Abstract
Background
The rapid adoption of next-generation sequencing in clinical oncology has enabled detection of molecular biomarkers which are shared between multiple tumour types. Intra-tumour heterogeneity is a mechanism of therapeutic resistance and therefore an important clinical challenge. However, the tumour-related copy number variants (CNVs), as key regulators of cancer origination, development, and progression, across various types of cancers are poorly understood.
Methods
We performed pan-cancer CNV analysis of cancer-related genes in 15 types of cancers including 1438 cancerous patients by next-generation sequencing using a commercially available pan-cancer panel (Onco PanScan™). Downstream bioinformatics analysis was performed in order to detect CNVs, cluster analysis of the found CNVs, and comparison of the frequency of gained CNVs between different types of cancers. LASSO analysis was used for identification of the most important CNVs.
Results
We also identified 523 CNVs among which 16 CNVs were common while 22 CNVs were caner-specific CNVs. Meanwhile, FAM58A was most commonly found in all studied cancers in this study and significant differences were found in FAM58A between female and male patients (p = .001). Common CNVs, such as FOXA1, NFKBIA, HEY1, MECOM, CHD7, AGO2, were mutated in 6.79%, 8.45%, 7.51%, 6.43%, 7.59%, 8.16% of tumours, while most of these mutations have proven roles in positive regulation of transcription from RNA polymerase II promoter. 11 features including sex, DIS3, EPHB1, ERBB2, FLT1, HCK, KEAP1, MYD88, PARP3, TBX3, and TOP2A were found as the key features for classification of cancers using CNVs.
Conclusion
The 16 common CNVs between cancers can be used to identify the target of pan-cancer drug design and targeted therapies. Additionally, 22 caner-specific CNVs can be used as unique diagnostic markers for each cancer type.
Introduction
Cancer, a complicated genetic disease with several types and subtypes, has become a worldwide concern. Multiple genetic factors, such as point mutation, DNA copy number variation, epigenetics changes, RNA transcription difference, protein expression difference, and so on [Citation1–4] have critical effects on the occurrence and progression of cancers. Copy number variants (CNVs) are an important form of genetic variation, which can affect cancer susceptibility by regulating gene expression [Citation5, Citation6].
With the advantage of next-generation sequencing (NGS), medical researchers have access to identify massive amounts of data around genomic profiles of cancers. Although all cancers are molecularly different; however, many of them share common molecular characteristics, such as common driver mutations [Citation7, Citation8], suggesting the widespread heterogeneity of tumours. The Cancer Genome Atlas dataset was the base for the first and still most comprehensive analysis, by which the term ‘pan-cancer’ was coined in. Analysis of molecular aberrations and their functional roles across multiple cancer types, known as pan-cancer analysis, has emerged as a powerful method to identify similarities and differences of molecular aberrations between cancers, which helps researchers and physicians to better understand tumours and provides broad-spectrum targets for the clinical diagnosis and treatment of multiple tumours. For example, Nguyen et al. demonstrated the potential diagnostic value of pan-cancer genomics-based homologous recombination deficiency testing for patient stratification towards treatment with poly ADP-ribose polymerase inhibitors [Citation9]. Jiang et al. found that ARID1A alterations could act as pan-cancer predictive biomarkers which can be targeted by immune checkpoint inhibitors treatment [Citation10]. To date, pan-cancer analysis based on CNVs has not been fully discussed among cancers, while we believe that it is a massive information resource.
Herein, we performed a pan-cancer analysis of CNVs among 15 types of cancer firstly by utilizing the 509 genes test panel through NGS technology to provide new directions for tumour biology research both in diagnosis and treatment.
