Abstract
Background
An increasing number of studies show that the intestinal flora is closely related to spinal cord injury. Many researchers are exploring the changes in the richness, diversity, and evenness of intestinal flora in spinal cord injury animal models to identify the characteristic bacteria.
Methods
A comprehensive literature search was conducted using three databases: PubMed, Embase, and Web of Science. A meta-analysis was performed using R 4.3.1 to evaluate the comparison of microbiota diversity, richness, and evenness and the relative abundance of intestinal microbiota in animals with spinal cord injury and blank controls.
Results
Fifteen studies were included in the meta-analysis, of which 12 involved gut microbiota distribution indicators and 11 included intestinal microflora relative abundance indicators. Meta-analysis of high-dimensional indicators describing the distribution of the gut microbiota identified a substantial decline in the evenness and richness of the intestinal flora. In addition, the Actinobacteria phylum and Erysipelotrichales and Clostridiales orders were significantly different between the spinal cord injury and sham groups; therefore, they may be the characteristic bacteria in spinal cord injury models.
Conclusion
Our meta-analysis suggested that the gut microbiota in the spinal cord injury animal model group was altered compared with that in the control group, with varying degrees of changes in richness and evenness and potentially pathogenic characteristic flora. More rigorous methodological studies are needed because of the high heterogeneity and limited sample size. Further research is needed to clinically apply intestinal microbiota and potentially guide fecal microbiota transplantation therapy.
Key Messages
Our meta-analysis showed that spinal cord injury significantly decreased the richness and evenness of intestinal flora in experimental animals.
No statistically significant changes in the phyla flora during spinal cord injury have been found.
Erysipelotrichales and Clostridiales may be the characteristic flora of gut microbiota changes during spinal cord injury.
1. Introduction
Spinal cord injury (SCI) is a common and severe neurological disease usually caused by acute external mechanical injury [Citation1], including traffic accidents and serious falls [Citation2]. Epidemiological studies show that SCI prevalence is the highest in the United States of America at 250 per million cases every year [Citation3]. SCI can seriously damage the physical and mental health of patients, affect social development, and impose a huge economic burden on the healthcare system; therefore, this disease cannot be ignored [Citation4].
SCIs can be devastating for several reasons based on the internal metabolic, biological, and pathophysiological changes that occur [Citation5]. Disorder of the spinal cord environment after SCI is an important reason for the death of neurons in the injured area and difficult recovery of motor function [Citation6]. Secondary pathophysiological changes after SCI (such as inflammation, edema, metabolic disorders, and other complications) further aggravate the injury [Citation7]. Researchers are beginning to see the significant impact of characteristic microbiota on certain diseases. This provides new ideas for treatment. Fecal microbiota transplantation (FMT) stands out and has already shown some success in gastrointestinal diseases and neuropsychiatric disorders [Citation8,Citation9]. Therefore, FMT can be regarded as a potential treatment for complex systemic reactions after SCI to help the body cope with the adverse effects of injury such as changes in inflammatory factors and hyperalgesia. Many researchers are exploring the efficacy of FMT for SCI [Citation10]. Changes in the diversity and richness of gut microbiota and relative abundance after SCI affect the original function of intestinal flora in regulating inflammatory response and metabolism and affect SCI progression and its complications via changes in the neuroendocrine system and intestinal brain axis [Citation6]. The gut microbiome regulates various cellular and molecular mechanisms that are critical for the progression of pathophysiological processes induced by acute central nervous system damage [Citation11,Citation12]. Changes in the gut flora can disrupt the balance of the bidirectional enterobrain axis. This exacerbates secondary brain injury, and impairs cognitive function and motor function that leads to a poor prognosis by triggering pro-inflammatory responses in the peripheral circulation and central nervous system [Citation11]. For example, diseases disrupt the balance of the gut-brain axis by affecting the absorption of food-related metabolites. Short-chain fatty acids (SCFAs) act on various targets by activating free fatty acid 2 receptors and are involved in everything from neuroplasticity to gene expression, food intake, and immune system regulation. Patients with irritable bowel syndrome lack SCFA-producing flora. The application of SCFA-producing gut microbiota in germ-free mice inhibits this activation; this suggests that a healthy gut microbiome can inhibit this neuronal signaling pathway [Citation13,Citation14]. The intestine is innervated by the parasympathetic vagus and sympathetic spinal nerves in SCIs that originate in the brainstem and spinal cord. Damaged nociceptors dominate the intestine and release inflammatory mediators following SCI. This can lead to a neurogenic bowel with patients experiencing constipation, fecal incontinence, and bloating [Citation9,Citation15,Citation16].
Intestinal flora animal models are being used to determine the cause of aggravated SCI [Citation17]. In addition, some researchers conclude that FMT plays a neuroprotective role by regulating the microenvironment of the lesion site in a mouse model of SCI [Citation18].
