Abstract
A simple method for reducing the uncertainty intervals of models describing the maturation of Venturia inaequalis (Cooke) Wint ascospores is described. The number of trapped ascospores related to the current accumulated degree days of the season, and the confidence interval predicted with the historical ascospore release datasets, were used to re-calculate the confidence interval for the ascospore proportion for the rest of the season. The historical uncertainty in ascospore proportion was described with logistic functions fitted to upper and lower confidence limits of the mean of fitted values for several years of ascospore release data. The re-calculated confidence interval was derived by the determination of the total number of ascospores that would be trapped during the season, if the current day cumulative release were at either the upper or lower confidence limit of the historical data. The method was examined by applying three models of ascospore maturation and using ascospore trap and weather data collected in Skierniewice, Poland during the period from 2005 to 2009. The historical uncertainties were established by the calibration of models to the data from 2005–2007. The effectiveness of the method was simulated using data of 2008 and 2009. In these years, the re-calculated confidence interval was never greater than the historical confidence interval and was often 3–4 times smaller than it, even though ascospore release observations in 2009 strongly differed from those of the years 2005–2007. In conclusion, the proposed method may be an effective approach to a more precise description of V. inaequalis ascospores maturation.
Résumé
Cet article traite d’une méthode simple pour réduire les intervalles d’incertitude des modèles décrivant la maturation des ascospores de Venturia inaequalis (Cooke) Wint. Le nombre d’ascospores piégées relativement au nombre de degrés-jours accumulés durant la saison ainsi que l’intervalle de confiance prédit avec l’ensemble des données historiques de libération des ascospores ont été utilisés pour recalculer l’intervalle de confiance relatif à la proportion d’ascospores pour le reste de la saison. L’incertitude historique associée à la proportion d’ascospores a été décrite avec des fonctions logistiques ajustées aux limites de confiance supérieures et inférieures de la moyenne des valeurs effectives de plusieurs années de données relatives à la libération d’ascospores. L’intervalle de confiance recalculé a été déduit en déterminant le nombre total d’ascospores qui seraient piégées durant la saison si la libération cumulée de la journée se situait soit à la limite de confiance supérieure ou inférieure des données historiques. La méthode a été testée en appliquant trois modèles de maturation d’ascospores ainsi qu’en utilisant un piège à ascospores et les données météorologiques collectées à Skierniewice, en Pologne, de 2005 à 2009. Les incertitudes historiques ont été établies par l’étalonnage de modèles en fonction des données de 2005 à 2007. L’efficacité de la méthode a été simulée avec les données de 2008 et 2009. Durant ces années, l’intervalle de confiance recalculé n’était jamais plus grand que l’intervalle de confiance historique et était souvent de trois à quatre fois plus petit que ce dernier, et ce, même si les observations de libération d’ascospores de 2009 différaient considérablement de celles de 2005 à 2007. Pour conclure, la méthode proposée peut servir à décrire avec plus de précision la maturation des ascospores de V. inaequalis.
Introduction
Apple scab, caused by the fungal pathogen Venturia inaequalis (Cooke) Wint, is the most harmful apple disease in temperate regions throughout the world (MacHardy Citation1996). Scab control in commercial orchards usually involves a series of 6 to 16 fungicide sprays per season (Holb Citation2009). Such extensive use of fungicides is expensive and contributes to accumulation of fungicide residues on the fruits, whilst creating a negative impact on the ecosystem (Culliney et al. Citation1992; Cooley & Manning Citation1995; Wang et al. Citation2009), and stimulating the development of fungicide resistance in the pathogen (Cox et al. Citation2008; Meszka et al. Citation2008; Viljanen-Rollinson et al. Citation2013).
The main approach aimed at limiting the number of sprays is based on fungicide programs predicting the timing of V. inaequalis ascospore release and the risk of scab infection. In Poland and in many other European Union countries, the software most widely used for calculating these parameters is the commercial RIMpro (Trapman Citation1993). Since its algorithms are not known, a new model with an accessible structure and algorithms called A-scab was elaborated (Rossi et al. Citation2007). The model was developed and validated basing on experimental data from the Po Valley, Italy. The main rationale for creating this new program was to allow users to adjust model performance to local conditions.
The work presented in this paper is part of a project which aims to create a similar model to the A-scab system, destined for the Polish region of Mazovia, and specifically the surroundings of the city of Grójec, known by its citizens as the ‘largest orchard in Europe’. On 5 October 2011, the EU Commission registered ‘Jabłka grójeckie’ (Grójec apples) as a Protected Geographical Indication, thus appreciating the importance of apple production in the region (Commission Implementing Regulation (EU) No 981/Citation2011).
In order to predict ascospore discharges, their seasonal maturation pattern was first described as a function of weather conditions. The mathematical models estimating the proportion of ascospores that already became mature (PAM) in relation to all ascospores that mature during the season have been developed and thoroughly examined for many years (Massie & Szkolnik Citation1974; Gadoury & MacHardy Citation1982; James & Sutton Citation1982b; MacHardy & Gadoury Citation1985; St-Arnaud & Neumann Citation1990; Stensvand et al. Citation1997, Citation2005; Rossi et al. Citation1999, Citation2000; Gadoury et al. Citation2004).
PAM can be described using the logistic function taking the accumulated degree days (DDC – degree days calculated in Celsius) as the argument. DDC is calculated in different ways in various models, but generally it is positively correlated to the level of moisture in the air at a given time.
Experimental data used to estimate the parameters of the logistic function can be obtained in two ways. Since PAM represents the actual proportion of mature ascospores during the season, it can be estimated by means of laboratory observation of ascospore maturation (Gadoury & MacHardy Citation1982). More often it is approximated with observed accumulation of ascospores trapped during release events in an orchard (PAT – proportion of ascospores trapped) (Rossi et al. Citation1999, Citation2000; Stensvand et al. Citation2005). The logistic curve used in ascospore maturation models represents the best prediction, showing the most probable course of the maturation of ascospores in the coming years. The predictions are presented along with curves representing the lower and upper limits of the confidence interval for the predictions (in the present study, the confidence limits were also described using the logistic function). The confidence band represents the uncertainty of the model in predicting the maturation of ascospores. In the A-scab model, the uncertainty limits are used to estimate the time when the risk of ascospore discharge begins and when it is at its maximum. The possibility of reducing the confidence band is very limited even for long-term observations because models generally include only a small number of factors which influence ascospore maturation and because of the intrinsically high variability of weather data.
