Abstract
DNA methyltransferases (DNMTs) play an important role in the epigenetic regulation of gene expression through the methylation of DNA. Since hypermethylation and consequent suppression of tumor suppressor genes are associated with cancer development and progression, DNA hypomethylating agents (HMAs) such as DNMT inhibitors have been proposed for cancer therapy. Two nucleoside analogues approved for the treatment of hematological cancers, decitabine and azacytidine, have poor pharmacokinetic properties, and hence there is a critical need for identifying novel HMAs. Virtual screening of a library of ∼40,000 compounds from the ZINC database, followed by molecular docking of 4,000 compounds having potential druggable properties with DNMT1, DNMT3A and DNMT3B were performed. One unique inhibitor (ZINC167686681) was identified that successfully passed through the Lipinski Rule of 5, geometry constraints as well as ADME/Tox filters and having strong binding energy for DNMTs. Further, molecular dynamics simulations of the docked complexes showed detailed structural features critical for its binding with the DNMTs and the stability of their interaction. Our study identified a compound with potential druggable properties and predicted to bind and inhibit DNMTs. Further investigations involving cellular and animal models of ZINC167686681 will help in potentially taking it into clinical trials for the treatment of cancers.
Communicated by Ramaswamy H. Sarma
1. Introduction
DNA methylation is a process of the addition of methyl group at the fifth position of the pyrimidine ring of cytosine residues to generate 5-methylcytosine (5-mC), which commonly takes place at CpG sites in the genome (Kumar et al., Citation2018). In normal development, it is a heritable epigenetic process that regulates gene function (Moore et al., Citation2013). Though there are overlaps in the functions, DNA methyltransferase 1 (DNMT1) is an epigenetic regulator enzyme that has a primary role in maintaining methylation (Lyko, Citation2018). The epigenetic alteration of DNA cytosine residues by methylation plays an important role in controlling gene expression without changing the original DNA sequence (Gao & Das, Citation2014). During DNA methylation, the MTase domain transfers the adenosyl-l-methionine (SAM) methyl group (–CH3) to the 5′-cytosine position in the DNA sequence, resulting in 5-methylcytosine (5mC) and S-adenosyl-l-homocysteine (SAH) (Moore et al., Citation2013). DNMT3A and DNMT3B are de novo methyltransferases that are in charge of establishing DNA methylation patterns during embryogenesis and establishing genomic imprints throughout germ cell development (Zhang & Xu, Citation2017). DNMT3A primarily methylates a subset of genes and sequences in the late stage of embryonic development, particularly after birth, whereas DNMT3B alters a wide region of genomic sequences in early embryos (Zhang & Xu, Citation2017). More functional details of DNMTs can be found in this review (Lyko, Citation2018).
DNA methylation is dysregulated in human cancer, resulting in localized hypermethylation and subsequent transcriptional suppression of CpG island-associated gene promoters (Anwar & Lehmann, Citation2014; Jithesh et al., Citation2013). DNMT1 is overexpressed in many forms of malignancies, such as breast cancer and acute myeloid leukemia (AML) (Wong, Citation2021; Wong et al., Citation2019), which is linked to cellular transformation, whereas lower expression of DNMT1 appears to have a protective effect. Overexpression of DNMT3A and DNMT3B were identified in cancer tissues including triple negative breast cancer (TNBC) and AML (Jinawath et al., Citation2005; Roll et al., Citation2008). Furthermore, DNMT3A has been associated with transcriptional activation of DNMTs in many cancers (Jinawath et al., Citation2005).
Targeting DNMTs is a promising strategy for cancer treatment due to the reversibility of these epigenetic alterations. A few such DNMT inhibitors, either nucleoside analogues or non-nucleoside compounds have been identified, examples of which are shown in , and reviewed in detail elsewhere (Lopez et al., Citation2022). Decitabine (DAC) and azacytidine (AZA) are conventional DNA hypomethylating agents (HMAs) that have demonstrated therapeutic efficacy in the management of hematologic malignancies (Jones & Taylor, Citation1980). These nucleoside analogues are integrated into DNA where they covalently bind DNMT1, −3A, and –3B, causing dose-limiting toxicities and DNA damage (Agrawal et al., Citation2018). However, these drugs have poor pharmacokinetic characteristics and stability, which limit target engagement in peripheral tissues (Stresemann & Lyko, Citation2008). A DNA methylation inhibitor with improved pharmacological characteristics and reduced toxicity is required since these combined qualities may restrict the clinical potential of current DNMT inhibitors. Thus, research into finding better HMAs is currently active, with a recent report of the first-in-class reversible DNMT1-specific HMA (GSK3685032), which is in the preclinical stage (Pappalardi et al., Citation2021). We hypothesized that screening a large number of compounds and analyzing the interactions with the DNMTs in silico will reveal potential DNMT inhibitors that may be taken forward for further characterization. In this study, we screened 40,000 compounds from the ZINC database (https://zinc.docking.org/) followed by docking with DNMTs and molecular dynamics simulations of selected compounds, revealing the potential catalytic site binding characteristics of some of the compounds.
2. Methods
2.1. Preparation of the target proteins
The 3D structures of DNMT1, DNMT3A and DNMT3B were retrieved from the PDB (https://www.rcsb.org/), with IDs 4WXX (Zhang et al., Citation2015), 6W8B (Zhang et al., Citation2018), and 6U8V (Gao et al., Citation2020) respectively. Chimera 1.6.1 (https://www.rbvi.ucsf.edu/chimera) was employed for the refinement of the crystal structure of DNMTs through the energy minimization process by applying an amber force field while the geometry optimization was done by using VEGA ZZ (https://www.ddl.unimi.it). Molecular Operating Environment (MOE) was also utilized for the preparation of the structures before docking (Scholz et al., Citation2015).
