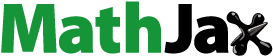
ABSTRACT
Inhibition is one of the core components of cognitive control. In experimental tasks which measure cognitive inhibition, performance may vary according to an interplay of individuals’ chronotype and the time of day of testing (“synchrony effect”, or the beneficial impact on cognitive performance of aligning testing with the time of day preferred by an individual’s chronotype). Some prior studies have reported a synchrony effect specifically emerging in activities which require cognitive inhibition, but not in general processing speed, but existing findings are inconsistent. If genuine, synchrony effects should be taken into account when comparing groups of participants. Here we explored whether synchrony effects emerge in a sample of young adults. In a multi-part online study, we captured various components of inhibition (response suppression; inhibitory control; switching) plus a general measure of processing speed across various times of the day. Individuals’ chronotype was included as a predictor of performance. Critically, we found no evidence of a synchrony effect (an association between chronotype and component of interest where the directionality is dependent on time of testing) in our study.
Introduction
Cognitive inhibition, or the ability to inhibit irrelevant information and responses, is one of the core components of executive function (Diamond Citation2013). Previous studies suggest that inhibition is at its peak in young adulthood, with older adults exhibiting weaker inhibitory abilities than young adults (e.g., Bedard et al. Citation2002; Carpenter et al. Citation2020; May Citation1999). Few studies performing between-group comparisons such as comparing performance of young and older individuals report the time of day at which participants carried out the study, but sometimes, to control for potential time of day effects, researchers may choose to test all participants at similar times. May et al. (Citation1993) performed an informal survey and reported that researchers are most likely to test both young and older adults between 12:00 h and 18:00 h. Doing so controls for potential time of day effects which might arise from fluctuations in circadian rhythm. Circadian rhythm is described as an internal clock that regulates the sleep-wake cycle on a roughly 24-hr basis. It manifests itself in a range of physiological parameters such as body temperature, but it also has consequences for a wide range of human behavior including attention, memory, and executive function (see Schmidt et al. Citation2007, for a comprehensive overview of circadian effects on cognition).
Circadian rhythm can vary from person to person and as a result, individuals differ in their preferred time of testing. Some people feel most alert in the morning and in turn, prefer to perform difficult physical and cognitive activities at this time (“morning type”) whereas others feel at their peak in the evening (“evening type”); many individuals do not exhibit a strong preference (“neutral type”). This preference towards performing daily activities in the morning, evening, or somewhere in between is captured by the notion of a “chronotype” (Levandovski et al. Citation2013). An individual’s chronotype can easily and reliably be captured via psychometric tools such as Horne and Ostberg’s (Horne and Östberg Citation1976) Morningness-Eveningness questionnaire (MEQ). MEQ scores correlate with physiological measures of circadian rhythm, including hormone secretion and body temperature (Bailey and Heitkemper Citation2001; Horne and Östberg Citation1977; Nebel et al. Citation1996). Importantly, studies have reported significant age differences in chronotypes (Cajochen et al. Citation2006; May and Hasher Citation1998; Roenneberg et al. Citation2007; Yoon et al. Citation1999), with young adults more likely to identify themselves as an “evening type” than a “morning type” but the reverse for older adults. Hence, as individuals age, their chronotype tends to shift from eveningness to morningness.
Depending on an individual’s chronotype, aspects of cognitive performance may fluctuate when assessed at various points across the day. Indeed, chronotype along with time of testing have been reported to influence performance in a variety of cognitive tasks measuring attention, working memory and verbal memory (e.g., Barner et al. Citation2019; Facer-Childs et al. Citation2018; Intons-Peterson et al. Citation1999; Lehmann et al. Citation2013; Maylor and Badham Citation2018; Schmidt et al. Citation2007; Yang et al. Citation2007; Yoon et al. Citation1999). The interplay between chronotype and testing time is captured in the notion of “synchrony”: when assessment time is aligned with the time of day favored by an individual’s chronotype, cognitive task performance is superior than when they are misaligned (May and Hasher Citation1998).
Given the impact that synchrony effects can have on cognitive task performance, testing all participants at the same time of day may be problematic, and particularly so in group comparisons. For example, as mentioned earlier, young adults tend to have a preference for eveningness while older adults usually possess a preference for morningness. Therefore, testing both age groups at the same time could potentially mask or exaggerate group differences in performance: testing both age groups in the morning could mask age-related differences in performance since the testing time is advantageous to older adults but a hindrance to young adults. Conversely, if the testing time was in the afternoon (as is oftentimes the case, as highlighted above; May et al. Citation1993) then group differences might be exacerbated. Hence an understanding of synchrony effects in a given cognitive domain is important in and by itself, but particularly so when making between-group comparisons.
Prior research suggests that synchrony effects may emerge in some measures of cognitive performance but not in others. Therefore, the level of importance of controlling for this effect may depend on what aspect of cognition is being explored. A possibility is that synchrony effects do not arise in tasks that rely on automatic responses but that they are particularly pronounced in activities which require inhibitory abilities (Lustig et al. Citation2007; May and Hasher Citation1998). No synchrony effects were found in tasks that measured vocabulary, processing speed and general knowledge (Borella et al. Citation2010; Lara et al. Citation2014; May Citation1999; May and Hasher Citation1998,Citation2017; Song and Stough Citation2000), presumably because these activities do not directly involve cognitive inhibition. On the other hand, in their pioneering study May and Hasher reported superior performance in the sentence completion task, which is presumed to index inhibition, among young adults tested at their preferred time of testing (i.e., the optimal group) than those tested at their non-preferred time of testing (i.e., the non-optimal group). Similar synchrony effects have been reported in other tasks which require inhibition including the Sustained Attention to Response Task and memory tasks which involve ignoring specific stimuli (e.g., Lara et al. Citation2014; May Citation1999; Ngo and Hasher Citation2017; Rothen and Meier Citation2016). However, synchrony effects among young adults have not consistently been found in tasks which are regarded as standard measures of inhibition (e.g., Borella et al. Citation2010; May and Hasher Citation1998; Schmidt, Peigneux, Leclercq et al. Citation2012b). For instance, as previously mentioned, May and Hasher (Citation1998) reported a synchrony effect in the sentence completion task, but no such effect was found for young adults in more frequently used inhibition tasks including Stroop and stop-signal tasks (however, it should be noted that results were in the direction of worse performance in the non-optimal than optimal group).
The reason as to why there is a discrepancy in findings between different measures of inhibition remains elusive but it might be partly attributable to the scarcity of published behavioral studies which have investigated this issue. Furthermore, many of the inconsistent results could also be attributed to small sample sizes (Richards et al. Citation2020). For example, the pioneering study by May and Hasher (Citation1998) reported two experiments which used a between-participants design with 48 younger and 48 older participants, half of them tested in the morning and the other half tested in the evening. Barclay and Myachykov (Citation2017) employed a repeated-measures design in which the performance of 26 younger individuals on the ANT was tracked across two times of the day. Despite the within-participant measurements, it is doubtful whether a sample of this size is substantial enough to reliably detect synchrony effects.
