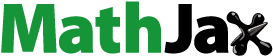
Abstract
Purpose
Our goal was to identify discrete clinical characteristics associated with safe discharge from an emergency department/urgent care for patients with a history of cancer and concurrent COVID-19 infection during the SARS-CoV-2 pandemic and prior to widespread vaccination.
Patients and Methods
We retrospectively analyzed 255 adult patients with a history of cancer who presented to Memorial Sloan Kettering Cancer Center (MSKCC) urgent care center (UCC) from March 1, 2020 to May 31, 2020 with concurrent COVID-19 infection. We evaluated associations between patient characteristics and 30-day mortality from initial emergency department (ED) or urgent care center (UCC) visit and the absence of a severe event within 30 days. External validation was performed on a retrospective data from 29 patients followed at Fred Hutchinson Cancer Research Center that presented to the local emergency department. A late cohort of 108 additional patients at MSKCC from June 1, 2020 to January 31, 2021 was utilized for further validation.
Results
In the MSKCC cohort, 30-day mortality and severe event rate was 15% and 32% respectively. Using stepwise regression analysis, elevated BUN and glucose, anemia, and tachypnea were selected as the main predictors of 30-day mortality. Conversely, normal albumin, BUN, calcium, and glucose, neutrophil–lymphocyte ratio <3, lack of (severe) hypoxia, lack of bradycardia or tachypnea, and negative imaging were selected as the main predictors of an uneventful course as defined as a Lack Of a Severe Event within Thirty Days (LOSETD). Utilizing this information, we devised a tool to predict 30-day mortality and LOSETD which achieved an area under the operating curve (AUC) of 79% and 74% respectively. Similar estimates of AUC were obtained in an external validation cohort. A late cohort at MSKCC was consistent with the prior, albeit with a lower AUC
Conclusion
We identified easily obtainable variables that predict 30-day mortality and the absence of a severe event for patients with a history of cancer and concurrent COVID-19. This has been translated into a bedside tool that the clinician may utilize to assist disposition of this group of patients from the emergency department or urgent care setting.
Introduction
COVID-19, the disease caused by severe acute respiratory syndrome coronavirus 2 (SARS-CoV-2), has affected over 170 million people, causing over 3.7 million deaths across 190 countries as of June 4, 2021 (Johns Hopkins Coronavirus Resource Center) (Citation1). Despite decreased emergency department volume, the severity of this illness resulted in increased hospital admission rates of up to 149.0% in New York City, during the early stages of the pandemic (Citation1a). Oncological patients with concurrent COVID-19 illness pose a unique challenge due to the increased risk of adverse events (Citation2) and a subsequent high rate of admission (Citation3). While there were a number of studies describing risk factors associated with adverse outcomes of COVID-19 in the general population (Citation4–7) and in the cancer patient population, (Citation3, Citation8–11) there was a lack of data on risk stratification, disposition planning, and follow-up for cancer patients with COVID-19 presenting in an emergency department or urgent care setting. Our goal was to identify discrete clinical characteristics that were associated with safe discharge from the emergency department or urgent care in patients with a history of cancer and concurrent COVID-19 during the early stages of the pandemic.
Patients and methods
Study design and setting
This was a retrospective observational study in adult cancer patients with concurrent COVID-19 and emergency department disposition at Memorial Sloan Kettering Cancer Center (MSKCC). MSKCC is a tertiary cancer center with a dedicated urgent care center (UCC) specializing in cancer-related emergencies located in New York City, New York. Approximately 23,000 patients per year are managed at MSKCC. In 2019, the UCC had a total of 24,740 unique patient visits, with an average admission rate of 63%. MSKCC’s institutional review board approved this study and waived the need for informed consent.
Patient selection
From March 1 to May 31, 2020, all adult (age >18 years) patients with a history of malignancy who presented to the UCC at MSKCC and whose laboratory analysis confirmed SARS-CoV2 infection within 24 hours after UCC presentation were included in the initial cohort. The late cohort extended this group to include patients from June 1 through January 2021. Patients with only a radiological or clinical diagnosis of COVID-19 were excluded from the study. Patients receiving SARS-CoV2 surveillance swabs prior to procedures, chemotherapy, or part of a proactive surveillance program were excluded. De-identified data for 29 adult patients with a cancer diagnosis being followed at Fred Hutchinson Cancer Research Center, Seattle, WA, who presented to the Emergency Department at University of Washington for COVID-19 were collected and used for external validation. Details on SARS-CoV2 testing can be found in the Supplemental Materials.
