Abstract
A coupled watershed–reservoir modeling system was applied to the Lake Waco reservoir and watershed to test possible sources of seasonal excess nutrient concentrations in Lake Waco. The Soil Water Assessment Tool (SWAT) was used for modeling watershed production of water and nutrients. This model included small lakes and dairies in the watershed, located spatially using geographic data. For the reservoir, the 2-dimensional, hydrodynamic model CE-Qual-W2 was used with inputs derived from the SWAT simulations. Calibration of the models was based on observed hydrographic information and stream and reservoir nutrient data. The relationship between predicted and observed stream flow values for the North Bosque River, the major tributary to the reservoir, were highly correlated (r 2= 0.86) for the calibration period 1997–1998. Predicted daily nutrient values near the inflow of the North Bosque into Lake Waco reservoir were variable but similar to previously estimated annual loading values. Comparison of predicted water quality characteristics from the CE-Qual-W2 model with observed values showed acceptably reliable correspondence seasonally. Chlorophyll-a was used as the main measure for calibration accuracy due to its importance for reservoir management and was predicted to within 6.6% based on the mean percent error or an absolute root mean square error of 9.76 μg/L of observed values. The prediction of stream values from the SWAT model and reservoir nutrient concentrations were sensitive and robust for the next phase of the Comprehensive Lake Waco Study, which includes evaluation of multiple watershed and reservoir management options.
Simulation modeling is a cost-effective method for estimating influential biotic and abiotic factors affecting water quality in linked watershed–reservoir systems. Once calibrated, these models provide a valid template for analyzing watershed and reservoir behavior, formulating best management practices and defining importance of physical and biological processes. Integration of hydrological modeling with geographical databases has advanced the ability to assess effects of land management on stream dissolved nutrients (CitationTong and Chen 2002). Differences exist between models that are important for different goals. Diagnostic hydrologic models have been developed to rigorously assess watershed properties important to water nutrients based on statistical analyses (CitationMoore et al. 2004). In contrast, prognostic models are based on physical representations of movement of materials, energy transfer and biogeochemistry and are characteristically predictive and mechanistic (CitationArnold and Fohrer 2005). Mechanistic reservoir modeling has also advanced with representation of spatial hydrodynamics and simulation of water movement for predicting nutrient distributions in water bodies (CitationHamilton and Schladow 1997).
The advantage of mechanistic models for modeling management activities is attributed to their adherence of first-principle approaches that rely on physically measurable parameters. However, complex ecosystem interactions necessitate numerous parameter measurements under the mechanistic paradigm. Calibration can be difficult where parameter estimation is constrained by reliance on values derived from limited observation. Successful calibration of mechanistic models confirms the mathematical and physical representation of underlying processes as exhibiting reasonable behavior (CitationGrant et al. 1997).
Linked watershed–lake modeling studies are rare because most watershed models have been derived from engineering and hydrology disciplines whereas lake and reservoir models have been generally developed by limnologists. Early model development focused on nutrient delivery from landscapes to waterbodies with less emphasis on the transport mechanisms or stream and lake transformations (CitationSummer et al. 1990, Reed-Anderson et al. 2000). More recent work has coupled physical watershed models with hydrodynamic models in lakes and reservoirs (CitationXu et al. 2007, CitationDebele et al. 2008) primarily to address the complexity of abiotic and biotic transformations of nutrients that occur along the length of water transport from precipitation to storage in water bodies.
In this paper we present the calibration of a watershed model, specifically the Soil Water Assessment Tool (SWAT), to predict water and nutrients inflow into the Lake Waco reservoir for 1997–1998. The predicted inflows from the SWAT model are then used as inputs in a reservoir model, CE-Qual-W2 (version 3.0), which is used to predict nutrients, dissolved oxygen and chlorophyll-a (chl-a) concentrations over the same time period. Calibration and verification of water quality of this linked modeling system are necessary for assessing watershed and reservoir management strategies. Key outcomes expected for this project were to (Equation1) represent major watershed features such as concentrated animal feeding operations (CAFO) and Public-Law 566 (PL-566) flood control reservoir structures and to (Equation2) develop a modeling system for future Lake Waco reservoir source water management scenario projections.
Study site
The Lake Waco watershed () covers approximately 10,340 km2 of the central Texas Great Plains with a subtropical climate (CitationKier et al. 1977, CitationBull 1991). The long-term average temperature observed by meteorological stations in the watershed is approximately 18 C with average annual precipitation of 817 mm that is distributed seasonally with the driest months during winter and wettest during late spring. The elevation of the Lake Waco watershed ranges from approximately 114 to 493 m a.s.l and is underlain by gently southeastern dipping sedimentary bedrock. Outcrops of limestone (e.g., Edwards Limestone) form watershed drainages while shale and sandstone make up alluvial material in valleys. The sedimentary-dominated stratigraphy results in a generally dendritic stream network. Major tributaries of the Lake Waco reservoir include the North Bosque, South Bosque and Middle Bosque rivers and Hog Creek, which converge in the reservoir as part of the original Bosque River. Direct drainage to the lake also occurs along the east shore of the reservoir, which includes urban areas associated with the City of Waco.
Figure 1 Features of Lake Waco watershed. Key features include Lake Waco, subwatersheds, major tributaries, PL-566 structures, Confined Animal Feeding Operations (CAFO) and meteorological observation locations relevant to the SWAT simulations.