The studies mentioned thus far recruited participants who were categorized as a “morning type” or an “evening type,” with participants who identified as a “neutral type” excluded from the study or not invited to complete the cognitive tasks. A consequence of focusing on individuals who belong to an “extreme” group of a morning or evening chronotype is that we do not have a complete understanding of how the interplay of chronotype and time of testing impacts cognitive task performance. Young and older “neutral types” were targeted in May and Hasher (Citation2017); participants completed tasks that measured a wide range of cognitive processes including inhibition, processing speed, memory and knowledge, with inhibition measured via a Stroop task. Participants were assigned to complete these tasks either in the morning (08:00 h-09:00 h), at noon (12:00 h-13:00 h) or in the afternoon (16:00 h-17:00 h). The authors expected the noon group to perform better at the Stroop task than the other time groups, based on the assumption that for “neutral types” the optimal time to perform cognitive tasks should be at noon. However, for the young participants very similar Stroop effects were found in the three time groups. It is unclear why no synchrony effect was found in young “neutral types,” given that previous studies implied synchrony effects for young “evening types” (e.g., May Citation1999). One potential explanation, provided by the authors, for this disparity in results is that young “neutral types” possess more cognitive flexibility than young “evening types.” Then again, there is not a clear explanation of why this would be the case. Overall, May and Hasher’s (May and Hasher Citation2017) study provided mixed results regarding synchrony effects in “neutral types.”
In summary, the notion of “synchrony” in cognitive inhibition provides an intriguing theoretical concept but empirical support for it is at present mixed. Results for or against the psychological reality of synchrony effects are inconsistent, with some informative findings but also a good deal of null results. As we hoped to have highlighted above, it is challenging to a) identify adequate empirical tasks which could potentially capture synchrony effects, b) amass sufficient samples which would provide clear evidence for or against the synchrony concepts.
The present study
We tracked performance of a group of over 100 young adults across three times of the day. A sample of this size was made possible by conducting the entire study online. To capture cognitive inhibition, we employed the so-called Faces task (Bialystok et al. Citation2006; explained in detail below). This task captures two potentially separable components of inhibition (the authors defined this as “response suppression” and “inhibitory control”, which is parallel to the “restraint” and “access” functions in Hasher et al. Citation2007, framework), plus a further potential indicator of inhibition (the ability to switch), within a single integrated experimental procedure. Participants’ chronotype was captured by their individual MEQ scores, and unlike in the majority of prior studies, throughout the analysis we treated chronotype as a continuous variable. The classification system of the MEQ can be considered arbitrary and by analyzing MEQ score in a continuous manner, we are able to identify how a whole spectrum of chronotypes (which is presumably representative of the target group; but see Discussion) respond to being tested at various times of the day. The analytic approach consisted of trying to predict performance corresponding to the various components of inhibition (see above) by individuals’ MEQ scores, at various times of the day. Additionally, we included a measure of processing speed, which allowed us to assess the claim (Lustig et al. Citation2007) that tasks which require automatic responses but no inhibitory skills are not subject to synchrony effects. We captured processing speed with the Deary-Liewald task (Deary et al. Citation2011) via the measurement of simple and choice reaction times. The former is measured by asking participants to respond to a single stimulus as quickly as possible while the latter is measured by asking participants to make an appropriate response to one of four stimuli. As for the Faces task, individuals’ performance on the Deary-Liewald task was tracked at three times of the day. Overall, our study substantially deviates from most prior studies in a number of respects, including the multi-sessional nature of our study, our analytic approach and our comparatively large sample size.
Our study was entirely performed online and to our knowledge, there is yet a study which has examined synchrony effects via an online setting. The platform “Gorilla” (https://www.gorilla.sc), an experiment builder tool that enables researchers to conduct studies online, was used to run the tasks and questionnaires. As highlighted above, a major advantage of performing online testing is that recruitment of a large sample in a multi-sectional study is feasible. Possible adverse consequences of online testing are highlighted in the Discussion.
Throughout our study, we treated chronotype as a continuous variable (captured by MEQ score) and we tried to predict a particular component of cognitive performance (response suppression; inhibitory control; task switching) based on a combination of MEQ and time of the day (morning; noon; afternoon). In this design, a synchrony effect would be demonstrated if we find a significant correlation between MEQ and the component of interest and if the nature of this correlation is dependent on time of testing. Specifically, the prediction is that for the morning session, a tendency towards morningness should lead to a smaller inhibitory component than a tendency towards eveningness, and the reverse for the afternoon session. Separating inhibition into multiple components allows us to explore whether all, or perhaps only a subset of them, are affected by synchrony. Finally, inclusion of the Deary-Liewald task allows us to investigate to what extent measures of inhibitory control dissociate from more general measures of processing speed.
Method
Participants
Initially, we recruited 332 young adult participants through a combination of Prolific (https://prolific.co/) and the participants pool of the University of Bristol. Participants recruited via Prolific received a monetary reward for their participation while the others received course credits. All participants were in the UK (GMT) time zone during testing. The study comprised four separate testing sessions (see below). 127 participants did not attend all the sessions and were therefore excluded from analysis. Further exclusions were made before the final analysis and will be discussed in more detail in the results section. All participants were self-reported as: monolingual, not color-blind, not shift-workers, having not recently travelled abroad and not having sleeping problems. All participants provided their informed consent and this study was approved by the University of Bristol Faculty of Life Sciences Research Ethics Committee (Approval code: 12121997085).
Procedure
Participants attended four consecutive online sessions. They could only access the sessions through desktop computers or laptops. All participants completed a practice session on the day preceding the experimental phase. In this session, participants read the information sheet, provided informed consent, and completed the background questionnaire as well as the MEQ. Then, they completed practice runs of both the Faces task and the Deary-Liewald task. This enabled participants to familiarize themselves with the respective procedures, and minimized practice effects across the critical sessions which started the following day.
Each participant completed three critical experimental sessions, conducted in the morning, noon, and afternoon. A third of participants completed the sessions in the order morning-noon-afternoon; a third completed the order noon-afternoon-morning (of next day for the morning session), and the remaining third completed the order afternoon-morning (next day)-noon (next day). Counterbalancing of the order was intended to further minimize the confounding of residual practice effects with session time. Morning sessions occurred between 08:00 h and 09:00 h (GMT Time), noon sessions occurred between 12:00 h and 13:00 h (GMT Time), and afternoon sessions occurred between 16:00 h and 17:00 h (GMT Time). Participants were informed to virtually attend these sessions through the messaging tools available at the University of Bristol and in Prolific. Additionally, Gorilla has a feature where researchers can track the exact time in which they perform tasks and questionnaires. Therefore, this feature allows us to ensure that participants are attending the online sessions at the correct time. In each session, participants completed a session questionnaire, the Faces task and finally the Deary-Liewald task. In the final session, participants were debriefed. Each session took approximately 25 minutes to complete.
Materials
Morningness-eveningness questionnaire (MEQ)
Chronotype was assessed through Horne and Ostberg’s (Horne and Östberg Citation1976) MEQ. This questionnaire consists of 19 multiple choice questions regarding preferred timings of activities and sleep-wake habits. An MEQ score, ranging from 16 and 86, is calculated by combining all the responses, with higher MEQ scores suggesting a greater morningness preference while lower MEQ scores indicating greater eveningness preference. Conventionally, individuals are categorized into one of five chronotypes based on their score: “definite morning” (70–86), “moderate morning” (59–69), “intermediate” (42–58), “moderate evening” (31–41), and “definite evening” (16–30). However, in our study, we treated MEQ and hence chronotype as a continuous variable.
Background questionnaire
14 questions in a background questionnaire elicited demographic information about the participants (i.e., sex, age, education, handedness and parents’ education) and information about participants’ level of involvement in activities including playing video games, using musical instruments and engaging in sports.