Data collection
Patient demographic data, laboratory results, anticancer treatment, and disposition from the UCC were automatically obtained from the electronic medical record (EMR). Clinical data, including baseline comorbidities, presenting symptoms, and outcomes, were obtained by chart review. “Basic” metabolic panel includes blood urea nitrogen (BUN), calcium, carbon dioxide, chloride, creatinine, glucose, potassium, and sodium. “Complete” metabolic panel includes all components of the basic metabolic panel but additionally measures albumin, total protein, alkaline phosphatase, alanine amino transferase (ALT or SGPT), aspartate amino transferase (AST or SGOT), and bilirubin. Chest radiography, including chest X-rays and chest CTs, obtained during the UCC visit were independently reviewed by two radiologists and categorized into “unilateral opacity,” “bilateral opacity,” “no changes.” Patients with extensive preexisting lung disease were labelled as “inconclusive.” Severe event includes acceptance to Intensive Care Unit (ICU), mechanical ventilation, or death.
For some domains, institutional data were extracted from either raw institutional data warehouses (vitals and labs from UCC visit), and a subset of MSKCC data (medications) conformed to the Observational Medical Outcomes Partnership common data model (OMOP CDM) using a quality-controlled pipeline. Specifically, using custom scripts data were loaded into an attribute-entity-value “observation table” extended from the observation table in the OMOP CDM by adding columns to capture uniform resource identifiers (URIs) for standardized concepts and reference ranges for labs. A Tableau dashboard operating on a HIPAA-compliant MSK server presented data elements.
Statistical analysis
This study evaluated the relationship between patients’ characteristics on the risk of experiencing a severe event, as defined the presence of any of the following: ICU level care, need for mechanical ventilation, or death within 30 days from the first UCC visit. The second outcome of interest was a death within 30 days from the first UCC visit. MSKCC started using investigational therapies (tocilizumab, remdesivir, hydroxychloroquine, steroids, azithromycin, convalescent plasma) and proning techniques as the pandemic progressed; we stratified by month to account for this. We evaluated associations between demographic, clinical, laboratory, and vital data from the first UCC visit and each outcome of interest by conducting the Cochran–Mantel–Haenszel test, stratifying by month of COVID-19 diagnosis (March vs. April/May). We performed stepwise logistic regression criterion Akaike information criterion (AIC) selection criterion to determine important predictors for outcome from a set of patient characteristics with p-values <0.05 in univariate analysis, adjusting for the month of COVID-19 diagnosis. Final models were selected by Akaike information criterion (AIC) criteria. Missing values for each laboratory and vital characteristic were imputed by sample means. Missing radiology measurements were assigned to be negative. We defined the multiplicative factor for the patient as
where
are selected characteristics by final models, and scores
are estimated log-odds ratios for each outcome of interest that are rounded to nearest integer. The ratio of multiplicative factor
calculated for risk factors
to a multiplicative factor
calculated for risk factors
represents the change in odds of experiencing an event by a patient
compared to a patient
Log-values of
called risk scores,
were calculated for each patient using final model for each outcome. Probability of safe discharge (or death with 30 days) were calculated by
for each risk score
with intercepts
estimated in the final model. The average area under the receiver operating characteristic curve (AUC) of the final predictive models were estimated by 5-fold cross-validation. In each round of cross-validation, we applied the same two-step approach to a training data and estimated AUC on testing data while adjusting for the month of COVID-19 diagnosis (Citation12). We validated our final risk scores,
on a sample of 29 patients from University of Washington Emergency Department; AUCs were estimated for each outcome of interest by calculating risk scores for each patient with scoring system in and Supplemental Tables 6, 7. All statistical analyses were performed using R programming language (Citation13).
Results
Our initial cohort consisted of 255 patients who presented to the MSKCC UCC from March–May 2020 and had a positive SARS-CoV-2 respiratory swab within 24 hours after their first UCC visit (). Of these 255 patients, 51% were >65 years of age, 53% were female, and 56% were white. Cancer subtypes included hematological (26%), lung (11%), and other solid malignancies (62%). Eight-one patients suffered a severe event (32%), of which 60/255 died (24%). Approximately 58% (153/255) of patients had at least one cardiac comorbidity; 16% (41/255) had pulmonary disease; and 84/255 (33%) had diabetes.