2.2. Preparation of the ligand
We obtained the library of 40,000 compounds from the ZINC database (https://zinc.docking.org/) (Irwin & Shoichet, Citation2005; Sterling & Irwin, Citation2015). All compounds were downloaded in .sdf format. MONA 2.13 tool was employed to remove redundancy among these compounds. Open Babel was utilized for the conversion of the .sdf format to .pdb (Yoshikawa & Hutchison, Citation2019). The MOE software was used for optimization of all downloaded compounds by adding partial charges through the protonate3D module. For energy minimization, the MMFF94X force fields were applied for each compound and then added to the MOE ligands database for the purpose of docking (Scholz et al., Citation2015).
2.3. Virtual screening
In virtual screening, all ligands with poor drug-like properties were eliminated, and the remaining hit molecules were passed through a series of filters for a better selection of drug-like compounds. The Osiris Property Explorer Applet and Osiris Data Warrior were employed to explore the Lipinski filter and geometry constraints of the screened compounds. From this enrichment process, we filtered 4,000 compounds with well-designed geometric constraints, LogP value of 1–5, molecular weight 180–450 kDa, five or less rotatable bonds, <5 hydrogen bonds, and <10 hydrogen bond acceptors (HBAs). Moreover, SCIfinder was used to check whether our filtered hits were previously mentioned in the literature, and then search results were compiled and all those hits with >70% similarity index were evaluated.
2.4. Molecular docking
The catalytic residues of DNMT proteins were identified based on available reports (Horton et al., Citation2022; Zhang et al., Citation2015). Site finder tools were also used to find the potential active residues of DNMTs, and then electrostatic surface maps were created around these residues to define docking sites. The MOE tools (Vilar et al., Citation2008) were used to perform the docking of DNMTs with 4,000 compounds. A triangular algorithm was used to find the 10 best poses of docking molecules (Vilar et al., Citation2008). We applied London dg function and force fields refinement algorithms to create 10 best poses per molecule and then minimized them. These top 10 molecules were selected based on their binding energies for further interaction analysis with active residues of DNMTs.
2.5. Interaction analysis and ADME/tox properties
LigX tool of MOE was used to prepare the 2D plots of receptor-ligand interactions focused on electrostatic/non-electrostatic interactions, hydrogen bonding, and hydrophobic interactions. For complexes, these interactions assist in validating the binding pattern of the compounds within the active site of the protein (Vilar et al., Citation2008). For further visualization and interaction analysis, Pymol was used. The 3D structures of protein-ligand complexes were created through Pymol.
After the interaction analysis, the top two best interacting compounds were selected for ADME/Tox (Absorption, Distribution, Metabolism, Excretion, and Toxicity) analysis, which is important to find the possible fate of compounds after administration into the human body. Pk-CSM (Pires et al., Citation2015) was employed to check the ADME/Tox for the selected compounds and then SwissADME (Daina et al., Citation2017) and ADMETopt (Yang et al., Citation2018) were used to crosscheck the pharmacokinetics properties. Finally, the top best compound was selected for MD simulation studies passing the ADME/Tox filter as well as ‘Lipinski’s Rule of Five’ from the molecular docking studies against DNMTs.
2.6. Molecular dynamics simulations
Molecular dynamics (MD) simulations of the holo- and apo DNMT structures were performed using the NAMD 2.13 software (Phillips et al., Citation2005) and CHARMM36 force field (Huang et al., Citation2017). Topology and parameter files for the simulations were generated using CHARMM-GUI online server (Jo et al., Citation2008). The biomolecular simulation systems were generated by dissolving the 3D structural models in explicit solvent using TIP3P cubic water box (Jorgensen et al., Citation1983) with 10 Å minimum distance between edge of box and any of the atoms in the model. Charges were neutralized by adding 0.15 M NaCl to the solvated system. The biomolecular simulation systems consisted of about 272544, 128529 and 135781 atoms for DNMT1, DNMT3A, and DNMT3B, respectively. Preceding the production run, the biomolecular simulation systems were subjected to energy minimization and thermal equilibration with Periodic Boundary Conditions applied as described previously (Ahmed et al., Citation2021; Altamash et al., Citation2021; Geethakumari et al., Citation2022). Following equilibration, 100 ns production simulation runs were initiated. Timestep of integration was set up at 2 fs and trajectory frames were saved every 10,000 steps. A 12 Å cut-off with 10 Å switching distance was chosen to handle short-range non-bonded interactions, while long-range non-bonded electrostatic interactions were handled using Particle-mesh scheme at 1 Å PME grid spacing (Essmann et al., Citation1995; Feller et al., Citation1995; Steinbach & Brooks, Citation1994).
Analysis of MD trajectories was performed using the available tools in VMD (Humphrey et al., Citation1996). Backbone root-mean-square deviation (RMSD) measurements were performed using ‘RMSD-trajectory Tool’ in VMD. Calculating root-mean-square fluctuation (RMSF) was performed for residues’ Cα atoms. Radius of gyration (Rog), solvent accessible surface area (SASA), and centre-of-mass distance between protein and ligand were all performed using VMD. Hydrogen bond (H-bond) analysis was performed at a cut-off distance and A-D-H angle of 3.5 Å and 20°, respectively, using the ‘Hydrogen Bonds’ analysis plugin in VMD. Trajectory movies representing the 100 ns simulation runs were generated using VMD ‘Movie Maker’ extension and compiled using Fiji distribution of ImageJ software (Schindelin et al., Citation2012).