Session questionnaire
The session questionnaire was completed in morning, noon and afternoon sessions, and consisted of six questions about participants’ drug usage, alcohol intake, caffeine intake, hours of sleep, sleepiness (measured by the Visual Analogue Scale) and alertness (measured by the Stanford Sleepiness Score; Hoddes et al. Citation1973). To our knowledge, previous studies have not asked participants about these factors and as a result, arguably it would be difficult to disentangle the impact of sleep, alcohol intake and drug use from any potential synchrony effects. Therefore, we attempted to control these confounding factors by excluding participants based on how they respond to these questions (see Analysis section for more detail in the exclusion criteria used).
Faces task
The Faces task was adapted from Bialystok et al. (Citation2006) and provides separate measures of inhibitory control, response suppression, and task switching. shows example trials and experimental manipulations for the task. On each trial, a fixation cross was first displayed for 250 ms, followed by a cartoon face which was shown for 100 ms. After the cartoon face disappeared, participants saw a blank screen for 200 ms followed by two boxes, with one box containing an asterisk which acted as a spatial cue. The cartoon face varied in eye color (green vs red) and this provided a cue for the response: on trials with faces with green eyes, participants were instructed to make a response ipsilateral to the spatial cue; on trials with faces with red eyes, they were to make a response contralaterally to the spatial cue position. Eyes additionally varied in the gaze direction, which could be pointing left, right, or upward. The eye gaze could either point toward the position of the following spatial cue (congruent), point to the opposite side (incongruent), or could be pointing upward and hence to neither side (neutral). Participants were instructed to base their response on eye color and spatial cue position, but to ignore gaze direction. They pressed the “q” key for a “left” response and the “p” key for a “right” response. If no response was made within 2,000 ms following display of the boxes, the next trial began.
Figure 1. Example trials of all experimental conditions.
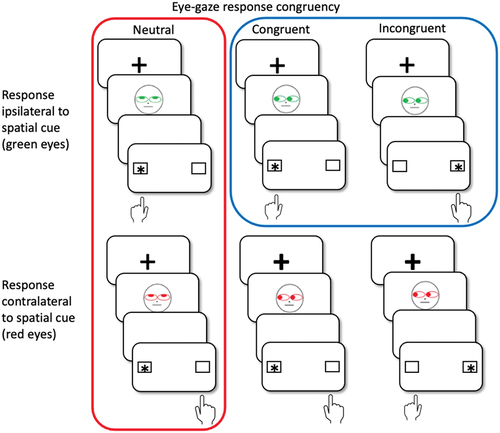
As shown in , our version of the Faces task implements a 3 (eye gaze: neutral, congruent, incongruent) × 2 (response ipsilateral vs. contralateral to spatial cue) experimental design. However, pilot experiments revealed that eye gaze and laterality of response interacted in somewhat unpredictable ways which made interpretation of an omnibus analysis of variance difficult. Specifically, the contralateral condition paired with incongruent eye gaze produced results which were difficult to interpret. Incongruent eye gaze cues the response side opposite to the one in which the spatial cue appears, but it also cues the correct side for the response because with red eyes, correct responses are contralateral to the cued location. For this reason, we decided to simplify the analysis by isolating the two relevant components of cognitive inhibition via specific contrasts. Response suppression was measured as the difference between green and red eyes (i.e., ipsilateral and contralateral responses) on neutral trials only. The two critical conditions are highlighted in red in . Inhibitory control was measured as the difference between congruent and incongruent eye gaze, but for green eyes (ipsilateral condition only). The two corresponding conditions are highlighted in blue in . To capture the third component of interest, task switch, all trials were coded with regard to whether task (i.e., ipsilateral vs. contralateral responses relative to the spatial cue) on the previous trial was the same as on the current one.
In the practice session (see below, “Procedure”), the Faces task involved 32 practice trials and 72 experimental trials. In the three critical sessions, the Faces task consisted of 32 practice trials and 252 experimental trials. In both the practice and critical sessions, half of the trials involved red eyes and the other half involved green eyes. Furthermore, one third of the experimental trials were congruent, one third were incongruent and one third were neutral.
Deary-liewald task
Our measure of processing speed was adapted from Deary et al. (Citation2011). The task consists of two components: a simple reaction time (SRT) task and a choice reaction time (CRT) task. In a SRT trial, a box was displayed on the screen, and participants were instructed to press the spacebar key as quickly as possible whenever an “X” appeared in the box. On each trial, one of six different wait times (i.e., time between the start of the trial and when the “X” appeared) was randomly chosen: 400 ms, 500 ms, 700 ms, 800 ms, 1,000 ms and 2,000 ms. In a CRT trial, four horizontally aligned boxes were shown and an “X” appeared randomly in one of the boxes, again following one of the six randomly chosen wait times. Participants pressed one of four designated response keys (“z” and “X” keys, pressed with the index and middle finger of the left hand, and “,” and “.” keys pressed with index and middle finger of the right hand) as quickly as possible. In both SRT and CRT trials, the “X” was displayed for 1,000 ms. All participants completed the SRT task first, followed by the CRT task. In both the practice and critical sessions, this task consisted of 30 SRT trials and 30 CRT trials.
Statistical analysis
Faces task
In an initial analysis, we analyzed response latencies and errors in the manner outlined in , attempting to establish the presence of the three components of interest, via separate one-way analyses of variance (ANOVAs). Subsequently we sought to establish whether latency effects were subject to synchrony effects (the interplay between time of testing and chronotype). To explore this issue, we computed “interference ratios” as described in Barzykowski et al. (Citation2021): for each participant, component and session time, the difference in latencies between the critical and the baseline condition was calculated, and these were divided by the baseline condition. Interference ratios were then z-transformed, separately for each component and session time. In this way, proportional scores were formed for which the overall level of latencies was no longer relevant. We then conducted regressions between interference ratios and MEQ, separately for each component and session time. If present, a synchrony effect would manifest itself as a slope of the regression lines deviating from zero, and more specifically, a positive slope for the morning session (i.e., smaller interference ratios for morning than for evening types) and/or a negative slope for the afternoon session (smaller ratios for evening than for morning types).
The results of analyses in which absolute latencies and errors, rather than interference ratios, were entered into a three-way ANOVA, with component (baseline vs. critical condition), session time, and MEQ as a covariate, and carried out separately for each component (response suppression; inhibitory control; switching) are reported in a Supplementary material document.
Deary-liewald task
Performance (latencies and errors) was analyzed first via a statistical comparison between SRT and CRT tasks. Subsequently, latencies were z-transformed for each task and session, and the transformed scores were analyzed regarding a potential correlation with MEQ scores. A potential synchrony effect on response latencies in the Deary-Liewald task would manifest itself as a regression line deviating from zero, with a predicted positive slope in the “morning” session (i.e., faster latencies for morning than for evening types) and/or a negative slope in the “afternoon” session (faster latencies for evening than for morning types).
Transparency and openness
We have reported all the data exclusions made and described all the measures used in our study. Our study’s design and the following analysis were not pre-registered. All the materials, data and analysis code used in our study are available at the Open Sciences Framework (OSF) repository and can be accessed at:
https://osf.io/xf5cn/?view_only=36a896247421460886278593c174140e
Results
Pre-processing
Participants were excluded from both the final Faces and Deary-Liewald task analysis for the following reasons: reported to not being a young adult (n = 1), reported to having less than 5 hours sleep before the first session and morning session (n = 15), reported to consuming recreation/prescription drugs which may impact cognitive performance (n = 10) and reported to consuming too much alcohol before at least one of the sessions (n = 3). Additionally, if the participant had error rates of more than 25% in one or both tasks, they were excluded from the final analysis of the task in which they performed poorly in. As a result, the number of eligible participants for the Faces task (n = 157) and Deary-Liewald task (n = 141) analysis differed slightly. Finally, we excluded random participants to ensure that for each task analysis, there were equal numbers of participants in each counterbalanced session order (e.g., whether the participants completed the morning, noon or afternoon session first).Footnote1 For the faces task, we randomly excluded 12 participants from the noon first group and 1 participant from the afternoon group. For the Deary-Liewald task, we excluded 8 participants from the morning first group and 10 participants from noon first group. The final analysis of the Faces task data consisted of 144 participants (mean age = 20.15 years old, Females = 102, Males = 42) while the analysis of the Deary-Liewald task data consisted of 123 participants (mean age = 20.15 years old, Females = 90, Males = 32, Prefer not to say = 1).
