Abstract
Water age and thermal structure of Lake Mead were modeled using the 3-dimensional hydrodynamic Environmental Fluid Dynamics Code (EFDC). The model was calibrated using observed data from 2005 and then applied to simulate 2 scenarios: high-stage with an initial water level of 370.0 m and low-stage with a projected initial water level of 320.0 m. The high-stage simulation described predrought lake hydrodynamics, while the low-stage simulation projected how lake circulation could respond under significant lake drawdown, should drought conditions persist. The results indicate that water level drawdown plays an important role in thermal stratification and water movement of Lake Mead during receding water levels. The impact of the dropping water level on lake thermal stratification is more significant in shallow regions such as Las Vegas Bay. Depth-averaged (the mean value of 30 vertical layers) water temperature in the low-stage was estimated to increase by 4–7 C and 2–4 C for shallow (<20 m) and deep (>70 m) regions, respectively. Further, depth-averaged water age decreased about 70–90 d for shallow regions and 90–120 d for deep regions under the simulated drought scenario. Such changes in temperature and water age due to continuous drought will have a strong influence on the hydrodynamic processes of Lake Mead. This study provides a numerical tool to support adaptive management of regional water resources by lake managers.
Large lakes and reservoirs in arid and semi-arid regions are prone to substantial fluctuations in water surface elevations due to cyclic climate patterns and increasing water demands. This phenomenon is very apparent in Lake Mead, Nevada, United States, where water surface elevations have dropped approximately 35 m since its modern peak of 370 m in 2000 (LMWD 2009). Understanding how lake hydrodynamic processes and characteristics are likely to change under receding water elevations is critical to supporting adaptive management of these systems under unprecedented conditions.
Changes in the water level of lakes, especially significant drawdown, play an important role in a lake's physical, hydrological and ecological processes (CitationBrauns et al. 2008). Recently, much attention has been paid to the impact of water level fluctuations (WLF) on socioeconomic and ecological processes in rivers (CitationJunk and Wantzen 2004) and lakes, including the Aral Sea (CitationUsmanova 2003), Lake Chad (CitationCoe and Foley 2001) and the Salton Sea (CitationBourne et al. 2005), among others. The literature describes how WLF affect the ambient environment (e.g., physical environment, landscape) and how WLF are projected to be impacted by climate change as well as how to manage and address WLF. In general, WLF will affect water circulation patterns and temperature regimes of lakes, changes in lake morphometry, and will eventually have effects on aquatic habitat, water quality and algal growth (CitationWantzen et al. 2008).
Water level fluctuations can result from anthropogenic disturbances, natural hydrologic fluctuations (CitationHofmann et al. 2008) or a combination of all of these processes, which is the case with Lake Mead. Anthropogenic disturbances are connected to the construction of dams and reservoirs for hydropower production and flood control, water abstraction for irrigation and other water uses (CitationDynesius and Nilsson 1994). Hydrologically induced WLF are associated with climatic changes, including reduced winter snowpack and rainfall and increased evaporation (CitationBrennwald et al. 2004). However, much of the previous research has concentrated on the impacts of WLF on the hydrological and ecological processes in rivers, large shallow lakes or small reservoirs (CitationNash and Gleick 1991, CitationHoerling and Eischeid 2000, CitationWantzen et al. 2008). As far as Lake Mead is concerned, these impacts are not well understood.
Lake Mead is the largest man-made reservoir in the United States, with an area of 635 km2 and a total volume of 35.5 km3. It was formed by the construction of Hoover Dam in the Black Canyon of the Colorado River in the 1930s (). Lake Mead provides recreational opportunities, fish and wildlife habitat, and drinking, irrigation and industrial water for approximately 25 million people (NASA 2003). Approximately 96% of the inflow water of Lake Mead comes from the Colorado River, and the outflow is similar from year to year (NASA 2003). Due to the sustained decrease in runoff from the Colorado River because of extended drought and over allocation of the available water resources, outflow has exceeded the inflow in Lake Mead for approximately a decade, resulting in a sharp decrease (about 35 m) in the water level since 2000. Further, the Intergovernmental Panel on Climate Change (IPCC) Working Group II concludes with very high confidence that there will be a 10–30% runoff reduction over some dry regions at mid-latitudes, including the Colorado River Basin, during the next 50 years. The projected decrease in runoff is expected to result from increasing air temperatures and evapotranspiration rates and declining precipitation (IPCC 2008). CitationBarnett and Pierce (2008) also estimated a 50% chance that Lake Mead will reach minimum power pool level by 2017 and become functionally dry by 2021 if the climate change continues as projected and future water use is not limited to less than current river compact levels.
Figure 1 Lake Mead (source: http://earthobservatory.nasa.gov/Features/LakeMead). Numbers 1, 2 and 3 represent the in-lake real-time monitoring sites named Las Vegas Bay, Sentinel Island and Virgin Basin, respectively.
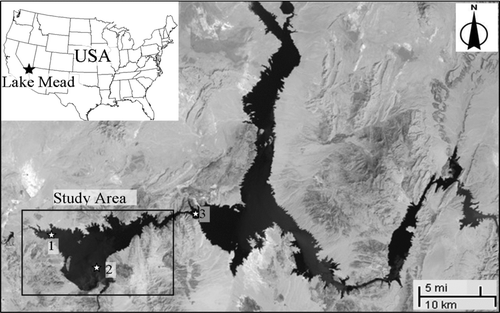
To manage this valuable water resource in the face of high uncertainty, it is useful to develop both an improved conceptual understanding and numerical models to support management decisions. Models can be used to investigate projected changes in spatial and temporal patterns of circulation, mixing, density stratification and ecological processes. Moreover, numerical models can provide a useful method to analyze the impact of different water availability and management scenarios. Recent research on Lake Mead has primarily focused on field measurements and lab experiments (e.g., CitationLaBounty and Burns 2007, CitationSteinberg et al. 2009). As for the present state of numerical models of Lake Mead, most have been conducted to predict hydrodynamic processes and water quality to understand past and present limnological conditions (SCOPEIS 2006). However, investigations of hydrodynamic responses to severe drops in water levels in Lake Mead have not been reported in the literature.