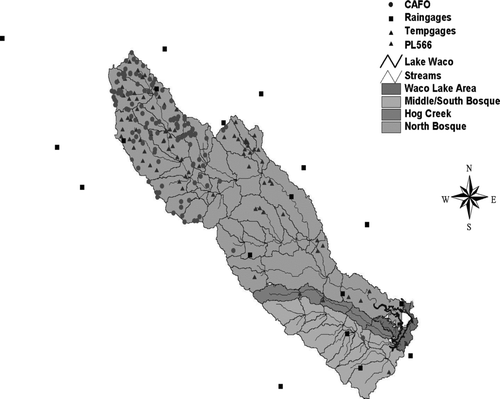
Vegetation of the watershed is characterized by oak–hickory woodlands on sandy soils and short to mid-grass species on clay soils in the natural state. Rangeland (62.4%) and woodlands (19.4%) are the dominant land cover type, followed by agriculture (15.4%) and urbanized areas (1.3%). Most of the vegetation in the western portion of the watershed is rangeland that gently grades to agriculture and woodlands in the east.
Materials and methods
Reservoir description
The City of Waco initially constructed Lake Waco as a municipal water supply in 1929. In 1954, Lake Waco was authorized for expansion construction under the Flood Control Act with initial construction beginning in 1958. Finalization of this project was in 1965 with an earthen dam constructed to intentionally impound water. The current dam structure is 7503.6 m in length with a maximum height of 42.6 m above the streambed of the Bosque River. Before October 2003, the average lake level was maintained at approximately 138.6 m a.s.l. In October 2003, the average lake level was raised to be maintained at an average elevation of 140.7 m a.s.l. for increased water storage and production for the City of Waco. This pool level change has resulted in peripheral flooding of shallow and lowland areas along the shoreline with a change in average surface area from 29.42 to 35.30 km2. Average depth of the reservoir has also increased slightly with this increase in conservation pool from 6.0 m (CitationSullivan et al. 1995) to 6.8 m (estimated from this study; ).
Table 1 Physical characteristics of Lake Waco.
Model descriptions
Watershed modeling
The watershed modeling presented in this study used SWAT2000, the most recent version of SWAT (CitationNeitsch et al. 2002a, Citation2002b) developed over 20 years ago to simulate point and nonpoint sources of pollution (CitationArnold and Fohrer 2005). SWAT is a continuous daily-time step model developed to predict the impact of management on water, sediment and nutrient yields in large, ungauged watersheds. The model is designed to (Equation1) use readily available inputs and (Equation2) be capable of simulating long periods for computing the effects of management changes (CitationNeitsch et al. 2002a, Citation2002b, CitationArnold and Fohrer 2005). Details concerning how the processes are simulated are presented in the SWAT manuals (CitationArnold et al. 1996, Citation1999, CitationNeitsch et al. 2002a, Citation2002b).
Reservoir modeling
The CE-Qual-W2 is a 2-dimensional, laterally averaged, water quality reservoir model developed for stream, reservoir, natural lakes and estuary systems (CitationCole and Wells 2000). It was chosen for this study because of its discrete physical representation of water flow through reservoirs, its system approach to nutrient and sediment and its potential to represent the most complete and potentially robust modeling tool for lake managers (CitationBorah et al. 2006). Nutrient cycling in CE-Qual-W2 is predominantly controlled by 4 major nutrient pools that may act as sources or sinks of nutrients: (Equation1) the water column, (Equation2) suspended organic matter, (3) algae or epiphytes and (Equation4) the sediment (). In the model, nutrients are imported into the reservoir via river inflow where they are partitioned into the major pools. Specific kinetic processes control the transfer of nutrients between the major pools.
Figure 2 Flow diagram of nutrients, organic matter, algae and sediments represented in the CE-Qual-W2 model.
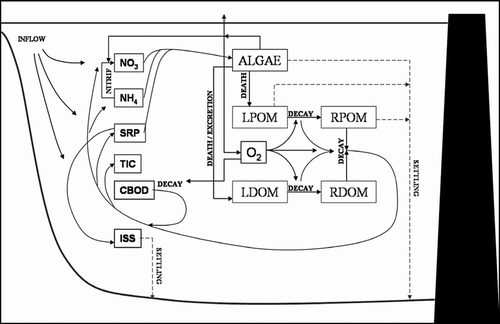
The nutrients modeled for this study included phosphorus and nitrogen as a first step to identifying potential management options for the watershed and reservoir. In CE-Qual-W2, nutrients are derived externally from inflowing waters. Within the reservoir, nutrients are part of living and dead organic matter and sediments. Living organic matter includes algae and epiphytes, which have specific nutrient uptake and release rates. Dead organic matter comes in dissolved and particulate forms that may be labile or refractory depending on the chemical availability of carbon for decomposer microorganisms present in the water. Sediment control over nutrient availability is related to oxygen levels that change the redox state of the substrate.
In this study, nutrient concentrations in the reservoir were primarily controlled by algal productivity. Algae are represented as 4 functional groups or assemblages (e.g., green algae, summer and winter diatoms, and cyanobacteria) with inherent photosynthesis and respiration rates controlled by light, nutrients and temperature. The number of functional groups was based on prior modeling of the algal communities of this reservoir (CitationFlowers et al. 2001, CitationUmorin 2006). Because each functional algal group also has a specific nitrogen and phosphorus stoichiometry, nutrient availability fluctuates in the model based on the net production rates of each algal group across the reservoir. Dead algal biomass also becomes part of the organic matter within either the water or the sediment.
Model initialization
Watershed model
The SWAT2000 model automatically derives exact landscape parameters from digital topographic, land cover and soils data using an ArcGIS®3.3 interface. Digital elevation model (DEM) data were derived from the US Geological Survey (USGS) National Elevation Dataset with a 30-m resolution elevation data. For land cover, we used the 1992 USGS National Land Cover Data (NLCD) set, which is based on classified Landsat Thematic Mapper (TM) satellite data, also at 30-m spatial resolution. The State Soil Geographic (STATSGO) database from the National Cooperative Soil Survey (NCSS) was used as input soils by depth, which is important for subsurface water movement and erosion predictions. Daily meteorological data were acquired from 18 National Climatic Data Center (NCDC) weather stations with all counties of the watershed to represent the varying weather conditions in the basin.
