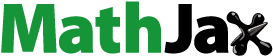
ABSTRACT
Some people contribute ideas for prosocial reasons in crowdsourcing; others do so for selfish reasons. Extending the theory of motivated information processing, the research posits that prosocial and proself individuals respond differently to reward structures in crowd idea generation. Two online experiments measured participants’ prosocial versus proself orientation and manipulated whether participants received a competitive or cooperative reward structure. Study 2 also manipulated whether participants viewed an original or a common peer idea. Proselfs produced more ideas when receiving competitive rewards; the idea generation of prosocials was not affected by the reward structure. This interaction effect was mediated by task effort and moderated the impact of peer ideas. Proselfs generated the most ideas when viewing an original peer idea and receiving competitive rewards; this effect was not observed for prosocials. The study contributes to crowdsourcing research by demonstrating that participants’ response to reward structures depends on their social value orientation. The implication is that crowdsourcing organizers should design tasks and rewards so they motivate participants with both prosocial and proself orientations.
Introduction
From software development [Citation73], new product design [Citation46] to public policy-making [Citation64], organizations are leveraging the creativity of crowds through crowdsourcing [Citation75]. Crowdsourcing is a production model that outsources an internal task or problem to a large number of distributed individuals through an open call in a digital space [Citation18, Citation46]. The model differentiates itself from traditional organization-led innovation models as it is open and large in scale. Therefore, crowdsourcing projects typically lack formal incentive systems and are sustained by volunteers who contribute their ideas. Research has demonstrated that people differ in their social value orientation (SVO) or motivation for contributing their ideas online: while some individuals are proself, primarily contributing for personal gain, others are prosocial, or driven by social-relational reasons such as collective good and altruism [Citation12, Citation34, Citation37, Citation40, Citation47, Citation61].
However, despite this demonstration, much research and practice implicitly treat self-interest as the dominant motivation for users’ online contributions. This is evident in the general popularity of tournaments and competitions across a wide range of online contexts. As an example, crowd contests, which have attracted considerable attention in crowdsourcing research [Citation3, Citation10, Citation14, Citation21, Citation45, Citation75], remain the most prevalent design. Since crowd contests cultivate competition and reward the best performers, implied in this common practice is that online participants are proself-oriented, motivated to maximize their personal gains and invigorated when competing with others. The effects of reward structures on prosocial-oriented participants have not been sufficiently investigated in previous research. Our study proposes that participants’ SVO (i.e., prosocial versus proself) will interact with environmental factors such as the reward structure to impact their performance in crowdsourcing.
Mixed findings exist regarding the impact of reward structures on participants’ online behavior. On the one hand, much research has supported the general positive effect of crowd contests and individual rewards on crowd contributions and performance [Citation13, Citation20, Citation50]. On the other hand, several studies have shown that external incentives such as monetary rewards can be detrimental to participants’ voluntary contributions online, particularly if their goal is prosocial [Citation27, Citation64, Citation76]. This inconsistency may be partially due to the different definitions and measures used to study prosocial and proself orientation.
Extending the theory of motivated information processing [Citation22, Citation53], this research probes the discrepancies in the existing literature and theorizes the motivation mechanisms of crowd behavior. We clarify individuals’ prosocial or proself motivation as a fundamental dispositional attribute—SVO, or preference regarding outcome distributions between oneself and others [Citation4, Citation8, Citation22]. Prosocials act to benefit others and/or collective outcomes. Self-interested individuals (proselfs), in contrast, act to benefit themselves and tend to focus on their relative gain compared to others. SVO is a stable trait across contexts [Citation8, Citation52]. The trait determines how individuals process social information related to their gains [Citation22, Citation53], therefore shaping how people respond to different reward structures.
We tested our proposition in two online crowdsourcing experiments in which participants engaged in a simulated crowdsourcing idea generation task. We measured participants’ SVO and then experimentally varied the reward structure in both studies. In Study 2, we also manipulated participants’ exposure to the ideas of other crowdsourcing participants. We found that proselfs invested more effort and had higher performance with competitive rewards than cooperative rewards. In contrast, the effort and performance of prosocials were not significantly affected by the reward structure. The results underscore the necessity for crowdsourcing research and practice to further study and address the difference in participants’ SVO since crowdsourcing will be most effective if participants are offered incentives that match their underlying disposition. While online crowdsourcing platforms may continue to offer competitive rewards to motivate proself participants, they should experiment with other incentive schemes to inspire the performance of prosocials.
Literature Review: Reward Structures and Crowd Performance
Much research on crowd behavior has examined the effects of reward structures on crowds’ contribution and performance [Citation3, Citation9, Citation13, Citation69]. Since crowdsourcing contests represent one of the most commonly applied task designs in crowdsourcing, the impact of competitive rewards on participant performance has been extensively studied. Many have found that competitive rewards, which offer individual incentives by rewarding the best performers, stimulate participants’ effort and performance [Citation13, Citation20, Citation50]. However, the impact of competitive rewards was not linear: competitive rewards were more effective in incentivizing participants’ performance when participants favored gains rather than minimizing losses [Citation77] and when the competitors were high performers [Citation75]. This evidence points to potential moderators of rewards’ influence on participants’ online behavior. Nonetheless, despite the wide acceptance that people vary in whether they participate online for prosocial or proself reasons [Citation15, Citation34, Citation40, Citation46, Citation62], how competitive rewards may affect the behavior of prosocial participants differently from that of proselfs has not yet been investigated.
Moreover, several studies showed that individual incentives may generate a crowding-out effect and demotivate voluntary contributions [Citation23, Citation27, Citation64, Citation76]. This is because when people contribute voluntarily, they receive emotional and other intrinsic benefits for behaving prosocially [Citation2]. Yet being compensated for voluntary contributions may dampen the positive emotions that participants experience, thus discouraging them from making contributions. When participants contribute for rewards, however, they may exert less effort when competition rises since they expect lower chances to be rewarded [Citation69], especially when they have a low skill level [Citation11] and the task is certain (such as a math task with a correct solution) [Citation9]. Underlying these investigations is the assumption that individuals are motivated by self-interest even when they behave prosocially. They make careful evaluations of their payoffs when participating online and are only motivated to contribute when they feel they can gain something from their contribution.
This brief review suggests that current research has not fully embraced the dispositional difference in participants’ SVO and still tends to view participants as dominated by the pursuit of self-interest. As a result, how reward structures influence participants with different SVOs are still unclear. In particular, how commonly applied task designs such as crowd contests affect the behavior of prosocial participants has not been fully investigated.
To fill the gap in the existing literature, we present the general proposition that the impact of reward structures on online idea generation depends on participants’ SVO such that variation in reward structures influences the behavior of prosocial and proself participants differently. This proposition is grounded in the theory of motivated information processing and is tested in a problem-solving crowdsourcing context. Next, we will develop our theoretical framework and articulate our hypotheses.
SVO, Reward Structure, and Crowd Idea Generation
SVO and Motivated Information Processing
SVO characterizes whether individuals are motivated more by self or collective outcomes [Citation4, Citation22, Citation52]. The construct is also known as social motives or social motivation. It was conceptualized in early experimental research utilizing behavioral games, where researchers found that some individuals were always more cooperative than others across different types of social dilemmas [Citation54]. After more than 50 years of research, scholars have established that SVO is a disposition that is stable across time and contexts [Citation4, Citation8]. Rudimentary forms of SVO can be observed in early childhood [Citation43]. Research in neural science has found that individuals with prosocial and proself orientations demonstrate different patterns of brain activity [Citation30], further supporting the stability of the trait. People with prosocial orientation (prosocials) cooperate more than those with proself orientation (proselfs) in different contexts, even when non-cooperation brings clear personal benefits [Citation4, Citation8, Citation48, Citation52]. In other words, people may behave altruistically because they are predisposed to help rather than because they would like to gain something from their behavior or make themselves feel good.