The objective of this study is to present a numerical tool to support adaptive management of Lake Mead by regional water resources and lake managers. In this study, water age and the thermal structure of Lake Mead were simulated and compared using the 3-dimensional (3-D) hydrodynamic Environmental Fluid Dynamics Code (EFDC) under both high- and low-stage conditions.
Methods
Model description
The thermal structure and water age of Lake Mead were simulated using the hydrodynamics and water quality modules of the EFDC. The details of the EFDC model are documented by CitationHamrick (1992). The model has been extensively applied to simulate circulation, thermal stratification, sediment transport, water quality and eutrophication processes in numerous lakes, rivers and estuaries (e.g., CitationJi et al. 2007, CitationElci et al. 2009).
Water age is defined as “the time that has elapsed since the particle under consideration left the region in which its age is prescribed as being zero” (CitationDelhez et al. 1999). More specifically, the age is zero at the entrance of the tributaries to the lake and the age at any specified location is representative of the time elapsed for a water particle to be transported from its boundary to that location (CitationShen and Wang 2007). In contrast, water residence time for each material element is defined as the time taken for the water particle to reach the outlet (CitationZimmerman 1976). Alternatively, water residence time is how long water particles, starting from a given location within a waterbody, will remain in the waterbody before exiting. Therefore, the concepts of residence time and water age are complementary (CitationTakeoka 1984). In this study, temperatures were simulated by using the surface heat exchange algorithm from CE-QUAL-W2 (version 3.1; Cole and Wells 2005), while water age was computed based on tracer and age concentrations as (CitationJi et al. 2007)

The above equations were solved with specified initial and boundary conditions.
Mesh generation
The study area was restricted to Boulder Basin due to the significance of this region to the City of Las Vegas (the most populous and rapidly expanding city in Nevada, USA) and the downstream areas, and the complex bathymetry associated with developing a whole Lake Mead model. However, because the surface area of Boulder Basin accounts for approximately one-third of Lake Mead, Boulder Basin provides a good representation of the whole lake. Boulder Basin has 2 primary inflows: the Las Vegas Wash (LVW), which drains the Las Vegas Valley; and the Colorado River via “the Narrows.” It also has 2 principal outflows: the Colorado River via Hoover Dam and the water intake for Southern Nevada near Saddle Island.
In this study, the lake bathymetry () was specified using recently conducted side-scan sonar imagery and high-resolution seismic-reflection profiles collected by the United States Geological Survey (USGS) and the University of Nevada, Las Vegas (UNLV; Twichell et al. 2003). A Cartesian computational mesh was generated using the EFDC-Explorer3 pre-processor and constructed in a rectangular and vertical sigma-stretched coordinate system. The mesh contained 3512 cells in the horizontal plane with a uniform grid size of 216 m, and 30 uniform layers along the vertical direction. Each vertical cell thickness was equal to the local water depth divided by the number of vertical layers (e.g., a 30 m water column would have 1 m layers; a 60 m water column would have 2 m layers).
Initial and boundary conditions
Initial conditions included water surface elevations, water column and bed temperatures, while boundary conditions consisted of atmospheric forcing, surface wind stress and lake inflows and outflows. Water column and lake bottom temperatures were initialized with observed values obtained at the USGS water quality monitoring platforms at Las Vegas Bay Station (LVB, USGS station number 3607001145051) and Sentinel Island Station (SI, USGS station number 3603141144505), when computational time equals zero (). Water temperatures for the LVW and the Colorado River inflows were obtained at the USGS monitoring platforms at LVB (USGS station number 3607001145051) and upstream of the Narrows (Virgin Basin Station, USGS station number 3609011143210), respectively. The platforms record water temperature profiles every 6 h. Daily water surface elevations for 2000 and 2005 were obtained from the US Bureau of Reclamation. Meteorological data were also obtained from the SI USGS monitoring platforms and included solar radiation, wind speed and direction, relative humidity, air temperature and barometric pressure. Lake inflow and outflow data were derived from USGS gauges on the LVW (USGS station number 09419700) and Hoover Dam (USGS station number 09421500), and the US Bureau of Reclamation reported pumping rates for the intake structure located near Saddle Island (CitationVermeyen 2003; ). Because the flow rates entering Boulder Basin from the Colorado River through the Narrows are not directly measured, daily flow rates through the Narrows were calculated by the principles of water mass balance of the lake, including net flows into the lake, precipitation, evaporation, the rate of change of the lake water level and the Boulder Basin depth-capacity curve. The estimated flows at the Narrows were then distributed vertically within all 30 layers, assuming the horizontal resultant velocities following the traditional logarithmic law along the vertical profile. Due to the extremely complex and dynamic outlet conditions at Hoover Dam, we simplified the structural boundary to a flow boundary and represented it as a logarithmic vertical profile at the 2 southernmost grid cells. Considering that the southernmost boundary grids in the computing domain are located about 400 m upstream of Hoover Dam, the assumption should have only a minor influence on the modeling results. For the boundary condition of water age, the tracers with a unit concentration of 1 (arbitrary units) were continuously released at the entrances of the LVW and the Colorado River through the Narrows.
