Abstract
Background
Acute-on-chronic liver failure (ACLF) is a distinctive and severe syndrome, marked by an excessive systemic inflammatory response. In vivo, interleukin 8 (IL-8) is an essential pro-inflammatory cytokine. We aimed to investigate the value of serum IL-8 levels in predicting mortality in ACLF patients in the background of hepatitis B virus-related cirrhosis.
Methods
In this study, we conducted a retrospective analysis of the clinical baseline characteristics of 276 patients with ACLF in the context of HBV-related cirrhosis. Logistic regression analysis was employed to identify independent risk factors for short-, intermediate-, and long-term mortality. Using these independent risk factors, we developed a nomogram model, which was subsequently validated. To assess the clinical usefulness of the nomogram model, we performed decision curve analysis (DCA).
Results
Out of the 276 patients with ACLF, 98 (35.5%), 113 (40.9%), and 128 (46.4%) died within 28, 90, and 180 days, respectively. Serum IL-8 levels were only an independent predictor of 28-day mortality and could simply classify ACLF patients. Conversely, mean arterial pressure (MAP), HBV-DNA, and COSHACLF IIs were independent predictors of mortality across all three observation periods. We constructed a nomogram based on IL-8 that was able to visualise and predict 28-day mortality with a C-index of 0.901 (95% CI: 0.862–0.940). Our calibration curves, Predicted Probability of Death & Actual Survival Status plot, and Confusion Matrix demonstrated the nomogram model’s strong predictive power. DCA indicated the nomogram’s promising clinical utility in predicting 28-day mortality in ACLF patients.
Conclusion
Serum IL-8 levels predict short-term mortality in ACLF patients in the background of HBV-associated cirrhosis, and the developed Nomogram model has strong predictive power and good clinical utility.
KEY MESSAGE
Systemic inflammatory response is a pathophysiological feature of patients with acute-on-chronic liver failure, and the serum level of interleukin-8 can predict the short-term prognosis of patients.
Introduction
Acute-on-chronic liver failure (ACLF) occurs in patients with chronic liver disease and is a life-threatening clinical disease characterised by a high 28-day mortality rate [Citation1]. Hepatitis B virus (HBV), which affects an estimated 350 million people worldwide, is a major cause of ACLF episodes, especially in the Asia-Pacific region [Citation2, Citation3]. Cirrhosis is a common chronic liver disease, and given that cirrhosis is a progressive disease, it can be complicated by ACLF. Furthermore, the knowledge of the prognosis in patients with cirrhosis is rapidly being updated, with most patients dying due to acute deterioration of liver function driven by precipitating events [Citation4], and ACLF is the clinical manifestation of this concept. The condition of ACLF patients at admission is related to the prognosis [Citation5], and the pathophysiological characteristics of ACLF are characterised by a highly dynamic process [Citation3]. Therefore, there is an urgent need for a biomarker that can enable early and accurate risk stratification of ACLF patients progressing to cirrhosis. However, there are no satisfactory biomarkers that address such dilemmas.
Systemic inflammation in patients with ACLF has been widely described. Immune imbalance is the core of its pathogenesis and outcome, and an excessive systemic inflammatory response can lead to organ failure [Citation4, Citation6, Citation7]. Leukocyte mitochondrial dysfunction with abnormal leukocyte immunometabolism has been reported in ACLF patients [Citation8], which gives us a new perspective on the relationship between the immune response products abnormally regulated by leukocytes and the severity of disease in ACLF patients. Interestingly, there is a correlation between proinflammatory factors and abnormal leukocyte energy metabolism in other diseases [Citation9]. Interleukin 8 (IL-8) is a classical inflammatory factor in the body. Studies have reported that the levels of certain inflammatory factors in the body predict prognosis in patients with ACLF [Citation10, Citation11]. However, it is not known what role circulating IL-8 levels play in the prognosis of ACLF patients. Based on the above evidence, the prognostic value of IL-8 in patients with HBV-ACLF should be discussed in depth.
