I onboard the Defence Meteorological Satellite Program satellite F15. The retrieved ice concentration is calculated from the observed brightness temperatures using the National Aeronautics and Space Administration (NASA) Team 2 (NT2) sea-ice algorithm (Markus & Cavalieri, Citation2000). In the current version of RIPS, NT2 ice concentration retrievals over fresh water are not assimilated.
The footprint size of the passive microwave channels used in the NT2 algorithm is significantly larger than the grid spacing of the analysis. To avoid contaminating small-scale details that are not represented in these ice concentration retrievals, a “footprint operator” is used as part of the observation operator, H. This footprint operator averages the gridded ice concentration (from either the background state or the analysis increment) over all grid points within a distance from the observation location equal to the footprint radius of the sensor, thus simulating the spatial averaging effect of the actual satellite measurement. If any of the grid points included in the footprint average has a non-zero land fraction, then the observation is not assimilated to avoid the use of any observations contaminated by land. The observation-error standard deviation and footprint radius used for each type of passive microwave observation are shown in .
Table 1. Parameters used for the assimilation of each type of observation. The background-error standard deviation in observation space (square-root of the diagonal elements of HBH T) is estimated with a randomization technique using 30 samples. HBH T depends on the background-error horizontal correlation length scale, the background-error standard deviation and the observation footprint size.
b CIS Daily Ice Charts and CIS RADARSAT Image Analyses
Daily ice charts are prepared manually in near real-time by CIS, as described by Carrieres et al. (Citation1996) and are available in graphical form on the CIS website (http://ice-glaces.ec.gc.ca). These charts are subjective analyses of the instantaneous ice concentration and its stage of development. The daily ice charts may be considered nowcasts because ice information from observations is projected forward in time to 1800 utc each day. Several types of remotely sensed data, ship and aircraft reports, and the analysis from the previous day all feed into the process.
Ice concentration from subjective analyses by CIS of RADARSAT synthetic aperture radar (SAR) images (which are an important input to the production of the daily ice charts) is also assimilated. Though these data only cover a small portion of the domain for any given time, the accuracy is expected to be relatively uniform over the image analysis. The CIS RADARSAT image analysis is valid for the actual time of the satellite image on which it is based and, therefore, may be assimilated in an analysis window other than the one centred on 1800 utc.
Because the CIS RADARSAT image analyses are also used in the production of the daily ice charts, this is effectively equivalent to assimilating the same information twice. However, because the daily ice charts are assimilated assuming an equal level of accuracy throughout, regardless of the source of observations used in their production, the result of also assimilating the image analyses is that more weight is given to the observations in areas where they have a higher level of accuracy, that is, in the regions and at times when the image analyses are available. The daily ice charts and RADARSAT image analyses are assimilated using the same value for the observation-error variance (see ). Both types of manual analyses are assimilated after being converted into gridded form at a resolution close to that of the RIPS analysis grid; therefore, a simple bilinear horizontal interpolation is used in the observation operator for this data source.
c CIS Lake Analyses
On a weekly basis, CIS also produces analyses of ice concentration over lakes. There are a total of 139 lake locations (some of which belong to the same large lake) included in this analysis product. Many of these lakes are too small to be represented on the RIPS analysis grid such that only 88 of the reported lake ice concentrations are assimilated (see ). The ice concentration reported is an average over the entire lake (or half of the lake where there are two locations for the same lake). Consequently, the observation operator for this data type is an average over all water points contiguous with the location of the reported ice concentration.
Fig. 2 Locations of lakes included in the CIS lake analysis. Of the 139 locations, 88 are assimilated (red dots) and 51 are rejected (blue dots). The rejected lakes cannot be assimilated because they are not resolved on the RIPS analysis grid. Note that these do not include the Great Lakes.
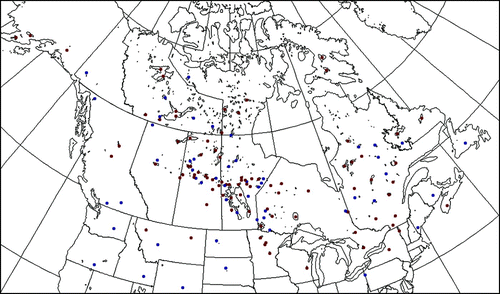
As can be seen in , the background-error standard deviation projected into observation space (H
H
T) depends on the size of the lake. The explanation for this is that for large lakes the observation operator averages the background error (along with the ice concentration itself) over many grid points, resulting in a lower background error standard deviation for this average lake ice concentration because of a partial cancelling of errors at different locations. The observation-error standard deviation is set to 0.01 for all lakes. The reason for specifying such a small value, which results in a relatively large influence on the analysis from these observations, is that it is the only observation type assimilated over these lakes, and it is only available once per week. The chosen value for the observation-error standard deviation results in a moderately close agreement between the RIPS analysis and the observations for large lakes (though, as will be shown later, it may not be sufficiently close). The observations for small lakes will have a larger influence on the analysis because the corresponding background-error standard deviation projected into observation space will be larger.