From the DEM data, watersheds and subsequently 89 sub-basins were defined from the SWAT topographic algorithms. These sub-basins were further broken down into 1830 hydrologic response units (HRU) that represent unique landcover–soil areas within a sub-basin also defined by the SWAT2000 software. For the watershed simulations, the HRU were the base simulation unit for simulating nutrient, sediment and water flux from the landscape to the stream systems.
Anthropogenic features were also incorporated in the watershed model and included (Equation1) concentrated animal feeding operation (CAFO) lagoons associated with dairies, (Equation2) CAFO dairy treatment fields (DTF), (3) PL-566 flood control reservoirs and (Equation4) 8 municipal waste water treatment plants (WWTP). In SWAT, the CAFO lagoons were simulated as small ponds with the physical dimension set as an average for all CAFO lagoons based on McFarland et al. (2000). However, this original study was based on an average 1000-cow dairy, which by 1997 was reduced to a 500-cow dairy. Thus, the McFarland et al. (2000) lagoon dimensions were reduced by 50%, resulting in an average CAFO lagoon drainage area of 3.45 ha, a surface area of 0.81 ha and an “emergency spillway” or maximum volume of 6865 m3. At the time of the simulations work, there were approximately 117 CAFO and about 85,000 milking cows for the study area. The CAFO–DTF were simulated by setting an auto-irrigation operation for the nearest closely grown agricultural HRU connected to the pond feature. Each CAFO lagoon was “pumped” by this setting to estimate on-site liquid waste dispersal management.
The nutrient and sediment content of the CAFO lagoons were based upon empirical equations related to livestock waste production within CAFO. The livestock manure volume for the typical single lagoon was estimated using a live animal weight (LAW) of 636 kg with each animal spending 6 hours a day in the milking parlor and feed lanes (CitationMcFarland et al. 2000). Daily manure production was estimated at 86 kg/1000 kg LAW with a density of dairy manure at 990 kg/m3(ASAE 1999) for a resultant 6.9 m3/day of manure added to each lagoon. The mass of nutrients lost via settling was calculated by the model as
The DTF were simulated based on the mass of manure calculated for each CAFO lagoon. For the sub-basin containing a CAFO, the calculated solid waste component of the manure was applied daily to agricultural lands within the same sub-basin. Note that only the top 10 cm of the soil layer were considered to be affected. For the liquid waste component of the simulated lagoons, the auto-irrigation algorithm draws water from the simulated lagoons. The nutrient composition of the solid and liquid waste was initialized with values of 0.005 mg PO4-P/L, 0.046 mg NH4-N/L and 0.001 mg NO2+NO3-N/L, which are the SWAT default values for fresh dairy manure.
The PL-566 reservoirs were modeled as wetlands in SWAT. Ponds and wetlands are handled the same in SWAT, except for water outflow. For volumes between the target (normal) storage and maximum storage, the wetland was hard-wired for 10 days to reach its target storage, close to what is expected for the PL-566 structures. Dimensional data for the reservoirs within the watershed were simulated using the US Army Corps of Engineers (USACOE) National Inventory of Dams data. Initial nutrient values for these structures were set to 0.0 mg/L but were allowed to increase over time based on upstream, landscape-derived inputs using a 1-year spin-up period (1996) before the 1997–1998 simulation. Direct point-source values for 8 permitted WWTP in the Lake Waco watershed were calculated from daily discharge data multiplied by daily average nutrients for each site. Daily average nutrients were derived from reported annual nutrient data divided by 365. This daily value was added to the stream flow and nutrient concentrations within SWAT.
Reservoir model
Bathymetric data from the Texas Water Development Board were merged with the USGS DEM data to produce a continuous elevation surface for the entire potential reservoir volume. The lake was then divided into 3 sections for simulation, including a North Arm (NA), South Arm (SA), and Main Body (MB), which are consistent with observed historical trends in lake chemistry and algal productivity (O.T. Lind, Baylor University, May 2003, pers. comm.). Within each of these sections, segment partitions were created within a Geographic Information System (GIS) to approximately evenly divide areas with relatively similar water depths and morphology. This process produced 24 simulation segments, with 8 in NA, 9 in SA and the remaining 7 in MB (). For each segment, maximum lake width at elevated pool rise, average bottom slope and average cross-sectional morphology were determined from analysis of the GIS data.
Water and nutrient inflow data were generated by outputs from the SWAT simulations described previously. The SWAT model does not predict organic matter (OM), however, which is a major component of the nutrient cycling mechanisms within CE-Qual-W2. Therefore, OM was derived from SWAT inflow predictions of organic phosphorus (OP; Flowers et al. 2001) based on a 63:1 stoichiometric relationship (CitationWilkie and Mulbry 2002) between OM and organic phosphorus (OP):
This organic matter was separated into 4 major compartments: labile dissolved organic matter (LDOM = 30%), refractory dissolved organic matter (RDOM = 60%), labile particulate organic matter (LPOM = 5%) and refractory particulate organic matter (RPOM = 5%; Wetzel 1984). The derived inflow of OM was assumed to be 75% refractory and 25% labile; however, partitioning into dissolved and particulate was based on inflow rates from tributaries. An association between total suspended solid (TSS) concentrations and stream flow was assumed with partitioning between OM forms (CitationFlowers et al. 2001) where higher flows deliver greater amounts of particulate OM. Values of TSS from SWAT with concentrations ?100 mg/L represented high stream flow where partitioning of refractory and labile OM into dissolved and particulate components was set at 25% and 75%, respectively. With TSS < 100 mg/L, the partitioning was set at 60% dissolved and 40% particulate.
Outflows in the model include gated release, emergency spillway and withdrawals. For the simulations, gated flows from the USACOE database for Lake Waco were used initially (http://swf67.swf-wc.usace.army.mil/waco/wacolake.htm). Data for withdrawal by the City of Waco were provided by the city's water utilities office. Because discharge records do not include tanier gate releases due to flooding events or downstream flow projects, water outflow data required some adjustment. In these simulations, water was subtracted or added to the gate discharge amounts to balance the lake elevation levels with observed values determined quantitatively.
