ABSTRACT
This study was carried out to quantify the physical processes of lakes in the Tibetan Plateau using the Community Land Model, version 4.5 (CLM4.5), coupled with a physically based, 10-layer lake model developed by the National Center for Atmospheric Research. The CLM was forced with 10 km resolution reanalysis data to attempt to understand detailed lake processes and how these processes affect lake surface temperature. In this study, we simulated seasonal and interannual variations of lake surface temperature for Lake Qinghai, Zhaling Co, and Nam Co in the Tibetan Plateau and compared these simulations with observations. The results showed that the CLM4.5 lake model simulations reproduced the observed lake surface temperatures for Lake Qinghai and Zhaling Co well but reproduced those for Nam Co poorly. Through detailed analysis, we found that the simulated biases for Nam Co result largely from the unrealistic parameterization of eddy diffusivity. By expanding this parameter, the lake surface temperature simulations improved remarkably. In addition, erroneous lake ice cover simulations contributed to the simulated lake surface temperature bias in the cold seasons.
RÉSUMÉ
[Traduit par la rédaction] Nous avons entrepris cette étude afin de quantifier les processus physiques des lacs du plateau tibétain à l’aide du Community Land Model, version 4.5 (CLM4.5), couplé à un modèle physique de lac à 10 niveaux, qu’a développé le National Center for Atmospheric Research. Le CLM est piloté par des données de réanalyse d’une résolution de 10 km pour que nous tentions de comprendre les processus détaillés des lacs et la façon dont ces processus agissent sur la température de surface du lac. Dans cette étude, nous simulons les variations saisonnières et interannuelles de la température de surface des lacs Qinghai, Zhaling et Namtso sur le plateau tibétain et nous comparons ces simulations avec les observations. Les résultats montrent que les simulations du modèle CLM4.5 reproduisent bien les températures observées à la surface des lacs Qinghai et Zhaling, mais qu’elles reproduisent mal celles du lac Namtso. Grâce à une analyse détaillée, nous constatons que les biais simulés pour le lac Namtso résultent en grande partie de la paramétrisation irréaliste de la diffusion des tourbillons. En élargissant ce paramètre, les simulations de la température de surface du lac s’améliorent remarquablement. De plus, des simulations erronées de la couverture de glace du lac contribuent au biais de la température simulée à la surface du lac pendant les saisons froides.
1 Introduction
The Tibetan Plateau (TP) well known as the “Asian Water Tower” (Xu et al., Citation2014) embraces the highest elevation inland lakes in the world (Zhang, Yao, Xie, Zhang, & Zhu, Citation2014). The lakes on the TP are sensitive indicators of climate change especially under the backdrop of global warming (Thiery et al., Citation2015; Zhang et al., Citation2016). Previous studies of the TP lakes focused mostly on the shrinkage or expansion of those lakes. The changes in water level and area are highly correlated with regional climate changes (Zhang, Xie, Duan, Tian, & Yi, Citation2011; Zhang, Xie, Kang, Yi, & Ackley, Citation2011; Zhang, Yao, Xie, Kang, & Lei, Citation2013). The reasons for these changes in the water level of the lakes on the TP mainly include precipitation, evaporation, and glacial melt water (Fang et al., Citation2016). Some research results have shown that in the central TP the lake areas have significantly expanded, and such expansion appears to be associated with an increase in precipitation, winter temperature, and runoff during the last decade (Lei et al., Citation2014; Liu, Wang, Yu, Yang, & Zhang, Citation2009).
The lake surface processes of the TP lakes, which often play a significant role in affecting local and regional climate, have not been well understood. Lake water surface temperature (WST) is an important indicator that reflects lake processes and their relationships with surrounding environments (Austin & Colman, Citation2007; Zhang, Yao, et al., Citation2014). WST has a significant impact on local precipitation and climate change (Laird, Desrochers, & Payer, Citation2009; Sun, Xie, Semazzi, & Liu, Citation2015), and it also influences lake surface heat and water fluxes (Haginoya et al., Citation2009). Much of the research has focused on deriving the WST from satellite data or in situ data or on using the WST to explore lake variations. Sharma et al. (Citation2015) offers a valuable dataset of summer lake WST and other related lake information for 291 lakes providing a good basis for investigating lake thermal conditions. Song et al. (Citation2016) assessed spatiotemporal variations in lake WST across the TP and analyzes possible factors that affect the WST variations. Thus, WST is an essential variable that can be used to explore lake–atmosphere interactions.