To date, several systematic reviews show associations between SCI and the gut microbiome. However, meta-analyses were not performed. This systematic review and meta-analysis investigated the association between SCI and intestinal flora in animal models and explored the characteristic changes.
2. Methods
2.1. Search strategy
This systematic review and meta-analysis followed the Preferred Reporting Items for Systematic Reviews and Meta-Analyses (PRISMA) guidelines [Citation19].
A comprehensive search was conducted in PubMed, Embase, and Web of Science using keywords such as ‘spinal cord injury’ and ‘gut microbiota’ (the detailed search format is provided in Appendix 1). The search time ranged from the establishment of the database to January 1, 2023 to retrieve all valuable animal models of SCI involving intestinal flora analysis; the search language and publication period were not limited ().
2.2. Eligibility criteria
The inclusion criteria were as follows: (1) the type of research design was controlled, (2) the research object was animals, (3) the outcome indicators were intestinal flora abundance or indicators of the richness or diversity of intestinal flora, and (4) the original documents were published.
The exclusion criteria were as follows: (1) non-animal research object; (2) research not focused on the SCI model; (3) incomplete data, unable to extract the original data statistics literature; (4) conference papers, dissertations, and review articles; and (5) non-English papers.
2.3. Data extraction
All data were independently extracted from the original text or charts by two authors. (1) General characteristics: the first author, publication year, species of animals, age, weight, injured spinal section, and sampling time after SCI. (2) Outcome indicators study: alpha diversity (observed species index, Chao1 index, Shannon index, Simpson index, Pielou’s evenness, ACE index), bacterial phyla, bacterial orders, and bacterial genera.
Data was either directly extracted from the original text or emails were sent to the corresponding author of the study to request the original data. The GetData Graph Digitizer was used to obtain the relevant outcome indicator data if there was no response from the corresponding author or accurate original data could not be inability obtained [Citation20].
2.4. Quality assessment
The risk of bias in the included studies was judged according to Cochrane Reviewer’s Handbook 5.1.0 [Citation21] and was assessed by two independent authors using six criteria: (1) random sequence generation, (2) allocation concealment, (3) blinding of participants and personnel, (4) incomplete outcome data, (5) selective reporting, and (6) other biases. Each criterion was assessed as having low, unclear, or high bias. Two researchers independently scored the risk of bias and conducted cross-verification. Cases of disagreement involved asking a third party for advice after discussion. A random number table or generating random numbers by computer are regarded as low risk reliable methods for random sequence generation. Unreliable methods such as artificially selecting numbers are regarded as high risk, and unknown generation methods are regarded as unclear. The risk for allocation concealment and blinding of participants and personnel depends on whether the blinding method is strictly and properly used. A blinding method that was likely to be damaged during the study was considered high risk; otherwise, it was considered low risk. Failure to report all pre-specified primary outcome measures was considered high-risk, whereas outcome measures in the fully published study plan were considered low-risk. Other biases depended on whether there was a significant risk of bias or falsification.
2.5. Data analysis
All the extracted data were converted into the same unit before calculations, and the continuous variables were expressed as the mean ± standard deviation according to the formula presented in Appendix 2 (m = median, a = the smallest value (minimum), b = the largest value (maximum)) [Citation22]. Data analysis was performed using R 4.3.1. The results were expressed as mean differences with 95% confidence intervals (CIs). Chi-squared tests and I2 statistics were used to analyze heterogeneity. p < .1 and I2 > 50% indicated a high likelihood of heterogeneity in the data. A random-effects model was used owing to the anticipated heterogeneity. Moreover, a subgroup analysis was performed to better interpret the results of this study, if necessary. Statistical significance was set at p < .05.
3. Results
3.1. Search results
A total of 218 studies were retrieved up to January 1, 2023, after searching Web of Science, PubMed, and Embase. Two researchers screened the titles and abstracts of the references using EndNote to remove duplicates. A total of 114 references were excluded as they did not meet the inclusion criteria, and 104 potential references were included. Fifteen studies were included in our meta-analysis after two researchers read the full texts.
3.2. Characteristics of selected studies
The animal model of SCI was a rat or mouse in 14 studies and a pig in 1 study (). The T10 sections were selected by over half of the authors (10 studies) for the selection of the SCI sections, whereas the C5 sections were selected by Schmidt and EKA [Citation34].
Table 1. General characteristics and outcome indicators in included studies.
Five researchers chose 28 d after the establishment of the SCI model as the most common fecal sample collection time. In fact, most studies obtained fecal samples within 28 d; Scott A. Myersa took the longest sampling time after modeling at 42 d.
There were primarily four outcome indexes: alpha diversity, phylum, order, and genus. The outcome indicators are shown in .
3.3. Risk of bias assessment
All the studies were judged to have a low risk of bias, random sequences, allocation methods, and blinding. However, there is an unclear risk of bias owing to incomplete outcomes, data, and other biases ( and ).