Initial reservoir state conditions were set based on water quality data collected at a site in the main body of the reservoir (TCEQ site #11942) by Texas Institute for Applied Environmental Research personnel in January 1997. Data collected and used to characterize general lake conditions included values for PO4-P, NH4-N, NO2+NO3-N, carbonaceous biological oxygen demand and conductivity. Conductivity values were then converted into total dissolved solids (TDS, mg/L) where TDS = conductivity (μmhos/cm)/0.64. Planktonic algal chl-a data were also collected and converted into algal biomass and distributed among the 4 algal simulation groups described previously. Given the simulation starting date of 1 January, initial biomass partitioning among the algal groups was 7.5% to each of the green algae (ALG1), summer diatoms (ALG4) and cyanobacteria (ALG3), with the remaining 77.5% partitioned to the winter diatom group (ALG2). Inclusion of a summer diatom group best represented the algal assemblage in Lake Waco reservoir (CitationUmorin 2006). Temperature kinetics of the primary diatom group (ALG2) reflects a cold water assemblage with a modeled optimum growth temperature of 17 C (CitationMontagnes and Franklin 2001). The secondary warm season diatoms (ALG4) were modeled with an optimum growth temperature set to 27 C.
Model simulations and calibration
Both models require meteorological data that include daily temperatures and precipitation. Insolation within each model is calculated internally based on latitude, diurnal temperature variation and precipitation. For SWAT, these values were input for each of the 18 NCDC observation sites described previously for 1 January 1994 through 31 December 1998. Note that simulations were run for the entire period but were mostly evaluated for 1997–1998 based on variability of observed data for subwatersheds. Each station was input with its geographic reference because SWAT assigns weather data based on the nearest station location to each subwatershed. For CE-Qual-W2, hourly weather data were utilized from the Waco Regional Airport and included average temperature, humidity, precipitation, wind speed, wind direction and sky conditions from 1 January 1997 to 31 December 1998.
Hydrologic calibration of SWAT followed the CitationNeitsch (2002b) guidelines. Hydrologic calibration and confirmation of the model on a daily basis focused on 3 subwatersheds (North Bosque, Hog Creek and Middle Bosque) watershed using available stream flow data from USGS records for 1994–1998. Note that the simulation data for 1996 were ignored as a precalibration phase of the modeling. Calibration accuracy was based on least-squares regression modeling between daily observed and predicted stream flow values. Criteria for acceptable calibration was set at r 2> 0.7, coefficient of efficiency (COE) >0.1, and residual error < 25% based on CitationNash and Sutcliffe (1970). Calibration accuracy of inorganic nutrients (i.e., PO4-P, NH4-N, NO2+NO3-N) predicted by SWAT was also based on least-squares regression modeling between monthly grab samples taken from the North Bosque River near Valley Mills, Texas, from 1997 to 1998 by Texas Institute for Applied Environmental Research (TIAER), and synoptic, predicted values. Criteria for acceptable nutrient calibration was set at r 2> 0.8, COE >0.7 and residual error <10%.
The CE-Qual-W2 model was calibrated using semimonthly chl-a, total phosphorus (TP) and total nitrogen (TN) data collected by TIAER from 1997–1998. Total phosphorus and nitrogen were compared here because CE-Qual-W2 simulates these values, and the total nutrient concentrations are considered the potential target for future management rather than the inorganic fractions. Calibration adequacy was judged based on the mean percent error (MPE) calculated as
Results
Calibration metrics for SWAT were met for all 3 major tributaries measured with r2 values ranging from 0.72 to 0.86 (). Comparison between SWAT predicted flows at the USGS Valley Mills station (#08095200) showed acceptable correlation for the North Bosque River, important as a primary driver for the reservoir (). Comparisons of monthly predicted and TIAER-measured values PO4-P and NO2+NO3-N values at the mouth of the North Bosque River as it empties into the Lake Waco reservoir were also favorable ( and , respectively). The North Bosque River is the largest contributor of both stream flow and nutrients into Lake Waco, accounting for 72% of stream flow, 79% of inorganic nitrogen and 88% of inorganic phosphorus that is loaded into the reservoir (). Utilizing the estimates of organic matter also shows that the North Bosque adds 1,422,000 kg/yr of TN and 300,080 kg/yr of TP, which is comparable but slightly higher than CitationMcFarland and Hauck (1999) loading estimates of 1,263,706 kg/yr for TN and 268,816 kg/yr TP. The Hog Creek, Middle Bosque and South Bosque watersheds account for roughly 25% of stream flow but only 19% of TN and 10.4% of TP. The direct drainage into Lake Waco accounts for 3% of stream flow and 2% of both TN and TP.
Table 2 Calibration statistics for the SWAT simulations based on a daily timestep for sub-watersheds of Lake Waco. The coefficient of efficiency (COE) was calculated based on CitationNash and Sutcliffe (1970). Time intervals vary among sub-watersheds based on availability of stream flow data.
Table 3 Percent watershed contribution of stream flow, total nitrogen and total phosphorus to Waco Lake as simulated with calibrated SWAT model for 1997–1998.
Figure 4 SWAT predicted (solid line) and observed (open circles) stream flow for the North Bosque River at Valley Mills for the calibration period between Oct 1996 and Sep 1997. Values are in m3/s, and the y-axis is shown in a natural logarithmic scale.
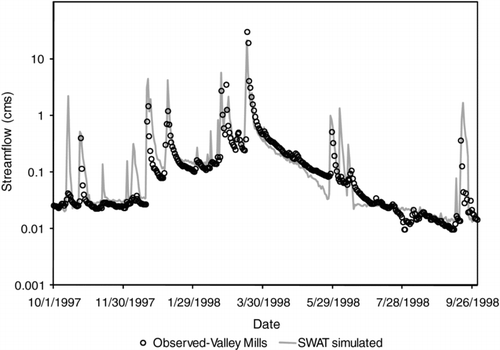
Figure 5 Comparisons of SWAT predicted and observed orthophosphorus-P (kg/d) for collections dates in 1997–1998 for the North Bosque River at the inlet to Waco Lake.