The aim of this study was to present a method for reducing the confidence band of V. inaequalis spore maturation model. The reduction is based on the redetermination of the logistic curves describing the model after each new ascospore release event occurring in the current year. The approach is tested with three different models of V. inaequalis ascospore maturation, and calibrated for Polish climate conditions by means of a fit to 3-year experimental PAT data collected.
Materials and methods
Monitoring of weather conditions at the experimental orchard
Weather monitoring was conducted at the Experimental Orchard of the Research Institute of Horticulture in Skierniewice, Poland (51°55ʹN, 20°6ʹE) located in the Grójec area. Weather data were collected over 5 consecutive years, from 2005 to 2009. The temperature, precipitation, relative humidity and leaf wetness were recorded continuously from January to June with an automatic weather station (model Metos-Compact, Pessl Instruments, Weiz, Austria). Leaf wetness was measured at a height of 20 cm above the ground, while the other weather parameters were measured at a height of 2 m. Data were collected at intervals of 12 min and averaged as hourly data.
Ascospore trapping
A Burkard 7-day recording volumetric spore trap (Burkard Manufacturing Co. Ltd, Rickmansworth, UK) was used to monitor the release of ascospores from the leaves of apple ‘McIntosh’. Approximately 2 m2 of the ground under the Burkard sampler were covered with apple leaves heavily infected by V. inaequalis in the previous season. The trees in the orchard were not treated with fungicides during the years of the experiment or during the previous 10 years. The spore trap was installed in the centre of this area and was adjusted to sample air 1 m above the ground at a rate of 0.6 m3 h−1. The tape from the Burkard spore trap (with a length of 336 mm and a width of 19 mm), was changed weekly. The weekly segments of tape were cut into seven 48 mm long and 19 mm wide segments, representing the length covering a period of 1 day sampling. The daily segments of tape were further cut into twenty-four 2 mm long and 19 mm wide strips corresponding to 1 h sampling.
In every year, the number of V. inaequalis ascospores deposited on every 2 mm long strip of the tape, starting from the beginning and ending with the closure of the ascospore discharge season, was counted using a compound microscope (200× magnification). Thus, a precise distribution of the number of spores deposited in the spore trap in the following hours of the season was obtained. Ascospore counts were converted into counts per unit volume of sampled air. The cumulative proportion of ascospores trapped (PAT) was calculated for each ascospore discharge event as the ratio between the amount of ascospores trapped from the beginning of the season to the end of the specific discharge, and the total number of ascospores trapped during the season (Rossi et al. Citation2000).
Ascospore release events
In this study, the ascospore release data were analysed on an hourly scale with the following principles, adopted for the description of the observational data. A discharge was considered to be terminated when it was followed by 4 hours without trapped ascospores. For an hour of discharge observation, we considered the mean of the release event calculated as a weighted average in the form:
Ascospore maturation models used in the study
In the study, three different models of V. inaequalis ascospore maturation were used: ‘All Hours’ model (New Hampshire model, Gadoury & MacHardy Citation1982), ‘Rainy Hours’ model (Rossi et al. Citation1999) and ‘Stensvand Rule’ model (Stensvand et al. Citation1998, Citation2005). In origin, the models were defined on a daily scale, but we adapted them to an hourly scale.
All three models are based on a proposal by Massie & Szkolnik (Citation1974) who introduced the first model of V. inaequalis ascospore maturation, describing the change in proportion of mature ascospores (PAM) observed in a given season as a function of cumulated degree day (DDC) above 0 °C. Since ascospores mature when exposed to moisture, generally the accumulation of temperature depends on the level of moisture in the air at a given time, and it is expressed as:
Furthermore, within all three models, we applied an approach introduced by Rossi et al. (Citation1999), in which the cumulative proportion of mature ascospores observed to the hour h is described as a logistic function of cumulative temperature:
The models of V. inaequalis ascospore maturation used in this study differ in the definition of the weight Wh. In the ‘All Hours’ model, this weight is equal to 1 (Wh = 1). In the ‘Rainy Hours’ model, Wh = 1 in the case of rainfall and Wh = 0 in the absence of precipitation. In the ‘Stensvand Rule’ model, Wh = 1 in the case of rain, in hour h and during the next 168 hours (7 days) and Wh = 0 at all other times.
In each model, the beginning of temperature accumulation (biofix) was determined as proposed by Rossi et al. (Citation1999), as the pseudothecial development stage 9.5 (the moment when 2% of pseudothecia contain pigmented and mature ascospores). The microscopic evaluation of the pseudothecial development was conducted each year between the second half of February and the end of ascospore discharge, usually by mid-June. At intervals of 7 days, 10 leaves from the ground were collected at random and 10 pseudothecia from each of them (100 pseudothecia in total) were examined under the microscope.