Table 1. Patient demographic and clinical characteristics (MSKCC cohort, n = 255).
The majority of patients (207/255, 81%) were admitted, and 183/207 (88%) of those hospitalized were admitted or placed under observation in a clinical decision unit (CDU) at the initial UCC visit. Of the 72/255 (28%) patients discharged from their first UCC visit, 30/72 (42%) returned to the MSKCC UCC, and 24/72 (33%) of these were admitted or placed in CDU. Sixteen of 24 (66%) were admitted within one week of initial presentation, and 42/72 (58%) patients were successfully discharged (i.e., no revisits or admissions outside of MSKCC). Of those returning after UCC discharge (n = 30), five suffered a severe event within 30 days, four at MSKCC and one at an outside hospital with one death within 30 days of initial UCC visit (Supplemental Table 1).
Looking at the initial cohort data, 38/255 (15%) patients died within 30 days. There was increased rate of mortality and severe events March 2020 as compared to April/May 2020 (Supplemental Tables 2 and 3) and the late cohort. MSKCC started performing surveillance swabs on all patients presenting to the hospital irrespective of symptoms starting March 26, 2020.
Univariate analysis demonstrated an increase in 30-day mortality for lung cancer patients compared to those with hematological and other solid tumors. Those with any cardiac risk factors, radiological changes, hypoalbuminemia, hypoxia, tachypnea, or elevation in troponin were strongly associated with death (Supplemental Table 4). Dyspnea, hypoxia, tachycardia, positive or inconclusive radiological findings, hypoalbuminemia, neutrophil-to-lymphocyte ratio >3, hypocalcemia, elevated troponin, and uremia were significantly associated with severe events (Supplemental Table 5).
In the final multivariable model selected by stepwise regression, the following variables in the complete metabolic panel (CMP) were selected as most important predictors of death within 30 days: hypoalbuminemia, uremia, hyperglycemia, anemia, tachypnea, and presence of radiological findings (). We next sought to identify predictors of lack of a severe event in thirty day (LOSETD). Higher oxygen saturation, normoglycemia, normal heart rate, and negative chest imaging were associated with a LOSETD (). Using these models, we calculated patients’ risk scores and assessed the 30-day mortality and LOSETD (,b)). We repeated stepwise regression utilizing only the basic metabolic panel (BMP) for both outcomes as seen on Supplemental Tables 6 and 7.
Table 2. Predictors for death within 30 days selected by stepwise logistic regression from demographic, clinical, complete lab panel and vital sign measurements.
Table 3. Predictors Lack of Severe Event within Thirty Days (LOSETD) selected by stepwise logistic regression from demographic, clinical, complete lab panel and vital sign measurements.
Figure 1. Distribution of risk scores in MSKCC cohort (n = 255). (a) Distribution of risk scores for mortality within 30 days in patients admitted at first UCC visit (left) and discharged from initial visit (middle), and probability of an outcome based on risk score (right). (b) Distribution of risk scores for LOSETD in patients admitted at first UCC visit (left) and discharged from initial visit (middle), and probability of an outcome based on risk score (right). Red curves: estimated baseline risk in March; blue curves: estimated baseline risk in April/May.
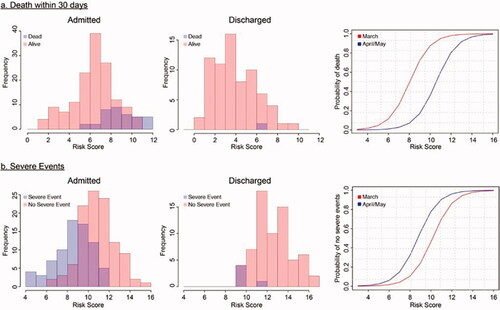
To assess our scoring system, receiving operating curves (ROC) were used to predict accuracy. Utilizing the CMP, an AUC of 79%, and 74% for 30-day mortality and LOSETD, respectively () was achieved. The narrower BMP attained an AUC of 79% and 71% for respective outcomes as seen in Supplement . External validation utilizing CMP with University of Washington Emergency Department cohort exhibited an AUC for 30-day mortality and LOSETD of 77% and 73%, respectively (). In the late MSKCC cohort, AUC with CMP was 71% and 66%, respectively.