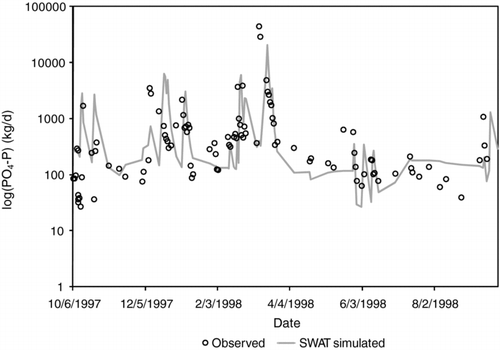
Figure 6 Comparisons of SWAT predicted and observed nitrite plus nitrate-N (kg/d) for collections dates in 1997–1998 for the North Bosque River at the inlet to Waco Lake.
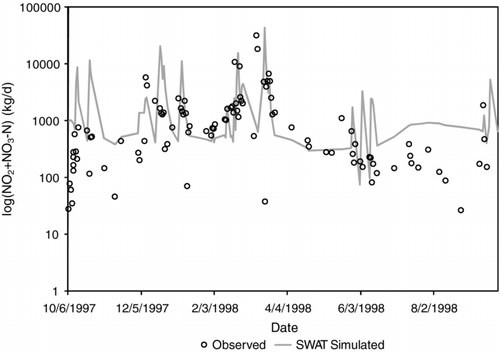
The analysis of more than 180 individual CE-Qual-W2 simulations showed that the best set of model parameters predicted chl-a and MPE values of 6.6% with an MSE of 9.76 μg/L (). This is also compared to TP and TN, which had MPE values of 14.5% and 3.7%, respectively, and RMSE values of 0.11 mg/L and 0.90 mg/L, respectively. This final set of parameters was used to simulate and analyze other physical and biological outputs predicted by CE-Qual-W2 based on the calibrated SWAT-predicted inflows. Surface temperature estimated from the main body of the reservoir showed high correspondence with observed values for the same location (). Dissolved oxygen values were similar, though there were some differences noted in the first year of the simulation (). The predicted and observed algal biomass values within the lake as measured by chl-a compare favorably () as indicated by the MPE analysis. Predicted and observed values of PO4-P () and NO2+NO3-N () showed that predicted values were higher. Average predicted PO4-P values were 0.06 mg/L compared to observed values of 0.02 PO4-P mg/L,and predicted NO2+NO3-N values were 0.58 mg/L compared to observed values of 0.38 mg/L. Comparison of timing of nutrient pulses between predicted and observed PO4-Pand NO2+NO3-N were similar, with NO2+NO3-N demonstrating the higher fidelity between the 2 nutrients.
Figure 7 Error assessment based on the root mean squared error (RMSE) analysis for calibration of the CE-Qual-W2 simulations. Simulation iteration number is shown on the x-axis, and MSE between observed and simulated chl-a is shown on the y-axis. The parameter set associated with simulation iteration #43 produced the lowest MSE value of 9.76 μg/L and was used for all subsequent analyses.
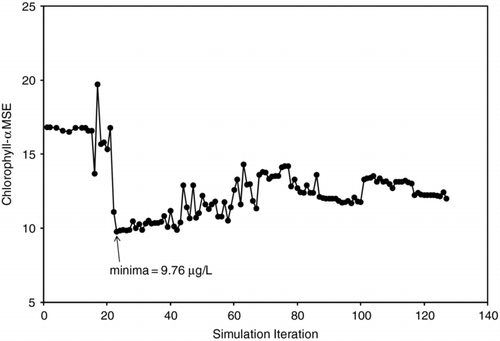
Figure 8 Comparison between observed (open circles) and simulated (solid line) water temperature (C) at 1 m for collections dates in 1997–1998 near the center of Waco Lake.
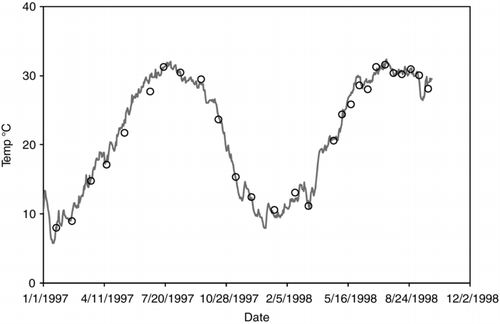
Figure 9 Comparison between observed (open circles) and simulated (solid line) dissolved oxygen (mg/L) at 1 m for collection dates in 1997–1998 near the center of Waco Lake.
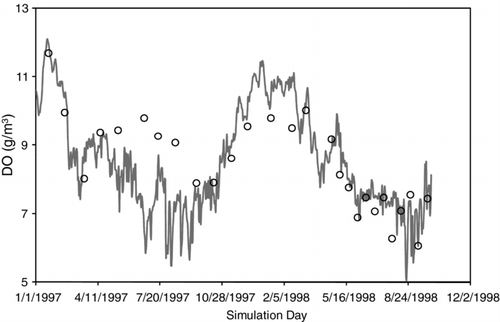
Figure 10 Comparison between observed (open circles) and simulated (solid line) chl-a (mg/m3) at 1 m for collections dates in 1997–1998 near the center of Waco Lake.