Methods
Patients
From January 2021 to January 2022, a total of 1438 Chinese patients from 15 types of cancer in Jiangxi Cancer Hospital were selected to be included in this study. They were composed of 27 patients with breast cancer (BC), 38 patients with cholangiocarcinoma (CHOL), 100 patients with colon cancer (COAD), 24 patients with colon and rectal cancer (COADREAD), 63 patients with oesophageal cancer (ESCA), 53 patients with gastric cancer (STAD), 115 patients with glioma (GLIOMA), 195 patients with liver cancer (LIHC), 525 patients with lung cancer (LUNG), 28 patients with ovarian cancer (OV), 47 patients with pancreatic cancer (PAAD), 33 patients with prostate cancer (PRAD), 73 patients with rectum carcinoma (READ), 39 patients with renal cell carcinoma (RCC), and 24 patients with bladder urothelial carcinoma (BLCA). Both biopsies and blood from cancer samples were collected for somatic testing using a commercially available pan-cancer panel: 509-gene panel (Onco PanScan™, Genetron Health). The study was approved by the Ethics Committee of Jiangxi Cancer Hospital and all participants signed an informed consent before sample collection.
CNV detection
Genomic DNA was extracted from whole blood or fresh tumour tissues using a QIAamp DNA & Tissue Mini Kit (Qiagen) and from formalin-fixed paraffin-embedded (FFPE) tumour tissue using a QIAamp DNA FFPE Tissue Kit (Qiagen), respectively. DNA samples were quantified with a spectrophotometer Nanodrop 2000/2000C (Thermo Scientific) and qualified through migration on agarose gel. DNA extracts were fragmented and tailed by the TIANSeq Fragment/Repair/Tailing Module (TIANGEN). Libraries were constructed using the NEXTflex Rapid DNA-Seq Kit (Bioo Scientific). Hybridization capture-based targeted NGS was processed on Illumina NavoSeq S4 flowcell (Illumina Inc.). A 509-gene panel (Onco PanScan™, Genetron Health) was used to profile CNVs. After removing joint sequences and low-quality regions with Trimmomatic software, sequencing reads were mapped to the reference genome: the hg19 genome (GRch37) with BWA software. Error correction and sequence reconstruction were conducted by Gencore software. Copy number variations (CNVs) were identified with cnvPicker software.
Data collection and preprocessing
Except for data on identified CNVs of each patient, we also collected demographic data, including sample ID, enrolment time, age, sex, sample source and cancer types. After combination of all data of identified CNVs of each patient by the ‘pandas’ python package, we matched the identified CNVs dataset with demographic dataset by ACESS software to obtain the final dataset for data analysis.
CNV data analysis
We used SPSS to calculate the frequency of each identified CNV of each type of cancer and demographic characteristics. The overlapping CNVs among all cancer types were shown by Venn plot, and defined as common CNVs. Namely, ‘common CNVs’ refers to the portion of CNV that is common to the detected in each cancer type, and the ‘cancer-specific CNVs’ refers to those that are detectable in only one cancer type and not in the rest. Gene set enrichment analysis (GSEA) was performed using enrichR (https://maayanlab.cloud/Enrichr/) for each cancer type using the most frequent CNVs to yield a wide range of signalling pathways for each cancer. Clustering analysis and heatmap of identified CNVs frequency was done using the TBtools [Citation11]. Evaluation of association between every pair of cancers based on the frequency of CNVs was performed using Pearson’s correlation test. Gene Ontology (GO) and Kyoto Encyclopedia of Genes and Genomes (KEGG) analysis of common CNVs among 15 kinds of cancers were performed by DAVID (the Database of Annotation, Visualization and Integrated Discovery). The logistic regression analysis of correlations between age (group 1: <31, group 2: 31–60, group 3: >60), sex, cancer type and the frequency of identified CNVs with the highest rate was conducted by SPSS. p < .05 was considered statistically significant.
LASSO for identification of most important CNVs
In order to find the key CNVs for differentiation between 15 types of cancers, we used least absolute shrinkage and selection operator (LASSO), which is a machine learning method. In addition, the importance of features was also calculated using random forest, support vector machine, and gradient boosting classifier (GBClassifier). This analysis was performed in Python (V. 3.10) using ScikitLearn (v. 1.3.0).