Despite its stability, SVO was shown to interact with environmental factors to influence behavior [Citation4, Citation42, Citation71]. Prosocials and proselfs respond differently to task interdependence [Citation48], managerial control and group identification [Citation26], and the framing of social issues [Citation71]. For example, Galletta and colleagues [Citation26] found that group identification and managerial control were more effective in promoting knowledge contribution from proselfs than from prosocials. These findings suggest that features of crowdsourcing tasks, including reward structure, may impact prosocial and proself participants differently.
Motivated Information Processing
An important theoretical perspective to explain prosocial and proself’s different responses to environmental cues is the theory of motivated information processing [Citation22, Citation53]. This theory posits that since SVO determines what people care about in social settings, it drives individuals’ information search and processing in social interactions. Because proselfs are interested in boosting their individual outcomes or outperforming others more than prosocials, they are more likely to attend to and evaluate information related to personal costs and benefits [Citation22, Citation38, Citation44, Citation53, Citation71].
In contrast, prosocials tend to pay little attention to environmental cues affecting their gains than proselfs. Research shows the effort and behavior of prosocials are less sensitive to rewards than proselfs. Prosocials contribute consistently high effort to tasks regardless of reward types [Citation4, Citation8]. In public goods dilemmas, prosocials took less from the shared resource than proselfs regardless of the resource size [Citation41]. After receiving negative supervisor feedback, prosocials reduced their job commitment less than proselfs because negative feedback was indicative of lower rewards [Citation44].
Interaction Effects of SVO and Reward Structure
Extending the theory of motivated information processing to understand crowd behavior, we propose that SVO and reward structure jointly determine crowd idea generation performance. Crowdsourced tasks are often structured as contests on crowdsourcing platforms [Citation55]. Crowdsourcing contests offer competitive rewards (e.g., money, and/or community reputation) for the best performer. Popular crowdsourcing platforms such as Innocentive and Kaggle represent prominent examples of platforms hosting crowdsourcing contests.
Although less popular, cooperative crowdsourcing tasks also exist. They are often organized as communities in which participants are encouraged to work collaboratively and sometimes rewarded collectively [Citation51]. These communities typically consist of consumers who voluntarily share and discuss their product development suggestions. When an idea generated by the community is adopted, the whole group can be considered as receiving a collective reward. Examples of cooperative crowdsourcing platforms include Dell’s IdeaStorm and Starbucks’ MyStarbucksIdea.com (no longer exists). Still others combine both competitive and cooperative features by hosting occasional contests in collaborative communities (e.g., Lego Ideas).
The variation in reward structure may alter how and what each participant will gain based on their contribution. In crowdsourcing contests, participants are competitors and the highest performers earn rewards. In collaborative communities, participants work together and help organizations develop their products, which usually do not generate direct personal benefits. Thus, reward structure is a central feature in crowdsourcing that may interact with participants’ SVO to impact participant performance.
We predict that changes in crowdsourcing reward structures are more likely to impact the idea generation performance of proselfs than of prosocials. As suggested by the motivated information processing theory, proselfs value individual gains and are sensitive to external rewards, whereas prosocials have concern for others and indifference to individual rewards [Citation4]. Thus, competitive rewards, compared to cooperative rewards, are likely to be more motivating to proselfs’ idea generation performance. Research has shown that in cooperative settings, proselfs are less invigorated by collaborating with others than prosocials and need additional incentives before they will help others [Citation26, Citation41]. Competitive rewards that cultivate rivalry and compensate individual achievement should act as an extra incentive for proselfs to work harder and produce more ideas. As support, proself groups generated more ideas in brainstorming when the task emphasized individual performance-based rewards instead of equal distribution rewards [Citation28]. However, the change in reward structure did not affect the idea generation performance of prosocial groups. Taken together, we propose:
Hypothesis 1 (H1): SVO moderates the effect of reward structures on crowd performance such that proselfs generate more ideas when receiving competitive rewards than cooperative rewards. This reward-structure effect will not be observed for prosocials.
We further postulate that task effort will mediate the joint effect of SVO and reward structure on idea generation performance. As discussed earlier, crowdsourcing research found that reward structure is related to task effort [Citation9]. Evidence from SVO research suggests that the disposition and reward structure may together influence participants’ task effort: after being rewarded for high effort, proselfs became more persistent on competitive tasks [Citation24]. Since task effort leads to higher performance in idea generation tasks [Citation58], we thus propose:
Hypothesis 2 (H2): The interaction effect of SVO and reward structure on crowd performance is mediated by task effort.
Moderating Effect of Peer Ideas
The joint impact of SVO and reward structure is likely to moderate the influence of other environmental factors on crowd idea generation. In crowdsourcing, participants are often exposed to ideas generated by other participants or “peers” [Citation16]. Exposure to peer ideas can have a priming effect on individuals and may stimulate creativity by providing memory cues that reduce the cognitive effort for idea search, combination, and generation [Citation19, Citation59]. When participants could view the ideas of other participants, they generated more ideas and their ideas were more creative [Citation3, Citation74]. Disclosing solutions and progress during crowdsourcing contests, as opposed to after the contest ends, helped participants improve their solutions [Citation10]—the exchange saved time for participants to seek better solutions and enhanced the overall collective performance, although it reduced experimentation and decreased the diversity in final solutions.
The quality of peer ideas that participants view matters [Citation39, Citation49]—original ideas that rarely occur, compared to common ideas that are frequently generated, tend to stimulate the most creativity [Citation51, Citation60, Citation72, Citation73]. Creative synergy can happen in group interaction if members can prime one another to come up with ideas that they cannot think of when brainstorming alone [Citation60]. Original ideas can serve as a stimulus to search for more uncommon information, fostering creative synergy among different participants. Research on crowdsourcing has shown that participants produced more creative ideas if others’ ideas they see were highly original [Citation3, Citation74]. Crowds were more creative if their members were highly diverse [Citation35], likely because members were exposed to more original ideas, which in turn inspired novel idea integration and generation.
However, the influence of peer ideas may be contingent: drawing on the theory of motivated information processing, we propose that the impact of peer ideas on participants’ idea generation depends on participants’ SVO and reward structure. To begin with, the effect of original peer ideas on proselfs’ idea generation may depend on the reward structure. The stimulating effect of original peer ideas on idea generation can only occur when participants cognitively process others’ ideas. Driven by self-interest, proselfs focus their attention on information that affects their individual gains [Citation38, Citation53]. They will pay less attention to others’ ideas than prosocials if no incentives are provided. Nonetheless, competition may make proselfs more mindful of others’ ideas than cooperation. When rewards are competitive, idea generation performance is compared across participants to determine the best performer. Therefore, others’ ideas have a direct impact on participants’ rewards. So proselfs in a competition should be more likely to pay attention to others’ ideas than in cooperation, which in turn enhances the positive stimulation of original peer ideas on their idea generation.
For prosocials, however, the positive influence of original peer ideas should be consistent across reward structures. Prosocials were more likely than proselfs to take others’ perspectives and find common ground to produce novel ideas even when competition and disagreement existed [Citation29]. In negotiation, prosocials used more cooperative heuristics and searched for integrative agreements that lead to better joint outcomes. In studies of organizational teams, minority dissent and team diversity were positively associated with team creativity and innovation only when members were prosocial-oriented [Citation67]. Prosocial-oriented group interaction (e.g., adding perspectives rather than arguing) is also found to facilitate knowledge integration and idea generation among participants in crowdsourcing [Citation3, Citation66]. Together, existing evidence suggests that prosocials should pay close attention to others’ ideas regardless of the rewards. Thus, the stimulating effect of original peer ideas on prosocials will not be affected by the structure of rewards. Therefore, we propose a three-way interaction effect of SVO, reward structure, and peer ideas on participants’ idea generation in crowdsourcing:
Hypothesis 3 (H3): SVO and reward structure together moderate the effect of peer ideas on crowd performance such that competitive (vs. cooperative) rewards enhance the positive impact of original peer ideas on the idea generation of proselfs but not of prosocials.
Method
To test our hypotheses, we conducted two online experiments using the crowdsourcing platform, Amazon Mechanical Turk (MTurk). In the experiments, participants performed an idea generation task highly relevant to their experience on the MTurk platform. We measured participants’ SVO at the beginning of the experiments and varied the reward structure. Study 1 investigated the interaction effect of SVO and reward structure on idea generation (H1). In Study 2, we measured task effort and examined it as a mediator of the interaction effect of SVO and reward structure (H2). We also manipulated peer idea exposure to test the moderating effect of SVO and reward structure on the influence of peer ideas (H3).