d Quality Control of Observations
The quality control of the observations is an important aspect of any data assimilation system. Without it, the quality of the analysis can be seriously degraded by assimilating relatively few “bad” observations. There are two levels of quality control employed in the present system. The first is a type of “background-check” to eliminate entire swaths of remotely sensed data that are unusable because of a sensor failure on the satellite, for example, or to eliminate an entire CIS daily ice chart or RADARSAT image analysis because of a problem (which has occasionally occurred) in the conversion from shapefiles to gridded data. The second level of quality control is only applied to retrievals from passive microwave satellite data and is necessary to reject incorrect ice concentration retrievals from the NT2 algorithm. Known problems with the NT2 algorithm include weather contamination, for example mistaking the wind-roughened sea surface for ice, and bias during the season when melt-ponds form on the surface of the ice and cannot be distinguished from open water.
1 Background-check
The background-check is a procedure in which the observations are compared with the background state in observation space and the difference between the two (i.e., the innovation) is used to identify major problems with the data. In the present context, the root-mean-square (rms) of the innovations over an entire CIS daily ice chart, CIS RADARSAT image analysis or swath of satellite data is calculated and the entire ice chart, image analysis or swath is rejected if the rms is above a specified threshold, given in . For the retrievals from passive microwave data, the calculation of the rms considers only the observations that passed all other quality control criteria. The thresholds were determined from an assimilation run for all of 2007 but also from other periods (February 2010 and August–September 2009) with known erroneous data. The thresholds used allow the rejection of all bad daily ice charts, RADARSAT image analyses and swaths during these test periods, while preserving all of the correct data with only a few exceptions. The number of rejected good data is much less than the number of rejected bad data. Five to ten SSM/I swaths were rejected during July 2007 not because brightness temperatures were bad but because the ice concentration retrievals were much smaller than the background values, possibly an indication that melt-water on sea ice was mistaken for open water. The result of discarding these SSM/I swaths actually led to an improved analysis. The spatially averaged bias of the innovation was also examined as a potential measure on which to base the rejection criteria, but it was found to be less efficient than the rms at identifying the bad data swaths.
Table 2. Thresholds applied to the rms innovation for each assimilated observation type. The entire ice chart or satellite data swath is rejected when its rms innovation is above the specified threshold.
2 Quality control applied to ice concentration retrievals from passive microwave data
To address the problem of mistaking melt-water on top of sea ice for open water, data are not assimilated where the 2 m surface air temperature, obtained from the CMC operational surface analysis system, is greater than 0°C. The surface air temperature analysis is based on a two-dimensional (2D) optimal interpolation that uses the 6-hour model forecast (from the NWP system) as the background state and assimilates in situ observations of 2 m temperature over land and from ice/ocean drifters. Higher temperature thresholds of 2°C and 4°C were also tested, but using 0°C gave the best results when verified using independent data.
To address the problem of erroneous ice concentration retrievals as a result of weather contamination, for example mistaking the wind-roughened sea surface for ice or erroneous sea ice retrieved in areas of intense precipitation, all non-zero ice concentration retrievals are rejected where the SST is greater than 4°C.
There are cases where the quality control described in the previous paragraph cannot detect spurious ice concentration retrievals. The climatological frequency of occurrence (FOO) of sea ice was introduced to remove non-zero ice concentration observations wherever the FOO is zero. The FOO is calculated using a composite of the products from the US National Ice Center (NIC) and CIS. The NIC 1972–2007 Arctic climatology product (National Ice Center, Citation2006) is available on a 25 km resolution grid and covers the entire RIPS domain on a monthly basis. However, because RIPS is at 5 km resolution, the difference in resolution creates the problem of missing values near coastlines. In order to correct this, the solution of Laplace's equation is obtained at all non-water grid points subject to the boundary conditions imposed by the surrounding points using the sequential relaxation method or Liebman relaxation (Thompson, Citation1961). As a result, values are obtained at all grid points within the RIPS domain. The 1971–2000 CIS weekly climatology product (CIS, Citation2011), with 1 km resolution and availability throughout the year depending on the region, is used to replace the FOO from the NIC climatology where and when it is available. The resulting fields of the climatological FOO are generated for each week of the year on the RIPS analysis grid. An example of the computed FOO is shown in . Use of quality control based on the FOO has shown satisfactory results in specific problematic cases while not affecting overall verification scores.