Results
Characteristics of all patients
All patients in the study included 825 males and 559 females, with an average age of 58.95 years. The baseline demographic characteristics of all patients are shown in .
Table 1. The baseline demographic characteristics of all patients.
Identified CNVs
A total of 523 CNVs were detected in all patients. All detected CNVs are gain types located on chromosomes. Of all CNVs, the three with the highest frequency of CNVs in all cancers were FAM58A (15.82%), ABCC5 (13.29%) and PRSS1 (11.56%). Of all cancers, the cancer with the highest frequency of CNV is COADREAD, and the CNVs with the highest frequency were ASXL1, PTPRT, SRC and ZNF217 (all 41.67%).
Comparison of CNV profiles between 15 types of cancers yielded 16 common CNVs, including ABCC5, AGO2, ARID5B, CHD7, FAM58A, FOXA1, HEY1, HLA-C, HLA-DQB1, MCL1, MECOM, MSN, NFKBIA, PRSS1, RAD21, and RECQL4. We also found 22 cancer-specific CNVs: ALOX12B of ovarian cancer (3.57%), APC of glioma (0.87%), BCL2L11 of glioma (0.87%), CBL of lung cancer (0.19%), CUL3 of LIHC (0.51%), CYP17A1 of glioma (0.87%), ELAC2 of lung cancer (0.19%), ESR1 of lung cancer (0.19%), ESR2 of lung cancer (0.38%), EXT2 of lung cancer (0.19%), FAS of lung cancer (0.19%), IGF2R of lung cancer (0.38%), MSR1 of glioma (0.87%), MST1R of urothelial carcinoma (8.33%), MUC16 of BC (3.70%), NCOR1 of RCC (2.56%), NUTM1 of lung cancer (0.19%), PTPRS of glioma (1.74%), ROS1 of lung cancer (0.38%), SETD2 of ovarian cancer (3.57%), SPRED1 of glioma (0.87%), SYK of glioma (1.74%). The frequency of each CNV in each cancer is shown in Supplementary Table 1. The heatmap of all 523 identified CNVs in each cancer is shown in . Identified common CNVs and cancer-specific CNVs are shown in .
GSEA analysis of identified CNVs
We compared 15 types of cancers in terms of significance of found signalling pathways based on CNV profiles. Despite the low similarity between CNV profiles between 15 cancer types, most of the cancers had similar signalling pathways which were found based on the CNV profiles. Accordingly, difference between PAAD and READ (p = .0175), OV and PAAD (p = .0116), GLIOMA and PAAD (p = .010), COADREAD and PAAD (p = .0248), and COAD and PAAD (p = .010), BLCA and PAAD (p = .0142), and BC and PAAD (p = .0106) was significant at the level of <.05 (significance level < .05, *). In addition, the difference between BC and CHOL (p = .004), BLCA and CHOL (p = .006), CHOL and COAD (p = .004), CHOL and ESCA (p = .004), CHOL and GLIOMA (p = .004), CHOL and READ (p = .008), and ESCA and PAAD (p = .009) was significant at the level of <.01 (significance level <.01, **). The Heatmap plot of GSEA analysis of identified CNVs is shown in .
Cluster analysis of all identified CNVs and CNV profiles of each cancer
By cluster analysis of CNV profiles of each cancer, we found that COAD and READ, as well as CHOL and LUNG had similar CNV profiles. The cluster heatmap is also shown in . Common CNVs in two cluster after cluster analysis is visualized by Venn plot in .
Figure 4. The Venn plot of common CNVs in two clusters after cluster analysis: (A) the Venn plot of commonly identified CNVs in COAD and READ; (B) the Venn plot of commonly identified CNVs in CHOL and LUNG.