3.4. Differences in outcome assessment between SCI models and the sham group
3.4.1. Alpha diversity
Several different types of alpha diversity indices were used to assess the microbial diversity within the same group, including estimated richness (observed species index, Chao1 index) and indices presenting richness and evenness (Shannon index, Simpson index, Pielou’s evenness, and ACE index) ().
Figure 4. Forest plots of alpha diversity in the gut microbiota of the SCI group and the control group ((1) Observed species index, (2) Shannon index, (3) Chao1 index, (4) Simpson index, (5) Pielou’s evenness, (6) ACE index).
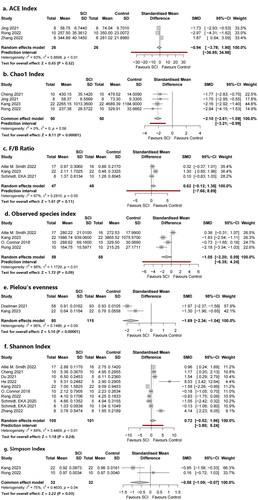
Alpha diversity is related to the number of species in a locally homogeneous habitat and is also known as intra-habitat diversity. β diversity (also known as between-habitat diversity) refers to the species composition of different habitat communities along environmental gradients or the rate of species replacement along environmental gradients. The Chao1 index was used to estimate the number of operational taxonomic units (OTUs) contained in a sample. The Chao1 algorithm is often used to estimate the total number of species in ecology, and is used to estimate the number of OTUs in a community. This was also proposed by Chao and is one of the common indices used to estimate the total number of species in ecology. Pielou’s evenness, Shannon index, and Simpson index were used to estimate the microbial diversity index in the samples and were often used to reflect the alpha diversity index. The higher the Shannon value, the higher the community diversity.
Four studies provided data on the observed species index and four studies provided data on the Chao1 index regarding richness. Subgroup analysis showed that the Chao1 indices in the SCI model group were significantly decreased compared with those in the sham group (standard mean difference (SMD) = −2.10, 95% CI −2.61 to −1.59, p < .00001, I2 = 0%;). The observed species index of the SCI model group was significantly lower than that of the control group in half of the four studies, although the meta-analysis did not show a statistically significant difference. There were no significant differences in SMDs of the observed species index (SMD = −1.05, 95% CI = −2.20 to 0.09, p = .09, I2 = 88%).
Ten studies provided data on Shannon’s index, two studies provided data on Simpson’s index, two studies provided data on Pielou’s evenness, and three studies provided data on the ACE index. Completely different results were observed in the 10 studies using Shannon’s index as the outcome indicator. Half of the studies found a significant increase in Shannon’s index in the SCI model group, whereas two studies showed significantly reduced Shannon’s indices; the remaining three studies found no statistically significant difference. No significant differences were found in our meta-analysis (SMD = 0.72, 95% CI = −0.52 to 1.96, p = .24, I2 = 88%). The number of articles included in the remaining three indicators was relatively small; Simpson’s indices in the SCI model group were significantly decreased compared with those in the control group (SMD= −0.58, 95% CI = −1.09 to −0.07, p = .03, I2 = 75%), and the same trend was found for Pielou’s evenness (SMD = −1.69, 95% CI = −2.34 to −1.04, p < .00001, I2 = 66%). Meanwhile, no significant differences were found in the ACE index (SMD = −0.94, 95% CI = −3.76 to 1.90, p = .52, I2 = 93%).
3.4.2. Bacterial phyla
Five phyla were identified (): Bacteroidetes, Actinobacteria, Verrucomicrobia, Firmicutes, and Proteobacteria. Additionally, the Firmicutes/Bacteroidetes (F/B) ratio was included as an outcome index. Meta-analysis showed that Actinobacteria were statistically significant (SMD = 0.37, 95% CI 0.10–0.643, p = .007, I2 = 42%). There was no statistically significant difference in the level of the remaining phyla or the F/B ratio.
3.4.3. Bacterial order
Four orders were identified: Erysipelotrichales, Verrucomicrobiales, Bacteroidales, and Clostridiales (). Meta-analysis showed that Erysipelotrichales and Clostridiales had statistically significant differences: SMD = −1.16, 95% CI= −1.82 to −0.50, p = .0006, I2 = 0% and SMD = 2.78, 95% CI = 1.97–3.59, p < .00001, I2 = 0%, respectively. However, there were only a small number of studies included in both analyses. No significant differences were observed in the flora of the remaining orders.
3.4.4. Bacterial genus
Six genera were identified: Akkermansia, Bacteroides, Lactobacillus, Alistipes, Alloprevotella, and Lachnospiraceae NK4A136 (). No significant differences were observed between the genera.
Figure 7. Forest plots of genera in the gut microbiota of the SCI and control groups ((1) Akkermansia, (2) Bacteroides, (3) Lactobacillus, (4) Alistipes, (5) Alloprevotella, (6) Lachnospiraceae_NK4A136_group).