Calibration of the models of ascospore maturation and determination of their confidence intervals
Observations of ascospore maturation performed between 2005 and 2007 were used to calibrate the models. The best prediction of each examined model and its confidence limits (estimating the uncertainty of the model predictions) were calculated according to Rossi et al. (Citation2000). At first, the model was fitted to the experimental data separately for each year of observations: the best parameters α and β of the logistic function (equation (3)) were determined for each year using a non-linear regression method implemented in R (Fox Citation2002). The obtained logistic function was then used to calculate the predicted ascospore proportions at constant intervals of cumulated temperature from 0 °C to the maximum DDC value obtained with that model. In the ‘All Hours’ model, the maximum DDC value was 1000 °C and the chosen interval was 10 °C, in the ‘Rainy Hours’ model the DDC reached 60 °C and the chosen interval was 0.5 °C, in the ‘Stensvand Rule’ model the maximum DDC value was 800 °C and the chosen interval was 8 °C. For each DDC value, the PAT mean from 3 years and its standard deviation were applied to compute the 95%, 99% and 99.5% (or 99.9%) confidence intervals for the means. As a result of the above procedure, seven sets of PAT data were created at constant DDC intervals for each model, formed by the 3-year mean values and their 3 upper and 3 lower confidence limits. Finally, the logistic function was fitted to each of seven datasets using a non-linear regression method. The logistic function corresponding to the 3-year mean PAT values estimated the best prediction of the model for the 3-year data, while other functions estimated its lower and upper uncertainty bands. The goodness of the best prediction of the model was estimated with the χ2 and R2 values. The χ2 for the combined 3-year data was calculated as:
Method for reducing the limits of uncertainty of the models of ascospore maturation
The proposed method for reducing the limits of uncertainty of the models of ascospore maturation will be presented alongside an example. First consider the predictions of ascospore maturation obtained for the year 2008 in the ‘All Hours’ model. The confidence limits of the model are based on the historical data from years 2005–2007 computed at the 99.5% level of significance and we consider the observation of the 10th ascospore release event in the 2008 season. The cumulative temperature and the absolute number of spores released up to the time of this observation are the kind of information obtained from each of the observed release events in 2008 (a). What remains unknown is the proportion of seasonal spores obtained in each of the release events. However, for a particular value of the total number of the ascospores in a given season, the proportion of seasonal ascospores for each release event can be calculated. Depending on the total number of seasonal ascospores, a different layout of observational points is obtained (). The range of acceptable layouts applying the predicted historical 3-year confidence interval can be reduced, assuming that none of the observational points is allowed to overcome the interval limits. Under such an assumption, two extreme layouts for the observed points can be found: the first one, in which the outermost point lies on the upper confidence limit and the second one, where the outermost point lies on the lower confidence limit (). One can find these layouts using a simple computer program such as Excel. Subsequently, the information about the historical confidence interval of the model is added to both the extreme layouts of the observed points, in the form of additional arbitrary points placed on each of the confidence limits above the observed points (). In this way, two sets of points are obtained, to which the logistic curves are fitted, and which become new updated limits of model uncertainty ().
Fig. 1 Outline of the proposed method for reducing the limits of uncertainty of the models of ascospore maturation. The outline is based on cumulative proportion of the season’s ascospores trapped (PAT) data from the year 2008, the ‘All Hours’ model, and the observation of the 10th ascospore release in the season. DDC, accumulated degree days in Celsius. The confidence interval of the model is based on data from the years 2005–2007, computed at the 99.5% level of significance. (a) Values of the cumulative temperature and the absolute numbers of ascospores in the subsequent release events. (b) Proportion of seasonal ascospores observed in the subsequent discharges, depending on the assumed total number of ascospores observed in 2008. (c), Extreme layouts of the observed points: first, in which the outermost point lies on the upper confidence band and the second in which the outermost point lies on the lower confidence band. (d), Inclusion of additional points lying on the confidence limits. (e), New updated limits of the model’s uncertainty.
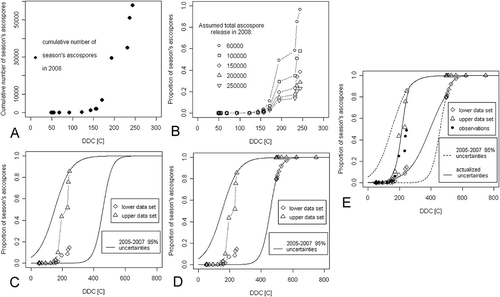
Additional arbitrary points on the limits of the confidence interval added to the two extreme layouts of the observed points could be supplemented in various amounts and locations on the confidence limits. One common method of addition of the arbitrary points was applied in each model of spore maturation used in this study. The same number of additional points as the number of ascospore release events observed to the current moment was used. The cumulative temperature of the first additional point was calculated, for both confidence limits, as the average between the DDC of the most recent ascospore release event and the maximum DDC value achieved in the model, in the years 2005–2007. The spacing between additional points was computed according to the geometric sequence with the common ratio equal to 2, which leads to the doubling of the distance between each successive pair of points. All these assumptions are arbitrary, but were dictated by the desire to maintain a balance between the impact of the known, already observed, and the unknown, ascospore releases in the season on the updated limits of model uncertainties, still awaiting observation.
Visualization of the method’s results
There are three ways of presenting the results of the method for reducing the limits of uncertainty of the ascospore maturation models. In the first manner, a different updated course of uncertainty limits obtained after each new observation of the ascospore discharge is presented. An example of such an arrangement is shown in . The second manner that can be used to evaluate the effectiveness of the updating method after the end of the ascospore release season is by using collective graphs, as shown in . For each pair of successive ascospore release events, i and i + 1, corresponding to the seasonal ascospore proportions PATi and PATi+1, the updated uncertainty limits calculated including all observations up to observation i are presented within the PATi – PATi+1 range. In this way, we obtain the envelope of the updated uncertainties calculated for a given model in the whole season. Such plots can be therefore called the ‘envelope’ plots. The third manner that can be applied to estimate the uncertainty of DDC for a given PAT value is presented in : the difference between the cumulative temperature values for the lower and upper uncertainty limits corresponding to each PAT value is plotted (for each PAT value this difference equals the width of the uncertainty interval presented in ).
Fig. 2 Visualization of the results of the method for reducing the limits of uncertainty of the models of ascospore maturation. (a) Updated course of uncertainty limits obtained after a new observation of the ascospore discharge. (b) ‘Envelope’ plot of the updated uncertainties calculated for a model after the end of the ascospore release season. (c), (d) Width of the uncertainty interval of the accumulated degree days for a given value of the proportion of season’s ascospores.