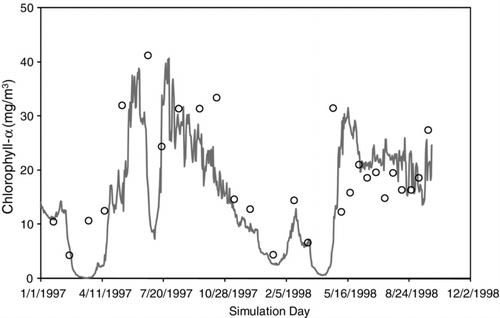
Discussion
Models for watershed and receiving water bodies have developed separately as a function of differences in terrestrial and aquatic processes and physical representation of these 2 systems. However, coupled modeling systems are essential for developing best management practices under current and future climate landscapes. Coupled models provide a secondary source of information about potential variability in water quality measures, which help fill in sampling gaps and provide a more comprehensive estimation of baseline conditions (CitationWang et al. 2005). The current version of SWAT (SWAT2005, CitationNeitsch et al. 2005) contains the 2-dimensional version of the CE-Qual-W2 model that allows SWAT users to estimate nutrient budgets and algal production in lakes and reservoirs embedded in the GIS framework required for SWAT. However, simulations of reservoirs and lakes with complex morphology can be difficult due to nonlinear formulas used to estimated changes in water movement and nutrient cycling (CitationFlowers et al. 2001). For SWAT, accurate hydrologic gauge data are essential for calibration due to the importance of base flow as an indicator of watershed function (CitationSanthi et al. 2001). In addition, attributes of GIS data, such as soils, can influence calibration efficiency. Early in this study, attempts to calibrate the SWAT model were unsuccessful due to errors in the digital soils data. The STATSGO database soil layers in the upper watershed were found to reference soil characteristics only found in south Texas and incorrectly produced an aquitard in the soil profile. This was corrected, allowing successful calibration of hydrologic discharge and subsequent sediment and nutrient yields.
Calibration of water quality parameters for both watershed and reservoir models were important for predicting temperature, dissolved oxygen, chl-a and nutrients in the reservoir as the end-point of the study's hydrologic system. Successful calibration of the SWAT model involved modifying hydrologic parameters for the watershed and nutrient kinetic parameters within the stream waters. An initial comparison of SWAT-predicted and observed stream flows using a hydrograph filter algorithm (CitationArnold and Allen 1999) indicated that SWAT overestimated the amount of water derived from overland or surface flow. Studies on adjacent watersheds in the study area region show similar results, which is attributed to over-predicted peak flows during flood events (CitationSanthi et al. 2001, CitationLarose et al. 2007). To adjust the stream flow, the time lag for surface runoff was increased in the model from the default value of 4–6 days, and runoff curve numbers for different landuse types were reduced by 10%. This allowed longer retention of water on the landscape with water transfer to lower levels in the soil and groundwater, which increased baseflow in the predicted hydrograph. Both of these adjustments were approximations of model parameters that are difficult to physically measure at this spatial extent. Because hydrologic discharge is a transport mechanism for nutrient inputs into waters, the predicted timing and magnitude were important to emulate for best prediction of nutrient delivery and concentrations.
For stream nutrient modeling in SWAT, sediment evolution of PO4-P and NH4-N were both decreased from the default value of 0.05 mg/m2/d to 0.001 mg/m2/d. In addition, nitrogen settling into an established benthos was reduced from 0.05 to 0.001 mg/m2/d. These reductions account for the presence of a solid limestone stream bottom found in much of the upper watershed reaches. Streams in the watershed have well-scoured bottoms for which a typical muddy sediment profile has not developed. Little nutrient release from the benthos likely occurs, and decreasing these parameters in the model increased the accuracy of stream nutrient predictions.
In the reservoir, representing algal dynamics dominated calibration accuracy of the CE-Qual-W2 model. The modeling philosophy was to characterize functional algal groups that could individually occupy all environmental space dynamically in the reservoir rather than to attempt to emulate specific taxonomic groups. In a previous modeling study of Lake Waco reservoir, researchers had some difficulty calibrating CE-Qual-W2 utilizing SWAT-predicted inflows of nutrients (CitationFlowers et al. 2001). Our study demonstrated acceptable calibration of these linked models that we attribute to the number of algal groups simulated. The addition of both winter and summer diatoms resulted in the persistence of algal biomass throughout the year (), similar to observations associated with specific growth temperatures (CitationBouterfas et al. 2002). The additional algal group provided stability in modeled nutrient cycling because large fluctuations in algal productivity increased organic matter production and settling, which tended to sequester nutrients in the modeling system rather than release them at intermediate time scales.
Figure 13 Temperature growth scalars for the four algal groups represented in the CE-Qual-W2 simulations for Waco Lake.
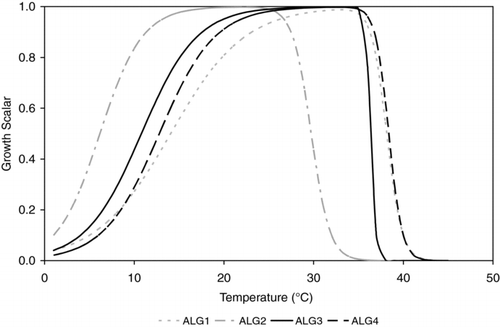
Lake Waco is characterized as a polymictic water body having very “muddy waters.” The importance of suspended sediments for algal growth is light attenuation. Initial simulations had limited algal growth due to light limitations associated with using the default growth parameter, light half saturation, set at 75 W/m2. Lichtman et al. (2003) showed that algae had light half saturation values ranging from 100 to 155 W/m2. Final light half saturation values for the calibrated model were 105, 70, 90 and 100 W/m2 for the green algae, winter diatoms, cyanobacteria, and summer diatoms, respectively. As with addition of the summer diatoms, increasing light response for algal groups increased persistence of algae in the water and buffered simulated nutrient cycling rates.
Simulated nutrient dynamics are associated with algal growth rates and are affected by kinetic coefficients. For P and N uptake, values derived by CitationDávalos-Lind and Lind (1998) were reasonable and provided model stability with regard to algal response to limiting nutrients and nutrient removal from the water column. However, N half saturation constants for the cyanobacteria group were minimized, set here at a value of 0.002 mg/L to represent the nitrogen-fixing capability of this algal group. Also, the cyanobacteria group was modeled with a higher affinity for NH4 than other algal groups (CitationKuwata and Miyazaki 2000). This increased predicted productivity of this algal group during late summer and early fall when NO2 and NO3 had lowered concentrations in the reservoir ().