Model calibration
Lake stage and temperature profiles at SI () between 1 January and 31 December 2005 were used to calibrate the EFDC model. The main calibrated parameters included horizontal and vertical eddy viscosities and diffusivities, bottom roughness height, the wind sheltering coefficient (which affects the hydrodynamic process) and several parameters related to the temperature simulation (). The turbulence parameters related to the Mellor-Yamada turbulence model (CitationMellor and Yamada 1982, CitationGalperin et al. 1988) were treated as constants, and their values were consistent with those used widely in other hydrodynamic models, such as the Princeton Ocean model (CitationMellor 1990) and the Estuary, Coastal and Ocean model (HydroQual 2001). A stepping time step, usually ranging from 1.0 to 15.0 sec, was used in this study rather than a fixed time step. To adapt to the decline and fluctuation of water levels, a moving water surface boundary was applied in the model by assigning a critical dry water depth (0.5 m) and the wetting/drying procedure proposed by CitationHamrick (1994). Although the bottom roughness height (z 0) is often adjusted for water level calibration, in this study it was set as a typical value of 0.02 m (CitationHamrick 1992, HydroQual 2001). The comparison of time series of lake water levels between observed values and modeled results at Sentinel Island closely agreed with the observed data ().
Figure 3 Time series of the simulated water level (solid line) and the observed data (dotted points) at Sentinel Island in 2005.
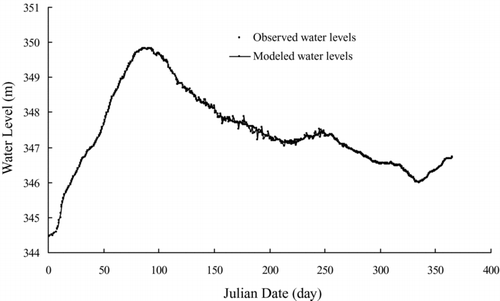
Table 1 Calibration parameters for the Lake Mead hydrodynamic simulation.
Temperature profiles of the water column in Lake Mead were investigated by numerical modeling and field measurements at LVB and SI on Days 100, 200 and 300 in 2005 (). The measured data showed that the stratification at Day 200 (19 July) ended at approximately 15 m at LVB (shallow region) and 40 m at SI (deep region). The temperature difference between the surface and the bottom layer was 7 C for LVB and 16 C for SI; however, the temperature profile on Day 100 and 300 for both LVB and SI were relatively uniform compared to Day 200. In both the shallow and deep lake regions, the model could capture the vertical thermal structure and turnover cycling processes; however, the simulated temperatures at the lower layers with depths of 30 to 40 m at Day 200 at SI were slightly larger than the observed data. The calibrated and monitored temperature time series were compared at 3 water layers at SI (), including the surface layer (1 m below water surface), middle layer (30 m below water surface) and bottom water layer (75 m below water surface). The results indicated that a strong agreement was achieved for the calibrated temperature time series at the top and middle water layers. However, the calibrated temperature time series at the bottom water layer diverged to a certain extent from the observation. The error is believed to be from the pressure gradient error caused by the sigma coordinate transformation. The sigma pressure gradient error is discussed in detail in the discussion section.
Figure 4 Comparison of simulated temperature profile (solid line) and the observed data (dotted points) at Las Vegas Bay (LVB) and Sentinel Island (SI) on Day 100, 200 and 300 in 2005. (a) LVB at Day 100; (b) LVB at Day 200; (c) LVB at Day 300; (d) SI at Day 100; (e) SI, Day 200; (f) SI at Day 300.
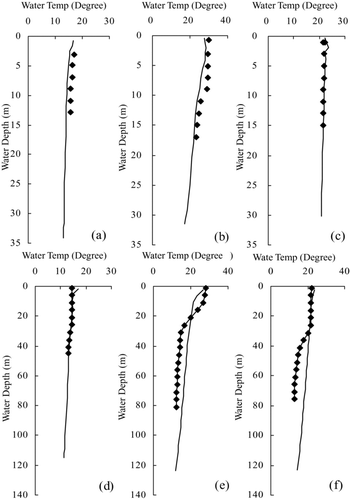
Figure 5 Time series of temperature calibration results at surface, middle and bottom water column from 1 January to 31 December 2005 at Sentinel Island.
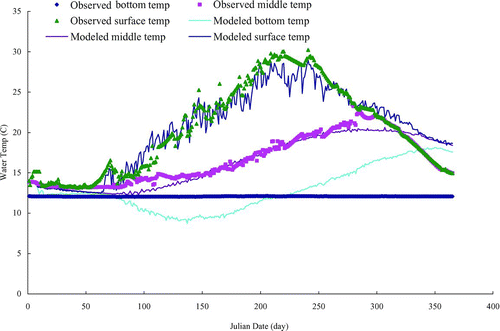
To quantify the errors and assess the calibration performance, the Absolute Mean Error (AME) and Mean Absolute Relative Error (MARE) were used to assess the performance of the model due to its direct interpretability.
The calculated AME and MARE for water level errors were 0.084 m and 0.02%, respectively. The AME for surface, middle and bottom water temperatures were 1.51, 1.04 and 1.42 C, respectively. Correspondingly, the water temperature MARE for surface, middle and bottom were 7.3, 6.9 and 10.9%. The results suggested that the calculated values agreed well with the simulations.