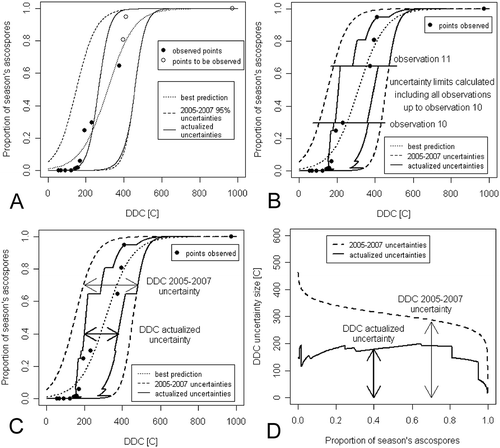
Testing the influence of arbitrary parameters of the method on its performance
To test the method performance, the influence of two arbitrary parameters, the number and location of the artificial points added to two extreme layouts of the observed points, upon the updated confidence limits, was examined. In the first test, for each model, and both the years 2008 and 2009, we varied the number of artificial points, considering 0.5, 2, 4 and 8 fold the number of ascospore release events observed to the current moment. In the second test, for each model, and both years 2008 and 2009, we used the same number of artificial points as the number of ascospore release events observed to the current moment, but varied the location of the artificial points on the confidence limits. The test was performed on four artificial datasets, created by:
Equal spacing between artificial points, with the DDC of the first artificial point equal to the DDC of the most recently observed ascospore release event (denoted as DDC_recent_observation).
Computing the spacing between artificial points according to a geometric sequence, with the DDC of the first artificial point equal to the DDC of the most recently observed ascospore release event.
Equal spacing between artificial points, with the DDC of the first artificial point calculated as the average of temperature corresponding to the most recent ascospore release event (DDC_recent_observation) and the maximum DDC value obtained in the model for the years 2005–2007 (denoted as DDC_max).
Computing the spacing between artificial points according to a geometric sequence with the common ratio equal to 2, with the DDC of the first artificial point calculated as the average of temperature between the most recent ascospore release event and the maximum DDC value for the years 2005–2007.
Numerical analysis
All datasets were analysed using R v. 2.9.2 (R Development Core Team Citation2009).
Results
Observation of ascospore release events
The ascospore release periods spanned from the middle of March to the middle of June, depending on the year of observation. The determined biofix dates were 9 April (2005), 14 April (2006), 10 March (2007), 12 March (2008) and 29 March (2009). In the year 2005, 48 345 ascospores were trapped during 28 release events observed between 16 April and 16 June. The highest amount of 211 659 ascospores was observed in the year 2006. The ascospores were trapped during 19 release events observed between 29 April and 1 June. The lowest amount of 9295 ascospores was observed in the year 2007. The ascospores were trapped during 20 release events observed between 21 March and 4 June. In 2008, 121 121 ascospores were trapped during 26 release events observed between 12 March and 13 June. In 2009, 118 373 ascospores were trapped during 19 release events observed between 17 April and 7 June.
Calibration of the models of ascospore maturation and their confidence intervals
The results of models calibration with the experimental PAT data from 2005–2007 are shown in . The greatest agreement with the data was obtained in the ‘Rainy Hours’ model (χ2 = 0.67, R2 = 0.93); the ‘Stensvand Rule’ model resulted in slightly weaker agreement (χ2 = 0.79, R2 = 0.91), while the least accurate was the ‘All Hours’ model (χ2 = 1.3, R2 = 0.86). The ‘Rainy Hours’ model showed the narrowest confidence intervals; the confidence intervals of the two remaining models were of comparable width, yet both broader than the confidence interval of the ‘Rainy Hours’ model. The results differed substantially for the ranges of cumulated temperatures. The highest cumulative temperature obtained for the data from 2005–2007 was 60 °C degree days for the ‘Rainy Hours’ model and 820 °C and 900 °C for the ‘Stensvand Rule’ and ‘All Hours’ models, respectively.
Fig. 3 Results of the calibration of the models used in the study to the cumulative proportion of season’s ascospores trapped (PAT) data, collected in Skierniewice, Poland, in the years 2005–2007. DDC, accumulated degree days in Celsius. (a), ‘All Hours’ model. (b), ‘Rainy Hours’ model. (c), ‘Stensvand Rule’ model.
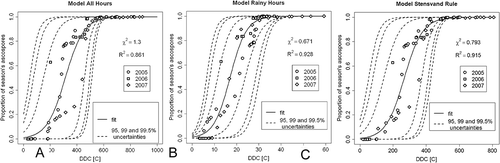
Reducing the limits of uncertainty of ascospore maturation models
The ascospore maturation data from 2008 and 2009, used for testing the proposed method of updating the limits of uncertainty in the ascospore maturation models, showed various levels of similarity to data from 2005–2007, which were used in the calibration of the models. The data from 2008 were similar to the predictions based on the observations from 2005–2007; however, the data from 2009 diverged significantly from them, being characterized by a very rapid maturation of ascospores in the early phase of their development. For this reason, and due to the varying accuracy of the models used in the study, for each model and each year, we applied confidence bands corresponding to different levels of statistical significance. We adopted the principle whereby the chosen 2005–2007 confidence intervals had to contain all the data from the tested season (either 2008 or 2009). Therefore, with the ‘All Hours’ model, the confidence interval was calculated for significance levels of 95% and 99.5% for the years 2008 and 2009, respectively, whereas with the ‘Rainy Hours’ model, the confidence interval corresponded to 99.5% and 99.9%, and for the ‘Stensvand Rule’ model, the confidence interval was 95% and 99%, respectively, for 2008 and 2009.
An example of the results obtained by applying our method to reduce the limits of uncertainty of the ascospore maturation models is shown in , where the updated uncertainty limits of the ‘All Hours’ model obtained after selected observations of ascospore release events in 2008 are presented. The full results obtained with each tested model are presented in the form of animations in the supplementary materials, as follows: Suppl. Anim. 1 and Suppl. Anim. 2 for the ‘All Hours’ model, Suppl. Anim. 3 and Suppl. Anim. 4 for the ‘Rainy Hours’ model and Suppl. Anim. 5 and Suppl. Anim. 6 for the ‘Stensvand Rule’ model. These results are also shown in the ‘envelope’ graph of .