We used the software R (R Core team Citation2021) with the package afex (Singmann et al. Citation2016) for all statistical analyses. Response latencies over 2,000 ms and under 150 ms were removed for the response latencies aspect of the Faces task analysis (5.1% of trials excluded) and Deary-Liewald task analysis (0% excluded).
Faces task
Morningness-eveningness questionnaire
Note that in our statistical analyses reported below, we did not use these categories but rather treated MEQ score as a continuous variable. However, for our sample of 144 participants, the results were as follows: “definite morning:” n = 1 (0.7%); “moderate morning:” n = 15 (10.4%), “intermediate:” n = 91 (63.2%), “moderate evening:” n = 35 (24.3%), “definite evening:” n = 2 (1.4%). This profile of chronotypes among young adults converges with those found in earlier studies (e.g., May and Hasher Citation1998, reported 0, 5, 58, 31, and 6% for the corresponding five chronotype types among their younger participants). Importantly, MEQ scores in our sample showed considerable variability (mean/median of 47, SD = 8.3, range = 27–70).
Main analysis
shows the results of an initial analysis in which response latencies were computed in the manner outlined in , attempting to establish the presence of the three components of interest. We found a 20 ms effect of “response suppression” (ipsilateral: 318 ms; contralateral: 338 ms; F(1, 143) = 41.35, MSE = 674, p < 0.001, ηp2 = .224, BF10 >1,000), a 27 ms effect of “inhibitory control” (congruent: 306 ms, incongruent: 333 ms; F(1, 143) = 106.86, MSE = 494, p < 0.001, ηp2 = .428, BF10 >1,000), and a 19 ms effect of “task switching” (repeat: 317 ms, switch: 336 ms; F(1, 143) = 73.58, MSE = 359, p < 0.001, ηp2 = .340, BF10 >1,000). A parallel analysis conducted on error scores indicated a 1.0% effect of “response suppression” (ipsilateral: 6.6%; contralateral: 5.6%; F(1, 143) = 8.46, MSE = 8.66, p = 0.004, ηp2 = .056, BF10 = 6.38), a 3.6% effect of “inhibitory control” (congruent: 6.0%, incongruent: 9.6%; F(1, 143) = 80.97, MSE = 11.38, p < 0.001, ηp2 = .362, BF10 >1,000), and a 2.6% effect of “task switching” (repeat: 5.5%, switch: 8.2%; F(1, 143) = 87.08, MSE = 5.78, p < 0.001, ηp2 = .378, BF10 >1,000).
Figure 2. Response latencies (top row) and error percentages (bottom row), for three components of cognitive control (from left to right: response suppression, inhibitory control, task switching).

Note that for “response suppression” only, effects in latencies and errors point in opposite directions, hence a speed-accuracy tradeoff is present (faster RTs, but more errors, in the ipsilateral compared to the contralateral condition). To explore whether the effect in latencies reflected a shift in response criterion rather than a genuine effect, we computed “linear integrated speed-accuracy scores” (LISAS; Vandierendonck Citation2017) in which latencies and errors are combined via the formula:
where RTj is a participant’s mean RT in condition j, PEj is the corresponding error proportion, and SRT and SPE are the participant’s overall RT and error standard deviations. The response suppression effect remained highly significant even when latencies were corrected for errors in this way (F(1, 143) = 20.72, MSE = 1033, p < 0.001, ηp2 = .127, BF10 >1,000).
For response latencies, split-half reliability of the three components were estimated via the adjusted Spearman-Brown prophecy formula. These were .63, .50, and .74 for response suppression, inhibitory control, and task switching respectively. These results suggest that our manipulation successfully captured the three components of interest.
shows a visual representation of interference ratios (see section “Faces task” under “Method”) of individual participants, separately for the three components (panels from left to right: response suppression, inhibitory control, task switching), with MEQ score of participants on the x-axis, and the three sessions color-coded (morning, noon, and afternoon; see legend). For each component and session time, a regression line plus confidence interval is shown. To reiterate, a synchrony effect would manifest itself as a slope of the regression lines deviating from zero, and more specifically, a positive slope for the morning session (i.e., smaller interference ratios for morning than for evening types) and/or a negative slope for the afternoon session (smaller ratios for evening than for morning types). Visual inspection of the figure suggests that this is not the case. The figure also shows correlations between MEQ score and interference ratios, separately for each component and session time. None of them reached significance, with Bayesian statistics indicating “moderate” evidence supporting the null hypothesis. The exception was “task switching” in the ‘noon’ and “afternoon” sessions where Bayes factors were “inconclusive.” However, it should be noted that for the “afternoon” session, the direction of the correlation (larger interference ratios for evening than for morning) was opposite to what would be predicted by a synchrony effect.
Figure 3. Interference ratios (see “Faces task” in section “Method”), dependent on morningness-eveningness questionnaire score (MEQ; x-axis), component (from left to right: response suppression; inhibitory control; task switching) and session time (morning, noon, afternoon session; see legend). R and p correspond to regression lines and confidence intervals. BF = BF10 (Bayes factor in favor of H1 over the null hypothesis).
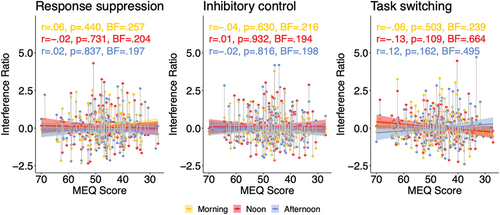
Deary-liewald task
Morningness-eveningness questionnaire
The MEQ results for the sample (n = 123) used for the Deary-Liewald analysis were as follows: “definite morning:” n = 1 (0.8%), “moderate morning:” n = 16 (13.0%), “intermediate:” n = 74 (60.2%), “moderate evening:” n = 29 (23.6%), “definite evening:” n = 3 (2.4%). In the following analysis we analyzed MEQ as a continuous variable.
Main analysis
Performance on the Deary-Liewald task is shown in . Latencies were 138 ms faster in the SRT than the CRT task (SRT: 312 ms; CRT: 450 ms, F(1, 122) = 1,436.82, MSE = 811, p < 0.001, ηp2 = .922, BF10 >1,000) and error rates were 5.1% lower in the SRT than the CRT task (SRT: 0.8%; CRT: 5.9%, F(1, 122) = 190.79, MSE = 8.43, p < 0.001, ηp2 = .610, BF10 >1,000).
Figure 4. Deary-Liewald task. Response latencies (left panel) and error percentages (right panel), separate for “simple reaction time” (SRT) and “choice reaction time” (CRT) tasks.