Numerical computer models are important tools for investigating lake process changes. One-dimensional (1-D) lake models have been widely used because these models can capture the first order of lake processes (Martynov, Sushama, & Laprise, Citation2010; Stepanenko et al., Citation2012). Most 1-D lake models perform well for shallow lake process simulations, while improvements are needed to simulate processes in deep lakes, such as vertical heat transfer, that are quite different than in shallow lakes (Ren, Sun, Chen, & Liu, Citation2013). In this study, we attempt to understand the physical processes that affect the WST on the TP and improve WST simulations for some typical TP lakes. The lake model used is from the Community Land Model, version 4.5 (CLM4.5; Oleson et al., Citation2013) developed by the National Center for Atmospheric Research.
2 Data, model, and methodology
a Study Area
The TP, located within 74°–104°E, 25°–40°N, lies mostly in the southwest of China with an average elevation of 4000–5000 m. The TP has about 1236 lakes with a total area of 47,000 km2 () (Zhang, Yao, Xie, Zhang, & Zhu, Citation2014). We chose three lakes to investigate lake processes for this study including Lake Qinghai, Zhaling Co, and Nam Co, which are larger than many other lakes in the TP. These three lakes are located in the eastern part of the TP where the most meteorological stations are located, so the atmospheric forcing data used to drive our lake model are relatively reliable. The locations of the three lakes in the TP are shown in .
Fig. 1 Geographical distribution of the lakes on the TP. (a) Nam Co, (b) Zhaling Co, and (c) Lake Qinghai.
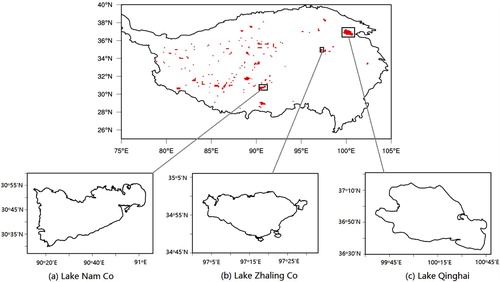
Lake Qinghai, China’s largest inland lake extending from 99°36´–100°47´E, 36°32´–37°15´N, is located in the northeast margin of the TP and is about 3193 m above sea level. It has a water surface area of 4317.69 km2. The closed and saline lake has a mean depth of 21 m, and its water supply comes mainly from precipitation and runoff (Liu et al., Citation2011; Zhang, Xie, Yao, Li, & Duan, Citation2014).
Zhaling Co (97°02´–97°27´E, 34°49´–35°01´N) is located in Maduo County in Qinghai Province and is the largest lake in the upper reaches of the Yellow River. This lake is also the highest freshwater lake in China and has a depth of 13 m at its deepest. The lake is about 1000 m higher in elevation than Lake Qinghai, with a surface area of 526 km2. The water supply to the lake mainly depends on surface runoff and precipitation (Yang & Wen, Citation2012).
Nam Co is located in the central TP (90°16´–91°03´E, 30°30´–30°55´N), with an elevation of 4718 m and is the highest and largest lake in the central TP with little influence from human activity. It has a maximum water depth of about 90 m (Wang et al., Citation2009) with a surface area of about 1920 km2 (Zhu, Xie, & Wu, Citation2010). The water supply to Nam Co is mainly from precipitation and glacier melt. Evaporation is the main cause of the lake water loss (Ma et al., Citation2016; You, Kang, Li, Li, & Liu, Citation2007).
b Data
In this study, we used the gridded China meteorological dataset produced by merging a variety of observational data sources. The dataset is developed by the hydro-meteorological research group at the Institute of Tibetan Plateau Research, Chinese Academy of Sciences (ITPCAS) (Chen et al., Citation2011; He, Citation2010). The dataset covers the 1979–2012 period with a spatial resolution of 0.1° and a time step of three hours. The dataset includes seven variables: near-surface air temperature, air pressure, wind speed, precipitation, specific humidity, and incoming shortwave and longwave radiation.