Fig. 4 Updated limits of uncertainty of the ‘All Hours’ model obtained after the chosen observations of ascospore release events in 2008. (a)–(d), Number of observations equals 8, 14, 20 and 24, respectively. Cumulative proportion of season’s ascospores trapped (PAT), described as a function of accumulated degree days in Celsius (DDC). The full results obtained in each tested model are presented in the form of an animation within the supplementary materials: Suppl. Anim. 1 and Suppl. Anim. 2 for the ‘All Hours’ model, Suppl. Anim. 3 and Suppl. Anim. 4 for the ‘Rainy Hours’ model and Suppl. Anim. 5 and Suppl. Anim. 6 for the ‘Stensvand Rule’ model.
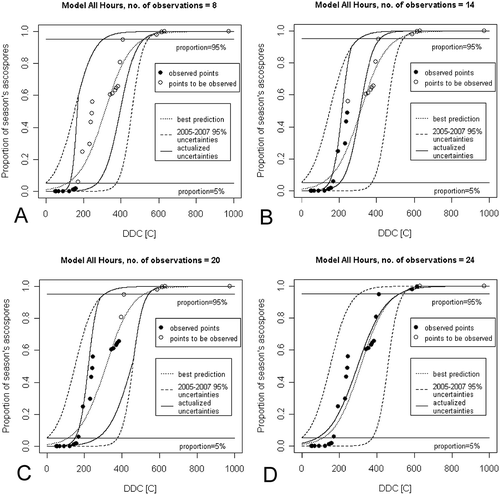
Fig. 5 ‘Envelope’ plots of updated uncertainties of the ascospore maturation models. (a), (b) ‘All Hours’ model. (c), (d), ‘Rainy Hours’ model. (e), (f), ‘Stensvand Rule’ model. Cumulative proportion of season’s ascospores trapped (PAT) described as a function of accumulated degree days in Celsius (DDC). The confidence intervals based on the 2005–2007 data used in each model are highlighted with bold dashed lines. Observations beyond the limits of the updated uncertainty are highlighted with circles. The graphs indicate also the levels of 5% and 95% mature ascospores in the season.
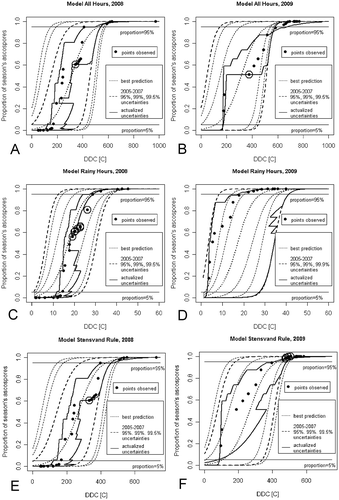
These figures indicate the levels of 5% and 95% mature ascospores in the season. In the majority of the investigated years, the observed ascospore release events before reaching the 5% level, and after crossing the 95% level, were much weaker than the release events in the remaining period of fast ascospore development. Hence, the indicated levels specify the most important period of ascospore maturation, whose correct description was our main goal.
As can be seen, for most of the observations, the method gives correct results, that is, when the next observation point fits within the reduced uncertainty limits calculated using all previous observations. For the data from 2008, for all models of ascospore development (, c and e), the method fails slightly for the 16th release event (in case of the ‘Rainy Hours’ model it happens also for seven following observations), which was associated with the sudden slowing of ascospore maturation accompanied by a significant increase of cumulative temperature. A similar, but more significant, underestimation of model uncertainty was observed for the third data point from the year 2009 in the ‘All Hours’ model (). Less significant error concerned the ‘Stensvand Rule’ model in the year 2009, for the points lying above the level of 95% mature ascospores, which partly also lay beyond the limits of the updated model uncertainty ().
The estimated uncertainty of the cumulative temperature for each PAT value, calculated as the difference between DDC values for the lower and upper uncertainty limits corresponding to the PAT value (the width of the uncertainty interval for each PAT value), are presented in . They demonstrate that it is possible to obtain even a 3–4 fold reduction in the uncertainty interval for some ranges of cumulative temperature compared with the uncertainty predicted solely with the historical ascospore release dataset (for example, for the ‘All Hours’ and ‘Rainy Hours’ models in 2008). Nevertheless, in the case of a small number of events of ascospore release, among all ascospore releases observed in 2008 and 2009, the updated uncertainties were underestimated.
Testing the influence of arbitrary parameters of the method on its performance
The ‘envelope’ plots showing the results of the proposed method, used with various numbers and locations of the artificial points added to two extreme layouts of the observed points, are presented in the supplementary materials (Suppl. Figs 1–12).
The plots presented in Suppl. Figs 1–6 were obtained for the numbers of artificial points 0.5, 2, 4 and 8 fold the number of ascospore release events observed to the current moment. They show that for all models used in both the years 2008 and 2009, the increase of the number of artificial points widened the uncertainty intervals calculated according to the proposed method.
In contrast, the plots in Suppl. Figs 7–12, obtained with various locations of the artificial points on the confidence limits, show that uncertainty bands calculated with the DDC of the first artificial point equal to DDC_recent_observation, were broader than uncertainty bands calculated with the DDC of the first artificial point equal to the average of DDC_recent_observation and DDC_max. The narrowest uncertainty bands were obtained for equally spaced artificial points starting at the average of temperature corresponding to the most recent ascospore release event and the maximum DDC value calculated from 2005–2007.
Discussion
In this study, we presented a method for reducing the limits of uncertainty of models describing the change in proportion (from 0 to 1) of mature V. inaequalis ascospores observed in a given season. The method was introduced in order to illustrate a more general approach based on a simple assumption that the predictions of the ascospore maturation, which result from the long-term observations in the previous years, can be combined with the observations of the ascospore discharges in the studied season.