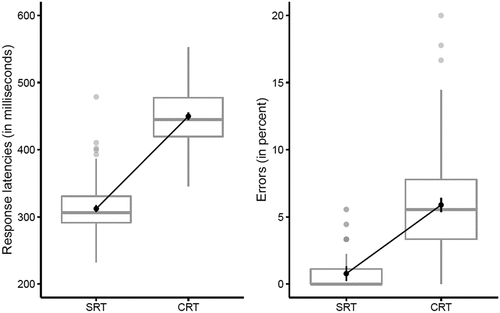
shows a visual representation of z-transformed response latencies for the SRT task (left panel) and CRT task (right panel), with MEQ score of participants on the x-axis, and the three sessions color-coded (morning, noon, and afternoon; see legend. As can be seen in the Figure, the slopes of the regression lines are very close to zero. For the SRT task the correlations between MEQ score and performance measure were not significant and the Bayesian statistics implies “moderate” evidence supporting the null hypothesis, suggesting the absence of synchrony effects on performance. For the CRT data, there appears to be a slight negative slope for lines representing performance in the noon and afternoon sessions. These correlations did not reach significance but the Bayesian statistics lend only “anecdotal” support for a null finding.
Figure 5. Deary-Liewald Task. Processing speed scores (see text), dependent on morningness-eveningness questionnaire score (MEQ; x-axis), task (left: SRT, right: CRT) and session time (morning, noon, afternoon session; see legend). R and p correspond to regression lines and confidence intervals. BF = BF10.

Discussion
The aim of our study was to explore whether consideration of time-of-testing along with an individual’s chronotype is required when designing studies of cognitive control, and particularly when exploring cognitive inhibition. To achieve this, we conducted an online study with young adults which aimed to establish whether a synchrony effect can be found in tasks which measure cognitive inhibition, as well as processing speed. We asked participants to repeatedly complete the Faces task across three times of the day (morning, noon and afternoon). We additionally included the Deary-Liewald task in all testing sessions, which provides a “pure” measure of processing speed with only minimal or no cognitive inhibition. Inclusion was based on Lustig et al.’s (Lustig et al. Citation2007) claim that a synchrony effect will emerge in measures of cognitive inhibition, but that tasks/activities which rely on automatic responses but which require little or no cognitive inhibition should be unaffected. The Faces task has been previously claimed to identify three aspects of cognitive inhibition (Bialystok et al. Citation2006): response suppression (restraint function in Hasher et al. Citation2007, terminology), inhibitory control (access), and task switching (deletion). Our results showed highly significant corresponding main effects of response suppression, inhibitory control, and switching (20, 27, and 18 ms respectively), implying that the task was able to capture the indices that presumably represent the three aspects of cognitive inhibition. Subsequently, we operationalized cognitive inhibition in terms of “interference ratios” (difference scores between the critical and the baseline condition, divided by the baseline condition) which were computed separately for inhibitory control, response suppression, and task switching, and for each testing session. A synchrony effect should emerge as an association between MEQ score and the inhibitory component, such that the directionality of this association (positive vs. negative) depends on the time of testing. However, no such association was found, with no systematic relation between individuals’ chronotype and performance in a given session (see ). No synchrony effect was found in the Deary-Liewald task either. Overall, we failed to find the predicted dissociation between tasks with and without a requirement for cognitive inhibition: both types of tasks were evidently not subject to synchrony effects.
Our results are consistent with studies which had also implemented a repeated-measure design (with each participant being tested at multiple times of the day) and had observed null findings regarding synchrony (e.g., Barclay and Myachykov Citation2017; Matchock and Mordkoff Citation2009) but they are not in line with previous reports of synchrony effects (e.g., Facer-Childs et al. Citation2018; May Citation1999; May and Hasher Citation1998; Ngo and Hasher Citation2017). It is worth exploring possible reasons for why no synchrony effects emerged in our results. A potential concern relates to statistical power. Our final analysis of the Faces task included data from 144 participants, and our main analysis consisted of simple correlations (cf. ). Given our sample size, the power of our study to detect a medium size correlation (r = .3) is .96. Assuming that a synchrony effect is genuine but small (r = .1) then the power of our study is only .22, and it would require 782 participants to achieve a power of .80. Given that in our study a considerable number of participants were excluded from the final analysis (e.g., due to not attending all the critical sessions at the correct times), a sample size in excess of 1,000 participants might be required to detect a genuine but small synchrony effect. This is probably unrealistic to achieve even with online testing. We attempted to guard against Type II errors through the use of Bayesian statistics which also allowed us to identify the strength of evidence against the presence of synchrony effects. In our case, for inhibitory control, response suppression and task switching measures this analysis rendered mostly “moderate” evidence in support of the null hypothesis. Nonetheless it is acknowledged that a genuine but small synchrony effect might be impossible to detect with the statistical power afforded by our study. Of course, the criticism regarding lack of statistical power also applies to many (perhaps most) of the previous studies on synchrony effects in inhibition, as insufficient power inflates not only potential false negatives but also generates false positives (Vankov et al. Citation2014). Previous studies tended to recruit no more than 50 participants per group in between-subject studies (e.g., ~30 young adults per time group in May and Hasher Citation2017, 20 young adults per time group in; Borella et al. Citation2010) or for the entire sample in within-subject studies (e.g., 26 participants in Barclay and Myachykov Citation2017, 34 participants in; Martínez-Pérez et al. Citation2020).
A further aspect of our study which may explain why we found no synchrony effects relates to the recruitment of young adults. In our study, the mean age of participants was approximately 20 years. It is generally agreed among researchers that cognition tends to peak at young adulthood and then steadily declines across middle adulthood to late adulthood (e.g., Deary et al. Citation2009). For that reason, one could argue that our findings reflect young adults’ broad window of optimal performance and in turn, demonstrate their cognitive flexibility. Indeed, May and Hasher (Citation2017) highlighted the cognitive flexibility of young “neutral types”. However, our study suggests this flexibility could be extended to a whole spectrum of chronotypes. Considering our findings, the next logical step would be to explore synchrony effects in inhibition for older adults. We can assume that older adults are less cognitively flexible than young adults and therefore, synchrony effects are more likely to emerge in this age group. Prior research appears to support this possibility (e.g., Borella et al. Citation2010; May and Hasher Citation1998, Citation2017). Therefore, a possibility is that the alignment of an individual’s chronotype and time of testing does not need to be considered when designing studies which compare inhibition performance between two groups of young adults. However, this may not be the case when comparing performance between young and older adults. Then again, it should be considered that the number of studies which have compared synchrony effects between young and older individuals is relatively small. Therefore, future research should replicate our study but recruit older adults.
Alternatively, the absence of a synchrony effect could be explained by our sampling of participants. Unlike in studies reporting positive findings, we chose to not pre-select participants based on their chronotype and we found that more than 60% of our sample is classified as “neutral” according to the conventional categorization. The overall profile of chronotypes in our sample matches the one found in earlier studies regarding young adults (e.g., May and Hasher Citation1998) but a recent study by May and Hasher (Citation2017) found that young “neutral types” are insensitive to synchrony effects. Hence, inclusion of a large proportion of “neutral” chronotypes might make it more difficult to detect a synchrony effect should it exist. A factor which potentially contributes to the lack of participants with “extreme” chronotypes is that the majority (70%) of participants in the Faces task were females. Given that young adults generally show a tendency towards eveningness but females are less likely than males to identify as “evening types” (e.g., Randler and Engelke Citation2019) this could have led to a preponderance of “neutral” chronotypes in our sample. The multi-sessional nature of our study could have additionally deterred individuals with “extreme” chronotypes from participating in our study, or they were more likely not to complete all the critical sessions than “neutral” types. For that reason, our decision to ask participants to attend multiple sessions may have resulted in a sampling bias. It is possible that recruitment of more participants who are classified as a “morning” or “evening type” would have revealed a potential synchrony effect more clearly.