The simulations were evaluated with remotely sensed data: Moderate-Resolution Imaging Spectroradiometer (MODIS) land products. Many studies have carried out substantial evaluations and validation of MODIS data mainly using in situ data (Wan, Zhang, Zhang, & Li, Citation2004). Monthly MODIS data from 2003 to 2012 with a spatial resolution of 0.05° were used in this study. We used the MODIS water surface temperature from two 0.05° resolution datasets: the Terra MOD11 product and the Aqua MYD11 product, which are derived from daily global products. According to the observed times of MODIS, we obtained four corresponding values from model hourly output and then produced monthly average data to compare with the MODIS products. Both model outputs and MODIS data were averaged over the TP lakes focused on in this study.
c The Lake Model
Much research on hydrodynamic and thermodynamic aspects of lakes has been carried out (Rizzi & Tubino, Citation2004). One-dimensional lake models are extensively used with the reasonable assumption that heat and mass transfer within lakes occur largely in the vertical direction. Some results have shown that no single lake model can accurately simulate the thermodynamic regime in a wide range of lakes under different climate conditions, but simulations of larger and deeper lakes show more significant biases (Stepanenko et al., Citation2012). The CLM4.5 used in our study includes a new lake scheme (Fang & Stefan, Citation1996; Subin, Riley, & Mironov, Citation2012). This version contains some improvements and modifications to the lake code when compared with previous versions (Hostetler, Giorgi, Bates, & Bartlein, Citation1994; Zeng, Shaikh, Dai, Dickinson, & Myneni, Citation2002). The CLM4.5 lake scheme solves the 1-D thermal diffusion equation by dividing the lake vertical profile into ten discrete layers, including water and ice. This lake model calculates WST through surface energy conservation. The vertical mixing considered in this model contains wind-driven eddies, molecular diffusivity, convection, and enhanced diffusivity. For this study, we interpolated the aforementioned gridded China meteorological variables at a three-hour step to a dataset with an hourly time step to drive CLM4.5. The model run time is from 1 January 2000 through 31 December 2012. The simulations for the first three years were discarded as spin-up, and the rest of the simulations were used for analysis. With such a long spin-up time (three years), the effects of the model initial conditions on our simulations were minimized for these shallow lakes (when compared with deep lakes (depth greater than 50 m)). Thus, the lake temperatures were simply initialized with a temperature of 277 K. The lake depths set in the model were mean depths for Lake Qinghai, Zhaling Co, and Nam Co, which were set to 16, 10, and 40 m, respectively.
3 Results and discussion
a Initial Results
The simulations of WST for the three aforementioned lakes over the 2003–2012 period were compared with MODIS data. As we can see in a and 2b, the WST simulations for Lake Qinghai and Zhaling Co agree well with observed MODIS data. We calculated the root mean square error (RMSE) and correlation coefficient (COR) of WST simulations for the three lakes ().
Fig. 2 Initial results of the simulations for the three lakes. The red line is the simulated WST (°C) and the black line shows the MODIS data for (a) Lake Qinghai, (b) Zhaling Co, and (c) Nam Co.
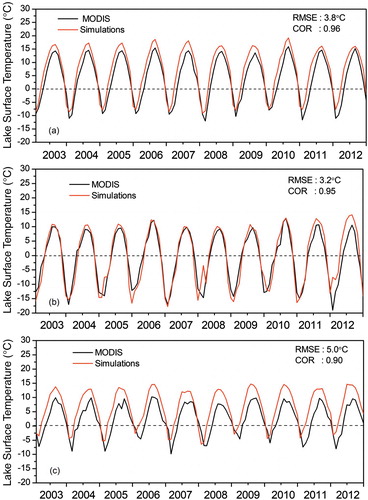
The WST simulations are reasonably accurate for Lake Qinghai and Zhaling Co with RMSEs of 3.8°C and 3.2°C and CORs of 0.96 and 0.95, respectively. However, the WST simulations for Nam Co have a larger RMSE (5.0°C) and lower COR (0.90) than those for Lake Qinghai and Zhaling Co. Thus, the lake model performs better with the WST simulations for Lake Qinghai and Zhaling Co but not for Nam Co. summarizes the results.