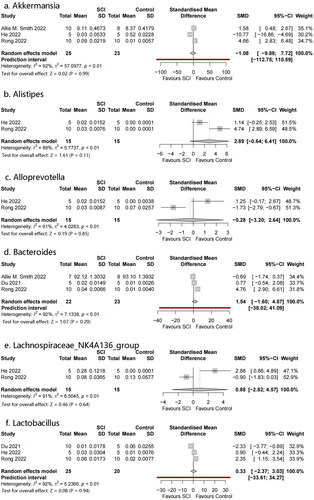
summarized the profile of the microbiota included in this study, including alpha diversity and relative abundance at the phylum, species, and genus levels. Different studies produced variable, or even completely opposite results. Consistent experimental results were observed for some indicators of alpha diversity. For example, all four included studies reported that the Chao1 index significantly decreased after SCI. Otherwise, some indicator experimental results were completely opposite to those reported by other researchers. For example, Shannon’s index (a commonly used indicator measuring bacterial richness) significantly increased in five studies after SCI, whereas two researchers concluded that it significantly decreased. This was especially true for the phylum flora. Meta-analysis showed statistically significant increases in Actinobacteria, Firmicutes, and Bacteroidetes (which have attracted much attention). Furthermore, there were often significantly different results owing to differences in experimental methods, such as different spinal cord sections or sampling times.
shows the phylogenetic characteristics of rich taxon differences in mice with SCI compared to those seen in normal mice at the phylum, order, and genus levels, and the colors indicate different bacterial variations. Red, green, orange and grey indicate increased abundance, decreased abundance, inconsistent variation and abundance not mentioned, respectively.
4. Discussion
Many studies have systematically analyzed existing studies on intestinal flora and SCI; however, no meta-analysis had been performed. To the best of our knowledge, this is the first meta-analysis investigating the relationship between changes in gut microbiota and SCI in animal models. We searched three of the most commonly used databases for determining the changes in the diversity, richness, and evenness of the gut microbiota after SC, and the possible characteristics of bacteria that preserve spinal cord function from impairment.
Fifteen high-quality studies were included in this meta-analysis. The following indicators were included to describe the overall characteristics of the gut microbiota according to previous studies on intestinal flora: observed species index: Shannon’s index, Chao1 index, Simpson’s index, ACE index, and Pielou’s evenness [Citation38,Citation39]. In our analysis, three of the six alpha diversity measures were statistically significant. Among them, the Chao1 and Simpson indices (representing richness) and Pielou’s evenness significantly decreased, although studies of the latter two were limited. Shannon’s index is the most common indicator of microbiota diversity; however, different researchers have obtained varied or opposite results. Five of the 10 studies involving Shannon’s index reported a significant increase in SCI vs. healthy subjects and two reported a significant decrease.
Most studies included relevant experimental analyses regarding beta diversity. We did not conduct a meta-analysis owing to the insufficient number of studies with available data.
The characteristic gut microbiome taxa were explored by selecting bacteria from two or more studies with sufficient data for meta-analysis. Bacteroidetes and Firmicutes are the two most abundant phyla; this was not completely consistent with previous studies. Different, or even completely opposite conclusions were reached in different studies. We observed that Bacteroidetes showed an increasing trend; this correlated with Kang’s study [Citation29]. Bacteroidetes are pro-inflammatory bacteria that can increase and aggravate symptoms after SCI. The dominant phylum Firmicutes in the intestinal tract was replaced by Bacteroidetes, the ratio of Firmicutes to Bacteroidetes decreased, and the abundance of Proteobacteria decreased. These bacterial translocations may be owing to increased permeability of intestinal epithelial cells when sympathetic nerves are inhibited and decreased gastrointestinal moisture and sensitivity of intestinal neurons to intestinal serotonin and nutrients when parasympathetic nerves are inhibited. This creates conditions for changes in the intestinal microenvironment [Citation40,Citation41]. The status of Firmicutes is complicated and research on this flora is currently unclear. Many people believe that it has a positive or side effect on SCI regulation, and we can obtain a glimpse of it from the heat map. The Firmicutes/Bacteroidetes ratio is considered an important indicator for describing intestinal disorders. However, no statistical significance was obtained in our analysis. This may be owing to the small sample size. Some studies suggest that the change in the ratio of Firmicutes to Bacteroides represented by a decrease in the abundance of Firmicutes and an increase in the abundance of Bacteroides is owing to a disorder of the intestinal microflora after SCI. Such changes in the microflora structure affect the production of SCFAs, resulting in severe inflammation and complex infections [Citation42,Citation43]. Actinobacteria has not received sufficient attention. In our analysis, Actinobacteria significantly increased in the SCI group. Its role in cellular and molecular mechanisms (such as bacterial translocation and inflammation induction) has not been clarified. The relative abundance of Clostridiales was significantly higher in SCI compared with healthy subjects, as described in many studies. No statistically significant bacterial genera were detected.