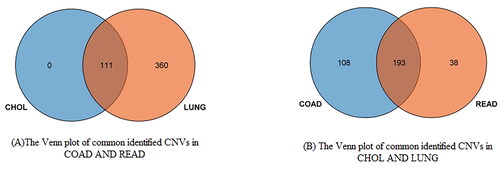
Results of Pearson’s correlation test showed that the most similar CNV profiles are found between BC and CHOL (r = 0.455, p = 4.16e − 28), BC and BLCA (r = 0.432, p = 3.58e − 025), and BLCA and CHOL (r = 0.266, p = 6.45e − 10), while a significant negative correlation was found between CNVs of GLIOMA and BLCA (r = 0.09, p = .039). Therefore, we found that studied CNVs are common between 15 types of cancers, but similar frequencies of CNVs were found between mentioned cancer types. In other words, it can be stated that each cancer type has its unique category of CNVs. Therefore, remaining types of cancers have non-significant similarities in terms of CNV profile. The correlation matrix between every pair of cancers is shown in .
KEGG and GO analysis of identified common CNVs
By GO analysis of identified common CNVs, the top three BP is Positive regulation of transcription from RNA polymerase II promoter (p < .001, FDR = 0.308), Notch signalling pathway (p < .001, FDR = 0.421), apoptotic process (p < .001, FDR = 0.624); the top three CC is nucleoplasm (p < .001, FDR = 0.021), nucleus (p < .001, FDR = 0.047), membrane (p < .001, FDR = 0.115); top three MF is DNA binding (p < .001, FDR = 0.137), MHC class II receptor activity (p < .001, FDR = 0.376), Peptide antigen binding (p < .05, FDR = 0.608). By KEGG analysis of common copy number variation genes, the top three pathway is Influenza A (p < .05, FDR = 0.629), Epstein–Barr virus infection (p < .05, FDR = 0.629), Human T-cell leukaemia virus 1 infection (p < .05, FDR = 0.629). The visual results of GO and KEGG analysis of identified common CNVs are, respectively, shown in .
Correlation analysis between age, sex and the highest frequency of identified CNVs FAM58A
By logistic regression analysis, we found that sex (OR = 0.588, 95%CI: 0.430–0.805, p = .001) was statistically significant. Sex was associated with the frequency of FAM58 ().
Table 2. The association of age, sex, cancer type and the frequency of FAM58A.
Classification of cancers using CNVs
Data from 525 features used in this study including 523 CNVs, age, and sex were analysed using LASSO algorithm to find the most important features for differentiation between 15 types of cancers. According to the results, 11 features including sex, DIS3, EPHB1, ERBB2, FLT1, HCK, KEAP1, MYD88, PARP3, TBX3, and TOP2A were found as the key features for this classification. The coefficients of each feature are represented in . In addition, the importance of features was calculated using RF, logistic regression, and GB classifier. The obtained coefficients are summarized in .
Table 3. Summary of the coefficients of important features for differentiation between 15 cancer types.
Discussion
Cancer diagnosis and treatment depend on accurate cancer classification. The promising classification method based on molecular alterations rather than tissue origins has been frequently discussed following the appearance of pan-cancer analysis [Citation12, Citation13]. In this study, we performed a pan-cancer analysis of CNVs among 15 types of cancer with the aim of clarifying the similarities and differences of gained CNVs between 15 types of cancers, and the association of CNV profiles with age and sex of patients in order to help find more efficient treatment methodologies and understand underlying mechanisms of cancers.
By analysing pan-cancer copy number variation data, we identified 16 common CNVs. FAM58A, the highest frequency of identified CNVs, also named CCNQ, played an important role in activating cyclin for cyclin-dependent kinase 10 (CDK10). CDK10 is an important kinase for neural development and it is known as a tumour suppressor [Citation14, Citation15]. Most recent study provide an in-depth analysis of CDK10 substrate specificity and function, and their results showed that CDK10 adopts a hybrid position in both cell cycle and transcriptional regulation [Citation16]. CDK10 expression alteration was related to multiple cancers, including hepatocellular carcinoma [Citation17], BC [Citation18, Citation19] and biliary tract cancer [Citation20], gastric cancer [Citation21, Citation22]. In addition, reduced CDK10 expression predicts a poor prognosis in patients with gastric cancer [Citation21]. Ectopic CDK10 expression inhibited gastric cancer cell proliferation, migration and invasion, while knockdown of CDK10 promoted these phenotypes [Citation22]. Rhie et al. found that gaining CNV of CDK10 led to better overall survival in all oropharyngeal squamous cell carcinoma patients [Citation4]. In this study, compared to normal tissues, the CNVs of FAM58A from tumour tissues among 15 types of cancer were gained. Weiswald et al. show that the gene expression level of CDK10 in human colorectal adenocarcinoma tissues and cell lines is significantly higher than in the normal colon, which may be associated with the gain of CNVs of FAM58A [Citation15]. In theory, the gain of CNVs of cyclin family member FAM58A could activate CDKs to expand anti-cancer function. Therefore, FAM58A has the potential to become a new molecular therapeutic target. Additionally, we uncovered the frequency of FAM58A differs with sex, which might be important for the gender differences in tumour incidence and survival.