Table 1. RMSEs and CORs of Lake Qinghai, Zhaling Co, and Nam Co.
b Model Calibration and Validation
To investigate the physical reason behind the poor simulations for Nam Co, we first examined our forcing data using station data that were collected near the lake shore. We found that our gridded forcing variables for the lake were consistent with the station data except for wind (figures not shown). Thus, we performed a linear regression between the gridded wind and station data and generated the corrected wind data for Nam Co to drive the CLM4.5 lake model. In this model, there are three components in the water diffusivity parameterization: wind-driven eddy diffusivity, enhanced diffusivity, and molecular diffusivity. We compared our simulations with the observed temperature profile data for Nam Co obtained from Lazhu et al. (Citation2016). In a and 3b, we can see that the simulated temperature is lower in the deeper part of the lake (e.g., below 12 m during summer and fall seasons) than observed, even though the simulated surface temperature is much higher than the observed over the same period, indicating that the heat transfer in the model is unrealistically weaker.
Fig. 3 Temperature profiles for Nam Co (°C): (a) observations (from Lazhu et al. (Citation2016)); (b) the default run; and (c) a run with the enhanced eddy diffusivity expanded 1000 times.
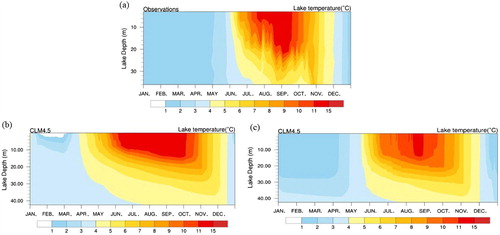
Some researchers have reported weaker water diffusion simulated with the CLM4.5 lake model, which was caused by a smaller eddy diffusivity (e.g., Gu, Jin, Wu, Ek, & Subin, Citation2013) that is parameterized mainly as a function of wind. In reality, eddy diffusion could be affected by other factors, such as lake internal waves as described in the nonlocal K-Profile Parameterization (KPP) for vertical water mixing (Large, McWilliams, & Doney, Citation1994). Subin et al. (Citation2012) improved the eddy diffusion parameterization by adding an enhanced value. However, this modification is still not sufficient during the strong lake stratification shown in this study (e.g., in the summer and fall seasons as shown in ). To better simulate the effects of eddy diffusion on the heat transfer in Nam Co, we increased the enhanced eddy diffusivity in the lake scheme 1000 times, which produced the best results compared with other numbers. The results show that both surface and interior lake temperature simulations were significantly improved (). The RMSE decreases from 5.0°C to 3.0°C and the COR increases from 0.90 to 0.96 compared with those from the default run with the CLM4.5 lake model.
4 Discussion
Although we have improved the WST simulations, biases still exist. The simulated WST is remarkably overestimated over the study period especially in the winter and spring (). We also examined the WST simulations of Lake Qinghai and Zhaling Co for winter and spring and found that the maximum bias reaches 5°C in Qinghai and 11°C in Zhaling Co. Our speculation is that erroneous ice simulations during the cold seasons may cause such biases. In this study, we compared the ice fraction simulations and MODIS data from Lu, Xiao, Jia, Zhang, and Luo (Citation2016) for Nam Co. We can see that the simulated ice cover has a shorter duration than that of the MODIS data and is significantly underestimated over half the 10-year study period (). These simulated ice cover biases may contribute to the biased WST simulations during the cold seasons. The poor ice cover simulations likely result from the oversimplified ice scheme in the CLM4.5 lake code, which only simulates ice fraction based on the energy balance equation. Many detailed ice cycling processes such as ice accumulation and melting are not considered in the model.
Under climate change in the TP (Yang et al., Citation2014), many lakes undergo an increase in water level due greatly to glacier melt. Our study period is from 2003 through 2012. Based on observed data for the 2003–2009 period (Zhang, Xie, Duan, et al., Citation2011), the level of Lake Qinghai increased by 0.67 m, from 3193.35 m to 3194.02 m. We performed sensitivity tests with lake depths of 14, 16, and 18 m to represent a large range of water level increases and examined how such increases affect our simulations. The RMSEs change between 3.86°C and 3.79°C and the CORs vary only between 0.962 and 0.965. The differences among these simulated WSTs using different depths are very insignificant (figure not shown).
In addition, our results indicate that the eddy diffusivity parameterization embedded in the CLM4.5 lake code is unable to handle water mixing during the strong lake stratification as shown in . More factors affecting water mixing, such as those included in KPP for ocean temperature simulations, should be considered in the lake scheme. To resolve the issue permanently, an improved eddy diffusion parameterization needs to be developed, which is beyond the scope of this study and will be an important focus of our research in the near future.