Study 1: SVO and Reward Structure
Participants
One hundred and seventy-six participants completed the study. Among them, 39% were male, 64% were between 25 and 44 years old, and 90% had at least some college education. Participants joined the study on MTurk through a Human Intelligence Task (HIT) for a payment of $0.75. Only participants with high reputation scores were invited to the study: that is, an approval rating of above 95% and completion of at least 100 HITs in the past.
Design and Procedure
The online experiment followed a 2 (SVO: Prosocial vs. Proself) x 2 (Reward structure: Competitive vs. Cooperative) between-subjects factorial design. Participants who accepted the HIT on MTurk were directed to Qualtrics, where they first signed informed consent and responded to the Slider Measure of SVO [Citation56]. Second, they read the reward structure manipulation as a task instruction. Participants then generated ideas. After finishing the idea generation task, participants responded to a post-experiment survey that asked about task effort, enjoyment and demographic information. Finally, they were debriefed and received a code to claim their payment in MTurk.
SVO
SVO was measured using a web-based version of the Slider Measure [Citation56, Citation57], which was developed from the decomposed game widely used in SVO research [Citation4]. The reliability and validity of the measure have been repeatedly shown in decades of research [Citation4, Citation8]. In particular, studies have shown that SVO measured by decomposed games was highly consistent when measured in different time periods [Citation8], regardless of task cooperativeness and competitiveness [Citation31] and was robust against social desirability bias [Citation43, Citation63].
Participants answered six questions regarding their choice of resource distribution between themselves and a hypothetical other. The complete measure is in the Online Supplemental Appendix. An SVO score was then calculated based on their answers following Equation (1), where Ao is the number of resources that participants assign to others, and As is the number that they assign to themselves
Following Murphy and colleagues [Citation56, Citation57], participants who scored higher than 22.45 were classified as prosocials, while the rest were proselfs. This is because given the design of the measure, individuals who choose to maximize joint gains, reduce unequal resource allocation, and maximize others’ gains — have attributes characteristic of a prosocial orientation—will obtain a score higher than 22.45. Individuals who choose to maximize their own gains and reduce others’ relative gains — have attributes characteristic of a proself orientation — will obtain a score equal to or lower than 22.45. Of the 176 participants, we found more prosocials (107) than proselfs (69).
Task
The idea generation task performed by the participants was: “What can MTurk requesters do to ensure workers perform their HITs with good quality?” This task resembles the sort of task commonly used for crowdsourcing, which typically solicits ideas from users to develop or improve products or services [Citation32]. It is also relevant to the actual experience of our participant pool, MTurkers [Citation18].
Reward Structure
The manipulation of reward structure followed a long tradition in group research [Citation6, Citation17, Citation28, Citation65] that either rewards group members equally (a cooperative reward structure) or differently based on individual performance (a competitive reward structure). This manipulation has been applied in different group tasks and shown to effectively induce a cooperative versus a competitive context: group members receiving cooperative rewards reported higher willingness to work with others than those receiving competitive rewards [Citation6], whereas members receiving competitive rewards showed stronger tendency to outperform others and win bigger rewards [Citation17, Citation28]. Following this stream of research, participants were randomly assigned to receive either a competitive or cooperative reward. In both conditions, they were grouped into batches of 9. The grouping follows the tradition on MTurk to mimic task performance on the platform because task batches with more than 9 participants are charged additional service fees. This grouping also creates smaller participant groups and facilitates our reward structure manipulation (described in more detail below). As in most crowdsourcing tasks, no restriction was posed on how long participants could work on the task.
Participants receiving a competitive reward were told that they were competing with 8 other workers in their group to win a task bonus:
You will compete in groups to generate ideas to answer a question posted by the requester. The HIT you are completing is grouped into batches of 9 assignments. You will compete with 8 other workers who accept the same batch to get the chance to earn a $15 bonus. If you generate the most non-redundant ideas in your batch, you will enter a lottery to win the bonus after the HIT is completed. For each non-redundant idea you generate, you get one chance in the lottery for the bonus. The more non-redundant ideas you generate, the more likely you are to receive the bonus.
Participants receiving a cooperative reward were told that they were collaborating with 8 other workers in their group to win a task bonus:
You will collaborate in groups to generate ideas to answer a question posted by the requester. The HIT you are completing is grouped into batches of 9 assignments. You will collaborate with eight other workers who accept the same batch to get the chance to earn a $15 bonus. You will enter a lottery for the bonus after the HIT is completed. For each non-redundant idea your batch generates, you get one chance in the lottery for the bonus. The more non-redundant ideas your batch collectively generates, the more likely you and other workers in your batch are to receive the bonus.
In each task condition, the participant was randomly selected based on the stated rule and rewarded a $15 bonus after the study was completed.
Manipulation Check
Participants answered a multiple-choice question after the task instructions to make sure they understood the manipulation:
On this task, I will: a) generate non-redundant ideas to win a $5 bonus; b) collaborate with my group of workers to generate non-redundant ideas and win a $15 bonus; c) compete with my group of workers to generate non-redundant ideas and win a $15 bonus; d) evaluate others’ ideas to win a $10 bonus.
Only participants who correctly answered this question (87.3%) were included in the analysis.
Measures
Idea Generation Performance
Based on prior research [Citation6, Citation28, Citation60], the number of non-redundant ideas served as the measure of idea generation. Two ideas were considered redundant if they had the same meaning. For example, “pay more” and “increase the payment” were considered redundant and were counted only once.
Covariates
The first covariate, participation time, was gauged as the total time spent on the study (i.e., the system recorded length of time in minutes). Since we conducted an online experiment, the variable was included in the analysis to control for participants’ variance in their participating time. We reasoned that participants who perceive the task as relevant may have more knowledge on the topic and enjoy generating ideas. As a result, in the post-experiment survey, we also measured the task’s relevance to the participants using a 7-point Likert scale ranging from strongly disagree to strongly agree. The scale was “In general, I found the question posted by the requester relevant to me.” One demographic variable, age, was also included as a covariate since older people may have more knowledge and experience. Age was measured by a 1-6 ordinal variable ranging from 18-24 years to 65 years or more.
Results
On average, each participant produced 6.23 ideas (SD = 3.59). Prosocials and proselfs did not significantly differ in the number of ideas generated. Participants reported the task to be highly relevant to them (M = 5.60, SD = 1.33) and spent an average of 12.74 minutes on the task (SD = 7.69). The descriptive statistics and correlation matrix are presented in .
Table 1. Study 1 descriptive statistics and correlations (n=176).
To test H1, we began with an omnibus analysis of covariance (ANCOVA) and then conducted planned contrasts to assess the hypothesized patterns. Unlike post-hoc comparisons, planned contrasts provide a direct test of customized patterns of difference among the experimental conditions [Citation25]. It thus has more statistical power than omnibus tests and is more appropriate if we need to test a specific pattern derived from theory. Planned contrasts can be used to test the hypothesized patterns even when the omnibus test is non-significant. However, to be conservative, we followed the common practice and started with the ANCOVA test.
First, a 2 (SVO: prosocial vs. proself) X 2 (Reward structure: competitive vs. cooperative) ANCOVA was conducted on idea generation performance, controlling for participation time, task relevance, and age. Consistent with prior research [Citation13, Citation20], the ANCOVA revealed a significant main effect of reward structure on idea generation, F(1,169) = 4.18, p < .05, ηp2 = .02. Participants produced more ideas when receiving a competitive (M = 7.06, SD = 3.94) than a cooperative reward (M = 5.52, SD = 3.10). There was no statistically significant difference between the idea generation performance of prosocials and proselfs (Prosocials: M = 6.37 SD = 3.57 vs. Proselfs: M = 6.00, SD = 3.62), F(1,169) = 0.77, p = .77.