Application of the Lake Mead model
The calibrated model was applied to calculate water ages and thermal structures in Lake Mead under two scenarios: (Equation1) a high-stage situation with an initial water level of 370.0 m, corresponding with the condition observed in 2000 (LMWD 2009) and (Equation2) a low-stage scenario with an initial water level 320.0 m, which is the minimum power pool level for Lake Mead, corresponding with a condition projected in the year 2017 by CitationBarnett and Pierce (2008). This condition represents one possible scenario should the current drought condition on the Colorado River continue into the next decade. The total drop of water level is approximately 50 m, and the water volume decrease is 18.5 km3 (from 30.8 to 12.3 km3) between the 2 scenarios (LMWD 2009). The maximum water depth would drop from 150 to 100 m (). Thus, the decline in water depth, volume and water surface area would be approximately 35, 50 and 40%, respectively, between the 2 scenarios. To assess the sole impact of water level drawdown, other boundary conditions (i.e., meteorology, discharge and initial water temperature) were assumed to be the same for the low-stage condition as those used in the high-stage scenario. Each condition was simulated for 365 d.
Characteristics of temperature and water age in Lake Mead
Temperature and water age were selected to study the impact of water level drawdown as indicative parameters of thermal regime and hydrodynamic processes. The temporal distributions of these 2 parameters were investigated over the high-stage simulation at 2 representative locations in a shallow region (site A) and a deep region (site B; ). Site A (36.47472° N; 114.80889° W) is located near the center of LVB and site B (36.06194° N; 114.74200° W) is located off the northeast corner of Saddle Island. Water depths at sites A and B were 88 and 150 m, respectively, at the initial day of the high-stage simulation. The results showed that lake temperature profiles changed seasonally, with warm and thermally stratified conditions in the summer and cool isothermal conditions in the winter (). The stratification duration was approximately 220 d for the high-stage condition. Minimum temperatures of approximately 11 C were generally found in December–February, while temperatures exceeding 28 C were persistent through much of the summer. The maximum temperature differences between surface and bottom layers during thermal stratification were approximately 18 C in deep regions and 12 C in shallow regions. The time series of water temperature for different layers of the water column (surface, middle and bottom) demonstrated that the surface water temperatures fluctuated substantially over time, while the temperature distributions were fairly uniform across the lake. However, the bottom water temperatures had slight temporal but strong spatial variations among the lake regions. During the thermal stratification period, the bottom temperatures in shallow regions (site A) were 5–8 C higher than that of deep regions. In addition, the duration of thermal stratification in shallow regions (∼200 d) was 50 d shorter than that of deep regions (∼260 d). All these simulated temperature results agreed well with the actual observations during 2000.
Figure 7 Calculated time series of temperature at sites A (a) and B (b) for the high-stage condition.
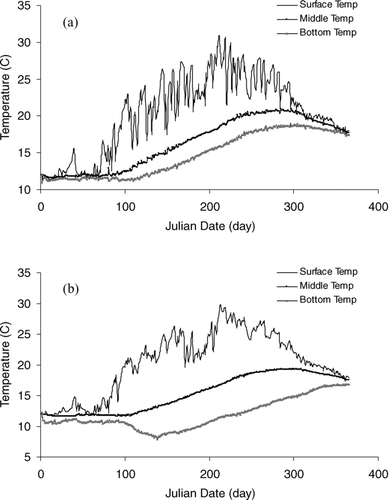
To improve understanding of transport in this complex hydrodynamic system, the water age was considered as an indicator of the transport timescales of conservative dissolved substances. The water age in Lake Mead illustrated high spatial and temporal heterogeneity. The time series of water age profiles for shallow conditions (site A; ) indicated that water age increased throughout the simulation, suggesting minimal interaction with incoming flow from the Colorado River over the simulation period. For site B (), water ages of the surface and middle layers appeared to approach their maximum values, whereas the bottom layer water age continued to increase throughout the simulation period. In general, the maximum depth-averaged (the mean value of 30 vertical layers) water age was 220 d for the shallow region (site A) and 190 d for the deep region (site B) during the high-stage simulation period. By comparing the vertical distribution of water age (), it was found that the vertical distribution of water age was quite uniform for the shallow region, whereas significant variations were found in the deep region. For example, water ages at Day 365 in the surface, middle and bottom layers of the deep region (site B) were 178, 211 and 266 d, respectively, suggesting that a higher degree of water exchange occurs in the surface water than in the bottom water.
Impact of water level drawdown on temperature stratification
Temporal patterns of water temperature were investigated over the 2 simulations at sites A and B. The results indicated that the extent and duration of thermal stratification were strongly influenced by declining water levels. Although the depth-averaged temperatures changed only slightly during winter months (Dec–Feb), there was a progressive increase in depth-averaged temperature differences from March through August (Julian days of 90 to Day 250 in Julian Date) due to the reduced water surface area. The maximum depth-averaged temperature difference (ΔT between high- and low-stage scenarios) at sites A and B were 4.3 C on 26 July (Day 208) and 2.5 C on 21 August (Day 234), respectively. Also based on the results (), the time period of lake thermal stratification will decrease from 305 d (in the high-stage condition) to 235 d (in the low-stage condition), which means it needs 70 fewer days to mix the lake completely (turnover) in the fall of the low-stage scenario.
Figure 9 Comparison of calculated time series of depth averaged temperature (a) and water age (b) at sites A and B.