The close relationship between SCI and intestinal flora was reviewed by several researchers [Citation44–49]. In this research, the influence of intestinal ecological changes after SCI is often mentioned. However, the relationship between intestinal flora changes and SCI is confusing [Citation49]. Following SCI, inflammatory mediators are produced via numerous pathways [Citation50]. Changes in gut microbiota can stimulate the production of cytokines such as IL-8, IL-10, and TNF in enteric-related lymphoid tissues that can reach the SCI site via the circulatory system and activate resting microglial cells in the central nervous system [Citation51–53]. One type of microglia called M1 produces a various pro-inflammatory factors (such as TNF-α, IL-1β, IL-6, NO, and reactive oxygen species) that can induce the apoptosis of neurons and oligodendrocytes, cause secondary injury, aggravate the pathophysiological reaction of SCI, and affect the functional recovery of the injured spinal cord [Citation54].
The gut microbiota-brain axis is an important topic in the study of intestinal flora and SCI. Experimental models of the central nervous system showed that the two-way communication between the central nervous system and the intestine was accompanied by ecological disorders after injury, neuroinflammatory responses mediated by gastrointestinal-associated lymphoid tissue, and neurotransmission of bacterial metabolites [Citation55]. Studies suggest that the gut microbiota – brain axis is associated with psychosis. Emotional and psychological changes after SCI may be related to changes in the volume of specific brain regions involved in information processing and emotions that can produce anxiety- and depression-like symptoms, with an incidence between 11% and 30% [Citation56].
At present, the study of intestinal flora has focused on pathogenesis. There are several proposed treatments for SCI, including FMT, melatonin therapy, and probiotic therapy [Citation57,Citation58]. Many treatments are being tested in animals and patients [Citation34,Citation57,Citation59]. Both the direct transplantation of healthy feces and the implantation of specific probiotics regulate impaired immune function and improve intestinal barrier function by producing antibacterial products, such as organic acids [Citation60]. An increasing number of studies were conducted on intestinal flora in the past 10 years, and breakthroughs and progress were made. However, the complex and specific composition of intestinal flora and the intestinal immune environment remind us that we should not ignore the differences between laboratory animals and humans, and between people of different races, genders, and ages [Citation61]. Exploring valuable intestinal changes in a complex population with significant differences is a future focus for developing and improving the treatment of SCI.
This meta-analysis has some limitations. First, the animal models included in this study were not identical, including animal type, sex, weight, and age; therefore, it was impossible to accurately estimate their impact on outcome indicators. Second, the sampling time was not completely consistent (although most were within 28 d or less), The longest sampling time was 42 d, while the shortest was 7 d However, the times were still within the traditional perception of acute SCI. We initially intended to perform subgroup analyses of features from different studies (such as the type of subjects studied and the timing of fecal sampling) or the SCI section to better illustrate the results. However, it was difficult to obtain meaningful subgroup analysis results from the chaotic data from different studies; therefore, we did not conduct a subgroup analysis. The inability to accurately analyze the effects of acute and chronic SCI on gut microbiota changes is an unresolved problem [Citation49]. Undeniably, there is no clear evidence to determine whether a phase effect causes significant changes in the intestinal flora within this period. Third, the small number of studies, the insufficient sample size of each study, and the high heterogeneity of some included studies resulted in limited statistical power.
5. Conclusion
Our meta-analysis suggested that the gut microbiota in the animal model group with SCI showed varying degrees of changes in richness and evenness compared to that in the control group, and potentially harmful characteristic flora were identified. More rigorous methodological studies are needed owing to the high heterogeneity and limited sample size. Further research is needed to clinically apply the intestinal microbiota and possibly guide FMT therapy.
Authors contributions
ZY, XL designed the study. ZY, NC performed the literature search and assessed study eligibility.
ZY, NC, YF, JF were involved in data extraction. All authors participated in summarizing the results.
ZY wrote and LX revised this manuscript. All the authors read and approved the final manuscript.
Acknowledgements
We really appreciate all the members in Prof. Li’s team for their valuable comments to the current study.
Disclosure statement
No potential conflict of interest was reported by the author(s).
Data availability statement
Data supporting the results of this study can be obtained through the corresponding author.
Additional information
Funding
References
- Chen J, Wang Z, Mao Y, et al. Liraglutide activates autophagy via GLP-1R to improve functional recovery after spinal cord injury. Oncotarget. 2017;8(49):1–16. doi: 10.18632/oncotarget.20791.
- Ma K, Xu H, Zhang J, et al. Insulin-like growth factor-1 enhances neuroprotective effects of neural stem cell exosomes after spinal cord injury via an miR-219a-2-3p/YY1 mechanism. Aging. 2019;11(24):12278–12294. doi: 10.18632/aging.102568.