H1 predicted a moderation effect of SVO on the impact of reward structure, such that proselfs generate more ideas if receiving competitive than cooperative rewards, but that the decline in idea generation under cooperative rewards would not be observed for prosocials. In support of H1, there was a statistically significant interaction effect of SVO and reward structure on idea quantity, F(1,169) = 4.43, p < .05, ηp2 = .03.
Since the omnibus test cannot reveal the specific patterns of between-group differences, we next applied a planned contrast analysis to test our hypothesized pattern of difference among our experimental conditions. We predicted that 1) prosocials will generate many ideas regardless of the reward structure and 2) proselfs will generate more ideas when receiving a competitive reward (vs. a cooperative reward). The means of each experimental condition and the weights applied in the planned contrast are summarized in . The contrast test was statistically significant, F(1,172) = 7.07, p < .01, ηp2 = .04, supporting the postulated pattern of H1.
Table 2. Study 1 planned contrast analysis of idea generation performance across experimental conditions.
Study 2: SVO, Reward Structure, and Peer Ideas
The purpose of the second study was two-fold. First, we wanted to replicate the main findings of Study 1. Second, we examined task effort as a mediator of the interaction effect of SVO and reward structure to test H2. Moreover, by adding a new factor, exposure to peer ideas, we examined whether the influence of peer ideas on idea generation is moderated by SVO and reward structure, testing H3.
Participants
Two hundred eighty-seven participants completed the study on MTurk. The demographic composition of the participants was similar to Study 1: 38% of the participants were male, 65% were between 25 and 44 years old and 92% had some college education or higher. Participant recruitment procedures were the same as Study 1. Of the 287 participants, 199 participants were classified as prosocial and 88 as proself, a similar distribution to Study 1.
Design and Procedure
The online experiment followed a 2 (SVO: prosocial vs. proself) x 2 (Reward structure: competitive vs. cooperative) x 2 (Peer idea: common vs. original) between-subjects factorial design. The classification of SVO, manipulation of reward structure, and idea generation task topic were the same as Study 1. The measurement of idea generation remained identical. The covariates, participation time, task relevance, and age were also kept consistent.
To test the mediating effect of task effort postulated in H2, we measured task effort using the average of two 7-point Likert scales (α = 0.82). The scales were: 1) “I tried hard to generate ideas to answer the question”; 2) “I was motivated to exert effort as I was generating the ideas”. The procedure of Study 2 was almost identical to Study 1, except that one additional procedure was added to manipulate peer idea exposure. After the reward structure manipulation and before the idea generation activity, participants were randomly assigned to one of two peer idea conditions.
Peer Idea Manipulation
One peer idea was provided as an example to participants following the task instruction. Since peer ideas with different levels of originality differ in their influence on participant creativity in brainstorming [Citation59, Citation73, Citation74], we varied the originality of peer ideas. Two ideas were selected from the ideas generated by participants in Study 1 to serve as experimental stimuli. One idea was high on originality; the other was low on originality (or common). Idea originality was measured based on how frequently an idea was generated across all participants in Study 1. Following Thompson and Brajkovich [Citation70], an idea that was generated by less than 5% of the participants in the study was considered as original and the rest were considered as common.
In the original idea condition, participants were told that “an example of an idea that helps solve the question is: improve the HIT’s website access and provide instructions on what to do if there’s a glitch.” The idea was highly original, generated by less than 5% of participants in Study 1. In the common idea condition, participants were provided with the example idea “offer bonuses for coherent and intelligent answers.” The idea was considered as common as it was generated by more than 50% of the participants in Study 1.
Manipulation Check
As a manipulation check, participants rephrased the example ideas in their own words. Only participants who passed the manipulation checks of both peer idea exposure and reward structure (79.0%) were included in the analyses.
Results
On average, each participant produced 7.09 ideas (SD = 4.78). Participants reported high task effort (M = 6.10, SD = 0.97), perceived the task to be highly relevant (M = 5.94, SD = 1.25) and spent 19.18 minutes on the task (SD = 15.96). Note that in Study 2, participants spent much longer time on the task than in Study 1 (M = 12.74). The average number of ideas generated by the participants was also slightly higher than that of Study 1 (M = 6.23). This may suggest that allowing participants to view peer ideas promoted idea generation. The descriptive statistics and correlation matrix are presented in .
Table 3. Study 2 Descriptive Statistics and Correlations (n=287).
As in Study 1, a 2 (SVO: prosocial vs. proself) X 2 (Reward structure: competitive vs. cooperative) x 2 (Peer idea: common vs. original) ANCOVA was first conducted on idea generation performance, controlling for participation time, task relevance, and age. The analysis showed that the main effect of task competition on idea quantity was in the expected direction: participants produced slightly more ideas when offered a competitive (M = 7.40, SD = 5.25) than a cooperative reward (M = 6.78, SD = 4.26), but the difference was not significant at the 0.05 level, F(1,276) = 3.06, p = .08, ηp2 = .01. There was a significant main effect of peer ideas on idea quantity, F(1,276) = 5.08, p < .05, ηp2 = .02. Participants produced significantly more ideas after seeing the original idea (M = 7.80, SD = 5.83) than the common idea (M = 6.47, SD = 3.52). Prosocials (M = 7.53, SD = 4.66) also produced more ideas than proselfs (M = 6.09, SD = 4.92), F(1,276) = 4.02, p < .05, ηp2 = .01. Since the positive effect of prosocial orientation on idea generation was not found in Study 1, it suggests that prosocials may be more stimulated by others’ ideas, regardless of idea originality.
Consistent with Study 1 and in support of H1, there was a statistically significant interaction effect of SVO and reward structure on idea generation, F(1,276) = 5.28, p < .05, ηp2 = .02. To test H1, we conducted the identical contrast tests as in Study 1 () and obtained a statistically significant result, F(1,283) = 12.59, p < .01, ηp2 = .04. The result supports H1 and again demonstrates that proselfs produced more ideas with competitive rewards than with cooperative rewards. Prosocials did not show this effect. graphically illustrates the interaction effect.
H3 posited that 1) the positive impact of original peer ideas on proselfs’ idea generation is stronger when competitive (vs. cooperative) rewards are offered and 2) prosocials are equally impacted by original peer ideas regardless of reward structure. In the ANCOVA, the three-way interaction effect of SVO, reward structure, and peer ideas (H3) was not statistically significant, F(1,276) = 2.49, p = .12. However, the hypothesis can be directly examined using planned contrasts, which have greater power to test specific patterns [Citation25].
Table 4. Study 2 planned contrast analysis for the effect of social value orientation and reward structure.
Figure 1. The effect of social value orientation and reward structure on idea generation and task effort (Study 2).
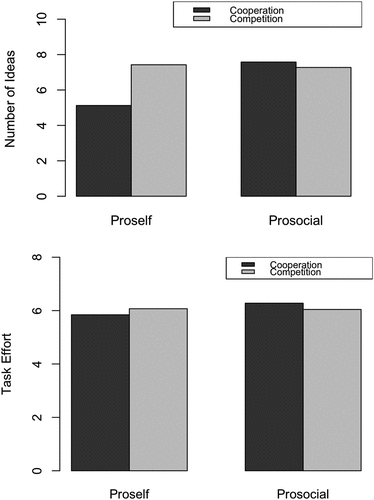
Based on H3, idea generation will be high for proselfs viewing an original peer idea and receiving competitive rewards and low for proselfs receiving cooperative rewards or viewing common ideas. For prosocials, they will produce more ideas when viewing an original versus a common idea, regardless of the rewards. We thus tested the hypothesized pattern using the contrast weights summarized in . Supporting H3, the contrast test result was statistically significant, F(1,283) = 18.82, p < .01, ηp2 = .06. This shows that proselfs’ idea generation was more impacted by original peer ideas when receiving competitive than cooperative rewards, but prosocials’ idea generation was enhanced by original peer ideas independent of reward structure.
Table 5. Study 2 planned contrast analysis for the effect of social value orientation, reward structure, and peer ideas.