Figure 2. Receiving operating curve (ROC) for predicting (a) mortality within 30 days (b) LOSETD based on demographics, clinical characteristics, laboratory and imaging studies in the MSKCC cohort and a validation cohort. Estimated ROC curves in the validation cohort were based on the multivariate model in and . For left panels, blue curves: ROC at each replication for 5- fold cross validation; red curves: are average ROC curves.
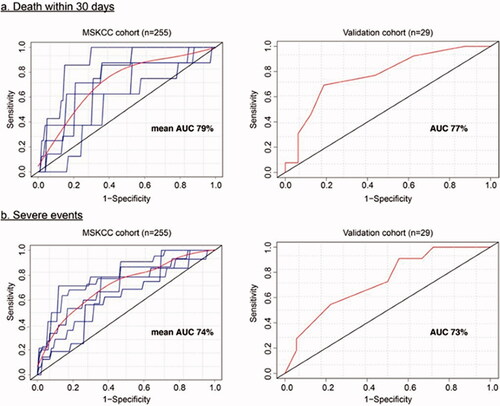
Discussion
As COVID-19 persists worldwide, it is important to utilize risk stratification tools for patients with acute infections. The ability to reliably predict patients that can be safely discharged from the emergency room setting would profoundly impact bed utilization and resource allocation. Such prediction is especially challenging in patients with significant co-morbidities and/or a higher baseline risk of critical illness, such as cancer patients. Here, we provide a retrospective analysis of 255 patients with a history of cancer and acute COVID-19 diagnoses and identify demographic, comorbidity, and clinical features correlated with 30-day mortality and lack of severe events at thirty days. Using this information, we generated and validated a scoring system to predict these outcomes with high reliability.
Studies from both from our institution (Citation3) and others (Citation8) have reviewed the relationship between cytotoxic chemotherapy and COVID-19 outcome, have not found evidence of additional risk of severe outcomes in patients receiving active cytotoxic treatment therapy. We chose not to delineate patients according to their anticancer regimens, as emergency providers may not have access to detailed records.
Vaccination at Memorial Sloan Kettering was initiated approximately January 15th 2021 and our results may not reflect this subpopulation. Early studies in the USA and Europe demonstrate decreased responsiveness to available vaccines in the cancer population, particularly amongst hematological malignancies. Follow up amongst the vaccinated population is warranted (Citation17–19).
There were 72 discharges from initial UCC visit and one death within 30 days, marking a 1.4% mortality rate in the initial cohort and zero deaths in the late cohort. This supports the remarkable power of physician judgement in view of a novel challenge. Our clinical prediction tool should therefore be used to complement and not supplant clinical judgment with our objective criteria as outlined above.
It was noted that discharged patients had significantly decreased 30-day mortality and severe events rate, compared to admitted patients with similar scores on the complete panel. This association persisted throughout the follow-up period. The reasons are unclear. This may be related to variables not measured with our scoring tool. A second and intriguing alternative possibility exists that hospitalization alone may incur risk, potentially related to immobility while hospitalized, or administration of untested and unproven therapeutics and interventions. Further investigation of this sub-group is warranted.
We observed similar scores corresponding to different mortality rates depending on the month of COVID-19 diagnosis, with later presentation showing improved outcome. This is consistent with national trends (Johns Hopkins Coronavirus Resource Center et al) and may be related to better understanding of the disease process and therapeutics, further large-scale follow-up is needed. Additionally, we also had increased surveillance testing starting late March 2020 possibly capturing less severe or even asymptomatic cases of COVID-19, further skewing the results to favorable outcomes.
The variables identified in our cohort as conferring increased risk are not novel and have been studied individually and in combination with other factors previously. Hypoxia and increased respiratory rate, shown to increase the risk of severe outcome in COVID-19, (Citation7) was similarly predictive in our model. Elevated urea utilized in the validated CURB-65 pneumonia prediction tool and predictive of mortality in the COVID-19 population (Citation6) was also a significant marker for our cancer-specific group. Hypoalbuminemia, a strong predictor of death in our population, was similarly identified in a COVID-19 noncancer-specific cohort in China (Citation14). It is unclear if albumin supplementation would alter the course of disease. Anemia was found in our analysis to be associated with increased mortality, and similar to Mehta et al. (Citation11) in a NYC cancer-specific population, transfusion thresholds for COVID-19 warrant further investigation.