- Singh A, Tetreault L, Kalsi-Ryan S, et al. Global prevalence and incidence of traumatic spinal cord injury. Clin Epidemiol. 2014;6:309–331. doi: 10.2147/CLEP.S68889.
- Zhang T, Li K, Zhang ZL, et al. LncRNA Airsci increases the inflammatory response after spinal cord injury in rats through the nuclear factor kappa B signaling pathway. Neural Regen Res. 2021;16(4):772–777. doi: 10.4103/1673-5374.295335.
- Li HX, Cui J, Fan JS, et al. An observation of the clinical efficacy of combining Riluzole with mannitol and hyperbaric oxygen in treating acute spinal cord injury. Pak J Med Sci. 2021;37(2):320–324. doi: 10.12669/pjms.37.2.3418.
- Huang Z, Gong J, Lin W, et al. Catalpol as a component of rehmannia glutinosa protects spinal cord injury by inhibiting endoplasmic reticulum stress-mediated neuronal apoptosis. Front Pharmacol. 2022;13:860757. doi: 10.3389/fphar.2022.860757.
- Zou HJ, Guo SW, Zhu L, et al. Methylprednisolone induces neuro-protective effects via the inhibition of A1 astrocyte activation in traumatic spinal cord injury mouse models. Front Neurosci. 2021;15:628917. doi: 10.3389/fnins.2021.628917.
- Vaughn BP, Rank KM, Khoruts A. Fecal microbiota transplantation: current status in treatment of GI and liver disease. Clin Gastroenterol Hepatol. 2019;17(2):353–361. doi: 10.1016/j.cgh.2018.07.026.
- Evrensel A, Ceylan ME. Fecal microbiota transplantation and its usage in neuropsychiatric disorders. Clin Psychopharmacol Neurosci. 2016;14(3):231–237. doi: 10.9758/cpn.2016.14.3.231.
- Tkach S, Dorofeyev A, Kuzenko I, et al. Current status and future therapeutic options for fecal microbiota transplantation. Medicina. 2022;58(1):84. doi: 10.3390/medicina58010084.
- Yuan B, Lu XJ, Wu Q. Gut microbiota and acute Central nervous system injury: a new target for therapeutic intervention. Front Immunol. 2021;12:800796. doi: 10.3389/fimmu.2021.800796.
- Zheng Z, Wang S, Wu C, et al. Gut microbiota dysbiosis after traumatic brain injury contributes to persistent microglial activation associated with upregulated Lyz2 and shifted tryptophan metabolic phenotype. Nutrients. 2022;14(17):3467. doi: 10.3390/nu14173467.
- Mayer EA, Nance K, Chen S. The Gut-Brain axis. Annu Rev Med. 2022;73(1):439–453. doi: 10.1146/annurev-med-042320-014032.
- Dalile B, Van Oudenhove L, Vervliet B, et al. The role of short-chain fatty acids in microbiota-gut-brain communication. Nat Rev Gastroenterol Hepatol. 2019;16(8):461–478. doi: 10.1038/s41575-019-0157-3.
- Bannerman CA, Douchant K, Sheth PM, et al. The gut-brain axis and beyond: microbiome control of spinal cord injury pain in humans and rodents. Neurobiol Pain. 2021;9:100059. doi: 10.1016/j.ynpai.2020.100059.
- Qi Z, Middleton JW, Malcolm A. Bowel dysfunction in spinal cord injury. Curr Gastroenterol Rep. 2018;20(10):47. doi: 10.1007/s11894-018-0655-4.
- Liu X, Liu N, Zhou M, et al. Bibliometric analysis of global research on the rehabilitation of spinal cord injury in the past two decades. Ther Clin Risk Manag. 2019;15:1–14. doi: 10.2147/TCRM.S163881.
- Jing Y, Bai F, Wang L, et al. Fecal microbiota transplantation exerts neuroprotective effects in a mouse spinal cord injury model by modulating the microenvironment at the lesion site. Microbiol Spectr. 2022;10(3):e0017722. doi: 10.1128/spectrum.00177-22.
- Page MJ, McKenzie JE, Bossuyt PM, et al. The PRISMA 2020 statement: an updated guideline for reporting systematic reviews. Int J Surg. 2021;88:105906. doi: 10.1016/j.ijsu.2021.105906.
- Haining L, Hao W, Can Y, et al. Advanced methods of data extraction for continuous outcomes in meta-analysis. Chin J Evid Based Med. 2017;17:117–121. doi: 10.7507/1672-2531.201612004.
- Higgins JP, Altman DG, Gøtzsche PC, et al. The cochrane collaboration’s tool for assessing risk of bias in randomised trials. BMJ. 2011;343(2):d5928–d5928. doi: 10.1136/bmj.d5928.
- Hozo SP, Djulbegovic B, Hozo I. Estimating the mean and variance from the median, range, and the size of a sample. BMC Med Res Methodol. 2005;5(1):13. doi: 10.1186/1471-2288-5-13.