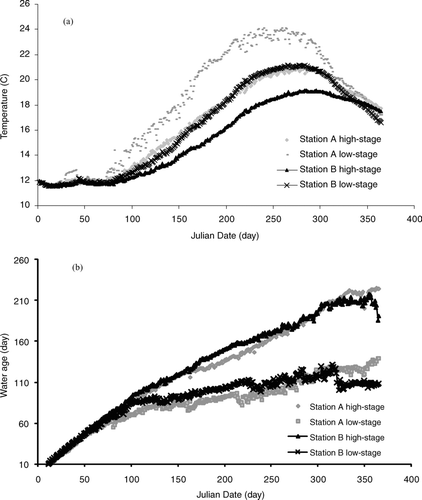
Changes in temperature (ΔT) between the 2 scenarios at the water surface, lake bottom and the depth-averaged condition were investigated during the periods with strong thermal stratification (). The results indicated large ΔTs in shallow regions. For instance, the depth-averaged water temperature increased by 4–7 C for shallow regions versus 2–4 C for deep regions. Temperature shifts were influenced by location in the water column. For example, for the depth-averaged water temperatures (), the regions with ΔT > 2 C and with ΔT > 5 C accounted for 99.9 and 15.7% of the total water surface area, respectively. However, for the water surface layer (), the percentages of the water surface area with ΔT > 2 C and > 5 C were 30.1 and 0.2%, respectively. The corresponding values for the bottom water layer () were 76.9 and 30.4%, respectively. In other words, the water in deeper layers displayed greater warming under low water levels than did the water higher in the water column. The vertical temperature profile () in the deep region (e.g., SI) also revealed the same trend. Thus, the surface water temperature is more strongly influenced by the atmospheric boundary conditions (e.g., irradiance, wind), whereas the bottom water temperatures are more influenced by the water depth.
Impact of water level drawdown on water age
The daily variation of depth-averaged water age at sites A and B over the 2 simulations showed that the differences in water age were notable during the simulation period (). The maximum change in water age (ΔWA) for site A was found at the end of the simulation period (Day 365), with a ΔWA of 84 d; while the maximum ΔWA for site B was found at Day 357 at 105 d. These results suggest that the declining water levels for Lake Mead will greatly accelerate the transport and discharge of dissolved substances from the lake.
To study the changes of the extent of horizontal and vertical water ages, the depth-averaged, surface water and bottom ΔWA between the 2 scenarios at Day 365 were investigated (), and the percentages of the water column with different ΔWA at Day 365 between the 2 scenarios at various water depths were compared (). The results showed that for the surface layer, the variation in ΔWA throughout the lake was relatively uniform, with ΔWA between 80 and 90 d for 55% of the lake. However, in the bottom layer, ΔWA was more evenly distributed between 70 and 150 d. The depth-averaged ΔWA showed an intermediate level of variability. These results also suggest that water level drawdown will have a stronger effect on the bottom water and deep lake regions than on the surface water and shallow regions in terms of water exchange ().
Figure 12 The spatial distribution of calculated water age differences (ΔWA) at Day 365 between high- and low-stage situations. ΔWA represents water age of high-stage subtracted from that of low-stage (a) Depth averaged ΔWA, (b) Surface ΔWA and (c) Bottom ΔWA.
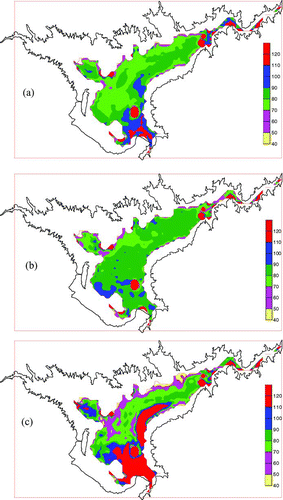
Table 2 Percentage of the lake area with different water age differences (ΔWA) at Day 365 between high- and low-stage simulations at different water depths.
Discussion
Impact of water level drawdown
The simulation results suggest that the thermal stratification and water age of Lake Mead will be significantly influenced by water level drawdown. Between the 2 simulated conditions, depth-averaged temperature increased by 4–7 C in shallow regions and 2–4 C in deep regions during the summer thermal stratification period. The ranges of temperature variation at the bottom layer are larger than those at the surface layer; however, the water temperatures at the surface layer didn't change substantially between the 2 conditions. The temperature profiles () suggested that most of the differences were caused by the reduction in metalimnion and hypolimnion layers, especially the latter.
The lake's thermal stratification refers to a change in the temperature at different depths in the lake, associated with solar radiations and other atmospheric conditions. Because the atmospheric boundary conditions of the low-stage simulation were assumed to be the same as those for the high-stage simulation, the water temperatures at the surface layer did not change substantially between the 2 conditions. However, under the low-stage condition, the bottom water temperatures appeared to be well mixed and unstratified in the shallower regions and the water temperatures remained relatively uniform. Further, the thickness of the hypolimnion and the metalimnion decreased for the stratified deep regions; therefore, the water in the deeper layers displayed greater warming under low water levels than did the water at higher layers in the water column. Climate change will likely further impact the thermal structure of the lake and the compounded effects of warming temperatures and lower water levels could drastically modify the lakes thermal patterns, which also should be taken into account for the lake's management.
Such temperature changes caused by water level drawdown would likely have a notable impact on the lake's aquatic habitat and food web (CitationPauly 1980). Particularly, the composition and distribution of fish species in the lake would be changed under the new temperature environment. Usually, each fish species exhibits a characteristic preferred temperature based on its thermal guild. For cold-water fish, when the water temperatures exceed their preferred temperature by 2–5 C, the fish will actively select and rapidly change their living area (CitationGunn 2002). The simulation results showed surface water temperatures changing from 28–30 C to 30–32 C and the bottom temperatures changing from 12–15 C to 15–20 C when water level changed from high to low-stage. The changes of water temperature will force fish to move away from their existing habitat and seek out refuge areas elsewhere. Due to the extreme water level drawdown, the reduction of water volume and increase in temperature will have notable negative impacts on the fish as well as on the overall ecosystem of the lake (CitationSloman et al. 2002).