On a broader level, the fact that we recruited undergraduate students for our study might itself have induced a selection bias. University students likely exhibit above-average cognitive control which could have imparted a ceiling effect on cognitive performance, hence curtailing our ability to detect synchrony effects in cognitive inhibition should they exist. Furthermore, chronotype is subject to variation due to genetic, cultural, social, ethnic, environmental and climatic influences (e.g., Randler Citation2008) and the profile exhibited by our participants (young educated individuals residing in the UK) might not generalize to other young adults. Future studies should strive for a sampling method which provides a more representative profile of the target group.
In our study, the “late” (afternoon) sessions occurred between 16:00 h and 17:00 h. It is possible that synchrony effects would have been found with substantially later testing times; for instance, participants categorized as a “definitely evening” type may not have been able to provide their optimal performance between 16:00 h and 17:00 h, but perhaps only later in the evening. Studies on time of day and synchrony effects have implemented large variations in testing times, with afternoon sessions starting after 12:00 h (Murphy et al. Citation2007), taking place between 15:00 h-18:30 h (Intons-Peterson et al. Citation1998), 16:00 h-17:00 h (Yang et al. Citation2007; Yoon et al. Citation1999), or between 16:15 h-17:15 h (Hasher et al. Citation2002), or at 17:00 h (Bugg et al. Citation2006; West et al. Citation2002). We chose to implement a similar timing of sessions to studies which have previously reported significant synchrony effects (e.g., Hasher et al. Citation2002; May and Hasher Citation1998; Yang et al. Citation2007). It is also worth noting that there are prior studies which implemented afternoon/evening sessions later than ours, but which nonetheless failed to detect synchrony effects. For instance, Matchock and Mordkoff (Citation2009) asked participants to complete the ANT task at four time points (08:00 h, 12:00 h, 16:00 h and 20:00 h) and showed that task performance for “evening types” did not differ between the 08:00 h condition and 20:00 h condition. To add to this, considering that in our study morning and afternoon sessions were at least 7 hours apart, we should still expect “morning types” to perform noticeably better in the morning than afternoon session, and vice versa for evening types. Hence, we consider it unlikely that the lack of synchrony effect in our findings can be explained by our choice of session timings. Nevertheless, we acknowledge that future studies should aim to investigate synchrony effects with later testing times.
A further limitation of our study is associated with our sleeping measures. Unlike in most previous studies, we attempted to ensure that lack of sleep did not impact cognitive task performance. Here, we assumed that sleeping for at least five hours the night before a critical session is sufficient for an individual. This measure of sleepiness could be seen as flawed due to individual differences on perceived sufficient hours of sleep (i.e., the optimal number of hours of sleep varies from person to person; Chaput et al. Citation2018). For example, some people may be able to be fully alert with five hours of sleep whereas others require eight hours of sleep. As a consequence, it may be the case that some of our participants have reported sleeping for more than 5 hours but still felt like they had a lack of sleep. Adding to this, we did not ask questions regarding their quality of sleep. Undergoing enough hours of sleep does not necessarily translate into good quality sleep. For example, some participants may have slept for 8 hours but they had disrupted sleep and in turn, did not feel refreshed after waking up. Indeed, poor quality of sleep has been associated with poor cognitive performance (e.g., Della Monica et al. Citation2018; Wilckens et al. Citation2014). Considering these limitations, arguably, we cannot entirely rule out the sleep-related influences in our study. Future studies may consider adding questions which assess a participant’s average and reported number of hours of sleep and their quality of sleep.
Our study was entirely conducted online. A growing number of published web-based studies in the cognition literature (Stewart et al. Citation2017) indicate that web-based studies can reproduce well-established effects including Flanker and Simon (Crump et al. Citation2013). Furthermore, performance of participants tested online does not appear significantly different from the performance of participants tested in the lab (Casler et al. Citation2013; Cyr et al. Citation2021; Germine et al. Citation2012; Semmelmann and Weigelt Citation2017). Anwyl-Irvine et al. (Citation2021) provided a systematic comparison of different web-building platforms (Gorilla, jsPsych, PsychPy etc.) running in a variety of browsers (Chrome, Edge, Firefox, and Safari) and operating systems (macOS and Windows 10) and found reasonable accuracy and precision for display duration and manual response time. We are therefore confident that online testing provides a valid alternative to laboratory-based research, and particularly so in cases where sample size needs to be substantial (as it the case when exploring individual differences, as in the current study). In our task, we excluded participants with overall error rates higher than 25% (N = 19 for the Faces task) and the remaining participants maintained impressive overall response speed (~330 ms) and accuracy (~92%). This level of performance closely mirrors the one in the lab-based study from which our task was adopted (Bialystok et al. Citation2006). Nonetheless, it is acknowledged that with online research, the experimenter has no control over the conditions under which participants carry out the study.
What are the theoretical and practical consequences of the findings of our study? Cognitive inhibition has long been considered a central component of executive functions. Our study, via inclusion of the Faces task which allows a fractionation into various aspects of cognitive inhibition, is generally in line with theories which subdivide cognitive inhibition into components (see Rey-Mermet et al. Citation2018 for overview). The question of whether cognitive inhibition (overall, or in terms of components) is subject to synchrony effects is an important facet of a larger debate on chronobiological effects on cognitive performance (e.g., Schmidt et al. Citation2007). Recently Rabi et al. (Citation2022) advocated that synchrony effects “ … should be considered in routine clinical practice and in research studies examining executive functions such as inhibitory control to avoid misinterpretation of results during improperly timed cognitive assessments.” (15). Contrary to this claim, our findings suggest that effects of time of day and chronotype seem to be of little concern, at least for studies which involve young adults only, and with tasks of the type used here. However, as emphasized throughout, synchrony effects in cognitive inhibition might more clearly emerge in older adults, and for a comparison of performance between young and older participants, an understanding of the interplay between chronotype and time of day might be more relevant.
Conclusion
To summarize, our results suggest that the interplay between chronotype and time- of- testing did not impact young adults’ response suppression, inhibitory control, or task switching, nor their processing speed. Failure to report a synchrony effect in measures of processing speed is in line with prior studies which likewise reported a null finding in tasks which require automatic response, but the lack of synchrony effects in activities which heavily rely on cognitive inhibition contradicts previous research which had suggested effects of this type. For that reason, our findings indicate that researchers may not need to be concerned about these effects when comparing cognitive inhibition and processing speed between groups. Then again, we speculate that the synchrony effect could be genuine but our inability to capture it could be attributed to our recruitment of young adults and the inclusion of a large proportion of “neutral” chronotypes. Future studies may replicate our study but aim to recruit more participants who belong to the “extreme” groups of “morning” and “evening” chronotypes, as well as to recruit older participants and compare their performance to the younger individuals.
Supplemental Material
Download PDF (1.2 MB)Acknowledgments
We would like to thank Wenting Ye, Polly Barr, and Ethan Crossfield for providing their valuable feedback on this manuscript.
Disclosure statement
No potential conflict of interest was reported by the author(s).
Data availability statement
Our interpretation of the data used in this study has been presented at the Behavioural Science Online (BeOnline2020) conference and at the 62nd Annual Meeting of the Psychonomic Society, 2021. The material, data and analysis code used in this study can be accessed on Open Science Framework (https://osf.io/xf5cn/?view_only=36a896247421460886278593c174140e).
Supplementary data
Supplemental data for this article can be accessed online at https://doi.org/10.1080/07420528.2023.2256843.