- Smith AM, Welch BA, Harris KK, et al. Nutrient composition influences the gut microbiota in chronic thoracic spinal cord-injured rats. Physiol Genomics. 2022;54(10):402–415. doi: 10.1152/physiolgenomics.00037.2022.
- Cheng J, Li W, Wang Y, et al. Electroacupuncture modulates the intestinal microecology to improve intestinal motility in spinal cord injury rats. Microb Biotechnol. 2022;15(3):862–873. doi: 10.1111/1751-7915.13968.
- Doelman A, Tigchelaar S, McConeghy B, et al. Characterization of the gut microbiome in a porcine model of thoracic spinal cord injury. BMC Genomics. 2021;22(1):775. doi: 10.1186/s12864-021-07979-3.
- Du J, Zayed AA, Kigerl KA, et al. Spinal cord injury changes the structure and functional potential of gut bacterial and viral communities. mSystems. 2021;6(3):e01356-20. doi: 10.1128/mSystems.01356-20.
- He N, Shen G, Jin X, et al. Resveratrol suppresses microglial activation and promotes functional recovery of traumatic spinal cord via improving intestinal microbiota. Pharmacol Res. 2022;183:106377. doi: 10.1016/j.phrs.2022.106377.
- Jing Y, Yu Y, Bai F, et al. Effect of fecal microbiota transplantation on neurological restoration in a spinal cord injury mouse model: involvement of brain-gut axis. Microbiome. 2021;9(1):59. doi: 10.1186/s40168-021-01007-y.
- Kang JN, Sun ZF, Li XY, et al. Alterations in gut microbiota are related to metabolite profiles in spinal cord injury. Neural Regen Res. 2023;18(5):1076–1083. doi: 10.4103/1673-5374.355769.
- Kigerl KA, Hall JC, Wang L, et al. Gut dysbiosis impairs recovery after spinal cord injury. J Exp Med. 2016;213(12):2603–2620. doi: 10.1084/jem.20151345.
- O'Connor G, Jeffrey E, Madorma D, et al. Investigation of microbiota alterations and intestinal inflammation post-spinal cord injury in rat model. J Neurotrauma. 2018;35(18):2159–2166. doi: 10.1089/neu.2017.5349.
- Rong Z, Huang Y, Cai H, et al. Gut microbiota disorders promote inflammation and aggravate spinal cord injury through the TLR4/MyD88 signaling pathway. Front Nutr. 2021;8:702659. doi: 10.3389/fnut.2021.702659.
- Rong ZJ, Cai HH, Wang H, et al. Ursolic acid ameliorates spinal cord injury in mice by regulating gut microbiota and metabolic changes. Front Cell Neurosci. 2022;16:872935. doi: 10.3389/fncel.2022.872935.
- Schmidt EKA, Torres-Espin A, Raposo PJF, et al. Fecal transplant prevents gut dysbiosis and anxiety-like behaviour after spinal cord injury in rats. PLOS One. 2020;15(1):e0226128. doi: 10.1371/journal.pone.0226128.
- Schmidt EKA, Raposo PJF, Torres-Espin A, et al. Beyond the lesion site: minocycline augments inflammation and anxiety-like behavior following SCI in rats through action on the gut microbiota. J Neuroinflammation. 2021;18(1):144. doi: 10.1186/s12974-021-02123-0.
- Myers SA, Gobejishvili L, Saraswat Ohri S, et al. Following spinal cord injury, PDE4B drives an acute, local inflammatory response and a chronic, systemic response exacerbated by gut dysbiosis and endotoxemia. Neurobiol Dis. 2019;124:353–363. doi: 10.1016/j.nbd.2018.12.008.
- Zhang Z, Sui R, Ge L, et al. Moxibustion exhibits therapeutic effects on spinal cord injury via modulating microbiota dysbiosis and macrophage polarization. Aging. 2022;14(14):5800–5811. doi: 10.18632/aging.204184.
- Kim BR, Shin J, Guevarra R, et al. Deciphering diversity indices for a better understanding of microbial communities. J Microbiol Biotechnol. 2017;27(12):2089–2093. doi: 10.4014/jmb.1709.09027.
- Feranchuk S, Belkova N, Potapova U, et al. Evaluating the use of diversity indices to distinguish between microbial communities with different traits. Res Microbiol. 2018;169(4-5):254–261. doi: 10.1016/j.resmic.2018.03.004.
- Williams EK, Chang RB, Strochlic DE, et al. Sensory neurons that detect stretch and nutrients in the digestive system. Cell. 2016;166(1):209–221. doi: 10.1016/j.cell.2016.05.011.
- Margolis KG, Gershon MD, Bogunovic M. Cellular organization of neuroimmune interactions in the gastrointestinal tract. Trends Immunol. 2016;37(7):487–501. doi: 10.1016/j.it.2016.05.003.