Ligand-binding free energy was estimated through the linear interaction energy (LIE) method [Aqvist, 1994 #5][Hansson, 1998 #6] using the CaFE 1.0 tool [Liu, 2016 #7] in VMD [Humphrey et al., Citation1996 #8]. Dynamic cross-correlation (DCC) analysis of Cα atoms of the three DNMTs in the absence or in the presence of the ligand was performed using the Bio3D package in R software (Feller et al., Citation1995; Steinbach & Brooks, Citation1994). Each trajectory was aligned using the Cα atoms prior to the analysis. The outcome of DCC analysis was represented as heatmap that range from +1 (high correlation, red) to 0 (no correlation, white) to −1 (high anti-correlation, blue).
3. Results
3.1. Virtual screening and molecular docking studies
The current study was conducted to perform a high-throughput integrative computational analysis to identify the most promising drug candidate against DNMTs. shows the overall workflow employed and the criteria used for selection of compounds and the results at each stage in this study.
Figure 2. Overall workflow showing the criteria used for screening and the outcomes at various stages.
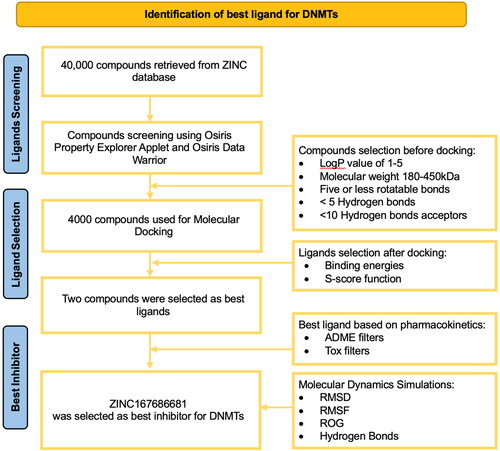
A dataset of 40,000 drug-like compounds were retrieved from the ZINC database (Irwin & Shoichet, Citation2005; Sterling & Irwin, Citation2015), and virtual screening was performed. The top 4,000 compounds were shortlisted based on geometry constraints and Lipinski Rule of 5 for further studies. To investigate the interactions of lead compounds with the active residues of DNMTs, these compounds were subjected to molecular docking analysis against the catalytic pocket of DNMTs. This analysis resulted in two best-docked compounds (ZINC167686681 and ZINC201939708) with strong binding energy (higher than −10 k cal/mol) towards the active site pocket of DNMTs ().
Table 1. Binding energies of the top two shortlisted compounds.
Of all the compounds screened, ZINC167686681 was found to be on top of the list exhibiting best binding energy and potential inhibitory activity against targets DNMT1, DNMT3A, and DNMT3B () and hence described here in detail. Molecular docking revealed that the lead inhibitor was bound with the active site of the DNMTs (). Comparing the calculated bond lengths between ZINC167686681 and binding residues from the targeted receptors (DNMT1, DNMT3A, and DNMT3B) binding site, it was identified that ligand binding was facilitated by hydrogen bond formation (with a cut-off distance of less than 3.5 Å). Interaction in the ZINC167686681-DNMT1 complex had the maximum number of the hydrogen bond propensities. Both the carbonyl groups of an oxane-3,4-dione moiety of ZINC167686681 were found to be engaged by three hydrogen bonds with C1226 and Q1227 residues at distances 2.2, 3.4 and 2.3 Å, respectively. Similarly, the hydroxyl groups of the benzene-1,3-diol region were connected by five hydrogen bonds with the key residues N1267, V1268, R1310 and R1312 at a distance measured 2.8, 3.3, 2.6, 2.7 and 2.6 Å, respectively. Furthermore, the interaction of ZINC167686681 bound with DNMT3A involved five hydrogen bonds in which the oxane-3,4-dione moiety was engaged by R891 at close vicinity of carbonyl with a bond length of 2.4 Å each. Interestingly, the key residues T645, L888, and S892 located in the close vicinity of the hydroxyl groups of benzene-1,3-diol region was engaged by three hydrogen bonds at a distance of 2.8, 3.1 and 2.2 Å, respectively. Moreover, the binding interaction mode of ZINC167686681 in complex with DNMT3B was through two hydrogen bonds with L829 and C651 residues (distances: 2.9 and 2.8 Å, respectively) facilitated with hydroxyl of benzene-1,3-diol and oxygen of methoxycyclohexane. Regarding the protein-ligand complex formed with ZINC167686681, the ligand was engaged with partially negatively charged groups (carbonyl and hydroxyl groups) at oxane-3,4-dione and benzene-1,3-diol moieties. Thus, the formation of hydrogen bonds with the lead inhibitor was identified in the molecular docking, with hydrogen bonds formed most strongly with DNMT1 amongst the 3 DNMTs studied here.
3.2. Physicochemical and pharmacokinetic properties
To better understand the clinical use of the two ligands, we employed pkCSM, ADMET-SAR and SwissADME to characterize the physicochemical and pharmacokinetic properties. ZINC167686681 had a favorable pharmacokinetic profile. The molecule has a weight of 300.266 g/mol, LogP close to 2 and molecular refractivity index of 76.24 (). Moreover, its intestinal absorption is high and is a substrate of P-glycoprotein. Furthermore, it cannot cross the central nervous system (CNS) barrier (), and no hepato- or Ames- toxicities were observed.
Table 2. Molecular properties of the final screening hit (ZINC167686681).
