Abstract
The development of new drugs against Mycobacterium tuberculosis is an essential strategy for fighting drug resistance. Although 3-dehydroquinate dehydratase (MtDHQ) is known to be a highly relevant target for M. tuberculosis, current research shows new putative inhibitors of MtDHQ selected by a large-scale ensemble-docking strategy combining ligand- and target-based chemoinformatic methods to deep learning. Initial chemical library was reduced from 216 million to approximately 460 thousand after pharmacophore, toxicity and molecular weight filters. Final library was subjected to an ensemble-docking protocol in GOLD which selected the top 300 molecules (GHITS). GHITS displayed different structures and characteristics when compared to known inhibitors (KINH). GHITS were further screened by post-docking analysis in AMMOS2 and deep learning virtual screening in DeepPurpose. DeepPurpose predicted that a number of GHITS had comparable or better affinity for the target than KINH. The best molecule was selected by consensus ranking using GOLD, AMMOS2 and DeepPurpose scores. Molecular dynamics revealed that the top hit displayed consistent and stable binding to MtDHQ, making strong interactions with active-site loop residues. Results forward new putative inhibitors of MtDHQ and reinforce the potential application of artificial intelligence methods for drug design. This work represents the first step in the validation of these molecules as inhibitors of MtDHQ.
Graphical Abstract
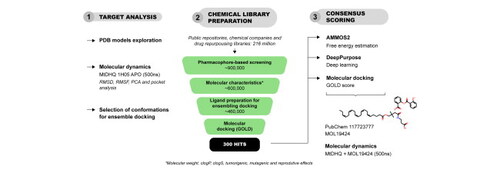
Communicated by Ramaswamy H. Sarma
Acknowledgments
Experiments presented in this paper were carried out using the Grid’5000 testbed, supported by a scientific interest group hosted by Inria and including CNRS, RENATER, and several Universities as well as other organizations (see https://www.grid5000.fr). Authors are also thankful towards CAPES, CNPq and Fundação Araucária.
Disclosure statement
No potential conflict of interest was reported by the authors.
Author contributions
Conceptualization, E.K., B.M. and R.C.; software, J.V.S., D.L., F.S. and B.M.; validation, E.K., B.M. and R.C.; formal analysis, J.V.S. and B.M.; investigation, J.V.S., E.K. and B.M.; writing–original draft preparation, J.V.S., L.M., R.C. and B.M.; writing–review and editing, J.V.S., L.M., D.L., F.S., E.K., B.M. and R.C.; supervision, E.K., B.M. and R.C. All authors have read and agreed to the published version of the manuscript.