Mediation Analysis
To test the mediation effect of task effort (H2), we followed the procedure proposed by Baron and Kenny [Citation5]. The complete analysis is summarized in . We began by using task effort as the dependent variable in the 2 (SVO: prosocial vs. proself) X 2 (Reward structure: competitive vs. cooperative) x 2 (Peer idea: common vs. original) ANCOVA, controlling for participation time, task relevance, and age. The analysis showed a significant interaction effect of SVO and reward structure on task effort, F(1,283) = 8.31, p < .01, ηp2 = .06, providing initial support for H2.
Table 6. The mediation analysis (Study 2).
Planned contrast analysis applying weights in was also statistically significant, F(1,283) = 18.82, p < .01, ηp2 = .03, revealing a consistent pattern of participants’ task effort with their idea generation performance. As illustrated in , proselfs exerted less effort when receiving cooperative than competitive rewards, but exerted a similar amount of effort as prosocials when offered competitive rewards. This pattern was consistent with their idea generation performance in the four experimental conditions.
In the next step, task effort was added into the ANCOVA with idea generation performance. After adding task effort, the interaction effect of SVO and reward structure was no longer statistically significant at the 0.05 level, F(1,283) = 3.29, p = .07. The reduction in significance suggests that task effort mediated the influence of the interaction effect on idea generation performance. Therefore, H2 was supported.
Note that after task effort was added to the ANCOVA model, the three-way interaction effect of SVO, reward structure, and peer ideas on idea generation performance remained statistically significant in the ANCOVA model, F(1,283) = 4.83, p < .05, ηp2 = .02. This suggests that the joint impact of SVO, reward structure, and peer ideas hypothesized in H3 cannot be explained by task effort. The planned contrast analysis on the three-way interaction effect on task effort () was also not statistically significant. It indicates that the pattern of task effort across the eight experimental conditions was different from the observed pattern of idea generation performance. Together, the results showed that task effort mediated the two-way interaction effect of SVO and reward structure on idea generation, but did not mediate the three-way interaction effect of SVO, reward structure, and peer ideas.
Nonetheless, the mediation analysis (Step 2 in ) revealed a statistically significant three-way interaction effect of SVO, reward structure, and peer ideas on task effort. Post-hoc comparisons showed that consistent with our general hypothesis, prosocials’ task effort did not differ across conditions (all p values > 0.1). However, proselfs reported significantly higher task effort when receiving competitive rewards and viewing a common (vs. an original) peer idea, F(1,276) = 4.52, p < .05, ηp2 = .02. This again confirms that proselfs’ behavior is more likely to be impacted by peer ideas when offered competitive rewards.
Discussion
The two experiments reported here support the call in information systems research to design tasks that appeal to participants with different SVOs in online communities [Citation40, Citation46]. Although scholars have acknowledged the importance of prosocial orientation in driving online contribution [Citation12, Citation13, Citation15, Citation37, Citation62], much research and practice still implicitly assume self-interest as the dominant drive. How prosocials react to variation in reward structures have been under-investigated. The current research fills this gap and highlights the necessity for research and practice to design reward structures that match and motivate participants with different SVOs.
Extending the motivated information processing theory [Citation22, Citation53], the research theorized that reward structures will have different impacts on prosocial and proself participants’ performance due to their distinct information processing styles. The two online experiments explicated the effects of reward structure on participants with different SVOs and probed the mediating mechanism and moderating effect on other environmental factors (i.e., peer ideas). These results elucidate the distinction in the behavior of prosocial and proself individuals and help reconcile the mixed findings regarding the impact of reward structure on performance [Citation13, Citation20, Citation27, Citation76]. They demonstrate the importance of differentiating participants’ SVO in crowdsourcing, offer insights on crowdsourcing task designs, and suggest approaches to detect the disposition based on online behavior.
Whereas prior research generally supports the positive effect of competitive rewards on crowd contribution and performance [Citation13, Citation20, Citation50], we found that this effect was mainly attributed to its impact on proself participants, who are cognitively sensitive to incentive cues and adjust their behavior to maximize individual gains. Research has shown that if the reward can be distributed to incentivize individuals who share their information, crowdsourced search can be more efficient [Citation68]. However, based on our findings, people who need to be incentivized to share are likely proselfs. Prosocials’ contributions tend to be consistent whether they are rewarded or not. Since both experiments recruited more prosocials than proselfs, our results suggest that a commonly applied crowdsourcing task design, crowdsourcing contests, may not be as indispensable as the popular belief.
In contrast, prosocials’ information processing does not focus on how and what they gain, and their idea production was not significantly affected by whether they were offered competitive or cooperative rewards. The finding that prosocials did not increase their idea generation when receiving cooperative rewards may be counter-intuitive. Our findings showed that even though prosocials are characterized by their intrinsic care for others, they did not perform better when rewarded as part of a group than when rewarded as an individual in competition with others. This could be because the variance in the two reward structures still mainly concerns participants’ own rewards, but not others’ gains. However, prosocials may be interested in making efforts to help others when the task is prosocial, and in particular altruistic, even without any gains of their own. For example, problems posted by OpenIdeo generally concern social issues such as education and poverty. Non-monetary incentives are common. Solving the issues does not directly benefit the contributors themselves, but usually a distant community. Compared to proselfs, prosocials may be more naturally attracted to contribute their ideas to this type of task since they are more concerned about others and care less about individual gains.
Proselfs react to environmental incentives with the goal of maximizing their gains and may choose to volunteer or contribute to prosocial tasks because they would like to enjoy an improved feeling of self or acquire other individual benefits such as social capital [Citation18]. However, this line of reasoning suggests that offering competitive financial rewards on prosocial tasks may not improve crowd performance and can even harm it. Since proselfs are sensitive to rewards and may choose to contribute to prosocial tasks for a better sense of self, monetary rewards in prosocial tasks may dampen the psychological gains that they expect from prosocial behavior and thus lower their performance [Citation2]. This helps explain a previous finding that individual financial incentives can hurt prosocial and voluntary contributions [Citation27, Citation64]. However, prosocials may not be significantly impacted by the form and number of rewards they receive on prosocial tasks. The postulations so far indicate that the interaction effect of reward structure and SVO may depend on the prosocial nature or framing of the task. Since all participants in our studies were paid, future research should compare offering versus not offering financial rewards in both prosocial and proself tasks to observe the potential behavioral difference in prosocials and proselfs.
Although previous crowdsourcing research has investigated the impact of viewing peer ideas on individual idea generation [Citation10, Citation72], it did not examine whether this effect is contingent on individual traits and reward structure. Our studies contribute to extant research by showing that the influence of peer ideas is not homogeneous, but rather depends on SVO and reward structure. Prosocials generated more ideas than proselfs after they viewed peer ideas in Study 2, but this difference was not found in Study 1 when participants did not see a peer idea. This may suggest that prosocials’ idea generation is promoted by peer ideas more than proselfs regardless of idea originality, as they pay more attention to others’ perspectives across different reward structures.
The aforementioned findings regarding the impact of peer ideas help explain prior research showing that sharing ideas during crowdsourcing contests can enhance crowd performance [Citation10]—it is perhaps because prosocials’ idea generation is promoted by other ideas in general and proselfs are more likely to be stimulated by others’ ideas in competition. Although the two-way interaction effect of SVO and reward structure on crowd idea generation performance was mainly attributed to task effort, participants’ task effort could not explain the interaction effect of SVO, reward structure, and peer ideas. This suggests that the moderating effect of peer ideas on SVO and reward structure was not motivational. The specific mechanism, however, awaits further investigation in future research.
Taken together, our findings highlight that crowdsourcing may be more effective if task rewards are matched with individual SVO. Research should further examine task designs that can motivate participants with different SVOs. To motivate proself participants, competition and performance-based incentives, or other individual rewards, may be leveraged. However, it is imperative to understand the contextual factors that significantly impact the effort and performance of prosocials.