5 Conclusions
In this study, we simulated WST for three large lakes in the TP for the 2003–2012 period. The performance of the lake model in simulating WST for Lake Qinghai and Zhaling Co was good while it simulated WST for Nam Co poorly.
With a large number of sensitivity tests, we found that the water diffusion in Nam Co was the main cause of the errors in the WST simulations. By expanding the enhanced eddy diffusivity 1000 times, we can obtain the best results. Furthermore, there is also a problem simulating the lake ice cover because of the oversimplified lake-ice scheme in the CLM4.5 code in which the ice cover starts early and does not last long. A more realistic ice cycling scheme is needed to better simulate the ice processes and their effects on the WST simulations for those high-elevation lakes.
Acknowledgements
The authors thank Atmosphere-Ocean science editor Guoqi Han and the anonymous reviewers for their invaluable advice. The gridded meteorological dataset for China used in this study as forcing data is accessible from the Third Pole Environment Database (http://en.tpedatabase.cn/portal/MetaDataInfo.jsp?MetaDataId=202). The MODIS lake surface temperature data products (MOD11 and MYD11) used in this study can be obtained from https://modis.gsfc.nasa.gov/data/dataprod.
Disclosure Statement
No potential conflict of interest was reported by the authors.
Additional information
Funding
References
- Austin, J. A., & Colman, S. M. (2007). Lake Superior summer water temperatures are increasing more rapidly than regional air temperature: A positive ice-albedo feedback. Geophysical Research Letters, 34(6), 125–141. doi: 10.1029/2006GL029021
- Chen, Y., Yang, K., He, J., Qin, J., Shi, J., Du, J., & He, Q. (2011). Improving land surface temperature modeling for dry land of China. Journal of Geophysical Research, 116, D20104. doi: 10.1029/2011JD015921
- Fang, X., & Stefan, H. G. (1996). Long-term lake water temperature and ice cover simulations/measurements. Cold Regions Science and Technology, 24(3), 289–304. doi: 10.1016/0165-232X(95)00019-8
- Fang, Y., Cheng, W., Zhang, Y., Wang, N., Zhao, S., Zhou, C., & Bao, A. (2016). Changes in inland lakes on the Tibetan Plateau over the past 40 years. Journal of Geographical Sciences, 26(4), 415–438. doi: 10.1007/s11442-016-1277-0
- Gu, H., Jin, J., Wu, Y., Ek, M. B., & Subin, Z. M. (2013). Calibration and validation of lake surface temperature simulations with the coupled WRF-lake model. Climate Change, 129, 471–483. doi: 10.1007/s10584-013-0978-y
- Haginoya, S., Fujii, H., Kuwagata, T., Xu, J., Ishigooka, Y., Kang, S., & Zhang, Y. (2009). Air-lake interaction features found in heat and water exchanges over Nam Co on the Tibetan Plateau. Sola, 5, 172–175. doi: 10.2151/SOLA.2009-044
- He, J. (2010). Development of a surface meteorological dataset of China with high temporal and spatial resolution [in Chinese]. Master’s Dissertation, Institute of Tibetan Plateau Research, Chinese Academy of Science.