Table 3. Pharmacokinetic/toxicity profiles and drug-likeness of the final screening hit (ZINC167686681).
The compound with the second-best binding energies (), ZINC201939708, was found to bind to the active site of DNMTs in our molecular docking studies. Based on the measured bond lengths (with a cut-off distance of less than 3.5 Å) between ZINC201939708 and the active site residues in the receptor (DNMT1, DNMT3A, and DNMT3B) binding sites, binding of the compound was found to be facilitated by hydrogen bond interactions ( in Appendix). For DNMT1, we found that residues F1229, K1275, K1242 and Q573 formed hydrogen bonds with the compound and had a docking score of −11.3841 kcal/mol. For DNMT3A, the residues R790, R792, V758 and W893 formed hydrogen bonds with a docking score of −12.8998 kcal/mol ( in Appendix). Similarly, DNMT3B showed good binding interactions with ZINC201939708 with a docking score of −12.8384 kcal/mol (). However, ZINC201939708 had a less favorable pharmacokinetic profile ( in Appendix). The molecule has a weight of 397.42 g/mol and molecular refractivity index of 97.87. Moreover, its intestinal absorption is very low and is a substrate of P-glycoprotein efflux transporter. Furthermore, it can cross the central nervous system (CNS) barrier, though no hepato- or Ames- toxicities were observed. ZINC201939708 also violated some drug-likeness rules like Ghose, Veber, Muegge, and Egan. Hence, we did not further explore ZINC201939708, but took forward the compound with better overall druggable qualities, ZINC167686681, for further analysis using molecular dynamics simulations to understand the stability of binding interactions with the DNMTs.
3.3. Molecular dynamic simulation analysis
After ADME/Tox analysis, we performed molecular dynamics (MD) simulation to study the stability of the ligand-protein complex formed by ZINC167686681 binding to the three DNMTs (). All atom, explicit solvent MD simulations were performed for a total of 100 ns using NAMD for each ligand-DNMT complex (holo). Additionally, we performed the simulations of the three proteins alone (apo) for the purpose of comparison. Root-mean-square deviation (RMSD) analysis of Cα of the proteins revealed more dynamic changes in both the apo and holo forms of DNMT1 compared to DNMT3A and DNMT3B, while DNMT3A showed a higher RMSD value compared to DNMT3B (). All structures reached an equilibrium RMSD value before 100 ns, suggesting that 100 ns is sufficient for analyzing the trajectories.
Figure 4. Stable interaction of the inhibitor with DNMTs. (A) Composite image of snapshots representing 100 ns of MD simulation, taken 10 ns apart. (B–E) Graph showing Cα root mean square deviation (RMSD) (B), Cα root mean square fluctuation (RMSF) (C), radius of gyration (Rog) (D), solvent accessible surface area (SASA) (E) analyses of apo- and holo-DNMTs over the 100 ns MD simulations. (F–H) Graph showing ligand SASA (F), protein-ligand center-of-mass distance (G) and number of protein-ligand H-bonds (H) in holo-DNMTs over the 100 ns MD simulations.
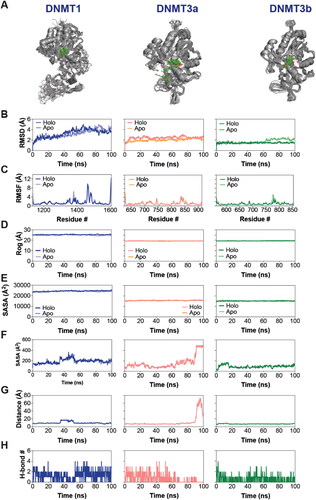
We then analyzed the root-mean-square fluctuation (RMSF) of Cα atoms, which revealed a region of high dynamic fluctuation in all DNMTs (aa 1450 − 1500 in DNMT1) with an enhanced fluctuation in DNMT1 in this region of the protein (). We also evaluated the overall shape of the proteins in the absence and in the presence of the ligand by measuring the radius of gyration (Rog) and solvent accessible surface area (SASA). This analysis revealed a relatively higher Rog and SASA for DNMT1 compared to DNMT3A and DNMT3B, with the latter two showing similar Rog and protein SASA profiles (). More importantly, there was no observable difference in RMSD, RMSF, Rog, and protein SASA between the apo and holo structure for the most part of the simulation, suggesting that ligand binding did not cause any discernable change in the protein dynamics. Calculation of ligand SASA in the DNMT-ligand complexes showed that the ligand was most buried in the DNMT3B binding pocket (). We note that while there was an initial surge in the ligand SASA values, the value returned to basal and was maintained throughout the simulation time in the case of the DNMT3B complex. Ligand SASA in DNMT1 complex showed a brief surge between ∼35 and ∼55 ns and then returned to basal value. On the other hand, ligand SASA values increased dramatically in DNMT3A at about 87 ns and reached the maximum value of ∼500 Å2 at ∼93 ns (). Calculation of the center-of-mass distances between the ligand and the proteins showed that the ligand leaves the binding site of DNMT3A but returns to interact with the protein at the surface (). Importantly, the distance was maintained throughout the simulation run in the case of DNMT3B complex while the DNMT1 complex showed some increase in the middle of the run. Finally, we analyzed the hydrogen bonding between the protein and the ligand in the three complexes which revealed a generally higher number of H-bonds in the DNMT1 and DNMT3A complexes compared to DNMT3B (), which is consistent with the docking results. Importantly, we found a sustained H-bond between the ligand and the Glu1266 side chain in the case of DNMT1 with an occupancy of 60.88% ( in Appendix). Such a highly sustained H-bond (with the Glu1266 residue) was not observed in the case of either DNMT3A or 3B although residues Thr645 in DNMT3A and Asp582 in DNMT3B showed significantly sustained H-bond with the ligand with occupancies of 21.16 and 21.12% ( in Appendix). Overall, it appears that the ligand, ZINC167686681, can interact with the DNMTs in structurally distinct ways.