Although not directly examined by the current study, prior research suggests that since prosocials’ information processing focuses on collective welfare and fairness, they may be more likely to assimilate to others’ behavior and take action when fairness is violated. For instance, prosocials are more likely to switch to public transit when they know that most others will choose public transit [Citation71]. They are also more likely to reciprocate others’ cooperation and punish others in social dilemmas when others defect, even at the expense of their own gains [Citation52]. Therefore, prosocials may be more prone to social influence and be more productive when they observe others investing effort. Online crowdsourcing platforms may ensure contributions from prosocials by setting performance standards through community performance statistics. Prior studies also found that prosocials are sensitive to social cues relevant to cooperation (e.g., signals of trustworthiness) [Citation8]. Thus, they may be better evaluators of others’ behavior and can be effective community managers or moderators. Crowdsourcing research and practice may experiment with assigning prosocials and proselfs to different tasks and roles within communities.
The discussion so far raises the question of whether crowdsourcing platforms should separate prosocial and proself participants and offer customized task designs to each group. The advantage of doing so can be that the platforms can design a more efficient incentive scheme as people with different SVOs are offered incentives that best suit their disposition. However, with different dispositions and information processing styles, prosocials and proselfs may have different cognitive strengths and diverse perspectives. The contribution from participants with different SVOs may enhance the integration of different ideas, and promote creative synergy and crowd performance in collective problem solving [Citation33]. As shown by Boudreau and colleagues [Citation10], disclosing solutions in the middle of an idea generation competition can reduce solution time and improve average collective performance, although it reduces experimentation. Partitioning participants based on their SVO may reduce the resilience of participants to task or contextual changes, as focus and specialization are found to be detrimental to people’s adaptation to environmental changes in online contexts [Citation36]. Therefore, limiting the interaction among participants and offering them customized incentives may be effective and retain crowd diversity for the short term, but may prevent the crowd from collective learning and hinder performance in the long run.
Design Implications for Crowdsourcing
Our findings suggest it is practically important for crowdsourcing platforms to identify and distinguish the SVO of participants and design tasks to promote idea generation based on this attribute. Our study measured SVO applying the Slider Measure [Citation56] because self-reported measures are used widely in prior SVO research [Citation4, Citation8]. However, scholars have started to infer psychological attributes, such as personality traits, based on online behavior traces [Citation1]. Results from the current research suggest that proselfs may be the ones who are most active when contests are held, whereas prosocials are consistent in their contribution regardless of changes in reward structure. By tracking this behavioral difference, crowdsourcing platforms can identify prosocial and proself participants in their communities and experiment with task designs to encourage performance from both groups.
Our findings also demonstrate that popular incentives to incite competition (e.g., contest, leaderboard) and reward individual performance may not be as essential as previously thought, particularly if the participant pool mainly consists of prosocials. Competitive rewards, or other forms of reward structures that compensates individual performance, may be useful when participants’ SVO is mainly proself. It may not be necessary to spend energy on calibrating reward structures in voluntary online communities mainly consisting of prosocials, since prosocials are not attentive to how they gain. In addition, although proselfs will want to gain some reward from their contribution, what they care about may not always be financial. Especially, to attract proselfs to tasks that are prosocial (e.g., improving education in remote communities), it could also be more useful to offer public recognition or glory of their contributions (e.g., reputation points, special community badges) instead of individual monetary rewards.
Crowdsourcing platforms and online communities alike should also experiment with various other task designs to stimulate performance in both prosocials and proselfs. For one, they could invest more time and resources to encourage participants to exchange their ideas if intellectual property is not a concern, as prior research suggests the general benefits of idea-sharing [Citation10] and the results of Study 2 showed that seeing peer ideas was particularly stimulating for prosocial participants. Moreover, as original ideas inspire idea generation of proselfs especially in a competition, crowdsourcing platforms may want to highlight highly original ideas produced by participants in crowdsourced ideation. This can be done by encouraging idea-sharing and evaluation (e.g., likes, up-votes, comments) and ranking published ideas based on their rarity and peer evaluations. This way, a competitive environment can be cultivated, enhancing the positive impact of original ideas on the idea generation of proselfs.
However, as discussed in the previous section, distinguishing participants’ SVO does not mean that crowdsourcing platforms should prevent participants with different SVOs from interacting with one another. When prosocials and proself are generating ideas in the same community, competition can be induced since they ensure contributions from proselfs and do not decrease idea productivity of prosocials. Different messages can be pushed to prosocial versus proself participants. For prosocials, messages can emphasize how their contribution will benefit the community and others, and information about peer performance and community norms (e.g., average/expected performance level; number of people who have contributed) can be included. Messages to proselfs can include their leaderboard information and their relative performance to others, and emphasize their chance to win a reward. Prosocials could also be invited to evaluate the originality of others’ ideas through private notifications. The produced originality scores could be published and highly original ideas can be recommended to all participants to promote the stimulating effects of peer ideas on their idea production.
Limitations and Future Research Directions
We recruited participants on an existing crowdsourcing platform (namely, MTurk) and used an idea generation task relevant to this particular sample. Although research has backed the quality of MTurk samples [Citation7], studying experienced participants on an existing platform may limit the generalizability of our findings to other online communities and tasks. Because we relied on MTurk, our research also did not examine the effect of SVO on choosing to participate in crowdsourced ideation but only examined the consequence of SVO once participants had decided to contribute. This is an important direction for future research.
It is possible that SVO also affects whether people participate in the platform to begin with and how they perceive their participation. For example, prosocials may interpret their participation in crowdsourced ideation as “helping companies find solutions to important problems to improve health and society”, whereas proselfs interpret their participation as “earning money and winning a contest for enjoyment.” Future research can probe how SVO may interact with environmental factors such as task framing, reward structure, and interaction structures on participants’ decision to join certain online communities.
The idea generation task was experimentally controlled. Following a common approach in group brainstorming [Citation59], participants did not interact with one another as they typically would in crowdsourcing to isolate the impacts of independent variables of interest. Future studies should examine the behavior of prosocials and proselfs in online communities to replicate and further explicate the interaction effects of SVO and task environment observed in the present experiments. More research is also needed to understand the incentive system that can motivate both prosocial and proself participants while promoting the benefit of diversity and synergy. In addition to idea production, future research can also address how SVO and the task environment together shape participants’ decision to participate in online tasks and their ability to evaluate and develop ideas produced by others.
Conclusion
Results from two online experiments demonstrate that the influence of rewards on crowd performance depends on participants’ SVO (i.e., prosocial vs. proself). Proselfs exerted more effort and generated more ideas when they were offered competitive than cooperative rewards. This effect was not observed for prosocials, who exerted high effort and generated many ideas regardless of reward type. Taken together, the findings suggest that proselfs and prosocials may respond differently to tasks, rewards, peer contributions, and other features of crowdsourcing platforms. Thus, the SVO of participants is an important parameter to consider in crowdsourcing application design and in future crowdsourcing research.
Supplemental Material
Download MS Word (36.8 KB)Acknowledgment
The authors would like to thank Peter Carnevale, Lian Jian, Jeff Nickerson, the editor, and the anonymous reviewers for their valuable feedback on the work. The work also benefited from Stevens Institute of Technology’s IS/Analytics Brownbag Research Seminar, organized by Aron Lindberg.
Disclosure statement
No potential conflict of interest was reported by the author(s).
Supplementary material
Supplemental data for this article can be accessed online at https://doi.org/10.1080/07421222.2022.2127451
Additional information
Funding
Notes on contributors
Bei Yan
Bei Yan ([email protected]; corresponding author) is an assistant professor at the School of Business, Stevens Institute of Technology. She received her Ph.D. degree from the University of Southern California. Dr. Yan studies technology-supported collaboration and influence processes of groups, ranging from small teams interacting with intelligent personal assistants to large online crowdsourcing communities. Prior to joining Stevens, she was a project scientist at the University of California, Santa Barbara.
Andrea B. Hollingshead
Andrea Hollingshead ([email protected]) is Professor of Communication in the Annenberg School of Communication and Journalism at the University of Southern California (USC). She has joint appointments in the Psychology Department and in the USC Marshall School of Business. Professor Hollingshead received her Ph.D. in Psychology from the University of Illinois Urbana-Champaign. Much of her research examines team transactive memory: how people communicate their expertise and share knowledge in teams. Other current research topics include team wellbeing, online incivility, and human-machine teaming.