None of the mathematical models proposed in the literature (Massie & Szkolnik Citation1974; Gadoury & MacHardy Citation1982; James & Sutton Citation1982b; MacHardy & Gadoury Citation1985; St-Arnaud & Neumann Citation1990; Stensvand et al. Citation1997, Citation2005; Rossi et al. Citation1999, Citation2000; Gadoury et al. Citation2004) would give exact predictions of ascospore maturation in any year and location. This is due to various reasons:
The different effects of moisture and temperature (the two main factors affecting ascospore development) on ascospore maturation in various seasons (James & Sutton Citation1982a). Moreover, the moisture may result from various sources such as rain, dew or high relative humidity (Rossi et al. Citation1999). In order to create a reasonable mathematical model, the number of weather factors must be limited and their interdependence must be simplified. The inclusion of too many factors may lead to the model over-fit: models give very good results for the data used to calibrate its parameters and very poor results with data collected from following years’ observations (Hawkins Citation2004);
The variability of weather conditions between seasons and various locations as well as changes in climate, observed in many countries including Poland, may influence the occurrence, prevalence and severity of plant diseases (Kożuchowski & Degirmendžić Citation2005; Blazejczyk Citation2006; Juroszek & Von Tiedemann Citation2011; Pautasso et al. Citation2012);
The possible variability in V. inaequalis development pattern due to high levels of genetic diversity within its populations and further evolution (Tenzer & Gessler Citation1999; Padder et al. Citation2011; Stukenbrock & Bataillon Citation2012).
The possibility of reducing the confidence band is very limited even for long-term observations for the same reasons as described above. One of the methods used to limit the uncertainty of the model predictions is to apply current observational data. Such an approach, as proposed in the present study, resembles the methods of real-time calculation performed in various fields. For example, all numerical weather models predict the future weather based on current weather conditions (Richardson Citation2007). Another method widely used in various real-time data analysis is called ‘sliding windows’ (Gembris et al. Citation2000; Esposito et al. Citation2003; Zhang et al. Citation2009). In this approach, a time window (of constant width) is used to select data for analysis and is stepped forward in regular increments in order to include the most recently available data. In the case of the proposed method, we do not slide the window of recent data but broaden it, starting at the beginning and finishing at the end of the current season. Additionally, we combine the current data with artificial data resulting from the long-term model predictions. The increment of the window in the following steps of the proposed procedure is not regular: the size of each window increment results from the interval between consecutive ascospore release events.
The method proposed in the study was applied to three different ascospore maturation models to assess its general correctness. The models of ascospore maturation chosen for examination of the method assume very different influences of weather conditions on temperature accumulation. The ‘All Hours’ model treats equally all weather conditions and therefore does not include the possibility of the occurrence of too dry weather conditions for the ascospore development. On the other hand, the ‘Rainy Hours’ model allows for accumulation of temperature only during rainy hours, hence omitting the prolonged effect on ascospore maturation of moisture accumulated in the orchard after the cessation of rainfall. The ‘Stensvand Rule’ model is based on a proposal by Stensvand et al. (Citation1998) to take into account both of the above factors. The model assumes that apple leaves lying on the orchard floor remain damp for some time after rain, and therefore the accumulation of temperature in this model occurs during rain and during 7 days after its cessation.
In the study, we concentrated on the demonstration of the principles of the proposed method, and therefore only 3-year weather and ascospore trapping data were applied to calibrate the chosen ascospore maturation models. Within future applications, a dataset collected over a period of at least 10 years should be used in order to take into account the inter-seasonal variability of the weather in the studied region of Poland. Moreover, though the region of Grójec is very homogeneous and has a characteristic microclimate (Council Regulation (EC) No 510/2006 ‘Jabłka Grójeckie’), the dataset utilized to calibrate the models should include data from more than one location to represent the variability of the population of V. inaequalis pseudothecia occurring in the region.
As shown by the study’s results (), the proposed method does reduce the uncertainty bands of the proportion of the season’s matured ascospores for all of the chosen spore maturation models. Most importantly, the predicted reduced uncertainty bands are correct, in the sense that in most cases they contain the consecutive ascospore release observations. Hence, the method may be applied independently of the maturation model used in a fungicide program forecasting the period of V. inaequalis ascospore releases and the scab infection risk.
The other important issue, from a practical point of view, is the prediction of the DDC for a given PAT value with the uncertainty of DDC as narrow as possible. The critical periods of ascospore development, crucial for orchard protection, such as those of highest infection probability, may be related to particular stages of ascospore maturity and hence particular values of the observed PAT. The proposed method allowed narrowing the initial width of DDC uncertainty obtained by calibration of the models to the 2005–2007 data from the width corresponding even up to a 99.5% and 99.9% level of significance to a width comparable to, or below, the width of DDC uncertainty calculated at the 95% level of significance ().
Fig. 6 Predicted uncertainty of the accumulated degree days (in Celsius – DDC), expressed as the width of the uncertainty interval for each observed value of the cumulative proportion of season’s ascospores trapped (PAT). (a), (b), ‘All Hours’ model. (c), (d), ‘Rainy Hours’ model. (e), (f), ‘Stensvand Rule’ model. Uncertainties based on the 2005–2007 confidence intervals, corresponding to three significance levels, 95%, 99% and 99.5% are drawn with dashed lines, with the line corresponding to the significance level chosen for a given model highlighted with the bold line. The updated uncertainties are highlighted with solid bold lines. Areas where the updated uncertainties of cumulative temperature do not contain all experimental points are distinguished with a dashed bold line.