AT-rich interaction domain 5B (ARID5B) is an AT-rich interaction domain DNA-binding motif-containing transcription factor. Cancer associated with ARID5B adenoid includes cystic carcinoma, such as ovarian cancer, prostate cancer, BC and gastric cancer. The expression of ARID5B gene in ovarian cancer tissues was significantly lower than that in the normal ovarian tissues. Patients with high expression of ARID5B gene were significantly better than those with low expression, and the prognosis of patients with high expression was better [Citation23]. The higher expression of ARID5B in primary prostate cancer than normal prostate also was observed [Citation24]. In BC, the prognostic value of ARID5B is reflected in the association between high expression of ARID5B and favourable outcomes [Citation25]. On the contrary, upregulation of ARID5B was related to a poor Hepatocellular Carcinoma outcome [Citation26]. In gastric cancer, the oncogenic properties of TEAD4 and its novel target ARID5B involved in cell proliferation and migration [Citation27]. In our pan-cancer analysis of 15 types of cancer, including ovarian cancer, prostate cancer, BC and gastric cancer, CNVs were found in them. The change in copy number of ARID5B in them may be related to the level of expression, and then the link between them needs to be confirmed in future research. Another pan-cancer analysis of ARID family members demonstrated that ARID5B among the family could serve as a novel biomarker for immune checkpoint inhibitor therapy [Citation28]. Considering the common characteristics among cancers in our study, the previously discovered treatment direction may also be extended to different types of tumours.
In addition to the above two, FOXA1 was also identified as a common CNVs, and has been demonstrated to predict response to targeted therapy. Under the alteration of somatic mutations (single nucleotide polymorphisms and small insertion/deletion polymorphism) in FOXA1, ADRB1 and ADRB2 gene expression increased, followed by reduction of the overall survival of solid tumour patients in the GDC Pan-Cancer set. Analysis of these tumour mutations might indicate whether an individual prostate cancer patient will respond to beta blockers [Citation29]. Suberoyl anilide hydroxamic acid (SAHA) is one of the most advanced pan-inhibitor of histone deacetylases inhibitor. A BC study revealed that SAHA can promote inhibitor of histone deacetylases of triple-negative BC cells via FOXA1 signals, which suggests that more attention should be paid when SAHA is used as anti-cancer agent for cancer treatment [Citation30].
The identification of cancer-specific biomarkers has important applications for the diagnosis and cancer-specific development of precision therapies. In this study, we also found some cancer-specific CNVs. For example, ROS1 (ROS proto-oncogene 1, receptor tyrosine kinase) is a protein-coding gene. Diseases associated with ROS1 include lung cancer susceptibility 3 and lung cancer. Drugs and therapeutics for lung cancer susceptibility 3 contain endostatins, endostar protein, gemcitabine, vinorelbine and afatinib.
By comparison, CNV profiles among 15 types of cancer, COAD and READ, as well as CHOL and LUNG had similar CNV profiles. COAD and READ belong to large intestine cancer. Molecular similarities among histologically or anatomically related cancer types provide a basis for focused pan-cancer analyses, such as pan-large intestine cancer, which in turn may inform strategies for future therapeutic development.