- Hostetler, S. W., Giorgi, F., Bates, G. T., & Bartlein, P. J. (1994). Lake-atmosphere feedbacks associated with paleolakes Bonneville and Lahontan. Science, 263(5147), 665. doi: 10.1126/science.263.5147.665
- Laird, N. F., Desrochers, J., & Payer, M. (2009). Climatology of lake-effect precipitation events over Lake Champlain. Journal of Applied Meteorology and Climatology, 48(2), 232–250. doi: 10.1175/2008JAMC1923.1
- Large, W. G., McWilliams, J. C., & Doney, S. C. (1994). Oceanic vertical mixing: A review and a model with a nonlocal boundary layer parameterization. Reviews of Geophysics, 32(4), 363–403. doi: 10.1029/94RG01872
- Lazhu, Y. K., Wang, J., Lei, Y., Chen, Y., Zhu, L., Ding, B., & Qing, J. (2016). Quantifying evaporation and its decadal change for Lake Nam Co, central Tibetan Plateau. Journal of Geophysical Research: Atmospheres, 121, 7578–7591. doi: 10.1002/2015JD024523
- Lei, Y., Yang, K., Wang, B., Sheng, Y., Bird, B. W., Zhang, G., & Tian, L. (2014). Response of inland lake dynamics over the Tibetan Plateau to climate change. Climate Change, 125(2), 281–290. doi: 10.1007/s10584-014-1175-3
- Liu, J., Wang, S., Yu, S., Yang, D., & Zhang, L. (2009). Climate warming and growth of high-elevation inland lakes on the Tibetan Plateau. Global and Planetary Change, 67(3), 209–217. doi: 10.1016/j.gloplacha.2009.03.010
- Liu, X., Lai, Z., Madsen, D., Yu, L., Liu, K., & Zhang, J. (2011). Lake level variations of Qinghai Lake in northeastern Qinghai-Tibetan Plateau since 3.7 ka based on OSL dating. Quaternary International, 236(1-2), 57–64. doi:10.1016/j.quaint.2010.08.09 doi: 10.1016/j.quaint.2010.08.009
- Lu, S., Xiao, G., Jia, L., Zhang, W., & Luo, H. (2016). Extraction of the spatial-temporal lake water surface dataset in the Tibetan Plateau over the past 10 years [in Chinese with English abstract]. Remote Sensing for Land and Resources, 28(3), 181–187. doi: 10.6046/gtzyyg.2016.03.28
- Ma, N., Szilagyi, J., Niu, G., Zhang, Y., Zhang, T., & Wang, B. (2016). Evaporation variability of Nam Co Lake in the Tibetan Plateau and its role in recent rapid lake expansion. Journal of Hydrology, 537, 27–35. doi: 10.1016/j.jhydrol.2016.03.030
- Martynov, A., Sushama, L., & Laprise, R. (2010). Simulation of temperate freezing lakes by one-dimensional lake models: Performance assessment for interactive coupling with regional climate models. Boreal Environment Research, 15(2), 143–164.
- Oleson, K. W., Lawrence, D. W., Bonan, G. B., Drewniak, B., Huang, M., Koven, C. D., & Yang, Z. (2013). Technical description of version 4.5 of the Community Land Model (CLM) (NCAR Technical Note. NCAR/TN-503+STR), Boulder, Colorado.
- Ren, X., Sun, S., Chen, W., & Liu, H. (2013). A review of researches on the lake numerical modeling [in Chinese with English abstract]. Advances in Earth Science, 28(3), 347–356.
- Rizzi, G., & Tubino, M. (2004). Hydrothermodynamics of Lake Tovel. Part II: Numerical modelling. Studi trentini di scienze naturali–Acta biologia., 81, 291–304.
- Sharma, S., Gray, D. K., Read, J. S., Reily, C. M., Schneider, P., Qudrat, A., & Woo, K. H. (2015). A global database of lake surface temperature collected by in situ and satellite methods from 1985-2009. Scientific Data, 2, 150008. doi: 10.1038/sdata.2015.8
- Song, K., Wang, M., Du, J., Yuan, Y., Ma, J., Wang, M., & Mu, G. (2016). Spatiotemporal variations of lake surface temperature across the Tibetan Plateau using MODIS LST product. Remote Sensing, 8(10), 854. doi: 10.3390/rs8100854
- Stepanenko, V. M., Martynov, A., Johnk, K. D., Subin, Z. M., Perroud, M., Fang, X., & Goyette, S. (2012). A one-dimensional model intercomparison study of thermal regime of a shallow turbid midlatitude lake. Geoscientific Model Development, 6(4), 1337–1352. doi: 10.5194/gmd-6-1337-2013
- Subin, Z. M., Riley, W. J., & Mironov, D. (2012). An improved lake model for climate simulations: Model structure, evaluation, and sensitivity analyses in CESM1. Journal of Advances in Modeling Earth Systems, 4(1). M02001. doi:10.1029/2011MS000072.