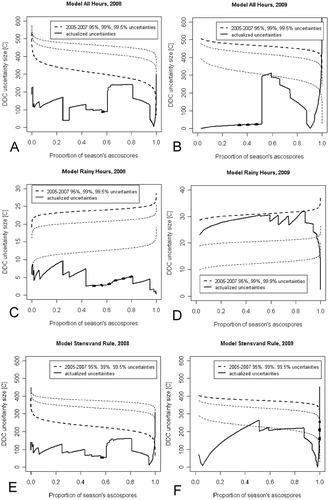
The usefulness of the proposed method can be judged especially considering the data from 2009, when ascospore release observations differed strongly from the observations of previous years. With these data it was necessary to apply very broad confidence intervals of model predictions obtained by calibration of the models to the 2005–2007 data, to avoid that some of the observation points would extend beyond the confidence limits. In the case of the ‘All Hours’ model in 2009, despite a broad confidence band, some points at PAT>95% were not contained within the initial confidence limits. Application of the proposed model allowed a correction of these wrong uncertainty predictions, giving safe and much narrower uncertainty limits, thus improving the predictive capabilities of the model itself.
The results of the proposed method are influenced by two arbitrary parameters: number and location of the artificial points added to the two extreme layouts of the observed points on the 3-year confidence bands. The location of the points seems to be most significant for the width of the reduced uncertainty bands with respect to their number. It is possible that adjustment of localization of the artificial points for a specific chosen model of ascospore maturation could lead to the improvement of the method’s effectiveness.
It should be taken into consideration that the proposed method was appraised using solely field data of the season’s cumulative proportion of trapped ascospores. The precision and correctness of the updated model’s uncertainties could be higher if the field data were supplemented with additional information about the development of ascospores in the season, resulting from laboratory analysis, which could lead to a direct assessment of the stage of ascospore maturity. In conclusion, the proposed method may be considered an effective approach to a more precise description of V. inaequalis ascospore maturation.
Supplementary material
Supplemental data for this article can be accessed here: http://dx.doi.org/10.1080/07060661.2014.969314
Supplemental Figure Legends
Download MS Word (38 KB)Supplemental Figure 12
Download TIFF Image (304.8 KB)Supplemental Figure 11
Download TIFF Image (310.1 KB)Supplemental Figure 10
Download TIFF Image (300.4 KB)Supplemental Figure 9
Download TIFF Image (321.3 KB)Supplemental Figure 8
Download TIFF Image (307.5 KB)Supplemental Figure 7
Download TIFF Image (318.8 KB)Supplemental Figure 6
Download TIFF Image (309 KB)Supplemental Figure 5
Download TIFF Image (314.5 KB)Supplemental Figure 4
Download TIFF Image (305.5 KB)Supplemental Figure 3
Download TIFF Image (319.8 KB)Supplemental Figure 2
Download TIFF Image (308.3 KB)Supplemental Figure 1
Download TIFF Image (313.1 KB)Supplemental Animation 6
Download Microsoft Video (AVI) (11.3 MB)Supplemental Animation 5
Download Microsoft Video (AVI) (14.6 MB)Supplemental Animation 4
Download Microsoft Video (AVI) (11.3 MB)Supplemental Animation 3
Download Microsoft Video (AVI) (14.6 MB)Supplemental Animation 2
Download Microsoft Video (AVI) (11.3 MB)Supplemental Animation 1
Download Microsoft Video (AVI) (14.6 MB)Acknowledgements
We thank Kris Pruski, Department of Plant and Animal Sciences, Dalhousie University, Canada for comprehensive revision of the manuscript.
References
- Blazejczyk K. 2006. Climate and bioclimate of Poland. In: Degórski M, editor. Natural and human environment of Poland. A geographical overview. Warsaw: Polish Academy of Sciences, Inst. of Geography and Spatial Organization, Polish Geographical Society; p. 31–48.
- Commission Implementing Regulation (EU) No 981/2011 of 30 September 2011 entering a name in the register of protected designations of origin and protected geographical indications [Jabłka grójeckie (PGI)]. 2011. Official Journal of the European Union. 5.10.2011. L 260/1-2.
- Cooley DR, Manning WJ. 1995. Estimating the risks and benefits of pesticides: considering the agroecosystem and integrated pest management in the use of EBDC fungicides on apples. Environ Pollut. 88:315–320.
- Council Regulation (EC) No 510/2006‘Jabłka Grójeckie’ EC No: PL-PGI-0005-0730-01.12.2008 PGI (X) PDO (). 2010. Official Journal of the European Union. 27.11.2010. C 322/35.
- Cox KD, Russo NL, Villani SM, Parker DM, Köller W. 2008. QoI qualitative resistance and CYP51A1 upstream anomalies in NY populations of the apple scab pathogen Venturia inaequalis. Phytopathology. 98:S42.
- Culliney TW, Pimentel D, Pimentel MH. 1992. Pesticides and natural toxicants in food. Agric Ecosyst Environ. 41:297–320.
- Esposito F, Seifritz E, Formisano E, Morrone R, Scarabino T, Tedeschi G, Cirillo S, Goebel R, Di Sallee F. 2003. Real-time independent component analysis of fMRI time-series. NeuroImage. 20:2209–2224. doi:10.1016/j.neuroimage.2003.08.012
- Fox J. 2002. Nonlinear regression and nonlinear least squares. Appendix to an R and S-PLUS companion to applied regression. Thousand Oaks, CA: Sage Publication Inc..
- Gadoury DM, MacHardy We. 1982. A model to estimate the maturity of ascospores of Venturia inaequalis. Phytopathology. 72:901–904. doi:10.1094/Phyto-72-901
- Gadoury DM, Seem RC, MacHardy E, MacHardy WE, Rosenberger DA, Stensvand A. 2004. A comparison of methods used to estimate the maturity and release of ascospores of Venturia inaequalis. Plant Dis. 88:869–874. doi:10.1094/PDIS.2004.88.8.869
- Gembris D, Taylor JG, Schor S, Frings W, Suter D, Posse S. 2000. Functional magnetic resonance imaging in real time (FIRE): Sliding-window correlation analysis and reference-vector optimization. Magn Reson Med. 43:259–268. doi:10.1002/(SICI)1522-2594(200002)43:2<259::AID-MRM13>3.0.CO;2-P
- Hawkins DM. 2004. The problem of overfitting. J Chem Inf Comput Sci. 44:1–12. doi:10.1021/ci0342472
- Holb IJ. 2009. Fungal disease management in organic apple orchards: epidemiological aspects and management approaches. In: Gisi U, Chet I, Gullino ML, editors, Proceedings of the 9th International Congress. Plant pathology in the 21st century: recent developments in management of plant diseases. Netherlands: Springer; p. 163–177.