4 Verifications
The ice concentration analyses produced by RIPS were verified using several types of independent verification data. The entire year of 2007 was chosen for validation to capture the growth and melt seasons for all regions.
a Objective Evaluation of RIPS Relative to the CMC Operational Global Ice Analysis System
The verification scores for RIPS were compared with the same scores computed from the current operational global ice analysis system run at CMC for the needs of the operational weather prediction systems. The CMC operational analysis is produced once per day by a simple averaging of the CIS daily ice chart and ice concentrations obtained from SSM/I data using the AES-York retrieval algorithm (Ramseier & Rubinstein, Citation1992). The analysis is produced on a global latitude-longitude grid with approximately 35 km grid spacing at the equator. Because the CMC operational analysis is deemed valid at 0000 utc, only the 0000 utc analyses from RIPS were used in these evaluations.
1 Verification data
Because there is no one ideal verification dataset of ice concentration, more than one dataset was used to evaluate both RIPS and the CMC operational ice analysis: CIS RADARSAT image analyses and the Interactive Multisensor Snow and Ice Mapping System (IMS) ice extent product.
The CIS RADARSAT image analyses contain ice concentration information valid at the time of the satellite data on which they are based. These image analyses are prepared manually by CIS SAR image analysts who have received extensive training to be able to interpret SAR imagery accurately while also using information on local environmental conditions, in situ observations from ships, and climatology. Recent image analyses in graphical form and an Ice Products Guide are available on the CIS website (http://ice-glaces.ec.gc.ca). The quality of the CIS RADARSAT image analyses is maintained and improved through routine feedback from ship-based users who rely on them to aid navigation. However, because these image analyses are intended for such CIS clients and are relatively labour-intensive to produce, they cover only a relatively small region that varies according to the season. This can cause the resulting verification scores to appear discontinuous for different periods of the year when the areal coverage of the image analyses changes abruptly. Another limitation is that the ice concentrations are only reported to the nearest tenth and assumed to be homogeneous over a limited number of polygons for each image analyzed. The RADARSAT image analyses used in the verification are valid between 0900 utc and 1500 utc following the 0000 utc valid time of both the RIPS analysis and the CMC operational analysis. This period of time has a high volume of image analyses and, because these image analyses are valid after the assimilation window, they are mostly independent from the data included in both the RIPS analysis and the CMC operational analysis (assuming the errors in image analyses from different days are uncorrelated, which may not always be true because of a misinterpretation of persistent changes in ice signatures, for example, from surface melting). The ice concentration from the two analysis systems is interpolated onto the RADARSAT image analysis grid, and the bias and standard deviation are calculated.
The IMS daily product is generated manually by meteorologists at the NIC showing ice extent (i.e., ice or open water) on a 4 km grid (Helfrich, McNamara, Ramsay, Baldwin, & Kasheta, Citation2007; Ramsay, Citation1998). It is generated by using information from a wide variety of satellite imagery, mapped products and surface observations. The IMS product covers the entire RIPS domain and is independent because it is not used in RIPS or the CMC operational system. To compare the ice concentration from the two analysis systems with the IMS ice extent product, the ice concentrations are first interpolated onto the 4 km grid used by IMS and then converted into a binary ice/open-water value using a threshold of 40% ice concentration. The following verification scores for the ice extent are then computed (for all of these, a perfect score is a value of one):
• | The frequency bias is computed as the number of ice grid points in the analysis divided by the number of ice grid points in the IMS product. | ||||
• | The total proportion correct is the number of grid points in the domain for which the analysis and the IMS product agree divided by the total number of grid points in the domain. | ||||
• | The proportion correct for ice is similar to the total proportion correct, except that only grid points identified as ice in the IMS product are included. | ||||
• | The proportion correct for water is similar to the total proportion correct, except that only grid points identified as open water in the IMS product are included. |
2 Results and analysis
The biases for both the RIPS analysis and the CMC operational analysis verified using the CIS RADARSAT image analyses are shown in . These results have been computed using all available image analyses for each week of the year. It should be noted that, because of the seasonal variation of the region where RADARSAT image analyses (and also CIS daily ice charts) are produced, the areas being verified will most often correspond to areas where CIS daily ice charts have recently been available and used in both analysis systems, which may explain the strong correlation in the temporal variation of the biases between the two systems. The overall bias for RIPS over the entire year is −0.019, compared with 0.020 for the CMC ice analysis. These results show biases with a similar small magnitude but with opposite sign. The CMC analysis bias is slightly positive, indicating the presence of more ice than is seen in the CIS RADARSAT image analyses. An important contribution to the difference in bias is that the “Open Water” and “Bergy Water” codes from the CIS daily ice charts and CIS RADARSAT image analyses are decoded as 5% ice concentration when ingested in the CMC operational analysis, but they are interpreted as 0% ice concentration in RIPS and in the verification system. The slightly negative bias for RIPS may be caused by the assimilation of ice concentration retrievals from AMSR-E data, which may underestimate ice concentration, as suggested by Meier and Notz (Citation2010). It is interesting to note that the bias for both analyses is higher during the melting season than during the ice growth season, possibly because of the use of verification data with a valid time later than that of the analyses. The seasonal variation in the bias is difficult to interpret because the regions included in the verification (that is, the regions where CIS RADARSAT image analyses are produced) during different parts of the year vary significantly.