To understand the structural dynamics of DNMTs and any alteration in the presence of ligands, we performed dynamic cross-correlation (DCC) analysis of the MD simulation trajectories. DCC has been used to determine dynamically correlated motions in proteins through the analysis of individual amino acid residue pairs in the protein structure over a simulation trajectory. For this, we first aligned all six trajectories using Cα atoms and determined DCC using the Bio3D package in R software (Grant et al., Citation2006; Skjærven et al., Citation2014). Our results indicate higher global dynamic correlated and anticorrelated motions in DNMT1 compared to DNMT3a or DNMT3b (). Moreover, residue positions between 1,500 and 1,600 seem to have higher dynamically correlated motions in the holo DNMT1 compared to the apo structure. While more global increase in this type of motion is observed in the holo DNMT3a complex compared to the apo. On the other hand, there seem to be a rather global decrease in the dynamically correlated motions in holo DNMT3b compared to apo structure. Finally, we determined the free energy change of ligand binding to the DNMTs using the LIE method () and found the values to be −15.27 ± 2.48, −13.24 ± 5.45 and −16.52 ± 1.45 kcal/mol for DNMT1, DNMT3a and DNMT3b, respectively. Overall, these data provide additional supportive evidence that the inhibitor interacts differently with the different DNMT subtypes.
4. Discussion
The present work finds a novel inhibitor that can potentially inhibit the aberrant function of DNMT1, DNMT3A, and DNMT3B. Molecular docking and molecular dynamics are commonly utilized during the drug discovery process to investigate ligand-receptor interactions (Sheikh et al., Citation2020). Here, we used virtual screening, molecular docking as well as ADMET analysis, and identified the best inhibitor for DNMTs from a large number of compounds. Moreover, in order to understand the interaction of the identified inhibitor, we performed all-atom, explicit solvent molecular dynamics (MD) simulations using the docked complexes. Additionally, we also performed simulations of the proteins without the ligand (apo) for the purpose of comparison. Generally, the inhibitor appears to bind to all three proteins with the exception of DNMT3A where it starts to unbind after around 90 ns but moves back towards the binding pocket, although the rebinding may not be as tight as it was in the beginning since the ligand continues to show higher solvent accessible surface area. Additionally, it was interesting to observe only a small increase in the protein RMSD in each case.
Two nucleoside analog DNMT inhibitors have been approved by FDA: azacytidine (5-azacytidine) and decitabine (5-aza-20-deoxycytidine) for the treatment of acute myeloid leukaemia (AML), myelodysplastic syndromes (MDS), and chronic myelomonocytic leukaemia (CMML) (Esteller, Citation2008). Pappalardi et al., reported a novel non-nucleoside DNMT1 inhibitor (GSK3685032) that showed better results in in vitro and animal studies for leukaemia (Pappalardi et al., Citation2021). Previously, it was reported that Val1580, Asn1578, Met1169 and Trp893, Arg891, Val665 are key residues in the binding site of DNMT1 and DNMT3A for the selectivity of inhibitors (Xie et al., Citation2019). However, our result is slightly different from this observation, as we observe only residue Arg891 of DNMT3A bind with our inhibitor and other key residues are different from previous findings.
Absorption, distribution, metabolism and excretion are important for efficacy and drug safety, identification of which is an important step in clinical trials (Reddy & Zhang, Citation2013). We compared the pharmacokinetic properties of our inhibitor with 31 previously reported, identified, and repurposed inhibitors. We identified that our inhibitor showed excellent properties as compared to all other reported inhibitors, including GSK3685032 and decitabine ( in Appendix). For example, GSK3685032 and decitabine may cause hepatotoxicity (Daina et al., Citation2017; Pires et al., Citation2015). The following factors indicate the potential for drug absorption: compound water solubility, cell permeability in the CaCO2 cell line, skin permeability, and human intestinal absorption, as well as whether the molecule is a P-glycoprotein substrate or inhibitor (Daina et al., Citation2017). The results obtained in our study showed that ZINC167686681 is soluble in water with high intestinal absorption and has lower CaCO2 permeability (0.57) as compared to all other compounds ( in Appendix) whereas, bazedoxifene, a recently reported novel drug for the treatment of TNBC (Huang et al., Citation2017) is poorly soluble in water and CaCO2 permeability is 1.068. The pharmacokinetic properties showed that ZINC167686681 follows the Lipinski’s Rule of 5 (Jo et al. 2007) having molecular weight <500 g/mol, hydrogen bond donor <5, and hydrogen bond acceptors <10. Moreover, drug-induced hepatoxicity is a very critical factor in drug designing as more than 1000 drugs induce liver injury and toxicity (Kuna et al., Citation2018). Our inhibitor was predicted not to induce hepatoxicity and hence can be considered a safe drug. Based on all these findings, we believe that ZINC167686681 could be used as a potential DNMT inhibitor, however, further in vitro and in vivo assays need to be performed to substantiate this observation. Lack of experimental validation is indeed an important limitation of our study.
5. Conclusions
In conclusion, we discovered a new potential DNMT inhibitor (ZINC167686681) having excellent pharmacokinetic properties that demonstrated key interaction with DNMTs. Further preclinical and clinical studies will reveal the potential of this inhibitor in treatment against cancers.