Limitations
Given the sample size and study design, the results obtained in this study need to be verified by larger samples or further gene expression analysis.
Conclusions
Our pan-cancer analysis of 523 CNVs among 15 types of cancer helps to find undiscovered cancer-associated CNVs, and provides a new classification of tumours based on their molecular characteristics that could probably better match individual patients.
Authors contributions
KPY, LN, WYZ and JZ designed the study. BYW, CWH, LD and CC collected the data. ZZL, CL, WHK and HHL analysed the data. KPY and LN wrote the manuscript. ZQL made significant contributions in revising the manuscript. All authors read and approved the final manuscript.
Supplemental Material
Download MS Excel (127.4 KB)Disclosure statement
The authors report no conflict of interest.
Data availability statement
The data that support the findings of this study are available from the corresponding author.
Additional information
Funding
References
- Wang K, Yuen ST, Xu J, et al. Whole-genome sequencing and comprehensive molecular profiling identify new driver mutations in gastric cancer. Nat Genet. 2014;46(6):1–9. doi: 10.1038/ng.2983.
- Kanwal R, Gupta S. Clin genet. Clin Genet. 2012;81(4):303–311. doi: 10.1111/j.1399-0004.2011.01809.x.
- Zhou R, Wu Y, Wang W, et al. Circular RNAs (circRNAs) in cancer. Cancer Lett. 2018;425:134–142. doi: 10.1016/j.canlet.2018.03.035.
- Rhie A, Park WS, Choi MK, et al. Genomic copy number variations characterize the prognosis of both P16-positive and P16-negative oropharyngeal squamous cell carcinoma after curative resection. Medicine (Baltimore). 2015;94(50):e2187. doi: 10.1097/MD.0000000000002187.
- Dear PH. Copy-number variation: the end of the human genome? Trends Biotechnol. 2009;27(8):448–454. doi: 10.1016/j.tibtech.2009.05.003.
- Wei R, Zhao M, Zheng C-H, et al. Concordance between somatic copy number loss and down-regulated expression: a pan-cancer study of cancer predisposition genes. Sci Rep. 2016;6:37358. doi: 10.1038/srep37358.
- Saghafinia S, Mina M, Riggi N, et al. Pan-cancer landscape of aberrant DNA methylation across human tumors. Cell Rep. 2018;25(4):1066–1080.e8. doi: 10.1016/j.celrep.2018.09.082.
- Yang X, Gao L, Zhang S. Comparative pan-cancer DNA methylation analysis reveals cancer common and specific patterns. Brief Bioinform. 2017;18(5):761–773.
- Nguyen L, Martens JWM, Van Hoeck A, et al. Pan-cancer landscape of homologous recombination deficiency. Nat Commun. 2020;11(1):5584. doi: 10.1038/s41467-020-19406-4.
- Jiang T, Chen X, Su C, et al. Pan-cancer analysis of ARID1A alterations as biomarkers for immunotherapy outcomes. J Cancer. 2020;11(4):776–780. doi: 10.7150/jca.41296.
- Chen C, Chen H, Zhang Y, et al. TBtools: an integrative toolkit developed for interactive analyses of big biological data. Mol Plant. 2020;13(8):1194–1202. doi: 10.1016/j.molp.2020.06.009.
- Zhu L, Miao Y, Xi F, et al. Identification of potential biomarkers for pan-cancer diagnosis and prognosis through the integration of large-scale transcriptomic data. Front Pharmacol. 2022;13:870660. doi: 10.3389/fphar.2022.870660.
- Cheerla N, Gevaert O. MicroRNA based pan-cancer diagnosis and treatment recommendation. BMC Bioinformatics. 2017;18(1):32. doi: 10.1186/s12859-016-1421-y.
- Bazzi ZA, Tai IT. CDK10 in gastrointestinal cancers: dual roles as a tumor suppressor and oncogene. Front Oncol. 2021;11:655479. doi: 10.3389/fonc.2021.655479.