Fig. 5 The ice concentration bias of the RIPS analysis (red) and the CMC operational global ice analysis (blue) verified using the CIS manual analyses of RADARSAT images. The solid curves are the results for all image analyses available each week, and the dashed lines are the temporally averaged biases for the entire year.
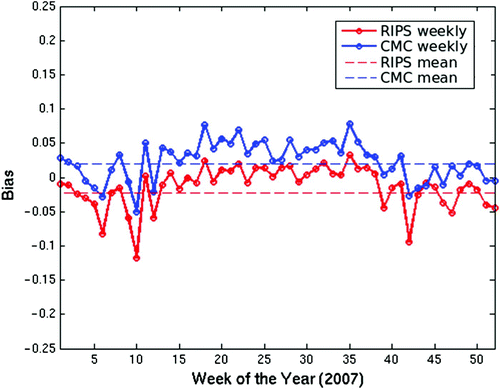
The standard deviations of the two ice analyses relative to CIS RADARSAT image analyses are shown in . The CMC analysis has a slightly better result, with a standard deviation of 0.19, compared with 0.20 for the RIPS analysis. This may be related to the difference in spatial resolution between the two analysis systems. The higher resolution of RIPS analyses and the resulting sharper ice edge may result in locally larger amplitude differences when compared with the CIS RADARSAT image analyses valid 9–15 hours after the valid time of the analysis.
Fig. 6 Similar to but showing the ice concentration error standard deviation of the RIPS analysis (red) and the CMC operational global ice analysis (blue) verified using the CIS manual analyses of RADARSAT images.
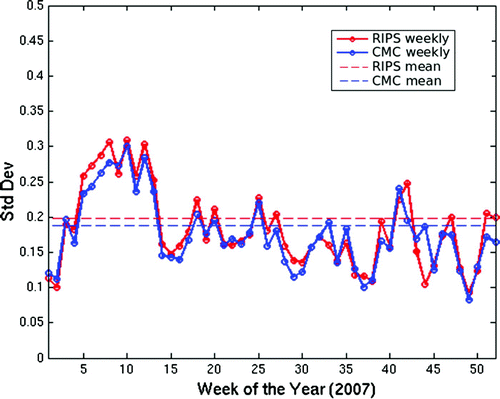
, and 9 show the verification scores over the entire domain for the RIPS analysis and CMC operational analysis obtained using the IMS ice extent product. shows the frequency bias for the two systems when averaged over all of 2007. In general, the frequency bias is close to one in areas where sea ice occurs, indicating that ice is present with approximately equal frequency in the analyses compared with the IMS product. However, in several isolated areas (e.g., Davis Strait, Baffin Bay and the Chukchi Sea) the frequency bias is much higher for the CMC operational analyses (right panel) than for the RIPS analyses (left panel). shows the total proportion correct for the two systems, averaged over the entire year. These results indicate that RIPS produces improved sea-ice concentrations relative to the CMC operational ice analysis system, most notably within the Canadian Arctic Archipelago and in areas near land throughout most of the domain. However, the larger Canadian lakes (not including the Great Lakes) clearly have a lower total proportion correct in the RIPS analyses than in the CMC operational analyses, suggesting that the CIS lake analysis product may not have sufficient influence on the RIPS analysis. shows time series of several verification scores averaged over the entire domain and filtered with a 30-day running mean. The total proportion correct (upper left panel) shows an overall advantage of the RIPS analysis over the CMC operational analysis for the entire year. The proportion correct for ice (upper middle panel) shows an apparent advantage for the CMC operational analysis, which is to be expected because of its larger positive frequency bias relative to RIPS (lower left panel). This can be understood by considering an analysis that always has ice over its entire domain because this would result in a perfect score for the proportion correct for ice. It should be noted that the ice extent from the RIPS and CMC operational analyses will agree with each other and also agree with a climatological mean ice extent for the same time of year over much of the domain. Consequently, differences in the verification scores only originate from a relatively limited fraction of the domain at any given time and should therefore not be expected to be large.