References
- Adamopoulos, P.; Ghose, A.; and Todri, V. The impact of user personality traits on word of mouth: text-mining social media platforms. Information Systems Research, 29, 3 (2018), 612–640.
- Andreoni, J. Giving with impure altruism: Applications to charity and Ricardian equivalence. Journal of Political Economy, 97, 6 (1989), 1447–1458.
- Armisen, A.; and Majchrzak, A. Tapping the innovative business potential of innovation contests. Business Horizons, 58, 4 (2015), 389–399.
- Balliet, D.; Parks, C.; and Joireman, J. Social value orientation and cooperation in social dilemmas: A meta-analysis. Group Processes & Intergroup Relations, 12, 4 (2009), 533–547.
- Baron, R.M.; and Kenny, D.A. The moderator–mediator variable distinction in social psychological research: Conceptual, strategic, and statistical considerations. Journal of Personality and Social Psychology, 51, 6 (1986), 1173–1182.
- Bechtoldt, M.N.; de Dreu, C.K.W.; Nijstad, B.A.; and Choi, H.-S. Motivated information processing, social tuning, and group creativity. Journal of Personality and Social Psychology, 99, 4 (2010), 622–637.
- Berinsky, A.J.; Huber, G.A.; and Lenz, G.S. Evaluating online labor markets for experimental research: Amazon.com’s Mechanical Turk. Political Analysis, 20, 3 (2012), 351–368.
- Bogaert, S.; Boone, C.; and Declerck, C. Social value orientation and cooperation in social dilemmas: A review and conceptual model. British Journal of Social Psychology, 47, 3 (2008), 453–480.
- Boudreau, K.J.; Lacetera, N.; and Lakhani, K.R. Incentives and problem uncertainty in innovation contests: An empirical analysis. Management science, 57, 5 (2011), 843–863.
- Boudreau, K.J.; and Lakhani, K.R. “Open” disclosure of innovations, incentives and follow-on reuse: Theory on processes of cumulative innovation and a field experiment in computational biology. Research Policy, 44, 1 (2015), 4–19.
- Boudreau, K.J.; Lakhani, K.R.; and Menietti, M. Performance responses to competition across skill levels in rank-order tournaments: field evidence and implications for tournament design. The RAND Journal of Economics, 47, 1 (2016), 140–165.
- Bulgurcu, B.; Van Osch, W.; and Kane, G.C. (Jerry). The rise of the promoters: User classes and contribution patterns in enterprise social media. Journal of Management Information Systems, 35, 2 (2018), 610–646.
- Burtch, G.; Hong, Y.; Bapna, R.; and Griskevicius, V. Stimulating online reviews by combining financial incentives and social norms. Management Science, 64, 5 (2017), 2065–2082.
- Cao, F.; Wang, W.; Lim, E.; Liu, X.; and Tan, C.-W. Do social dominance-based faultlines help or hurt team performance in crowdsourcing tournaments? Journal of Management Information Systems, 39, 1 (2022), 247–275.
- Chang, H.H.; and Chuang, S.-S. Social capital and individual motivations on knowledge sharing: Participant involvement as a moderator. Information & Management, 48, 1 (2011), 9–18.
- Choi, H.S.; Oh, W.; Kwak, C.; Lee, J.; and Lee, H. Effects of online crowds on self-disclosure behaviors in online reviews: A multidimensional examination. Journal of Management Information Systems, 39, 1 (2022), 218–246.
- De Dreu, C.K.W.; Giebels, E.; and Van de Vliert, E. Social motives and trust in integrative negotiation: The disruptive effects of punitive capability. Journal of Applied Psychology, 83, 3 (1998), 408–422.
- Deng, X.; and Joshi, K.D. Why individuals participate in micro-task crowdsourcing work environment: Revealing crowdworkers’ perceptions. Journal of the Association for Information Systems, 17, 10 (2016), 648–673.
- Dennis, A.R.; Minas, R.K.; and Bhagwatwar, A.P. Sparking creativity: Improving electronic brainstorming with individual cognitive priming. Journal of Management Information Systems, 29, 4 (2013), 195–216.
- Dissanayake, I.; Mehta, N.; Palvia, P.; Taras, V.; and Amoako-Gyampah, K. Competition matters! Self-efficacy, effort, and performance in crowdsourcing teams. Information & Management, 56, 8 (2019), 103158.
- Dissanayake, I.; Nerur, S.; Wang, J.; Yasar, M.; and Zhang, J. The impact of helping others in coopetitive crowdsourcing communities. Journal of the Association for Information Systems, 22, 1 (2021), 67–101.
- de Dreu, C.K.W.; Nijstad, B.A.; Bechtoldt, M.N.; and Baas, M. Group creativity and innovation: A motivated information processing perspective. Psychology of Aesthetics, Creativity, and the Arts, 5, 1 (2011), 81–89.
- Dubé, J.-P.; Luo, X.; and Fang, Z. Self-signaling and prosocial behavior: A cause marketing experiment. Marketing Science, 36, 2 (2017), 161–186.
- Eisenberger, R.; Kuhlman, D.M.; and Cotterell, N. Effects of social values, effort training, and goal structure on task persistence. Journal of Research in Personality, 26, 3 (1992), 258–272.
- Furr, R.M.; and Rosenthal, R. Evaluating theories efficiently: The nuts and bolts of contrast analysis. Understanding Statistics, 2, 1 (2003), 33–67.
- Galletta, D.F.; Marks, P.V.; Polak, P.; and McCoy, S. What leads us to share valuable knowledge? An experimental study of the effects of managerial control, group identification, and social value orientation on knowledge-sharing behavior. In Proceedings of the 36th Annual Hawaii International Conference on System Sciences. 2003, pp. 1–10.
- Gneezy, A.; Imas, A.; Brown, A.; Nelson, L.D.; and Norton, M.I. Paying to be nice: Consistency and costly prosocial behavior. Management Science, 58, 1 (2011), 179–187.
- Goncalo, J.A.; and Kim, S.H. Distributive justice beliefs and group idea generation: Does a belief in equity facilitate productivity? Journal of Experimental Social Psychology, 46, 5 (2010), 836–840.
- Grant, A.M.; and Berry, J.W. The necessity of others is the mother of invention: Intrinsic and prosocial motivations, perspective taking, and creativity. Academy of Management Journal, 54, 1 (2011), 73–96.
- Haruno, M.; and Frith, C.D. Activity in the amygdala elicited by unfair divisions predicts social value orientation. Nature Neuroscience, 13, 2 (2010), 160–161.
- Hertel, G.; and Fiedler, K. Fair and dependent versus egoistic and free: Effects of semantic and evaluative priming on the “Ring Measure of Social Values.” European Journal of Social Psychology, 28, 1 (1998), 49–70.
- von Hippel, E. Democratizing Innovation. The MIT Press, Cambridge, Mass., 2006.
- Hong, L.; Page, S.; and Riolo, M. Incentives, information, and emergent collective accuracy. Managerial and Decision Economics, 33, (2012), 323–334.
- Jadin, T.; Gnambs, T.; and Batinic, B. Personality traits and knowledge sharing in online communities. Computers in Human Behavior, 29, 1 (2013), 210–216.
- Jeppesen, L.B.; and Lakhani, K.R. Marginality and problem-solving effectiveness in broadcast search. Organization Science, 21, 5 (2010), 1016–1033.
- Jiang, J.; Maldeniya, D.; Lerman, K.; and Ferrara, E. The wide, the deep, and the maverick: Types of players in team-based online games. Proceedings of the ACM on Human-Computer Interaction, 5, CSCW1 (2021), pp. 191: 1–191:26.
- Khansa, L.; Ma, X.; Liginlal, D.; and Kim, S.S. Understanding members’ active participation in online question-and-answer communities: A theory and empirical analysis. Journal of Management Information Systems, 32, 2 (2015), 162–203.
- van Kleef, G.A.; and van Lange, P.A.M. What other’s disappointment may do to selfish people: Emotion and social value orientation in a negotiation context. Personality and Social Psychology Bulletin, 34, 8 (2008), 1084–1095.