In view of the complex problem of investigating the internal hydrodynamic processes of Lake Mead under declining water levels, the concept of water age was investigated. Because the primary inflow river for Lake Mead is the Colorado River, accounting for 96% of the total inflow, water age at any location in Lake Mead mainly represents the time elapsed for water parcels (or conservative dissolved substance) to be transported to a given location from the Colorado River via the Narrows. The water age varies with time and space depending on the variations of the dynamic conditions (e.g., wind-induced circulation and mixing, inflow discharge) during the modeling period. Our results indicated that the maximum depth-averaged water ages in the shallow regions were 30 d shorter than those in the deep region during high-stage simulation. Similarly, the vertical distributions of water ages were quite uniform in the shallow region and significantly variable in the deep region. Water age was found to be highly related to the lake circulation and thermal structure. Simulation results of lake circulation in the high-stage condition revealed that the water in the shallow regions moved faster than in the deep regions, and the water at surface moved even faster than in the lower layers. The results of thermal structure showed that the lake was well stratified in summer with higher water temperature at surface than bottom. The age distributions were in close agreement with the descriptions of lake circulation and thermal stratification; therefore, water age can be used as a reasonable indicator of the lake's hydrodynamic processes. Additionally, we found that water ages were highly dependent on the wind magnitude and direction, suggesting that wind plays an important role on the age distribution and lake hydrodynamic processes.
The decrease in depth-averaged water age by 80–100 d in the low-stage simulation compared to the high-stage condition () means the water particles were transported faster from the Colorado River through the Narrows to any location in the study area, and the retention time of Lake Mead was much smaller. The intensified water exchange caused by water level drawdown could substantially modify the lake's thermal stratification period and turnover processes as suggested by the results of our temperature simulation. The relatively younger water at the lake surface compared to the older water at the lake bottom suggests that distinct vertical patterns exist in the lake's circulation processes. Additionally, it has been shown that in many years Boulder Basin does not completely destratify (CitationLaBounty and Burns 2007). This vertical pattern could impact the lake's hydrodynamic process as well and may impact the distribution of the simulated water age. Comparing the differences in water age at the surface and bottom of the lake between the 2 simulations revealed that the water age changed faster for the bottom water than it did for the surface water, suggesting that water level drawdown could accelerate the bottom water's movement. Water movement in Lake Mead is primarily induced by the comprehensive actions of wind, inflow–outflow mechanisms and density differences caused primarily by temperature. The drawdown of water level in the low-stage scenario decreased the lake surface area markedly, resulting in the drop of wind stress on the lake surface due to the reduction of wind fetch; therefore, the mixing process in the lake induced by winds would be weakened. However, the impact of the inflow/outflow on water movement became stronger due to the significant decrease of water volume in the lake. During this process, the bottom water will likely be exchanged faster under the low-stage situation when compared to the high-stage condition. This could subsequently affect the transfer and transport of pollutants. Thus, water age provides a useful tool to describe the complex hydrodynamic processes and substance transport properties.
Pressure gradient error
The abilities of sigma coordinate models to resolve the bottom and surface layers is an attractive feature of this class of models. Unfortunately, this model of Lake Mead with sigma coordinate transformation has suffered from numerical errors while calculating horizontal pressure gradients (PG) over steep topography. The source of the problem is that in sigma coordinates, the x components of the internal pressure are written as
Much attention has been paid to the errors in computing horizontal pressure gradients in the region of steep topography, and many alternative PG schemes have been proposed to reduce the errors, including vertical interpolation schemes, higher-order methods and methods retaining integral properties and subtracting reference state (CitationMellor et al. 1998, CitationBerntsen and Furnes 2005). Further, methods associated with the specific characteristics of a study area have been suggested to find suitable model parameters to reduce errors to an acceptable level including grid characteristics, horizontal and vertical resolution, and horizontal viscosity and smoothing topography (CitationBerntsen and Furnes 2005). CitationBerntsen and Thiem (2007) described that the erroneous flows may be reduced considerably by using higher values of horizontal viscosity in areas where there is a combination of stratification and varying topography. The formulation of the Smagorinsky method (CitationSmagorinsky 1963) for calculating horizontal viscosity is shown as
For this study, 2 methods were investigated to reduce the PG errors to an acceptable level, including increasing the vertical resolution and applying large horizontal viscosity by using a large CM value. For the first method, the PG error on 3 vertical resolutions, 14, 20 and 30 verticallevels, were investigated. The AME of bottom temperatures with 14, 20 and 30 vertical layers were 2.47, 2.05 and 1.37 C at site B (), respectively. As expected, the higher the vertical resolution, the lower the PG errors. However, the higher vertical resolution requires a longer CPU calculation time. For example, during the simulation period from 1 March to 31 October 2005, the case with 30 vertical layers required approximately 120 CPU hours (Dell, Intel Core 4-CPU processor, 2.6 GHz), whereas the case with 14 layers required only 40 CPU hours.
Figure 13 Time series of bottom temperature at site B using 3 different vertical resolutions: 14, 20 and 30 levels from 1 March to 31 October 2005.
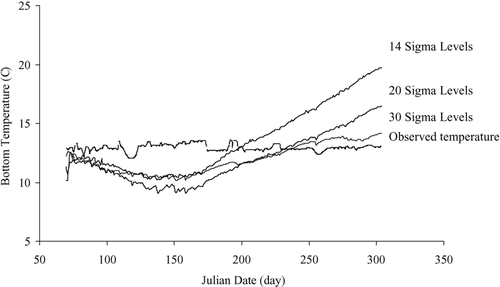
For the second method, different multiples of the recommended CM value (0.2) were applied to investigate the PG error. To test the impact of the CM value, a comparison between the time series of bottom temperatures at site B () with CM ranging from 0.2 to 1.0 was conducted (). The mean values and standard deviations of errors of calculated AM values at Site B for 2 cases during the simulation time were 1.28 ± 0.24 (CM = 0.2) and 3.35 ± 2.59 (CM = 1.0) m2/s, respectively. The AME of the bottom temperatures were 2.46 C (CM = 0.2) and 2.30 C (CM = 1.0) at site B (). The results indicated that the model is not highly sensitive to moderate changes to CM . However, the model was unstable under larger adjustments to CM . Thus, the present study did not verify CitationBerntsen and Thiem's (2007) finding that it is possible to reduce the PG errors by varying CM by a factor of 100 from the typically recommended value of 0.2 due to model instability.