Fig. 7 The ice extent frequency bias for all of 2007 for the RIPS analysis (left panel) and the CMC operational ice analysis (right panel) verified using the IMS ice extent product. Frequency bias is measured as the total number of locations with ice in the analysis divided by the total number of locations with ice in the IMS product.
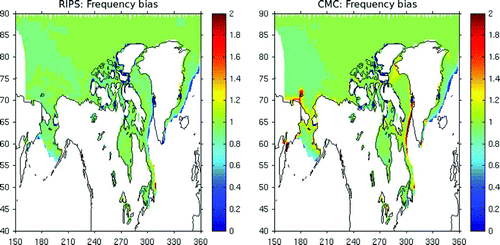
Fig. 8 The total proportion correct for the RIPS analysis (left panel) and the CMC operational ice analysis (right panel) verified using the IMS ice extent product. This quantity is defined as the total number of locations for which the analysis and the IMS product are consistent, divided by the total number of locations.
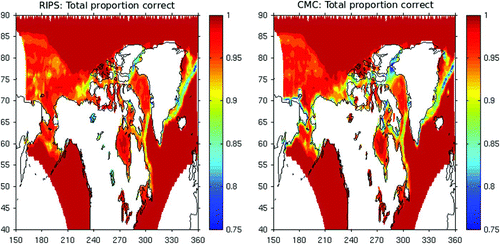
b Objective Evaluation of RIPS Relative to CIS Daily Ice Charts
RIPS was also evaluated in comparison with all available CIS daily ice charts. The early morning (in Canada this is around 1200 utc) is a time when CIS clients require up-to-date ice information to help plan marine operations for the day. However, because the CIS daily ice chart is only produced around 1800 utc, these clients must currently rely on the chart from the previous day, valid approximately 18 hours earlier. The purpose of this comparison is to evaluate the potential use of the 0600 utc RIPS analysis as an alternative to the daily ice chart from the previous day. Consequently, the verification is performed for the 1800 utc daily ice chart and the 0600 utc RIPS analysis (valid 12 hours later) both verified using independent data, preferably close to 1200 utc.
1 Verification data
In this verification, the only dataset used to evaluate the RIPS analyses and CIS daily ice charts was the CIS RADARSAT image analyses. As in the verification relative to the CMC operational analysis, the CIS RADARSAT image analyses chosen fall between 0900 utc and 1500 utc following the valid time of the RIPS analysis and the CIS daily ice chart. These data are thus valid starting 15 hours after the CIS daily ice chart, and 3 hours after the 0600 utc RIPS analysis. The CIS RADARSAT image analyses used for verification are not used in the creation of either product for the specific date being verified; therefore, they are mostly independent of the analyses being verified, except in cases where errors in observations at different times are correlated.
2 Results and analysis
The comparison of the 0600 utc RIPS analysis with the CIS daily chart is shown in and . The overall bias (shown in ) for the RIPS analysis is −0.016, and for the CIS daily chart it is −0.002. The overall standard deviation (shown in ) is 0.18 for the RIPS analysis and 0.20 for the daily chart. This improvement in the RIPS analysis is seen to result from a consistent reduction in standard deviation during all times of the year. This indicates that at the time the 0600 utc RIPS analysis is available to CIS clients it provides valuable additional information compared with the most recent CIS daily ice chart.
c Subjective Evaluation of the Davis Strait Ice Edge
Two sea-ice forecasters at CIS, Norm Hart and Langis de Ladurantaye, performed subjective evaluations of the RIPS analyses in the Davis Strait region. The intent was to determine the accuracy of the sea-ice analysis and its suitability in producing an automated Davis Strait ice edge product. To evaluate the system, the forecasters compared the 1200 utc RIPS analyses to the weekly or biweekly CIS regional ice charts for 2007. This chart covers the entire area being evaluated and can also be considered relatively independent from the RIPS analysis because it is produced by CIS analysts several days after its valid time and, therefore, incorporates a wider range of data sources than can be used for the CIS daily ice charts.
In these comparisons, a difference of 10 nautical miles (nm) or less between the ice edge in the RIPS analysis and the CIS regional ice chart is considered to be a very good correspondence. Because the evaluation is in the context of automating an ice edge product currently produced by ice forecasters, an acceptable error of the RIPS analysis is the difference between the ice edges produced by two forecasters using the same available observations for the Davis Strait region. The typical distance between these ice edges is believed to be about 20 nm, based on the past experience of ice forecasters at CIS. This relatively large discrepancy in ice edge location is a result of the nature of the product, which is essentially a nowcast valid at 1800 utc and covering a pre-defined region. Consequently, high-resolution satellite observations close to the valid time of 1800 utc are not generally available for the entire region covered by the product, resulting in larger errors.