Differences between predicted and observed PO4-P are expected because of the transient nature of this compound in solution due to rapid release and uptake by phytoplankton (). The observed PO4-P values are the instantaneous values that are compared to daily average simulated values. Transport of inorganic P through the lake is also affected by P accumulation to and release from lake sediments and the adsorption of P onto inorganic suspended particles. Sediment release of P under anaerobic conditions was set to 0.0 because investigation showed no release from sediments (CitationEsten and Wagner 2010). Lake Waco exhibits continual mixing; therefore anaerobic conditions at the reservoir bottom are rare, and P release from sediments did not occur in these simulations.
Both P and N were overestimated on average and during peak storm flows to the reservoir. For example, on 10 March 1998, large storms in the western portion of the watershed resulted in high stream flow and nutrient loading, which was adequately simulated by the SWAT for the North Bosque River (). Simulated reservoir P and N were too high during this event likely due to (Equation1) the lack of modeled sorption to clay particles and (Equation2) concentration of N and P delivered to the reservoir in organic matter. Phosphorus adsorption can occur as clay particles and can provide an active site for PO4 binding. Precipitation-delivered nutrient additions to the reservoir were modeled utilizing TIAER annual average values of 0.022 mg PO4-P/L, 0.369 mg NH4-N/L and 0.274 mg NO2+NO3-N/L for every precipitation event. This static input could also overestimate actual deposition due to high seasonal variability in these constituents (CitationAdams and McFarland 2002). However, nutrient loads from atmospheric deposition derived from the simulation accounted for only 0.9 and 1.0% of the TP and TN loads, respectively. Errors in nutrient inputs via precipitation are likely to be inconsequential.
Considering the high concentrations of nutrients flowing into the lake, the role of internal recycling should be diminished as compared to natural lakes. One issue for future consideration is the temporal variation in organic matter nutrient stoichiometry. Material entering the reservoir may differ in nutrient composition dependent on sources. In this model, organic matter was predicted for inflow based on SWAT-predicted organic P utilizing a constant stoichiometry of 63:1. Under baseflow conditions, OM may come from consistent sources such as soil leachates (CitationRasmussen et al. 1989) or WWTP. Under storm flow events, organic debris may be carried from overland flow along riparian zones, breached wastewater lagoons and eroded stream banks. These sources have varying amounts of N and P and varying OM:OP ratios, whereas static values were utilized here. Because OM is a significant source of latent nutrients for the reservoir, use of a constant value could partially explain some of the over- and under-estimation of N and P in the simulations and should be examined to account for temporally varying values.
Acknowledgments
We thank the City of Waco, particularly Mr. Tom Conry, for his assistance for providing the funding and guidance for this study through ENSR. We thank Dr. Ken Wagner from ENSR/AECOM for his leadership in managing this project in addition to his early comments on the design of this study. Finally, we thank the anonymous reviewers who provided constructive criticism of the content and form of this manuscript in its earlier form. Any information on the parameter values utilized in this study for either model may be acquired by contacting the corresponding author of this paper.
References
- Adams , T and McFarland , A . 2002 . Semiannual Water Quality Report for the Bosque River Watershed Texas Institute for Applied Environmental Research , 108 Stephenville , TX : Tarleton State University . TR0216
- [ASAE] American Society of Agricultural and Engineers . 1999 . ASAE D384.1 DEC98. Manure production characteristics , St. Joseph , (MI) : American Society of Agricultural Engineers .
- Arnold , J G and Allen , P M . 1999 . Validation of automated methods for estimating base flow and groundwater recharge from stream flow records . J. Am Water Resour. Assoc. , 35 : 411 – 424 .
- Arnold , J G and Fohrer , N . 2005 . SWAT2000: Current capabilities and research opportunities in applied watershed modeling . Hydrol Process. , 19 : 563 – 572 .
- Arnold , J G , Neitsch , S L and Williams , J R . 1999 . Soil and Water Assessment Tool user's manual , Temple , (TX) : USDA-ARS Grassland Soil and Water Research Laboratory . Version 98.1
- Arnold , J G , Williams , J R , Srinivasan , R and King , K W . 1996 . SWAT: Soil and Water Assessment Tool , Temple , (TX) : USDA-ARS Grassland Soil and Water Research Laboratory .
- Borah , D K , Yagow , G , Saleh , A , Barnes , P L , Rosenthal , W , Krug , E C and Hauck , L M . 2006 . Sediment and nutrient modeling for TMDL development and implementation . Trans Am Soc Agric Biol Eng , 49 : 967 – 986 .
- Bouterfas , R , Belkoura , M and Dauta , A . 2002 . Light and temperature effects on the growth rate of three freshwater algae isolated from a eutrophic lake . Hydrobiologia , 489 : 207 – 217 .
- Bull , W B . 1991 . Geomorphic responses to climate change , 326 New York : Oxford University Press .
- Cole , T M and Wells , S A . 2000 . CE-Qual-W2: A two-dimensional, laterally averaged, Hydrodynamic and Water Quality Model, Version 3.0 , Vicksburg , (MS) : US Army Engineering and Research Development Center . Instruction Report EL-2000
- Dávalos-Lind , L D and Lind , O T . 1998 . The algal growth potential of and growth-limiting nutrients in Lake Waco and its tributary waters , Waco , (TX) : Department of Biology, Baylor University, Limnology Laboratory . An interim report to TIAER
- Debele , B , Srinivasan , R and Parlange , J-Y . 2008 . Coupling upland watershed and downstream waterbody hydrodynamic and water quality models (SWAT and CE-QUAL-W2) for better water resources management in complex river basins . Environ Model Assess. , 13 : 135 – 153 .