Author contributions
ZJ, WSA performed experiments, analysed data, and contributed to writing. PVJ and KHB conceptualized, implemented, supervised, analysed, and wrote the manuscript. All authors read and approved the final manuscript.
Abbreviations | ||
DNMT | = | DNA methyltransferase |
MD | = | molecular dynamics |
RMSD | = | root mean square deviation |
RMSF | = | root mean square fluctuation |
Rog | = | radius of gyration |
SASA | = | solvent-accessible surface area |
DCC | = | dynamic cross-correlation |
DAC | = | decitabine |
AZA | = | azacytidine |
ESOL | = | estimated solubility |
BBB | = | blood-brain barrier |
CNS | = | central nervous system |
PAINS | = | Pan-Assay INterference compoundS |
Disclosure statement
No potential conflict of interest was reported by the author(s).
Data availability statement
Publicly available datasets and tools were employed for this study.
Additional information
Funding
References
- Agrawal, K., Das, V., Vyas, P., & Hajdúch, M. (2018). Nucleosidic DNA demethylating epigenetic drugs – a comprehensive review from discovery to clinic. Pharmacology & Therapeutics, 188, 45–79. https://doi.org/10.1016/j.pharmthera.2018.02.006
- Ahmed, W. S., Philip, A. M., & Biswas, K. H. (2021). Decreased interfacial dynamics caused by the N501Y mutation in the SARS-CoV-2 S1 spike: ACE2 complex. Front Mol Biosci., 9, 846996. https://doi.org/10.1101/2021.01.07.425307
- Altamash, T., Ahmed, W., Rasool, S., & Biswas, K. H. (2021). Intracellular ionic strength sensing using NanoLuc. International Journal of Molecular Sciences, 22(2), 677. https://doi.org/10.3390/ijms22020677
- Anwar, S. L., & Lehmann, U. (2014). DNA methylation, microRNAs, and their crosstalk as potential biomarkers in hepatocellular carcinoma. World Journal of Gastroenterology, 20(24), 7894–7913. https://doi.org/10.3748/wjg.v20.i24.7894
- Daina, A., Michielin, O., & Zoete, V. (2017). SwissADME: A free web tool to evaluate pharmacokinetics, drug-likeness and medicinal chemistry friendliness of small molecules. Scientific Reports, 7, 42717. https://doi.org/10.1038/srep42717
- Essmann, U., Perera, L., Berkowitz, M. L., Darden, T., Lee, H., & Pedersen, L. G. (1995). A smooth particle mesh Ewald method. The Journal of Chemical Physics, 103(19), 8577–8593. https://doi.org/10.1063/1.470117
- Esteller, M. (2008). Epigenetics in cancer. The New England Journal of Medicine, 358(11), 1148–1159. https://doi.org/10.1056/NEJMra072067
- Feller, S. E., Zhang, Y., Pastor, R. W., & Brooks, B. R. (1995). Constant pressure molecular dynamics simulation: The Langevin piston method. The Journal of Chemical Physics, 103(11), 4613–4621. https://doi.org/10.1063/1.470648
- Gao, F., & Das, S. K. (2014). Epigenetic regulations through DNA methylation and hydroxymethylation: Clues for early pregnancy in decidualization. Biomolecular Concepts, 5(2), 95–107. https://doi.org/10.1515/bmc-2013-0036
- Gao, L., Emperle, M., Guo, Y., Grimm, S. A., Ren, W., Adam, S., Uryu, H., Zhang, Z.-M., Chen, D., Yin, J., Dukatz, M., Anteneh, H., Jurkowska, R. Z., Lu, J., Wang, Y., Bashtrykov, P., Wade, P. A., Wang, G. G., Jeltsch, A., & Song, J. (2020). Comprehensive structure-function characterization of DNMT3B and DNMT3A reveals distinctive de novo DNA methylation mechanisms. Nature Communications, 11(1), 3355. https://doi.org/10.1038/s41467-020-17109-4
- Geethakumari, A. M., Ahmed, W. S., Rasool, S., Fatima, A., Nasir Uddin, S. M., Aouida, M., & Biswas, K. H. (2022). A genetically encoded BRET-based SARS-CoV-2 Mpro protease activity sensor. Communications Chemistry, 5(1), 117. https://doi.org/10.1038/s42004-022-00731-2
- Grant, B. J., Rodrigues, A. P. C., ElSawy, K. M., McCammon, J. A., & Caves, L. S. D. (2006). Bio3d: An R package for the comparative analysis of protein structures. Bioinformatics, 22(21), 2695–2696. https://doi.org/10.1093/bioinformatics/btl461
- Horton, J. R., Pathuri, S., Wong, K., Ren, R., Rueda, L., Fosbenner, D. T., Heerding, D. A., McCabe, M. T., Pappalardi, M. B., Zhang, X., King, B. W., & Cheng, X. (2022). Structural characterization of dicyanopyridine containing DNMT1-selective, non-nucleoside inhibitors. Structure, 30(6), 793–802.e5. https://doi.org/10.1016/j.str.2022.03.009
- Huang, J., Rauscher, S., Nawrocki, G., Ran, T., Feig, M., de Groot, B. L., Grubmüller, H., & MacKerell, A. D. (2017). CHARMM36m: An improved force field for folded and intrinsically disordered proteins. Nature Methods, 14(1), 71–73. https://doi.org/10.1038/nmeth.4067