- Weiswald L-B, Hasan MR, Wong JCT, et al. Inactivation of the kinase domain of CDK10 prevents tumor growth in a preclinical model of colorectal cancer, and is accompanied by downregulation of Bcl-2. Mol Cancer Ther. 2017;16(10):2292–2303. doi: 10.1158/1535-7163.MCT-16-0666.
- Düster R, Ji Y, Pan K-T, et al. Functional characterization of the human Cdk10/Cyclin Q complex. Open Biol. 2022;12(3):210381. doi: 10.1098/rsob.210381.
- Zhong X-Y, Xu X-X, Yu J-H, et al. Clinical and biological significance of Cdk10 in hepatocellular carcinoma. Gene. 2012;498(1):68–74. doi: 10.1016/j.gene.2012.01.022.
- Khanal P, Yun HJ, Lim SC, et al. Proyl isomerase Pin1 facilitates ubiquitin-mediated degradation of cyclin-dependent kinase 10 to induce tamoxifen resistance in breast cancer cells. Oncogene. 2012;31(34):3845–3856. doi: 10.1038/onc.2011.548.
- You Y, Li H, Qin X, et al. Decreased CDK10 expression correlates with lymph node metastasis and predicts poor outcome in breast cancer patients – a short report. Cell Oncol (Dordr). 2015;38(6):485–491. doi: 10.1007/s13402-015-0246-4.
- Yu J-H, Zhong X-Y, Zhang W-G, et al. CDK10 functions as a tumor suppressor gene and regulates survivability of biliary tract cancer cells. Oncol Rep. 2012;27(4):1266–1276. doi: 10.3892/or.2011.1617.
- Zhao BW, Chen S, Li YF, et al. Low expression of CDK10 correlates with adverse prognosis in gastric carcinoma. J Cancer. 2017;8(15):2907–2914. doi: 10.7150/jca.20142.
- You Y, Bai F, Ye Z, et al. Downregulated CDK10 expression in gastric cancer: association with tumor progression and poor prognosis. Mol Med Rep. 2018;17(5):6812–6818.
- Yang Y, Yin X, Ni N. The expression of ARID5B gene in ovarian cancer based on oncomine database and the intervention study of sanguinarine. J Pract Obstet Gynecol. 2019;35(7):539–543.
- Yamakawa T, Waer C, Itakura K. At-rich interactive domain 5B regulates androgen receptor transcription in human prostate cancer cells. Prostate. 2018;78(16):1238–1247. doi: 10.1002/pros.23699.
- Zhang JF, Hou SY, You ZL, et al. Expression and prognostics of ARID family members in breast cancer. Aging (Albany NY). 2021;13(4):5621–5637. doi: 10.18632/aging.202489.
- Sun J, Cheng NS. Comprehensive landscape of ARID family members and their association with prognosis and tumor microenvironment in hepatocellular carcinoma. J Immunol Res. 2022;2022:1688460.
- Lim B, Park JL, Kim HJ, et al. Integrative genomics analysis reveals the multilevel dysregulation and oncogenic characteristics of TEAD4 in gastric cancer. Carcinogenesis. 2014;35(5):1020–1027. doi: 10.1093/carcin/bgt409.
- Zhu Y, Yan C, Wang XF, et al. Pan-cancer analysis of ARID family members as novel biomarkers for immune checkpoint inhibitor therapy. Cancer Biol Ther. 2022;23(1):104–111. doi: 10.1080/15384047.2021.2011643.
- Lehrer S, Rheinstein PH. The ADRB1 (adrenoceptor beta 1) and ADRB2 genes significantly co-express with commonly mutated genes in prostate cancer. Discov Med. 2020;30(161):163–171.
- Wu S, Luo Z, Yu P-J, et al. Suberoylanilide hydroxamic acid (SAHA) promotes the epithelial mesenchymal transition of triple negative breast cancer cells via HDAC8/FOXA1 signals. Biol Chem. 2016;397(1):75–83. doi: 10.1515/hsz-2015-0215.