In fact, several prognostic scores exist to assess patients with chronic liver disease, such as the Child-Turcotte-Pugh (CTP), Model for End-Stage Liver Disease (MELD), Model for End-Stage Liver Disease with Sodium (MELD-Na) and COSSHACLF IIs [Citation12]. However, there are still limitations of these scores. For example, the CTP score is highly subjective and fails to take into account the impact of different aetiologies on liver function. The MELD score and MELD-Na score have limited application in some special cases, such as artificial liver support therapy, and hepatorenal syndrome. The COSSHACLF IIs, a prognostic score for predicting HBV-related ACLF recently developed by a team from China, currently demonstrates excellent predictive ability, but the score items fail to adequately take into account the inorganic immune inflammatory profile. Therefore, the development of a new score based on the level of pro-inflammatory biomarkers is worth trying and necessary.
In this study, we aimed to identify the prognostic value of serum IL-8 levels in predicting HBV-associated ACLF patients at different time points to develop a new model to predict mortality in ACLF patients based on serum IL-8 levels.
Methods
Patients
This retrospective study included patients with HVB-ACLF who were hospitalised in the First Affiliated Hospital of Nanchang University from April 2021 to March 2022. The inclusion criteria were 1) ≥18 years old, 2) diagnosed with ACLF and 3) clinical information is available including serum IL-8 levels. Exclusion criteria included 1) Combined with other causes of hepatitis, 2) hepatocellular carcinoma, 3) previous liver transplantation, 4) complications associated with other severe chronic non-hepatic diseases, such as coronary heart disease or acute heart failure under anticoagulation treatment, uncontrolled diabetes, or chronic kidney disease, 5) pregnancy and 6) infection with HIV or receiving immune-suppressive medication. The study flow diagram was shown in , and a total of 276 patients with HBV-related-ACLF on a cirrhotic background were included in this cohort and subsequently analysed. The study flow diagram is shown in , and a total of 276 patients with HBV related ACLF on a cirrhotic background were included in this cohort and subsequently analysed. Our study was approved by the Ethics Committee of the First Affiliated Hospital of Nanchang University (IIT [2021] 09). Informed consents of the patients were waived by the Ethics Committee of the First Affiliated Hospital of Nanchang University because of the retrospective and anonymous nature of the study.
Definitions
The diagnostic criteria for ACLF are established by the 2019 consensus of the Asia-Pacific Association for the Study of the Liver (APASL) and are defined as follows: patients presenting with acute liver injury demonstrated by jaundice (serum bilirubin ≥ 5 mg/dL) and coagulation dysfunction [international normalised ratio (INR) ≥1.5 or prothrombin activity < 40%], complicated within 4 weeks by clinical ascites and/or encephalopathy, and previously diagnosed or undiagnosed chronic liver disease/cirrhosis [Citation3]. The CTP score [Citation13] is computed based on albumin, ascites, serum bilirubin, hepatic encephalopathy, and prothrombin time. The MELD score [Citation14] = 3.8 × log (bilirubin [mg/dL]) + 11.2 × log (INR) + 9.6 × log (creatinine [mg/dL]) + 6.43. MELD-Na formula [Citation15] = (0.025 × MELD × (140–Na)) + 140. COSSH-ACLF IIs [Citation12] = 1.649 × ln(INR)+ 0.457 × HE score (HE grade: 0/1, 1–2/2 and 3–4/3) + 0.425 × ln(neutrophil [109/L]) +0.396 × ln(TB [umol/L]) + 0.576 × ln(serum urea [mmol/L]) + 0.033 × age.