- Bazzocchi G, Turroni S, Bulzamini MC, et al. Changes in gut microbiota in the acute phase after spinal cord injury correlate with severity of the lesion. Sci Rep. 2021;11(1):12743. doi: 10.1038/s41598-021-92027-z.
- Rodenhouse A, Talukder MAH, Lee JI, et al. Altered gut microbiota composition with antibiotic treatment impairs functional recovery after traumatic peripheral nerve crush injury in mice: effects of probiotics with butyrate producing bacteria. BMC Res Notes. 2022;15(1):80. doi: 10.1186/s13104-022-05967-8.
- Jing Y, Bai F, Yu Y. Spinal cord injury and gut microbiota: a review. Life Sci. 2021;266:118865. doi: 10.1016/j.lfs.2020.118865.
- Fengzhi L, Xiaoxin C. Intestinal flora and complications of spinal cord injury. Chin J Microecol. 2020;32(6):741–745. doi: 10.13381/j.carolcarrollnki.CJM.202006026.
- Xiaohua Z, Ruihan Z, Wei G, et al. Research progress on the potential effect of intestinal flora on spinal cord injury. Chin J Rehabil Theory Pract. 2019;25(12):1434–1438.
- Jogia T, Ruitenberg MJ. Traumatic spinal cord injury and the gut microbiota: current insights and future challenges. Front Immunol. 2020;11:704. doi: 10.3389/fimmu.2020.00704.
- Hamilton AM, Sampson TR. Traumatic spinal cord injury and the contributions of the post-injury microbiome. Int Rev Neurobiol. 2022;167:251–290. doi: 10.1016/bs.irn.2022.06.003.
- Faber W, Stolwijk-Swuste J, van Ginkel F, et al. Faecal microbiota in patients with neurogenic bowel dysfunction and spinal cord injury or multiple sclerosis—a systematic review. J Clin Med. 2021;10(8):1598. doi: 10.3390/jcm10081598.
- Ding W, You Z, Chen Q, et al. Gut microbiota influences neuropathic pain through modulating proinflammatory and anti-inflammatory T cells. Anesth Analg. 2021;132(4):1146–1155. doi: 10.1213/ANE.0000000000005155.
- Mazzon C, Zanotti L, Wang L, et al. CCRL2 regulates M1/M2 polarization during EAE recovery phase. J Leukoc Biol. 2016;99(6):1027–1033. doi: 10.1189/jlb.3MA0915-444RR.
- Jha MK, Lee WH, Suk K. Functional polarization of neuroglia: implications in neuroinflammation and neurological disorders. Biochem Pharmacol. 2016;103:1–16. doi: 10.1016/j.bcp.2015.11.003.
- Wang H, Liu C, Han M, et al. TRAM1 promotes microglia M1 polarization. J Mol Neurosci. 2016;58(2):287–296. doi: 10.1007/s12031-015-0678-3.
- Xu S, Zhu W, Shao M, et al. Ecto-5'-nucleotidase (CD73) attenuates inflammation after spinal cord injury by promoting macrophages/microglia M2 polarization in mice. J Neuroinflammation. 2018;15(1):155. doi: 10.1186/s12974-018-1183-8.
- Wallace DJ, Sayre NL, Patterson TT, et al. Spinal cord injury and the human microbiome: beyond the brain-gut axis. Neurosurg Focus. 2019;46(3):E11. doi: 10.3171/2018.12.FOCUS18206.
- Musleh-Vega S, Ojeda J, Vidal PM. Gut Microbiota-Brain axis as a potential modulator of psychological stress after spinal cord injury. Biomedicines. 2022;10(4):847. doi: 10.3390/biomedicines10040847.
- Zhang Y, Lang R, Guo S, et al. Intestinal microbiota and melatonin in the treatment of secondary injury and complications after spinal cord injury. Front Neurosci. 2022;16:981772. doi: 10.3389/fnins.2022.981772.
- Kigerl KA, Mostacada K, Popovich PG. Gut microbiota are disease-modifying factors after traumatic spinal cord injury. Neurotherapeutics. 2018;15(1):60–67. doi: 10.1007/s13311-017-0583-2.
- Blaser MJ. Fecal microbiota transplantation for dysbiosis – predictable risks. N Engl J Med. 2019;381(21):2064–2066. doi: 10.1056/NEJMe1913807.
- Sanders ME, Merenstein DJ, Reid G, et al. Probiotics and prebiotics in intestinal health and disease: from biology to the clinic. Nat Rev Gastroenterol Hepatol. 2019;16(10):605–616. doi: 10.1038/s41575-019-0173-3.
- Pang R, Wang J, Xiong Y, et al. Relationship between gut microbiota and lymphocyte subsets in Chinese Han patients with spinal cord injury. Front Microbiol. 2022;13:986480. doi: 10.3389/fmicb.2022.986480.
Appendix
1. Search strategy for PubMed
2.
Search strategy for EMBASE
3. Search strategy for Web of Science