During the winter season, the ice cover in Davis Strait in the RIPS analysis generally corresponded very well with the CIS regional ice chart. In the cases where the agreement between the RIPS analysis and the regional ice chart was not as good, there was often less ice shown in the RIPS analysis. This is likely because of the tendency for passive microwave data to underestimate ice cover (Meier & Notz, Citation2010), resulting in an ice edge too far to the west. Another noticeable discrepancy is that the analysis becomes less reliable north of 67°N, where differences in edge positions sometimes exceeded the 20 nm threshold. However, in the area of Disko Bay (69°N) the availability of data used by the analysts tends to be limited and relying on the RIPS analysis may be comparable to an analyst's capabilities. During the late summer and fall, there is little ice in the area of interest. The ice that is present is captured very well by the RIPS analysis, often including small coastal or open ocean patches. In the arguably more sensitive area east of Hudson Strait, there are days when the ice edge in the RIPS analysis is in error by more than 20 nm in any direction, clearly misrepresenting transient features in the ice edge, with the larger discrepancies typically involving lower concentrations.
Overall, the subjective evaluation found the RIPS analyses to have very good potential as a tool for operational ice forecasters.
5 Implementation
Based primarily on the results shown in this paper that were obtained from the historical 12-month period of 2007, it was decided to implement RIPS in experimental mode at CMC. Consequently, it has been running in real time since March 2011. Because one expected use of RIPS is to provide CIS clients with an early morning update from the previous 1800 utc CIS daily ice chart, a timely analysis is necessary. Thus, at the time of implementation, an additional daily run of RIPS was created to generate an early version of the 0600 utc analysis. However, it is the later 0600 utc analysis, which assimilates a more complete set of observations, that is used as the background field for the following 1200 utc analysis. The difference in the quality of the early and late analyses has not been evaluated, and it should be noted that the analyses used to produce the verification scores in Section 4 are equivalent to the late analyses.
Graphical products for various sub-domains are produced in both Portable Network Graphics (png) and geotiff formats and are made available to internal users. shows an example of the ice concentration graphical product for the entire RIPS domain. To avoid including areas that are less accurate because of a lack of recently assimilated observations, no concentration is shown for grid points that have not been directly influenced by an assimilated observation within the previous eight days. These areas are shown as white in the graphical products. It is important to note that when the ice concentration is set to zero at a grid point because the SST is above 4°C, that grid point is treated as if it has been influenced by an observation. Some of the areas shown as white are close to land where CIS daily ice charts and CIS RADARSAT image analyses were not available and, to avoid land contamination, passive microwave observations cannot be used. Other areas without recent observations, such as south of Alaska in this example, may not have been influenced by observations because the air temperature is above zero and, therefore, passive microwave observations would have been rejected by the quality control procedure, but the SST is below 4°C. shows a more detailed view of the same information in but for the Davis Strait sub-region.
Fig. 12 The ice concentration graphical product from RIPS for the entire analysis domain valid for 19 April 2012 at 0600 utc. Areas where no observations were assimilated for a period greater than eight days are shown in white.
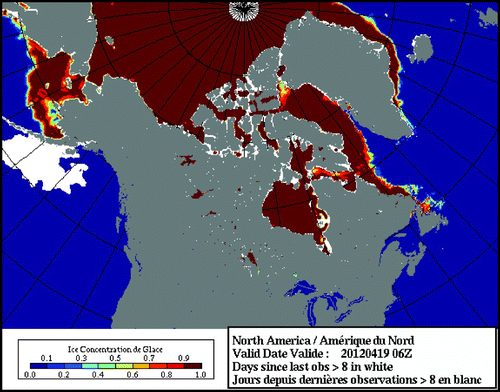
Since the time RIPS was implemented in March 2011, several changes have been made. A new SST analysis system has been implemented at CMC (Brasnett, Citation2008), and the resulting improved SST analyses are now used by RIPS for the quality control procedures and adjustments to the 3D-Var analyses described earlier. Also, the AMSR-E sensor stopped functioning on 4 October 2011; therefore; since that date RIPS only assimilates data from SSM/I, CIS daily ice charts and CIS RADARSAT image analyses. This has certainly resulted in decreased accuracy of the RIPS analyses which will be addressed in the near future by introducing additional sources of assimilated satellite data: first from the Advanced Scatterometer (ASCAT), the Special Sensor Microwave Imager/Sounder (SSMIS) and the Advanced Microwave Scanning Radiometer 2 (AMSR2) and later from visible-infrared and SAR sensors.