Clinical data and treatment
The following demographic and clinical data were collected from the patient’s electronic medical record system. Clinical data also included inflammatory factors such as serum interleukin-8 (IL-8). IL-8 levels were determined with a cytokine detection reagent kit (AtomLife, Nanjing, China) based on flow cytometry with fluorescence and luminescence technology. The samples were analysed using a DxFLEX flow cytometer (Beckman, CA, USA). Laboratory parameters were measured in all subjects using fasting venous blood samples within the first 24 h of admission. All HBV-ACLF patients were followed up for 28, 90 and 180 days and mortality was measured at 28, 90 and 180 days.
All patients were administered nucleoside analogues, which were oral anti-HBV drugs, and received standard supportive care during their hospitalisation. Moreover, patients were also closely monitored for potential complications. All decisions related to patient management were made by the attending clinicians based on the standard recommendations [Citation3, Citation16] and the individual patient’s condition.
Statistical analysis
Continuous variables are shown as the mean and standard deviation (SD) or median and interquartile range (IQR), while categorical variables are shown as frequencies (%). We tested whether the explanatory variable had an interaction and found no significant interactions within the included variables. Student’s t tests or Mann–Whitney U test were performed for group comparisons. The diagnostic accuracy of rebleeding was assessed by receiver operating characteristic (ROC) analysis. Areas under the ROC curves (AUCs) were compared by the method of DeLong et al. All levels of significance were set at a two-sided 5% level. All analyses were performed in R 4.1.2 (R Project for Statistical Computing, Vienna, Austria). The R statistical packages gtsummary tidyverse, performance, pROC, rms, compareGroups, performance, and barplot3d were used. The source file “stdca.r” was obtained from the website www.mskcc.org, which was used to draw the decision curve analysis (DCA) curves.
Results
Baseline characteristics of ACLF patients in the background of hepatitis B-related cirrhosis
This study retrospectively analysed 276 patients with ACLF in the background of hepatitis B-related cirrhosis (), the majority of whom were men, and the median age of this cohort was 49.3 years. Patients had a slightly lower mean arterial pressure level (86.8 ± 12.4 mmHg), a significantly abnormal liver function status including abnormally elevated levels of TBIL, AST, ALT, ALP, GGT, and decreased serum albumin levels. Some patients have impaired renal function, as evidenced by elevated serum creatinine levels and/or serum urea nitrogen. The majority of the patients did not have hepatic encephalopathy at the time of admission, and a comparison of IL-8 levels under specific complications is shown in Supplementary Table 1. Included patients had elevated liver-related prognostic scores at admission, as demonstrated by elevated CTP, MELD, MELD-Na, and COSSHACLF IIs. Moreover, the patients in this cohort had mortality rates of 35.5%, 40.9%, and 46.4% at 28, 90, and 180 days, respectively.
Table 1. Baseline characteristics of the enrolled patients.
Univariate and multivariate analyses identified risk factors for mortality in HBV-ACLF patients
To further identify the risk factors for mortality at different time points in HBV-ACLF patients, logistic regression analysis was used to analyse these clinical data. With 28 day mortality as the endpoint, univariate analysis revealed gender, age, INR, PT, MAP, WBC, TBIL, AST, BUN, cholesterol, IL-8, HBV-DNA, HBeAg, CTP, MELD, MELD-Na, and COSSHACLF IIs as risk factors. To avoid model overfitting due to multicollinearity between variables, the variance inflation factor (VIF) was employed [Citation17] (Supplemental Figure 1A). We found that the VIF of INR was in an unacceptable range at this time and removed it from the multivariate regression analysis. Ultimately, we identified gender, MAP, IL-8, HBV-DNA, COSSHACLF IIs as independent risk factors for 28-days mortality in patients ().
Table 2. Univariate and multivariate analysis for 28-day mortality in HBV-ACLF patients.
Similar approaches were used to identify independent risk factors for 90- and 180-day mortality (Supplementary Tables 2 and 3). The VIF of variables was also calculated before inclusion in the multivariate analysis (Supplementary Figure B,C). Notably, serum IL-8 was only an independent risk factor for 28-day mortality but not for 90- and 180-day mortality. MAP, HBV-DNA, COSSHACLF IIs were independent risk factors shared among the three time points.