Additional information
Funding
Notes
1. Deleting data from participants in order to achieve counterbalancing of session order is only necessary if potential learning or practice effects existed across successive sessions. When we re-analysed our data by order of administered session, we found overall RTs of 354 ms for the first block, 321 ms for the second block, and 311 ms for the third block. This suggests that there are indeed substantial learning/practice effects in this task and that therefore session order should be fully counterbalanced.
References
- Anwyl-Irvine A, Dalmaijer ES, Hodges N, Evershed JK. 2021. Realistic precision and accuracy of online experiment platforms, web browsers, and devices. Behav Res Methods. 53:1407–1425. doi:10.3758/s13428-020-01501-5.
- Bailey SL, Heitkemper MM. 2001. Circadian rhythmicity of cortisol and body temperature: morningness-eveningness effects. Chronobiol Int. 18:249–261. doi:10.1081/CBI-100103189.
- Barclay NL, Myachykov A. 2017. Sustained wakefulness and visual attention: moderation by chronotype. Exp Brain Res. 235:57–68. doi:10.1007/s00221-016-4772-8.
- Barner C, Schmid SR, Diekelmann S. 2019. Time-of-day effects on prospective memory. Behav Brain Res. 376:112179. doi:10.1016/j.bbr.2019.112179.
- Barzykowski K, Wereszczyński M, Hajdas S, Radel R. 2021. An inquisit-web protocol for calculating composite inhibitory control capacity score: an individual differences approach. MethodsX. 8:101530. doi:10.1016/j.mex.2021.101530.
- Bedard AC, Nichols S, Barbosa JA, Schachar R, Logan GD, Tannock R. 2002. The development of selective inhibitory control across the life span. Dev Neuropsychol. 21:93–111. doi:10.1207/S15326942DN2101_5.
- Bialystok E, Craik FI, Ryan J. 2006. Executive control in a modified antisaccade task: effects of aging and bilingualism. J Exp Psychol Learn Mem Cogn. 32:1341–1354. doi:10.1037/0278-7393.32.6.1341.
- Borella E, Ludwig C, Dirk J, De Ribaupierre A. 2010. The influence of time of testing on interference, working memory, processing speed, and vocabulary: age differences in adulthood. Exp Aging Res. 37:76–107. doi:10.1080/0361073X.2011.536744.
- Bugg JM, DeLosh EL, Clegg BA. 2006. Physical activity moderates time-of-day differences in older adults’ working memory performance. Exp Aging Res. 32:431–446. doi:10.1080/03610730600875833.
- Cajochen C, Münch M, Knoblauch V, Blatter K, Wirz‐Justice A. 2006. Age‐related changes in the circadian and homeostatic regulation of human sleep. Chronobiol Int. 23:461–474. doi:10.1080/07420520500545813.
- Carpenter SM, Chae RL, Yoon C. 2020. Creativity and aging: positive consequences of distraction. Psychol Aging. 35:654–662. doi:10.1037/pag0000470.
- Casler K, Bickel L, Hackett E. 2013. Separate but equal? A comparison of participants and data gathered via Amazon’s MTurk, social media, and face-to-face behavioral testing. Comput Human Behav. 29:2156–2160. doi:10.1016/j.chb.2013.05.009
- Chaput JP, Dutil C, Sampasa-Kanyinga H. 2018. Sleeping hours: what is the ideal number and how does age impact this? Nat Sci Sleep. 10:421–430. doi:10.2147/NSS.S163071.
- Crump MJ, McDonnell JV, Gureckis TM, Gilbert, S. 2013. Evaluating Amazon’s mechanical turk as a tool for experimental behavioral research. PloS One. 8(3):e57410. doi:10.1371/journal.pone.0057410.
- Cyr AA, Romero K, Galin-Corini L. 2021. Web-based cognitive testing of older adults in person versus at home: within-subjects comparison study. JMIR Aging. 4:e23384. doi:10.2196/23384.
- Deary IJ, Corley J, Gow AJ, Harris SE, Houlihan LM, Marioni RE, Starr JM, Rafnsson SB, Starr JM. 2009. Age-associated cognitive decline. Br Med Bull. 92:135–152. doi:10.1093/bmb/ldp033.
- Deary IJ, Liewald D, Nissan J. 2011. A free, easy-to-use, computer-based simple and four-choice reaction time programme: the Deary-Liewald reaction time task. Behav Res Methods. 43:258–268. doi:10.3758/s13428-010-0024-1.
- Della Monica C, Johnsen S, Atzori G, Groeger JA, Dijk DJ. 2018. Rapid eye movement sleep, sleep continuity and slow wave sleep as predictors of cognition, mood, and subjective sleep quality in healthy men and women, aged 20-84 years. Front Psychiatry. 9:255. doi:10.3389/fpsyt.2018.00255.
- Diamond A. 2013. Executive functions. Ann Rev Psychol. 64:135–168. doi:10.1146/annurev-psych-113011-143750.
- Facer-Childs ER, Boiling S, Balanos GM. 2018. The effects of time of day and chronotype on cognitive and physical performance in healthy volunteers. Sports Medicine-Open. 4:1–12. doi:10.1186/s40798-018-0162-z.
- Germine L, Nakayama K, Duchaine BC, Chabris CF, Chatterjee G, Wilmer JB. 2012. Is the web as good as the lab? Comparable performance from web and lab in cognitive/perceptual experiments. Psychon Bull Rev. 19:847–857. doi:10.3758/s13423-012-0296-9.
- Hasher L, Chung C, May CP, Foong N. 2002. Age, time of testing, and proactive interference. Can J Exp Psychol/Revue canadienne de psychologie expérimentale. 56:200. doi:10.1037/h0087397.
- Hasher L, Lustig C, Zacks RT. 2007. Inhibitory mechanisms and the control of attention. In: Conway A, Jarrold C, Kane M Towse J, editors. Variation in working memory. New York: Oxford University Press. p. 227–249. doi:10.1093/acprof:oso/9780195168648.003.0009.
- Hoddes E, Zarcone V, Smythe H, Phillips R, Dement WC. 1973. Quantification of sleepiness: a new approach. Psychophysiology. 10:431–436. doi:10.1111/j.1469-8986.1973.tb00801.x
- Horne JA, Östberg O. 1976. A self-assessment questionnaire to determine morningness-eveningness in human circadian rhythms. Int J Chronobiol. 4:97–110.
- Horne JA, Östberg O. 1977. Individual differences in human circadian rhythms. Biol Psychol. 5:179–190. doi:10.1016/0301-0511(77)90001-1.
- Intons-Peterson MJ, Rocchi P, West T, McLellan K, Hackney A. 1998. Aging, optimal testing times, and negative priming. J Exp Psychol Learn Mem Cogn. 24:362. doi:10.1037/0278-7393.24.2.362.
- Intons-Peterson MJ, Rocchi P, West T, McLellan K, Hackney A. 1999. Age, testing at preferred or nonpreferred times (testing optimality), and false memory. J Exp Psychol Learn Mem Cogn. 25:23–40. doi:10.1037/0278-7393.25.1.23.
- Lara T, Madrid JA, Correa Á, van Wassenhove V. 2014. The vigilance decrement in executive function is attenuated when individual chronotypes perform at their optimal time of day. PloS One. 9:e88820. doi:10.1371/journal.pone.0088820.
- Lehmann CA, Marks AD, Hanstock TL. 2013. Age and synchrony effects in performance on the Rey auditory verbal learning test. Int Psychogeriatr. 25:657–665. doi:10.1017/S1041610212002013.