6 Conclusions
A new automated sea-ice analysis was developed for North American waters. The system is based on a 3D-Var approach and assimilates CIS daily ice charts, CIS RADARSAT image analyses, CIS lake analyses, and ice concentration retrievals from both AMSR-E (until 4 October 2011) and SSM/I passive microwave satellite imagery using the NT2 algorithm. Ice concentration analyses are produced on a 5 km rotated latitude-longitude grid every six hours. These analyses were objectively verified using independent data and subjectively verified by CIS ice forecasters. The objective verification demonstrated that the analyses are of consistently superior accuracy to the operational CMC global ice analyses in terms of the ice extent. It has also been demonstrated that the early morning (0600 utc) analyses can provide a useful update to the CIS daily ice charts (1800 utc) for CIS clients, including the shipping community. Subjective verifications of the system in the context of defining the Davis Strait ice edge location indicated that the system provides estimates that are of acceptable quality during the periods of the year with significant shipping activity.
While the initial version of the analysis component of RIPS described here has already been shown to provide useful information, several improvements are planned for the near future. These include the assimilation of additional observations (from ASCAT, SSMIS and AMSR2) and the use of a numerical sea-ice model to both provide the background estimate in the assimilation cycle and to produce short-term forecasts (up to approximately 48 hours). Further planned improvements that are the focus of current research are the direct assimilation of higher resolution satellite data, such as visual-infrared and SAR imagery, and the use of improved data assimilation techniques, such as ensemble-based methods.
Acknowledgements
The authors thank Jean-François Lemieux and two anonymous reviewers for helpful comments made on an earlier version of the manuscript. Funding for this work was provided from the following sources: the Natural Resources Canada Program on Energy Research and Development through the Operational Ice Modelling and the Canadian Arctic Archipelago Ice Model projects; the Canadian Space Agency Government Related Initiatives Program (GRIP) SAR and AVHRR Data Assimilation project; the International Polar Year Variability and Change in the Canadian Cryosphere – A Canadian Contribution to “State and Fate of the Cryosphere” project; and, the Government of Canada Metareas project.
References
- Brasnett , B. 1997 . A global analysis of sea surface temperature for numerical weather prediction . Journal of Atmospheric and Oceanic Technology , 14 : 925 – 937 . (doi:10.1175/1520-0426(1997)014<0925:AGAOSS>2.0.CO;2)
- Brasnett , B. 2008 . The impact of satellite retrievals in a global sea-surface-temperature analysis . Quarterly Journal of the Royal Meteorological Society , 134 : 1745 – 1760 . (doi:10.1002/qj.319)
- Carrieres , T. , Greenan , B. , Prinsenberg , S. and Peterson , I. K. 1996 . Comparison of Canadian ice charts with surface observations off Newfoundland, winter 1992 . Atmosphere-Ocean , 34 : 207 – 236 . (doi:10.1080/07055900.1996.9649563)
- Caya , A. , Buehner , M. and Carrieres , T. 2010 . Analysis and forecasting of sea ice conditions with three-dimensional variational data assimilation and a coupled ice-ocean model . Journal of Atmospheric and Oceanic Technology , 27 : 353 – 369 . (doi:10.1175/2009JTECHO701.1)
- CIS (Canadian Ice Service). (2011). 30-year Climatic Ice Atlases. Retrieved from http://www.ec.gc.ca/glaces-ice/default.asp?lang=2n&n=090AF7D6.1
- Gauthier , P. , Charette , C. , Fillion , L. , Koclas , P. and Laroche , S. 1999 . Implementation of a 3D variational data assimilation system at the Canadian Meteorological Centre. Part I: The global analysis . Atmosphere-Ocean , 37 : 103 – 156 . (doi:10.1080/07055900.1999.9649623)
- Gilbert , J.-C. and Lemaréchal , C. 1989 . Some numerical experiments with variable-storage quasi-Newton algorithms . Mathematical Programming , 45 : 407 – 435 . (doi:10.1007/BF01589113)
- Helfrich , S. R. , McNamara , D. , Ramsay , B. H. , Baldwin , T. and Kasheta , T. 2007 . Enhancements to, and forthcoming developments in the Interactive Multisensor Snow and Ice Mapping System (IMS) . Hydrological Processes , 21 : 1576 – 1586 . (doi:10.1002/hyp.6720)