Table 3. Comparison of patient characteristics in the high IL-8 level and low IL8- level groups.
IL-8 can preliminarily discriminate high-risk populations for 28-day mortality
To further investigate the prognostic value of IL-8, we found the optimal cutoff value for IL8 (log10IL8 = 1.516). Based on the cutoff value, the cohort patients were divided into high IL-8 level and low IL-8 level groups, and their clinical baseline characteristics were further compared (). We found a significant difference in 28-day mortality rate between the two groups, while the high IL-8 level group had higher TBIL, and sCr levels, and poorer liver function as indicated by higher MELD, MELD-Na, and COSSHACLF IIs scores.
Development and validation of nomogram based on IL-8 levels for predicting 28-day mortality
A nomogram was developed based on independent risk factors for 28-day mortality in HBV-ACLF patients (). Calibration curves were used to evaluate the predictive ability of the nomogram model, as shown in , with a high degree of agreement between predicted and observed survival probabilities. The C-index of the nomogram model was 0.901 (95% CI: 0.862–0.940), also suggesting a high accuracy of the model prediction. is a predicted probability plot that intuitively demonstrates the predicted probability of death and true survival for the entire cohort of patients. The confusion matrix is a situation analysis table in the prediction model that summarises the prediction results of the classification model. The accuracy, precision and recall of the nomogram model were 0.855, 0.931 and 0.837, respectively. The confusion matrix was displayed as a 2D planar plot () and a 3D stereoscopic bar plot (), which intuitively displayed the number of classified patients and the real number of patients in the model. Altogether, the above results suggested that the nomogram based on the IL-8 level could predict 28-day mortality of patients with high accuracy.
Comparison of the nomogram model based on IL-8 level and existing liver related prognostic models
First, we compared the AUROCs of the nomogram model and other existing prediction models (scores), including CTP, MELD, MELD-Na, COSSTACLF IIs. As shown in , the accuracy of prediction of the nomogram model was higher than that of the three models used for comparison, and the differences were statistically significant. The receiver operating characteristic curve also illustrated the high accuracy of the predictive ability of the nomogram model (). We further compared the prediction performance of IL-8 alone and the nomogram, and the results showed that the prediction performance of the nomogram was significantly better than that of IL-8 alone.
Figure 4. Area under the receiver operating characteristic curves analysis. (A) AUROC of CTP predicted 28-day mortality vs. AUROC of the nomogram model predicted 28-day mortality. (B) AUROC of MELD predicted 28-day mortality vs. AUROC of the nomogram model predicted 28-day mortality. (C) AUROC of MELD-Na predicted 28-day mortality vs. AUROC of the nomogram model predicted 28-day mortality. (D) AUROC of COSSHACLF IIs predicted 28-day mortality vs. AUROC of the nomogram model predicted 28-day mortality.
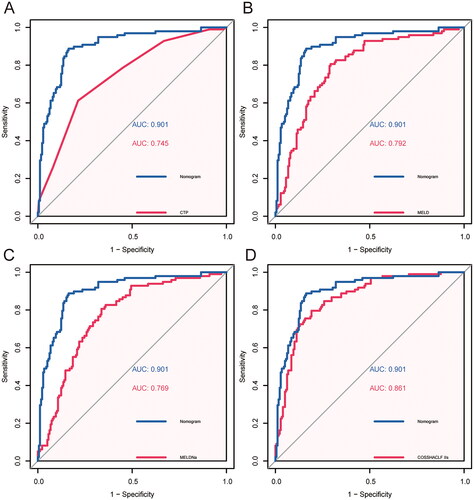
Table 4. Accuracy of different models to predict 28-days mortality in patients with HBV-ACLF.
Clinical utility analysis of the nomogram
DCA is an appropriate method for assessing predictive models by the net benefit and the range of threshold probabilities and it offers advantages over other commonly used measurements and techniques [Citation18]. In predicting 28-day mortality in HBV-ACLF patients, the net benefit of the Nomogram based on IL-8 level model was higher than that of the MELD, MELD-Na and COSSTACLF IIs, which suggests its high value for clinical application ().