- Humphrey, W., Dalke, A., & Schulten, K. (1996). VMD: Visual molecular dynamics. Journal of Molecular Graphics, 14(1), 33–38. https://doi.org/10.1016/0263-7855(96)00018-5
- Irwin, J. J., & Shoichet, B. K. (2005). ZINC − a free database of commercially available compounds for virtual screening. Journal of Chemical Information and Modeling, 45(1), 177–182. https://doi.org/10.1021/ci049714+
- Jinawath, A., Miyake, S., Yanagisawa, Y., Akiyama, Y., & Yuasa, Y. (2005). Transcriptional regulation of the human DNA methyltransferase 3A and 3B genes by Sp3 and Sp1 zinc finger proteins. The Biochemical Journal, 385(Pt 2), 557–564. https://doi.org/10.1042/BJ20040684
- Jithesh, P. V., Risk, J. M., Schache, A. G., Dhanda, J., Lane, B., Liloglou, T., & Shaw, R. J. (2013). The epigenetic landscape of oral squamous cell carcinoma. British Journal of Cancer, 108(2), 370–379. https://doi.org/10.1038/bjc.2012.568
- Jo, S., Kim, T., Iyer, V. G., & Im, W. (2008). CHARMM-GUI: A web-based graphical user interface for CHARMM. Journal of Computational Chemistry, 29(11), 1859–1865. https://doi.org/10.1002/jcc.20945
- Jones, P. A., & Taylor, S. M. (1980). Cellular differentiation, cytidine analogs and DNA methylation. Cell, 20(1), 85–93. https://doi.org/10.1016/0092-8674(80)90237-8
- Jorgensen, W. L., Chandrasekhar, J., Madura, J. D., Impey, R. W., & Klein, M. L. (1983). Comparison of simple potential functions for simulating liquid water. Journal of Chemical Physics. 79(2), 926–935. https://doi.org/10.1063/1.445869
- Kumar, S., Chinnusamy, V., & Mohapatra, T. (2018). Epigenetics of modified DNA bases: 5-Methylcytosine and beyond. Frontiers in Genetics, 9, 640. https://doi.org/10.3389/fgene.2018.00640
- Kuna, L., Bozic, I., Kizivat, T., Bojanic, K., Mrso, M., Kralj, E., Smolic, R., Wu, G. Y., & Smolic, M. (2018). Models of drug induced liver injury (DILI) – current issues and future perspectives. Current Drug Metabolism, 19(10), 830–838. https://doi.org/10.2174/1389200219666180523095355
- Lopez, M., Gilbert, J., Contreras, J., Halby, L., & Arimondo, P. B. (2022). Inhibitors of DNA Methylation. Advances in Experimental Medicine and Biology, 1389, 471–513. https://doi.org/10.1007/978-3-031-11454-0_17
- Lyko, F. (2018). The DNA methyltransferase family: A versatile toolkit for epigenetic regulation. Nature Reviews-Genetics, 19(2), 81–92. https://doi.org/10.1038/nrg.2017.80
- Moore, L. D., Le, T., & Fan, G. (2013). DNA methylation and its basic function. Neuropsychopharmacology, 38(1), 23–38. https://doi.org/10.1038/npp.2012.112
- Pappalardi, M. B., Keenan, K., Cockerill, M., Kellner, W. A., Stowell, A., Sherk, C., Wong, K., Pathuri, S., Briand, J., Steidel, M., Chapman, P., Groy, A., Wiseman, A. K., McHugh, C. F., Campobasso, N., Graves, A. P., Fairweather, E., Werner, T., Raoof, A., … McCabe, M. T. (2021). Discovery of a first-in-class reversible DNMT1-selective inhibitor with improved tolerability and efficacy in acute myeloid leukemia. Nature Cancer, 2(10), 1002–1017. https://doi.org/10.1038/s43018-021-00249-x
- Phillips, J. C., Braun, R., Wang, W., Gumbart, J., Tajkhorshid, E., Villa, E., Chipot, C., Skeel, R. D., Kalé, L., & Schulten, K. (2005). Scalable molecular dynamics with NAMD. Journal of Computational Chemistry, 26(16), 1781–1802. https://doi.org/10.1002/jcc.20289
- Pires, D. E. V., Blundell, T. L., & Ascher, D. B. (2015). pkCSM: Predicting small-molecule pharmacokinetic and toxicity properties using graph-based signatures. Journal of Medicinal Chemistry, 58(9), 4066–4072. https://doi.org/10.1021/acs.jmedchem.5b00104
- Reddy, A. S., & Zhang, S. (2013). Polypharmacology: Drug discovery for the future. Expert Review of Clinical Pharmacology, 6(1), 41–47. https://doi.org/10.1586/ecp.12.74]
- Roll, J. D., Rivenbark, A. G., Jones, W. D., & Coleman, W. B. (2008). DNMT3b overexpression contributes to a hypermethylator phenotype in human breast cancer cell lines. Molecular Cancer, 7, 15. https://doi.org/10.1186/1476-4598-7-15
- Schindelin, J., Arganda-Carreras, I., Frise, E., Kaynig, V., Longair, M., Pietzsch, T., Preibisch, S., Rueden, C., Saalfeld, S., Schmid, B., Tinevez, J.-Y., White, D. J., Hartenstein, V., Eliceiri, K., Tomancak, P., & Cardona, A. (2012). Fiji: An open-source platform for biological-image analysis. Nature Methods, 9(7), 676–682. https://doi.org/10.1038/nmeth.2019