- Koh, T.K.; and Cheung, M.Y.M. Seeker exemplars and quantitative ideation outcomes in crowdsourcing contests. Information Systems Research, 33, 1 (2022), 265–284.
- von Krogh, G.; Haefliger, S.; Spaeth, S.; and Wallin, M.W. Carrots and rainbows: Motivation and social practice in open source software development. MIS Quarterly, 36, 2 (2012), 649–676.
- de Kwaadsteniet, E.W.; van Dijk, E.; Wit, A.; and de Cremer, D. Social dilemmas as strong versus weak situations: Social value orientations and tacit coordination under resource size uncertainty. Journal of Experimental Social Psychology, 42, 4 (2006), 509–516.
- van Lange, P.A.M. Beyond self-interest: A set of propositions relevant to interpersonal orientations. European Review of Social Psychology, 11, 1 (2000), 297–331.
- van Lange, P.A.M.; Otten, W.; de Bruin, E.M.; and Joireman, J.A. Development of prosocial, individualistic, and competitive orientations: Theory and preliminary evidence. Journal of Personality and Social Psychology, 73, 4 (1997), 733–746.
- Lebel, R.D.; and Patil, S.V. Proactivity despite discouraging supervisors: The powerful role of prosocial motivation. Journal of Applied Psychology, 103, 7 (2018), 724–737.
- Lee, H.C.B.; Ba, S.; Li, X.; and Stallaert, J. Salience bias in crowdsourcing contests. Information Systems Research, 29, 2 (2018), 401–418.
- Leimeister, J.M.; Huber, M.; Bretschneider, U.; and Krcmar, H. Leveraging crowdsourcing: Activation-supporting components for it-based ideas competition. Journal of Management Information Systems, 26, 1 (2009), 197–224.
- Li, G.; and Wang, J. Threshold effects on backer motivations in reward-based crowdfunding. Journal of Management Information Systems, 36, 2 (2019), 546–573.
- Liebrand, W.B.G.; Wilke, H.A.M.; Vogel, R.; and Wolters, F.J.M. Value orientation and conformity: A study using three types of social dilemma games. Journal of Conflict Resolution, 30, 1 (1986), 77–97.
- Lindberg, A.; Majchrzak, A.; and Malhotra, A. How information contributed after an idea shapes new high-quality ideas in online ideation contests. MIS Quarterly, 46, 2 (2022), 1195–1208.
- Liu, T.X.; Yang, J.; Adamic, L.A.; and Chen, Y. Crowdsourcing with all-pay auctions: A field experiment on taskcn. Management Science, 60, 8 (2014), 2020–2037.
- Malhotra, A.; and Majchrzak, A. Greater associative knowledge variety in crowdsourcing platforms leads to generation of novel solutions by crowds. Journal of Knowledge Management, 23, 8 (2019), 1628-1651.
- McClintock, C.G.; and Liebrand, W.B.G. Role of interdependence structure, individual value orientation, and another’s strategy in social decision making: A transformational analysis. Journal of Personality and Social Psychology, 55, 3 (1988), 396–409.
- Meglino, B.M.; and Korsgaard, A. Considering rational self-interest as a disposition: organizational implications of other orientation. The Journal of Applied Psychology, 89, 6 (2004), 946–959.
- Messick, D.M.; and McClintock, C.G. Motivational bases of choice in experimental games. Journal of Experimental Social Psychology, 4, 1 (1968), 1–25.
- Mo, J.; Sarkar, S.; and Menon, S. Know when to run: Recommendations in crowdsourcing contests. MIS Quarterly, 42, 3 (2018), 919–944.
- Murphy, R.O.; and Ackermann, K.A. Social value orientation: Theoretical and measurement issues in the study of social preferences. Personality and Social Psychology Review, 18, 1 (2014), 13–41.
- Murphy, R.O.; Ackermann, K.A.; and Handgraaf, M. Measuring social value orientation. Judgment and Decision Making, 6, 8 (2011), 771–781.
- Nijstad, B.A.; Stroebe, W.; and Lodewijkx, H.F.M. Persistence of brainstorming groups: How do people know when to stop? Journal of Experimental Social Psychology, 35, 2 (1999), 165–185.
- Nijstad, B.A.; Stroebe, W.; and Lodewijkx, H.F.M. Cognitive stimulation and interference in groups: Exposure effects in an idea generation task. Journal of Experimental Social Psychology, 38, 6 (2002), 535–544.
- Paulus, P.B.; and Yang, H.-C. Idea generation in groups: A basis for creativity in organizations. Organizational Behavior and Human Decision Processes, 82, 1 (2000), 76–87.
- Peddibhotla, N.B.; and Subramani, M.R. Contributing to public document repositories: A critical mass theory perspective. Organization Studies, 28, 3 (2007), 327–346.
- Phang, C.W.; Kankanhalli, A.; and Huang, L. Drivers of quantity and quality of participation in online policy deliberation forums. Journal of Management Information Systems, 31, 3 (2014), 172–212.
- Platow, M.J. An evaluation of the social desirability of prosocial self—other allocation choices. The Journal of Social Psychology, 134, 1 (1994), 61–68.
- Qiao, D.; Lee, S.-Y.; Whinston, A.B.; and Wei, Q. Financial incentives dampen altruism in online prosocial contributions: A study of online reviews. Information Systems Research, 31, 4 (2020), 1361–1375.
- Rosenbaum, M.E.; Moore, D.L.; Cotton, J.L.; Cook, M. S.; Hieser, R. A.; Shovar, M. N.; & Gray, M. J. Group productivity and process: Pure and mixed reward structures and task interdependence. Journal of Personality and Social Psychology, 39, 4 (1980), 626–642.
- Sun, Y.; Tuertscher, P.; Majchrzak, A.; and Malhotra, A. Pro-socially motivated interaction for knowledge integration in crowd-based open innovation. Journal of Knowledge Management, 24, 9 (2020), 2127–2147.
- Taggar, S. Individual creativity and group ability to utilize individual creative resources: A multilevel model. The Academy of Management Journal, 45, 2 (2002), 315–330.
- Tang, J.C.; Cebrian, M.; Giacobe, N.A.; Kim, H.-W.; Kim, T.; and Wickert, D. “Beaker.” Reflecting on the DARPA Red Balloon Challenge. Communications of the ACM, 54, 4 (2011), 78–85.
- Terwiesch, C.; and Xu, Y. Innovation contests, open innovation, and multiagent problem solving. Management Science, 54, 9 (2008), 1529–1543.
- Thompson, L.; and Brajkovich, L.F. Improving the creativity of organizational work groups. The Academy of Management Executive, 17, 1 (2003), 96–111.
- van Vugt, M.; Meertens Ree, M.; and van Lange, P.A.M. Car versus public transportation? The role of social value orientations in a real‐life social dilemma. Journal of Applied Social Psychology, 25, 3 (2006), 258–278.
- Wang, K.; and Nickerson, J.V. A wikipedia-based method to support creative idea generation: The role of stimulus relatedness. Journal of Management Information Systems, 36, 4 (2019), 1284–1312.
- Yan, J.; Leidner, D.E.; and Benbya, H. Differential innovativeness outcomes of user and employee participation in an online user innovation community. Journal of Management Information Systems, 35, 3 (2018), 900–933.
- Yu, L.; and Nickerson, J.V. Cooks or cobblers? Crowd creativity through combination. In Proceedings of the SIGCHI Conference on Human Factors in Computing Systems. ACM, New York, NY, USA, 2011, pp. 1393–1402.
- Zhang, S.; Singh, P.V.; and Ghose, A. A structural analysis of the role of superstars in crowdsourcing contests. Information Systems Research, 30, 1 (2019), 15–33.
- Zhao, L.; Detlor, B.; and Connelly, C.E. Sharing knowledge in social Q&A sites: The unintended consequences of extrinsic motivation. Journal of Management Information Systems, 33, 1 (2016), 70–100.
- Zheng, H.; Xu, B.; Hao, L.; and Lin, Z. Reversed loss aversion in crowdsourcing contest. European Journal of Information Systems, 27, 4 (2017), 434–448.