- Lindsay , R. W. and Zhang , J. 2006 . Assimilation of ice concentration in an ice-ocean model . Journal of Atmospheric and Oceanic Technology , 23 : 742 – 749 . (doi:10.1175/JTECH1871.1)
- Lisæter , K. A. , Evensen , G. and Laxon , S. 2007 . Assimilating synthetic CryoSat sea ice thickness in a coupled ice-ocean model . Journal of Geophysical Research , 112 : C07023 doi:10.1029/2006JC003786
- Lisæter , K. A. , Rosanova , J. and Evensen , G. 2003 . Assimilation of ice concentration in a coupled ice-ocean model using the ensemble Kalman filter . Ocean Dynamics , 53 : 368 – 388 . (doi:10.1007/s10236-003-0049-4)
- Markus , T. and Cavalieri , D. J. 2000 . An enhancement of the NASA team sea ice algorithm . IEEE Transactions on Geoscience and Remote Sensing , 38 : 1387 – 1398 . (doi:10.1109/36.843033)
- Meier, W., & Notz, D. (2010). A note on the accuracy and reliability of satellite-derived passive microwave estimates of sea-ice extent, CilC Arctic Sea Ice Working Group Consensus Document. Retrieved from http://www.wmo.int/pages/prog/www/OSY/Meetings/GCW-IM1/GCW_CliC_Sea_ice_Reliability.pdf
- Meier , W. N. and Maslanik , J. A. 2003 . Effect of environmental conditions on observed, modeled, and assimilated sea ice motion error . Journal of Geophysical Research , 108 ( C5 ) : 3152 doi:10.1029/2002JC001333
- Meier , W. N. , Maslanik , J. A. and Fowler , C. W. 2000 . Error analysis and assimilation of remotely sensed ice motion within an Arctic sea ice model . Journal of Geophysical Research , 105 ( C2 ) : 3339 – 3356 . (doi:10.1029/1999JC900268)
- National Ice Center. (2006, updated 2009). National Ice Center Arctic sea ice charts and climatologies in gridded format. Edited and compiled by F. Fetterer & C. Fowler. Boulder, CO, USA: National Snow and Ice Data Center. Digital media. Retrieved January 27, 2009.
- OSI SAF (Ocean & Sea Ice Satellite Application Facility). (2011). Sea ice product manual version 3.7, SAF/OSI/Met.no/TEC/MA/125. Retrieved from http://www.osi-saf.org
- Pellerin , P. , Ritchie , H. , Saucier , F. J. , Roy , F. , Desjardins , S. , Valin , M. and Lee , V. 2004 . Impact of a two-way coupling between an atmospheric and an ocean-ice model over the Gulf of St. Lawrence . Monthly Weather Review , 132 : 1379 – 1398 . (doi:10.1175/1520-0493(2004)132<1379:IOATCB>2.0.CO;2)
- Posey, P. G., Hebert, D. A., Metzger, E. J., Wallcraft, A. J., Cummings, J. A., Preller, R. H., Smedstad, O. M., & Phelps, M. W. (2011). Real-time data assimilation of satellite derived ice concentration into the Arctic Cap Nowcast/Forecast System (ACNFS). Proceedings of Oceans 2011, 19–22 Sept. 2011, Waikoloa, Hawaii. pp. 1–4.
- Ramsay , B. H. 1998 . The interactive multisensory snow and ice mapping system . Hydrological Processes , 12 : 1537 – 1546 . (doi:10.1002/(SICI)1099-1085(199808/09)12:10/11<1537::AID-HYP679>3.0.CO;2-A)
- Ramseier, R. O., & Rubinstein, I. G. (1992). Microwave remote sensing of sea ice. In Geophysical Monograph, 68, Chapter 8, Washington, DC: American Geophysical Union.
- Rollenhagen , K. , Timmermann , R. , Janjić , T. , Schröter , J. and Danilov , S. 2009 . Assimilation of sea ice motion in a finite-element sea ice model . Journal of Geophysical Research , 114 : C05007 doi:10.1029/2008JC005067
- Scott , K. A. , Buehner , M. , Caya , A. and Carrieres , T. 2012 . Direct assimilation of AMSR-E brightness temperatures for estimating sea-ice concentration . Monthly Weather Review , 140 ( 3 ) : 997 – 1013 . (doi:10.1175/MWR-D-11-00014.1)
- Stark , J. D. , Ridley , J. , Martin , M. and Hines , A. 2008 . Sea ice concentration and motion assimilation in a sea ice-ocean model . Journal of Geophysical Research , 113 : C05S91 doi:10.1029/2007JC004224
- Thompson , P. D. 1961 . Numerical weather analysis and prediction , New York : The MacMillan Co .
- Weaver , A. T. and Courtier , P. 2001 . Correlation modeling on the sphere using a generalized diffusion equation . Quarterly Journal of the Royal Meteorological Society , 127 : 1815 – 1846 . (doi:10.1002/qj.49712757518)
- Zhang , J. , Thomas , D. R. , Rothrock , D. A. , Lindsay , R. W. and Yu , Y. 2003 . Assimilation of ice motion observations and comparisons with submarine ice thickness data . Journal of Geophysical Research , 108 ( C6 ) : 3170 doi:10.1029/2001JC001041