Current research into COVID-specific imaging, such as the Brixia score, (Citation15) may have utility in certain situations. We felt that a simpler normal versus abnormal determination of pneumonia on imaging, validated by our study, to be more useful for the urgent care or emergency provider. Additionally, our findings demonstrate that a more cumbersome CT scan may not be necessary. Although prior studies confer additional risk for pulmonary malignancy, (Citation11, Citation16) our data demonstrated that in the absence of parenchymal disease, the prognosis was similar to other cancer types.
Strengths of our study include a population within a closed network with comprehensive follow-up from sites that were initial epicenters of COVID-19 in the United States. Limitations of the study include its retrospective nature which is particularly relevant in a constantly evolving pandemic. The small sample size and unique demographics of our patient population may limit generalizability. Additionally, as the MSKCC population is spread over a large geographic region, we may have had a nonrepresentative sample (e.g., individuals that were more acutely ill may have chosen local emergency facilities), potentially altering the results. Moreover, our low severe event rate post discharge may be related to special institutional follow-up protocols, including daily nursing calls postdiagnosis, with clear instructions of when to return to the hospital. We included external validation which included a catchment area of local emergency departments to attempt to address these limitations. Indeed, the validation cohort had a higher 30-day mortality rate and severe event rate (38% and 55%, respectively) than the MSKCC cohort. Finally, although follow-up was considered complete, outside hospitalizations after discharge may not have been captured for every patient.
In summary, in a sample of cancer patients, we identified vital signs, labs, and imaging at presentation that were predictive of 30-day mortality and lack of a severe event. These findings have implications in triage and disposition planning in the urgent care and emergency settings. Prospective validation in cancer-specific and noncancer populations is necessary to determine broader utility.
Supplemental Material
Download PDF (512.3 KB)Acknowledgements
This study is dedicated to all the front-line hospital workers, doctors, nurses, aides, transport, technicians, and all others who have answered the call and fought bravely, for some the ultimate sacrifice.
Disclosure statement
Consulting or Advisory Role: G1 Therapeutics; Partners Healthcare, BeyondSpring; Sandoz; Amgen; ER Squibb (Inst); Merck; Jazz Pharm; Kallyope; TEVA; Seattle Genetics; Samsung; Spectrum.
Correction Statement
This article has been republished with minor changes. These changes do not impact the academic content of the article.
References
- Coronavirus 2019‐nCoV, CSSE. Coronavirus 2019‐nCoV Global Cases by Johns Hopkins CSSE. (https://gisanddata.maps.arcgis.com/apps/opsdashboard/index.html#/bda7594740fd40299423467b48e9ecf6. a.Jeffery MM, D'Onofrio G, Paek H, Platts-Mills TF, Soares WE, Hoppe JA, et al. Trends in emergency department visits and hospital admissions in health care systems in 5 states in the first months of the COVID-19 pandemic in the US. JAMA Intern Med. 2020;180(10):1328–1333.
- Liang W, Liang H, Ou L, Chen B, Chen A, Li C, China Medical Treatment Expert Group for COVID-19, et al. Development and validation of a clinical risk score to predict the occurrence of critical illness in hospitalized patients with COVID-19. JAMA Intern Med. 2020;180(8):1081–1089. doi:https://doi.org/10.1001/jamainternmed.2020.2033.
- Robilotti EV, Babady NE, Mead PA, Rolling T, Perez-Johnston R, Bernardes M, et al. Determinants of COVID-19 disease severity in patients with cancer. Nat Med. 2020;26(8):1218–1223. doi:https://doi.org/10.1038/s41591-020-0979-0.
- Richardson S, Hirsch JS, Narasimhan M, Crawford JM, McGinn T, Davidson KW, the Northwell COVID-19 Research Consortium, et al. Presenting characteristics, comorbidities, and outcomes among 5700 patients hospitalized with COVID-19 in the New York city area. JAMA. 2020;323(20):2052–2059. doi:https://doi.org/10.1001/jama.2020.6775.
- Petrilli CM, Jones SA, Yang J, Rajagopalan H, O’Donnell L, Chernyak Y, et al. Factors associated with hospital admission and critical illness among 5279 people with coronavirus disease 2019 in New York City: prospective cohort study. BMJ. 2020;369:m1966. doi:https://doi.org/10.1136/bmj.m1966.