- Scholz, C., Knorr, S., Hamacher, K., & Schmidt, B. (2015). DOCKTITE-a highly versatile step-by-step workflow for covalent docking and virtual screening in the molecular operating environment. Journal of Chemical Information and Modeling, 55(2), 398–406. https://doi.org/10.1021/ci500681r
- Sheikh, A., Al-Taher, A., Al-Nazawi, M., Al-Mubarak, A. I., & Kandeel, M. (2020). Analysis of preferred codon usage in the coronavirus N genes and their implications for genome evolution and vaccine design. Journal of Virological Methods, 277, 113806. https://doi.org/10.1016/j.jviromet.2019.113806
- Skjærven, L., Yao, X.-Q., Scarabelli, G., & Grant, B. J. (2014). Integrating protein structural dynamics and evolutionary analysis with Bio3D. BMC Bioinformatics, 15(1), 399. https://doi.org/10.1186/s12859-014-0399-6
- Steinbach, P. J., & Brooks, B. R. (1994). New spherical-cutoff methods for long-range forces in macromolecular simulation. Journal of Computational Chemistry, 15(7), 667–683. https://doi.org/10.1002/jcc.540150702
- Sterling, T., & Irwin, J. J. (2015). ZINC 15 – Ligand discovery for everyone. Journal of Chemical Information and Modeling, 55(11), 2324–2337. https://doi.org/10.1021/acs.jcim.5b00559
- Stresemann, C., & Lyko, F. (2008). Modes of action of the DNA methyltransferase inhibitors azacytidine and decitabine. International Journal of Cancer, 123(1), 8–13. https://doi.org/10.1002/ijc.23607
- Vilar, S., Cozza, G., & Moro, S. (2008). Medicinal chemistry and the molecular operating environment (MOE): Application of QSAR and molecular docking to drug discovery. Current Topics in Medicinal Chemistry, 8(18), 1555–1572. https://doi.org/10.2174/156802608786786624
- Wong, K. K. (2021). DNMT1: A key drug target in triple-negative breast cancer. Seminars in Cancer Biology, 72, 198–213. https://doi.org/10.1016/j.semcancer.2020.05.010
- Wong, K. K., Lawrie, C. H., & Green, T. M. (2019). Oncogenic roles and inhibitors of DNMT1, DNMT3A, and DNMT3B in acute myeloid leukaemia. Biomarker Insights, 14, 1177271919846454. https://doi.org/10.1177/1177271919846454
- Xie, T., Yu, J., Fu, W., Wang, Z., Xu, L., Chang, S., Wang, E., Zhu, F., Zeng, S., Kang, Y., & Hou, T. (2019). Insight into the selective binding mechanism of DNMT1 and DNMT3A inhibitors: A molecular simulation study. Physical Chemistry Chemical Physics, 21(24), 12931–12947. https://doi.org/10.1039/C9CP02024A
- Yang, H., Sun, L., Wang, Z., Li, W., Liu, G., & Tang, Y. (2018). ADMETopt: A web server for ADMET optimization in drug design via scaffold hopping. Journal of Chemical Information and Modeling, 58(10), 2051–2056. https://doi.org/10.1021/acs.jcim.8b00532
- Yoshikawa, N., & Hutchison, G. R. (2019). Fast, efficient fragment-based coordinate generation for Open Babel. Journal of Cheminformatics, 11(1), 49. https://doi.org/10.1186/s13321-019-0372-5
- Zhang, W., & Xu, J. (2017). DNA methyltransferases and their roles in tumorigenesis. Biomarker Research, 5, 1. https://doi.org/10.1186/s40364-017-0081-z
- Zhang, Z.-M., Liu, S., Lin, K., Luo, Y., Perry, J. J., Wang, Y., & Song, J. (2015). Crystal structure of human DNA methyltransferase 1. Journal of Molecular Biology, 427(15), 2520–2531. https://doi.org/10.1016/j.jmb.2015.06.001
- Zhang, Z.-M., Lu, R., Wang, P., Yu, Y., Chen, D., Gao, L., Liu, S., Ji, D., Rothbart, S. B., Wang, Y., Wang, G. G., & Song, J. (2018). Structural basis for DNMT3A-mediated de novo DNA methylation. Nature, 554(7692), 387–391. https://doi.org/10.1038/nature25477
Appendix
Figure A1. Interaction analysis of DNMT1, DNMT3A and DNMT3B with ZINC201939708. (A) Presents the docked complex of DNMT1 with ZINC201939708. Left side of the figure shows the docked complex and the domains of DNMT1 (CXXC ; BAH1; BAH2; MTase; RFTS). The interacting residues are shown in the inset picture. (B) Shows the docked complexes of DNMT3A and DNMT3B with ZINC201939708. Superimposed image of the docked complex shows the highly similar structure of DNMT3A and DNMT3B. The inset figure shows the interacting residues of DNMT3A and DNMT3B with ZINC201939708.
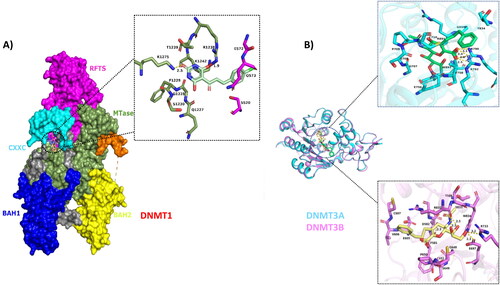
Table A1. Physicochemical and pharmacokinetic properties of ZINC201939708.
Table A2. Occupancy of hydrogen bonds between the protein and the ligand in the complexes.
Table A3. Pharmacokinetic/toxicity profiles and drug-likeness of the lead compound compared with several medications including DNMT inhibitors.
Table A4. Comparison of binding energies and interaction analysis of DNMT1, DNMT3A and DNMT3B with ZINC201939708 and ZINC167686681.