Figure 5. Decision curve analysis. (A) DCA curves of CTP and the nomogram model to predict 28-day mortality. (B) DCA curves of MELD and the nomogram model to predict 28-day mortality. (C) DCA curves of MELD-Na and the nomogram model to predict 28-day mortality. (D) DCA curves of COSSHACLF IIs and the nomogram model to predict 28-day mortality.
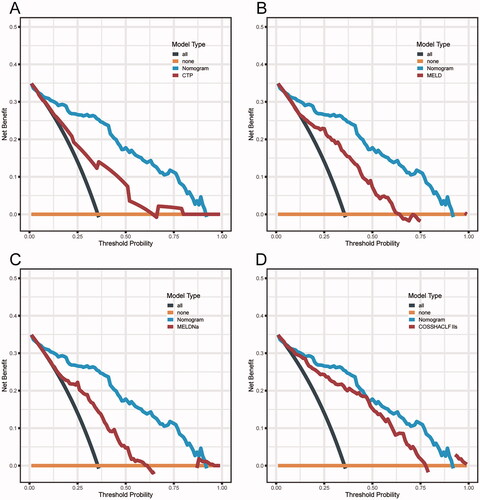
Discussion
The aetiology of ACLF in the Asia-Pacific region is mainly related to hepatitis B virus infection [Citation19], which is different from the disease spectrum of ACLF patients defined by the EASL-CLIF Consortium [Citation4], as their main causes of the disease are mainly alcoholic and hepatitis C. At the same time, the APASL’s standards do not emphasise the onset of liver cirrhosis in the context, and there is currently a gap in the prognosis of this segmented population. In this study, we reported the prognostic value of serum IL-8 levels in HBV-associated-ACLF in the background of cirrhosis. A simple classification based on serum IL-8 levels was able to initially classify ACLF patients into high- and low-risk groups. The nomogram model, based on serum IL-8 levels and common clinical indicators, accurately predicts 28-day mortality but not 90-day and 180-day mortality. Moreover, DCA demonstrated that the developed nomogram model holds promising clinical utility.
The positive criteria defined in the APASL-ACLF Consortium emphasise: a prior diagnosis of chronic liver disease, such as cirrhosis or noncirrhosis, which is a significant point of differentiation from the diagnostic criteria of the EASL-ACLF Consortium [Citation3, Citation4]. To bridge the gap between diagnostic criteria, we examined the prognostic status of cirrhotic patients with ACLF using the APASL-ACLF criteria. With effective medical management, patients with acute decompensated cirrhosis can return to a compensated or even precirrhotic state [Citation20, Citation21]. This presents an urgent need for a time window for the treatment of such patients, and early identification and classification of such patients can greatly improve their survival.
Systemic inflammation has been extensively described in the pathophysiology of ACLF and its ability to contribute to the progression of the disease course of ACLF [Citation6, Citation22, Citation23]. Alberto Zanetto et al. [Citation24] also found that the severity of systemic inflammation was a major predictor of ACLF in patients with acute decompensated liver cirrhosis. In fact, the inflammatory hypothesis suggests that the systemic inflammation of cirrhosis consists of a continuous translocation of bacterial products from the leaky gut to the systemic circulation and the resultant immune imbalance [Citation23]. Unlike the above studies, our study used levels of cytokines secreted by immune cells, such as serum IL-8 levels, to predict patient prognosis, rather than traditional inflammatory markers such as practical C-reactive protein or procalcitonin.