Conclusions
A 3-D numerical model, EFDC, was calibrated for Lake Mead based on observed data from 2005 and was applied to study the impacts of water level drawdown on thermal structure and hydrodynamic processes of the lake. The model results indicated that water level decline would have a stronger impact on temporal stratification in the shallow regions of the lake than in the deeper regions. Further, depth-averaged temperatures increased by 4–7 C for shallow regions and 2–4 C for deeper regions during the summer thermal stratification period. However, water level drawdown may have a stronger effect on the bottom water and deep lake regions than on the surface water and shallow regions in terms of water exchange. Depth-averaged water age decreased by approximately 70–90 d for shallow regions and 90–120 d for deep regions. Such changes in the thermal regime and water ages of the lake would likely have a significant impact on the fishery and ecosystem of Lake Mead. Additionally, application of the widely used EFDC model required careful attention to account for the pressure gradient error in the presence of steep bottom slopes in Lake Mead. An increase in the vertical resolution of the model reduced the error to an acceptable level for the Lake Mead model. In general, this work provides useful information for understanding the thermal and hydrological processes in Lake Mead under extreme water level drawdown.
Acknowledgments
This paper was written while the first author (Yiping Li) was a visiting postdoctoral fellow at the Desert Research Institute (DRI), Nevada, USA. The research was supported by Chinese National Science Foundation (51009049); Fundamental Research Funds for the Central Universities; Grant #20070294019 from the Ph.D. Programs Foundation of Ministry of Education, China; The research was also supported by Grant # 2010CB951101, IRT0717, 50979022, 1069-50986312 2008ZX07101-002 and 2008ZX07101-006. We would like to thank Ronald Veley with the US Geological Survey (USGS) for providing monitoring data, and thank Drs. Sen Bai, Scott C. James and Craig Jones for their help in developing the Lake Mead model.
References
- Barnett , T P and Pierce , D W . 2008 . When will Lake Mead go dry? . Water Resour Res. , 44
- Berntsen , J and Furnes , G . 2005 . Internal pressure errors in sigma-coordinate ocean models-sensitivity of the growth of the flow to the time stepping method and possible non-hydrostatic effects. . Cont Shelf Res , 25 : 829 – 848 .
- Berntsen , J and Thiem , O . 2007 . Estimating the internal pressure gradient errors in a sigma-coordinate ocean model for the Nordic Seas. . Ocean Dynam , 57 : 417 – 429 .
- Brauns , M , Garcia , X F and Pusch , M T . 2008 . Potential effects of water-level fluctuations on littoral invertebrates in lowland lakes. . Hydrobiologia , 613 : 5 – 12 .
- Brennwald , M S , Peeters , F , Imboden , D M , Giralt , S , Hofer , M , Livingstone , D M , Klump , S , Strassmann , K and Kipfer , R . 2004 . Atmospheric noble gases in lake sediment pore water as proxies for environmental change. . Geophys Res Lett. , 31 : L04202
- Bourne , J K , Joel , K and Ludwig , G . 2005 . Eccentric Salton Sea. . Natl Geogr , 07 : 88 – 107 .
- Coe , M T and Foley , J A . 2001 . Human and natural impacts on the water resources of the Lake Chad basin. . J Geophys Res-Atmos , 106 : 3349 – 3356 .
- Cole , T and Wells , S A . 2005 . CE-QUAL-W2: A two-dimensional laterally averaged, hydrodynamic and water quality model, version 3.2. , Vicksburg , MS : US Army Corps of Engineers, Waterway Experiment Station . Instructional Report EL-03-01
- Delhez , E JM , Campin , J M , Hirst , A C and Deleersnijder , E . 1999 . Toward a general theory of the age in ocean modeling. . Ocean Model , 1 : 17 – 27 .
- Dynesius , M and Nilsson , C . 1994 . Fragmentation and flow regulation of river systems in the northern third of the World. . Science , 266 : 753 – 762 .
- Elci , S , Bor , A and Caliskan , A . 2009 . Using numerical models and acoustic methods to predict reservoir sedimentation. . Lake Reserv Manage , 25 : 297 – 306 .
- Galperin , B , Kantha , L H , Hassid , S and Rosati , A . 1988 . A quasi-equilibrium turbulent energy-model for geophysical flows. . J Atmos Sci , 45 : 55 – 62 .
- Gunn , J M . 2002 . Impact of the 1998 El Nino event on a lake charr, Salvelinus namaycush, population recovering from acidification. . Environ Biol Fish , 64 : 343 – 351 .
- Hamrick , J M . 1992 . A three-dimensional Environmental Fluid Dynamics Computer Code: Theoretical and computational aspects Special Report in Applied Marine Science and Ocean Engineering, No. 317 College of William and Mary, VIMS, p. 63
- Hamrick , J M . 1994 . Application of the EFDC, environmental fluid dynamics computer code to SFWMD Water Conservation Area 2A , Williamsburg , VA : J.M. Hamrick and Associates . Report JMH-SFWMD-94-01. 126 pp
- Hoerling , M and Eischeid , J . 2000 . Past peak water in the West. . Southwest Hydrol. , 6 ( 1 ) : 18 – 19 .