- Sun, X., Xie, L., Semazzi, F., & Liu, B. (2015). Effect of lake surface temperature on the spatial distribution and intensity of the precipitation over the Lake Victoria Basin. Monthly Weather Review, 143(4), 1179–1192. doi: 10.1175/MWR-D-14-00049.1
- Thiery, W., Davin, E. L., Panitz, H. J., Demuzere, M., Lhermitte, S., & Lipzig, N. V. (2015). The impact of the African Great Lakes on the regional climate. Journal of Climate, 28(10), 4061–4085. doi: 10.1175/JCLI-D-14-00565.1
- Wan, Z., Zhang, Y., Zhang, Q., & Li, Z. (2004). Quality assessment and validation of the MODIS global land surface temperature. International Journal of Remote Sensing, 25(1), 261–274. doi: 10.1080/0143116031000116417
- Wang, J., Zhu, L., Daut, G., Ju, J., Lin, X., Wang, Y., & Zhen, X. (2009). Bathymetric survey and modern limnological parameters of Nam Co, central Tibet [in Chinese with English abstract]. Journal of Lake Sciences, 21(1), 128–134. doi: 10.18307/2009.0117
- Xu, X., Zhao, T., Lu, C., Guo, Y., Chen, B., Liu, R., & Shi, X. (2014). An important mechanism sustaining the atmospheric “water tower” over the Tibetan Plateau. Atmospheric Chemistry and Physics, 14, 11287–11295. doi: 10.5194/acp-14-11287-2014
- Yang, K., Wu, H., Qin, J., Lin, C., Tang, W., & Chen, Y. (2014). Recent climate changes over the Tibetan Plateau and their impacts on energy and water cycle: A review. Global and Planetary Change, 112(1), 79–91. doi: 10.1016/j.gloplacha.2013.12.001
- Yang, X., & Wen, J. (2012). Numerical simulation of characteristic of atmospheric boundary layer over Lake Gyaring and Ngoring. Plateau Meteorology, 31(4), 927–934.
- You, Q., Kang, S., Li, C., Li, M., & Liu, J. (2007). Variation features of meteorological elements at Namco station, Tibetan Plateau [in Chinese with English abstract]. Meteorological Monthly, 33(3), 54–60.
- Zeng, X., Shaikh, M., Dai, Y., Dickinson, R. E., & Myneni, R. (2002). Coupling of the common land model to the NCAR community climate model. Journal of Climate, 15(14), 1832–1854. doi: 10.1175/1520-0442(2002)015<1832:COTCLM>2.0.CO;2
- Zhang, G., Xie, H., Duan, S., Tian, M., & Yi, D. (2011). Water level variation of Lake Qinghai from satellite and in situ measurements under climate change. Journal of Applied Remote Sensing, 5(1), 3532. doi: 10.1117/1.3601363
- Zhang, G., Xie, H., Kang, S., Yi, D., & Ackley, S. F. (2011). Monitoring lake level changes on the Tibetan Plateau using ICESat altimetry data (2003-2009). Remote Sensing of Environment, 115(7), 1733–1742. doi: 10.1016/j.rse.2011.03.005
- Zhang, G., Xie, H., Yao, T., Li, H., & Duan, S. (2014). Quantitative water resources assessment of Qinghai Lake basin using Snowmelt Runoff Model (SRM). Journal of Hydrology, 519(976–987), 976–987. doi: 10.1016/j.jhydrol.2014.08.022
- Zhang, G., Yao, T., Piao, S., Bolch, T., Xie, H., Chen, D., & Zhang, H. (2016). Extensive and drastically difference alpine lake changes on Asia’s high plateaus during the past four decades. Geophysical Research Letters, 44, 252–260. doi: 10.1002/2016GL072033
- Zhang, G., Yao, T., Xie, H., Kang, S., & Lei, Y. (2013). Increased mass over the Tibetan Plateau: From lakes or glaciers? Geophysical Research Letters, 40(10), 2125–2130. doi: 10.1002/grl.50462
- Zhang, G., Yao, T., Xie, H., Qin, J., Ye, Q., Dai, Y., & Guo, R. (2014). Estimating surface temperature changes of lakes in the Tibetan Plateau using MODIS LST data. Journal of Geophysical Research: Atmospheres, 119(14), 8552–8567. doi: 10.1002/2014JD021615
- Zhang, G., Yao, T., Xie, H., Zhang, K., & Zhu, F. (2014). Lake’s state and abundance across the Tibetan Plateau. Chinese Science Bulletin, 59(24), 3010–3021. doi: 10.1007/s11434-014-0258-x
- Zhu, L., Xie, M., & Wu, Y. (2010). Quantitative analysis of lake area variations and the influence factors from 1971 to 2014 in the Nam Co basin of the Tibetan Plateau. Chinese Science Bulletin, 55(13), 1294–1303. doi: 10.1007/s11434-010-0015-8