In the pathophysiology of ACLF, excessive inflammation and altered immune status are the main features of the disease [Citation25], and the use of inflammatory factor levels to characterise the prognosis of HBV-ACLF patients in the background of cirrhosis is inferred to be reasonable. Using a similar approach described above, Lehmann et al. [Citation10] found that circulating CXCL10 predicted mortality in patients with ACLF, and Ruiz-Margáin et al. [Citation26] found that fibroblast growth factor 21 served as an early predictor of acute chronic liver failure in critically ill patients with cirrhosis. Although the above inflammatory factor-related biomarkers have been shown to be strongly associated with ACLF, detection of the above biomarkers is still not available in most health care centres.
IL-8 is produced by various types of cells in inflammation and is a chemotactic cytokine for leukocytes [Citation27]. Multivariate logistic regression analysis based on cohort data showed that IL-8 was an independent risk factor for 28-day mortality in patients with HBV-ACLF. Our data suggest that the best cutoff value obtained for serum IL-8 levels at patient admission can be used to initially classify patients, and that patients with high levels of IL-8 have a higher 28-day mortality rate. This may imply a more disturbed immune status and higher levels of inflammation in patients with high mortality, consistent with the fact that basic research has also shown that IL-8 ligands can activate different inflammation-related pathways [Citation27, Citation28].
Interestingly, the COSSHACLF IIs, which developed based on the Chinese cohort, showed better accuracy in predicting mortality within 28 days, with an AUROC of 0.861 in our cohort, such that the results were similar to those currently available [Citation12, Citation29]. Moreover, serum HBV-DNA copy number was also considered an independent predictor of mortality in patients with ACLF. This is in agreement with the pathophysiology of HBV-ACLF patients, due to the fact that HBV reactivation increases the risk of acute exacerbation of liver failure and mortality in cirrhosis [Citation30]. Hemodynamic disturbances are present in patients with ACLF and cirrhosis, and the haemodynamics of patients with ACLF are very similar to those of patients with decompensated cirrhosis [Citation31], which allows MAP to be a risk factor for mortality in patients with ACLF occurring in the cirrhotic setting.
There are potential limitations to this study. First, this is a single-centre retrospective study and there is inevitably bias. However, we performed a complete statistical analysis of this cohort and we strictly adhered to the ranking criterion in order to minimise bias caused by human factors. Second, we were unable to include data from other medical centres for external validation. That is, we were missing data for the validation cohort. To save this limitation, the internal validation of our data suggests that the nomogram model developed based on IL-8 has reliable predictive accuracy. Finally, due to the experimental design, we were not able to record the dynamic changes in IL-8 levels. In the high IL-8 level group, is there a consistency in the changes of IL-8 in those patients who survived and those who died? This change in the dynamics of inflammation in vivo during hospitalisation is worthy of a subsequent study by our team.
In conclusion, we report that serum IL-8 predicts short-term mortality but not medium- and long-term mortality in patients with ACLF in the background of post hepatitis B cirrhosis. The nomogram, constructed using the IL-8 level and common clinical indicators, provides a convenient tool for clinicians to classify patients with varying risks of ACLF by visualising 28-day mortality.
Ethics approval and consent to participate
This study was approved by the Ethics Committee of the First Affiliated Hospital of Nanchang University (Approval IIT-2021-09). Informed consents of the patients were waived by the Ethics Committee of the First Affiliated Hospital of Nanchang University because of the retrospective and anonymous nature of the study.
Author contributions
L.X.L. and P.C. contributed equally to this study. L.X.L. designed and wrote the original draft, P.C. analysed the data and wrote the manuscript, N.X.X. and Q.L. collected the data. X.Z. critically revised the manuscript.
Supplemental Material
Download Zip (830.2 KB)Disclosure statement
No potential conflict of interest was reported by the author(s).
Data availability
The data used to support the findings of this study are available from the corresponding author upon reasonable request.
Additional information
Funding
References
- Moreau R, Gao B, Papp M, et al. Acute-on-chronic liver failure: a distinct clinical syndrome. J Hepatol. 2021;75 Suppl. 1(Suppl. 1):1–11. doi: 10.1016/j.jhep.2020.11.047.