- Hofmann , H , Lorke , A and Peeters , F . 2008 . Temporal scales of water-level fluctuations in lakes and their ecological implications. . Hydrobiologia , 613 : 85 – 96 .
- HydroQual Inc. 2001 . A Primer for ECOMSED, Version 1.2: User Manual , 179 Mahwah, (NJ) : HydroQual, Inc. . 1 Lethbridge Plaza.
- [IPCC] Intergovernmental Panel on Climate Change . 2008 . “ Summary for policy makers in climate change 2007. ” . In Working group II: Impacts, adaptation, and vulnerability , Edited by: Parry , M , Canziani , O , Palutikof , J , van , d er , Linden , P and Hansson , C . New York : Cambridge Univ .
- Ji , Z G , Hu , G D , Shen , J A and Wan , Y S . 2007 . Three-dimensional modeling of hydrodynamic processes in the St. Lucie Estuary . Estuar Coast Shelf Sci , 73 : 188 – 200 .
- Junk , W J and Wantzen , K M . 2004 . “ The flood pulse concept: New aspects, approaches, and applications—an update. ” . In Proceedings of the 2nd Large River Symposium (LARS), Pnom Penh, Cambodia , Edited by: Welcomme , R and Petr , T . 117 – 149 . Bangkok : RAP Publication .
- LaBounty , J F and Burns , N M . 2007 . Long-term increases in oxygen depletion in the bottom waters of Boulder Basin, Lake Mead, Nevada-Arizona, USA. . Lake Reserv Manage , 23 : 69 – 82 .
- Liang , Q H and Borthwick , A GL . 2008 . Adaptive quadtree simulation of shallow flows with wet-dry fronts over complex topography. . Comput Fluids , 38 : 221 – 234 .
- [LMWD] Lake Mead Water Database . 2009 . http://lakemead.water-data.com/index2.php. Accessed 20 Dec 2009
- Mellor , G L . 1990 . User's guide for a three-dimensional, primitive equation, numerical ocean model. , 44 Pinceton , NJ : Princeton University . Atmospheric and Ocean Sciences Program
- Mellor , G L , Oey , L Y and Ezer , T . 1998 . Sigma coordinate pressure gradient errors and the Seamount problem. . J Atmos Ocean Tech , 15 : 1122 – 1131 .
- Mellor , G L and Yamada , T . 1982 . Development of a turbulence closure-model for geophysical fluid problems. . Rev Geophys , 20 : 851 – 875 .
- Nash , L and Gleick , P . 1991 . The sensitivity of stream flow in the Colorado Basin to climatic changes. . J Hydrol , 125 : 221 – 241 .
- [NASA] National Aeronautics and Space Administration . 2003 . EO study: Drought lowers Lake Mead http://earthobservatory.nasa.gov/Study/LakeMead/lake_mead.html. Accessed Feb 2008
- Pauly , D . 1980 . On the interrelationships between natural mortality, growth-parameters, and mean environmental-temperature in 175 fish stocks. . J Cons Int Explor Mer , 39 : 175 – 192 .
- Shen , J and Wang , H V . 2007 . Determining the age of water and long-term transport timescale of the Chesapeake Bay. . Estuar Coast Shelf Sci , 74 : 585 – 598 .
- Sloman , K A , Wilson , L , Freel , J A , Taylor , A C , Metcalfe , N B and Gilmour , K M . 2002 . The effects of increased flow rates on linear dominance hierarchies and physiological function in brown trout, Salmo trutta. . Can J Zool , 80 : 1221 – 1227 .
- Smagorinsky , J . 1963 . General circulation experiments with the primative equations, I. The basic experiment. . Mon Weather Rev , 91 : 99 – 164 .
- Song , Y T . 1998 . A general pressure gradient formulation for ocean models. Part I: Scheme design and diagnostic analysis. . Mon Weather Rev , 126 : 3213 – 3230 .
- Steinberg , S M , Nemr , E L and Rudin , M . 2009 . Characterization of the lignin signature in Lake Mead, NV, sediment: comparison of on-line flash chemopyrolysis (600A degrees C) and off-line chemolysis (250A degrees C). . Environ Geochem Hlth , 31 : 339 – 352 .
- [SCOPEIS] Systems Conveyance and Operations Program Environmental Impact Statement . 2006 . National Park Service, Bureau of Reclamation Available at: http://www.cleanwatercoalition.com/Home/FederalApprovalProcess/EnvironmentalImpactStatement/tabid/82/Default.aspx. Accessed 21 Oct 2006
- Takeoka , H . 1984 . Fundamental concepts of exchange and transport time scales in a coastal sea. . Cont Shelf Res. , 3 ( 3 ) : 322 – 326 .
- Twichell , D C , Cross , V A and Belew , S D . 2003 . Mapping the floor of Lake Mead (Nevada and Arizona): Preliminary discussion and GIS data release USGS Open-File Report 03-32
- Usmanova , R M . 2003 . Aral Sea and sustainable development. . Water Sci Technol , 47 : 41 – 47 .
- Vermeyen , T B . 2003 . Acoustic Doppler Velocity measurements collected in Las Vegas Bay and Boulder Basin, Lake Mead, Nevada. Technical report , Denver , CO : US Department of the Interior, Bureau of Reclamation . Technical Service Center
- Wantzen , K M , Rothhaupt , K O , Mortl , M , Cantonati , M , Laszlo , G T and Fischer , P . 2008 . Ecological effects of water-level fluctuations in lakes: an urgent issue. . Hydrobiologia , 613 : 1 – 4 .
- Zimmerman , J TF . 1976 . Mixing and flushing of tidal embayments in the Western Dutch Wadden Sea, Part I: distribution of salinity and calculation of mixing time scales . Neth J Sea Res , 10 : 149 – 191 .