- Knight SR, Ho A, Pius R, Buchan I, Carson G, Drake TM, ISARIC4C investigators, et al. Risk stratification of patients admitted to hospital with covid-19 using the ISARIC WHO Clinical Characterisation Protocol: development and validation of the 4C Mortality Score. BMJ. 2020;370:m3339 doi:https://doi.org/10.1136/bmj.m3339.
- Haimovich AD, Ravindra NG, Stoytchev S, Young HP, Wilson FP, van Dijk D, et al. Development and Validation of the Quick COVID-19 Severity Index: a prognostic tool for early clinical decompensation. Ann Emerg Med. 2020;76(4):442–453. doi:https://doi.org/10.1016/j.annemergmed.2020.07.022.
- Lee LY, Cazier J-B, Angelis V, Arnold R, Bisht V, Campton NA, et al. COVID-19 mortality in patients with cancer on chemotherapy or other anticancer treatments: a prospective cohort study. Lancet. 2020;395(10241):1919–1926. doi:https://doi.org/10.1016/S0140-6736(20)31173-9.
- Jee J, Foote MB, Lumish M, Stonestrom AJ, Wills B, Narendra V, et al. Chemotherapy and COVID-19 outcomes in patients with cancer. J Clin Oncol. 2020;38(30):3538–3546. doi:https://doi.org/10.1200/JCO.20.01307.
- Luo J, Rizvi H, Preeshagul IR, Egger JV, Hoyos D, Bandlamudi C, et al. COVID-19 in patients with lung cancer. Ann Oncol. 2020;31(10):1386–1396. doi:https://doi.org/10.1016/j.annonc.2020.06.007.
- Mehta V, Goel S, Kabarriti R, Cole D, Goldfinger M, Acuna-Villaorduna A, et al. Case fatality rate of cancer patients with COVID-19 in a New York hospital system. Cancer Discov. 2020;10(7):935–941. : doi:https://doi.org/10.1158/2159-8290.CD-20-0516.
- Janes H, Pepe MS. Adjusting for covariate effects on classification accuracy using the covariate-adjusted receiver operating characteristic curve. Biometrika. 2009;96(2):371–382. doi:https://doi.org/10.1093/biomet/asp002.
- R Development Core Team: R: a language and environment for statistical computing. Vienna (Austria), R Foundation for Statistical Computing, 2016.
- Huang J, Cheng A, Kumar R, Fang Y, Chen G, Zhu Y, et al. Hypoalbuminemia predicts the outcome of COVID-19 independent of age and co-morbidity. J Med Virol. 2020;92(10):2152–2158. doi:https://doi.org/10.1002/jmv.26003.
- Borghesi A, Maroldi R. COVID-19 outbreak in Italy: experimental chest X-ray scoring system for quantifying and monitoring disease progression. Radiol Med. 2020;125(5):509–513. doi:https://doi.org/10.1007/s11547-020-01200-3.
- Dai M, Liu D, Liu M, Zhou F, Li G, Chen Z, et al. Patients with cancer appear more vulnerable to SARS-CoV-2: a multicenter study during the COVID-19 outbreak. Cancer Discov. 2020;10(6):783–791. : doi:https://doi.org/10.1158/2159-8290.CD-20-0422.
- Agha M, Blake M, Chilleo C, Wells A, Haidar G. Suboptimal response to COVID-19 mRNA vaccines in hematologic malignancies patients. Preprint. medRxiv. 2021. doi:https://doi.org/10.1101/2021.04.06.21254949.
- Monin L, Laing AG, Muñoz-Ruiz M, McKenzie DR, Del Molino Del Barrio I, Alaguthurai T, et al. Safety and immunogenicity of one versus two doses of the COVID-19 vaccine BNT162b2 for patients with cancer: interim analysis of a prospective observational study. Lancet Oncol. 2021;22(6):765–778. doi:https://doi.org/10.1016/S1470-2045(21)00213-8.
- Palich R, Veyri M, Marot S, Vozy A, Gligorov J, Maingon P, Marcelin AG, et al. Weak immunogenicity after a single dose of SARS-CoV-2 mRNA vaccine in treated cancer patients. Ann Oncol. 2021;32(8):1051–1053. doi:https://doi.org/10.1016/j.annonc.2021.04.020. Epub 2021 Apr 29. PMID: 33932501; PMCID: PMC8081573