- Esten , M E and Wagner , K J . 2010 . Investigation of benthic phosphorus flux controls in Lake Waco, TX . Lake Reserv Manage , 26 : 114 – 122 .
- Flowers , J D , Hauck , L M and Kiesling , R L . 2001 . Water quality modeling of Lake Waco using CE-QUAL-W2 for assessment of phosphorus control strategies , 76 Stephenville , (TX) : Tarleton State University. Institute for Applied Environmental Research . TR0114
- Grant , W E , Pedersen , E K and Marin , S L . 1997 . Ecology and natural resource management: Systems analysis and simulation , 373 Chichester , (GB) : John Wiley .
- Hamilton , D P and Schladow , S G . 1997 . Prediction of water quality in lakes and reservoirs . Part I – Model description. Ecol Model. , 96 : 91 – 110 .
- Kier , R S , Garner , L E and Brown , L F . 1977 . Land resources of Texas, special report , 45 Austin , (TX) : University of Texas at Austin, Bureau of Economic Geology .
- Kuwata , A and Miyazaki , T . 2000 . Effects of ammonium supply rates on competition between Microcystis novacekii (Cyanobacteria) and Scenedesmus quadricauda (Chlorophyta): simulation study . Ecol Model. , 135 : 81 – 87 .
- Larose , M , Heathman , G C , Norton , L D and Engel , B . 2007 . Hydrologic and atrazine simulation of the Cedar Creek watershed using the SWAT model . J Environ Qual. , 36 : 521 – 531 .
- Litchman , E , Steiner , D and Bossard , P . 2003 . Photosynthetic and growth responses of three freshwater algae to phosphorus limitation and daylength . Freshw Biol. , 48 : 2141 – 2148 .
- McFarland , A M and Hauck , L M . 1999 . Existing nutrient sources and contributions to the Bosque Watershed , Stephenville , (TX) : Tarleton State University, Texas Institute of Applied Environmental Research . Pr 9911
- McFarland , A M , McFarland , M J and Sweeten , J M . 2000 . Dairy lagoon design and management under chronic rainfall . Appl Eng Agric. , 16 : 285 – 292 .
- Montagnes , D J and Franklin , D J . 2001 . Effect of temperature on diatom volume, growth rate, and carbon and nitrogen content: Reconsidering some paradigms . Limnol Oceanogr. , 46 : 2008 – 2018 .
- Moore , R B , Johnston , C M , Robinson , K W and Deacon , J R . 2004 . Estimation of total nitrogen and phosphorus in New England streams using spatially referenced regression models USGS Scientific Investigations Report 2004–5012
- Nash , J E and Sutcliffe , J E . 1970 . River flow forecasting through conceptual models, Part I, A discussion of principles . J Hydrol. , 10 : 282 – 290 .
- Neitsch , S L , Arnold , J G , Kiniry , J R , Williams , J R and King , K W . 2002a . Soil and Water Assessment Tool dheoretical Documentation: Version 2000 , College Station , (TX) : Texas Water Resources Institute . TWRI Report TR-191
- Neitsch , S L , Arnold , J G , Kiniry , J R , Srinivasan , R and Williams , J R . 2002b . Soil and Water Assessment Tool user's manual: Version 2000 , College Station , (TX) : Texas Water Resources Institute . TWRI Report TR-192
- Neitsch , S L , Arnold , A G , Kiniry , J R , Srinivasan , J R and Williams , J R . 2005 . Soil and Water Assessment Tool user's manual: Version 2005 , College Station , (TX) : Texas Water Resources Institute . TR-192
- Reed-Andersen , T , Carpenter , S R and Lathrop , R C . 2000 . Phosphorus flow in a watershed-lake ecosystem . Ecosystems , 3 : 561 – 573 .
- Rasmussen , J B , Godbout , L and Schallenberg , M . 1989 . The humic content of lake water and its relationship to watershed and lake morphometry . Limnol Oceanogr. , 34 : 1336 – 1343 .
- Santhi , C , Arnold , J G , Williams , J R , Dugas , W A , Srinivasan , R and Hauck , L M . 2001 . Validation of the SWAT model on a large river basin with point and nonpoint sources . J Am Water Resour Assoc. , 37 : 1169 – 1188 .
- Sullivan , S , Thomas , D , Elliott , W and Segura , S . 1995 . Volumetric survey of Waco Lake , 15 Austin , (TX) : Texas Water Development Board .
- Summer , R M , Alonso , C V and Young , R A . 1990 . Modeling linked watershed and lake processes for water quality management decisions . J Environ Qual. , 19 ( 3 ) : 421 – 427 .
- Tong , S T and Chen , W . 2002 . Modeling the relationship between land use and surface water quality . J Environ Manage , 66 : 377 – 393 .
- Umorin , M . 2006 . Phytoplankton growth model for a turbid pulsed aquatic ecosystem , PhD dissertation 156 Baylor , (TX) : Baylor University, Dept. of Biology .
- Wang , S H , Huggins , D G , Frees , L , Volkman , C G , Lim , N C , Baker , D S , Smith , V and deNoyelles , F . 2005 . An integrated modeling approach to total watershed management: Water quality and watershed management of Cheney Reservoir, Kansas, USA . Water Air Soil Pollut. , 164 : 1 – 19 .
- Wetzel , R G . 1984 . Detrital dissolved and particulate organic carbon functions in aquatic ecosystems . B Mar Sci. , 35 : 503 – 509 .
- Wilkie , A C and Mulbry , W W . 2002 . Recovery of dairy manure nutrients by benthic freshwater algae . Bioresour Tech. , 84 : 81 – 91 .
- Xu , Z , Godrej , A N and Grizzard , T J . 2007 . The hydrological calibration and validation of a complexly-linked watershed-reservoir model for the Occoquan watershed, Virginia . J Hydrol. , 345 : 167 – 183 .