- Levandovski R, Sasso E, Hidalgo MP. 2013. Chronotype: a review of the advances, limits and applicability of the main instruments used in the literature to assess human phenotype. Trends Psychiatry Psychother. 35:3–11. doi:10.1590/s2237-60892013000100002.
- Lustig C, Hasher L, Zacks RT. 2007. Inhibitory deficit theory: Recent developments in a “new view. In: Gorfein DS MacLeod CM, editors. Inhibition in cognition. American Psychological Association. p. 145–162. doi:10.1037/11587-008.
- Martínez-Pérez V, Palmero LB, Campoy G, Fuentes LJ. 2020. The role of chronotype in the interaction between the alerting and the executive control networks. Sci Rep. 10:1–10. doi:10.1038/s41598-020-68755-z.
- Matchock RL, Mordkoff JT. 2009. Chronotype and time-of-day influences on the alerting, orienting, and executive components of attention. Exp Brain Res. 192:189–198. doi:10.1007/s00221-008-1567-6.
- May CP. 1999. Synchrony effects in cognition: the costs and a benefit. Psychon Bull Rev. 6:142–147. doi:10.3758/BF03210822.
- May CP, Hasher L. 1998. Synchrony effects in inhibitory control over thought and action. J Exp Psychol Hum Percept Perform. 24:363–379. doi:10.1037/0096-1523.24.2.363.
- May CP, Hasher L. 2017. Synchrony affects performance for older but not younger neutral-type adults. Timing Time Percept. 5:129–148. doi:10.1163/22134468-00002087.
- May CP, Hasher L, Stoltzfus ER. 1993. Optimal time of day and the magnitude of age differences in memory. Psychol Sci. 4:326–330. doi:10.1111/j.1467-9280.1993.tb00573.x.
- Maylor EA, Badham SP. 2018. Effects of time of day on age-related associative deficits. Psychol Aging. 33:7–16. doi:10.1037/pag0000199
- Murphy KJ, West R, Armilio ML, Craik FI, Stuss DT. 2007. Word-list-learning performance in younger and older adults: intra-individual performance variability and false memory. Aging Neuropsychol Cogn. 14:70–94. doi:10.1080/138255890969726.
- Nebel LE, Howell RH, Krantz DS, Falconer JJ, Gottdiener JS, Gabbay FH. 1996. The circadian variation of cardiovascular stress levels and reactivity: relationships to individual differences in morningness/eveningness. Psychophysiology. 33:273–281. doi:10.1111/j.1469-8986.1996.tb00424.x
- Ngo KJ, Hasher L. 2017. Optimal testing time for suppression of competitors during interference resolution. Memory. 25:1396–1401. doi:10.1080/09658211.2017.1309437.
- Rabi R, Chow R, Paracha S, Hasher L, Gardner S, Anderson ND, Alain C. 2022. The effects of Aging and time of day on inhibitory control: an event-related potential study. Front Aging Neurosci. 14:821043. doi:10.3389/fnagi.2022.821043.
- Randler C. 2008. Morningness-eveningness comparison in adolescents from different countries around the world. Chronobiol Int. 25:1017–1028. doi:10.1080/07420520802551519.
- Randler C, Engelke J. 2019. Gender differences in chronotype diminish with age: a meta-analysis based on morningness/chronotype questionnaires. Chronobiol Int. 36:888–905. doi:10.1080/07420528.2019.1585867.
- R Core Team. 2021. R: a language and environment for statistical computing. Vienna, Austria: R Foundation for Statistical Computing. https://www.R-project.org/.
- Rey-Mermet A, Gade M, Oberauer K. 2018. Should we stop thinking about inhibition? Searching for individual and age differences in inhibition ability. J Exp Psychol Learn Mem Cogn. 44:501. doi:10.1037/xlm0000450.
- Richards A, Kanady JC, Huie JR, Straus LD, Inslicht SS, Levihn‐Coon A, Neylan TC, Neylan TC. 2020. Work by day and sleep by night, do not sleep too little or too much: effects of sleep duration, time of day and circadian synchrony on flanker‐task performance in internet brain‐game users from teens to advanced age. J Sleep Res. 29:e12919. doi:10.1111/jsr.12919.
- Roenneberg T, Kuehnle T, Juda M, Kantermann T, Allebrandt K, Gordijn M, Merrow M. 2007. Epidemiology of the human circadian clock. Sleep Med Rev. 11:429–438. doi:10.1016/j.smrv.2007.07.005.
- Rothen N, Meier B. 2016. Time of day affects implicit memory for unattended stimuli. Conscious Cogn. 46:1–6. doi:10.1016/j.concog.2016.09.012.
- Schmidt C, Collette F, Cajochen C, Peigneux P. 2007. A time to think: circadian rhythms in human cognition. Cogn Neuropsychol. 24:755–789. doi:10.1080/02643290701754158.
- Schmidt C, Peigneux P, Cajochen C, Collette F. 2012a. Adapting test timing to the sleep-wake schedule: effects on diurnal neurobehavioral performance changes in young evening and older morning chronotypes. Chronobiol Int. 29:482–490. doi:10.3109/07420528.2012.658984.
- Schmidt C, Peigneux P, Leclercq Y, Sterpenich V, Vandewalle G, Phillips C, Collette F, Berthomier C, Tinguely G, Gais S. 2012b. Circadian preference modulates the neural substrate of conflict processing across the day. PloS One. 7:e29658. doi:10.1371/journal.pone.0029658.
- Semmelmann K, Weigelt S. 2017. Online psychophysics: reaction time effects in cognitive experiments. Behav Res Methods. 49:1241–1260. doi:10.3758/s13428-016-0783-4.
- Singmann H, Bolker B, Westfall J, Aust F. 2016. Afex: analysis of factorial experiments. R Package Version 0.1-145. doi:10.1080/02643290701754158.
- Song J, Stough C. 2000. The relationship between morningness–eveningness, time-of-day, speed of information processing, and intelligence. Pers Indiv Differ. 29:1179–1190. doi:10.1016/S0191-8869(00)00002-7.
- Stewart N, Chandler J, Paolacci G. 2017. Crowdsourcing samples in cognitive science. Trends Cogn Sci. 21:736–748. doi:10.1016/j.tics.2017.06.007.
- Vandierendonck A. 2017. A comparison of methods to combine speed and accuracy measures of performance: a rejoinder on the binning procedure. Behav Res Methods. 49:653–673. doi:10.3758/s13428-016-0721-5.
- Vankov I, Bowers J, Munafò MR. 2014. Article commentary: on the persistence of low power in Psychological Science. Q J Exp Psychol. 67:1037–1040. doi:10.1080/17470218.2014.885986.
- West R, Murphy KJ, Armilio ML, Craik FI, Stuss DT. 2002. Effects of time of day on age differences in working memory. J Gerontol B. 57:3–P10. doi:10.1093/geronb/57.1.P3.
- Wilckens KA, Woo SG, Kirk AR, Erickson KI, Wheeler ME. 2014. Role of sleep continuity and total sleep time in executive function across the adult lifespan. Psychol Aging. 29:658–665. doi:10.1037/a0037234.
- Yang L, Hasher L, Wilson DE. 2007. Synchrony effects in automatic and controlled retrieval. Psychon Bull Rev. 14:51–56. doi:10.3758/BF03194027.
- Yoon C, May CP, Hasher L. 1999. Aging, circadian arousal patterns, and cognition. In: Park D, Schwartz N, Knaüper, B., Sudman, S., editors. Cognition, aging, and self-reports. Psychology Press/Erlbaum (UK) Taylor & Francis. p. 117–143.