- James JR, Sutton TB. 1982a. Environmental factors influencing pseudothecial development and ascospore maturation of Venturia inaequalis. Phytopathology. 72:1073–1080. doi:10.1094/Phyto-72-1073
- James JR, Sutton TB. 1982b. A model for predicting ascospore maturation of Venturia inaequalis. Phytopathology. 72:1081–1085.
- Juroszek P, Von Tiedemann A. 2011. Potential strategies and future requirements for plant disease management under a changing climate. Plant Pathol. 60:100–112. doi:10.1111/j.1365-3059.2010.02410.x
- Kożuchowski K, Degirmendžić J. 2005. Contemporary changes of climate in Poland: trends and variation in thermal and solar conditions related to plant vegetation. Pol J Ecol. 53:283–297.
- MacHardy WE. 1996. Apple scab biology, epidemiology and management. St. Paul, MN: The American Phytopathological Society.
- MacHardy WE, Gadoury DM. 1985. Forecasting the seasonal maturation of ascospores of Venturia inaequalis. Phytopathology. 75:381–385. doi:10.1094/Phyto-75-381
- Massie LB, Szkolnik M. 1974. Prediction of ascospore maturity of Venturia inaequalis utilising cumulative degree-days. Proceedings of the American Phytopathological Society. 1: 140. (abstr.).
- Meszka B, Broniarek-Niemiec A, Bielenin A. 2008. The status of dodine resistance of Venturia inaequalis populations in Poland. Phytopathol Pol. 47:57–61.
- Padder BA, Shah MD, Ahmad M, Sofi TA, Ahanger FA, Hamid A. 2011. Genetic differentiation among populations of Venturia inaequalis in Kashmir: a North-Western State of India. Asian J Plant Pathol. 5:75–83. doi:10.3923/ajppaj.2011.75.83
- Pautasso M, Döring TF, Garbelotto M, Pellis L, Jeger MJ. 2012. Impacts of climate change on plant diseases-opinions and trends. Eur J Plant Pathol. 133:295–313. doi:10.1007/s10658-012-9936-1
- R Development Core Team. 2009. R: A language and environment for statistical computing. Vienna, Austria: R Foundation for Statistical Computing. ISBN 3-900051-07-0. [Internet] Available from: http://www.R-project.org.
- Richardson LF. 2007. Weather prediction by numerical process. 2nd ed. New York, USA: Cambridge University Press.
- Rossi V, Giosuè S, Bugiani R. 2007. A-scab (Apple-scab), a simulation model for estimating risk of Venturia inaequalis primary infections. EPPO Bulletin. 37:300–308. doi:10.1111/j.1365-2338.2007.01125.x
- Rossi V, Ponti I, Marinelli M, Giosuè S, Bugiani R. 1999. Field evaluation of some models estimating the seasonal pattern of airborne ascospores of Venturia inaequalis. J Phytopathol. 147:567–575. doi:10.1046/j.1439-0434.1999.00436.x
- Rossi V, Ponti I, Marinelli M, Giosuè S, Bugiani R. 2000. A new model estimating the seasonal pattern of air-borne ascospores of Venturia inaequalis (Cooke) Wint. in relation to weather conditions. J Plant Pathol. 82:111–118.
- St-Arnaud M, Neumann P. 1990. Évaluation au Québec d’un modèle de prédiction de la fin de la période annuelle d’éjection des ascospores du Venturia inaequalis.. Phytoprotection. 71:17–23. doi:10.7202/705978ar
- Stensvand A, Eikemo H, Gadoury DM, Seem RC. 2005. Use of a rainfall frequency threshold to adjust a degree-day model of ascospore maturity of Venturia inaequalis. Plant Dis. 89:198–202. doi:10.1094/PD-89-0198
- Stensvand A, Gadoury DM, Amundsen T, Semb L, Seem, RC. 1997. Ascospore release and infection of apple leaves by conidia and ascospores of Venturia inaequalis at low temperatures. Phytopathology. 87:1046–1053. doi:10.1094/PHYTO.1997.87.10.1046
- Stensvand A, Gadoury DM, Seem RC, Amundsen T, Falk SP. 1998. Some recent advances in epidemiology of apple scab. Proceedings of the 7th International Congress of Plant Pathology, Edinburgh, Scotland (abstract).
- Stukenbrock EH. Bataillon T. 2012. A population genomics perspective on the emergence and adaptation of new plant pathogens in agro-ecosystems. PLoS Pathog. 8:e1002893. doi:10.1371/journal.ppat.1002893
- Tenzer I, Gessler C. 1999. Genetic diversity of Venturia inaequalis across Europe. Eur J Plant Pathol. 105:545–552. doi:10.1023/A:1008775900736
- Trapman MC. 1993. Control of scab on the basis of RIM. De Fruitteelt. 50: 31–34. (in Dutch).
- Viljanen-Rollinson SLH, Thompson SM, Keenan S, Bulman SR, Wright PJ, Wood PN, Park NM, Beresford RM. 2013. Resistance of Venturia inaequalis to quinone outside inhibitor (QoI) fungicides in New Zealand apple orchards. N Z Plant Prot. 66:284–292.
- Wang Q-Y, Zhou D-M, Cang L. 2009. Microbial and enzyme properties of apple orchard soil as affected by long-term application of copper fungicide. Soil Biol Biochem. 41:1504–1509. doi:10.1016/j.soilbio.2009.04.010
- Zhang T, Yue D, Gu Y, Wang Y, Yu G. 2009. Adaptive correlation analysis in stream time series with sliding windows. Comput Math Appl. 57:937–948. doi:10.1016/j.camwa.2008.10.083