- TRéPO C, Chan HLY, Lok A. Hepatitis B virus infection. Lancet. 2014;384(9959):2053–2063. doi: 10.1016/S0140-6736(14)60220-8.
- Sarin SK, Choudhury A, Sharma MK, et al. Acute-on-chronic liver failure: consensus recommendations of the Asian Pacific Association for the Study of the Liver (APASL): an update. Hepatol Int. 2019;13(4):353–390. doi: 10.1007/s12072-019-09946-3.
- Moreau R, Jalan R, Gines P, et al. Acute-on-chronic liver failure is a distinct syndrome that develops in patients with acute decompensation of cirrhosis. Gastroenterology. 2013;144(7):1426–1437.e9. doi: 10.1053/j.gastro.2013.02.042.
- Gustot T, Fernandez J, Garcia E, et al. Clinical course of acute-on-chronic liver failure syndrome and effects on prognosis. Hepatology. 2015;62(1):243–252. doi: 10.1002/hep.27849.
- Casulleras M, Zhang IW, LóPEZ-Vicario C, et al. Leukocytes, systemic inflammation and immunopathology in acute-on-chronic liver failure. Cells. 2020;9(12):2632. doi: 10.3390/cells9122632.
- Triantafyllou E, Woollard KJ, Mcphail MJW, et al. The role of monocytes and macrophages in acute and acute-on-chronic liver failure. Front Immunol. 2018;9:2948. (doi: 10.3389/fimmu.2018.02948.
- Zhang IW, Curto A, LóPEZ-Vicario C, et al. Mitochondrial dysfunction governs immunometabolism in leukocytes of patients with acute-on-chronic liver failure. J Hepatol. 2022;76(1):93–106. doi: 10.1016/j.jhep.2021.08.009.
- Hernandez-Mijares A, Rocha M, Rovira-Llopis S, et al. Human leukocyte/endothelial cell interactions and mitochondrial dysfunction in type 2 diabetic patients and their association with silent myocardial ischemia. Diabetes Care. 2013;36(6):1695–1702. doi: 10.2337/dc12-1224.
- Lehmann JM, Claus K, Jansen C, et al. Circulating CXCL10 in cirrhotic portal hypertension might reflect systemic inflammation and predict ACLF and mortality. Liver Int. 2018;38(5):875–884. doi: 10.1111/liv.13610.
- Wu Z-B, Zheng Y-B, Wang K, et al. Plasma interleukin-6 level: a potential prognostic indicator of emergent HBV-Associated ACLF. Can J Gastroenterol Hepatol. 2021;2021:5545181–5545187. (doi: 10.1155/2021/5545181.
- Li J, Liang X, You S, et al. Development and validation of a new prognostic score for hepatitis B virus-related acute-on-chronic liver failure. J Hepatol. 2021;75(5):1104–1115. doi: 10.1016/j.jhep.2021.05.026.
- Pugh RN, Murray-Lyon IM, Dawson JL, et al. Transection of the oesophagus for bleeding oesophageal varices. Br J Surg. 1973;60(8):646–649. doi: 10.1002/bjs.1800600817.
- Kamath PS, Wiesner RH, Malinchoc M, et al. A model to predict survival in patients with end-stage liver disease. Hepatology. 2001;33(2):464–470. doi: 10.1053/jhep.2001.22172.
- Kim WR, Biggins SW, Kremers WK, et al. Hyponatremia and mortality among patients on the liver-transplant waiting list. N Engl J Med. 2008;359(10):1018–1026. doi: 10.1056/NEJMoa0801209.
- Liver Failure and Artificial Liver Group CSOID, Chinese Medical Association; Severe Liver Disease and Artificial Liver Group, Chinese Society of Hepatology, Chinese Medical Association. Guideline for diagnosis and treatment of liver failure. Zhonghua Gan Zang Bing Za Zhi. 2019;27(1):18–26.
- Marcoulides KM, Raykov T. Evaluation of variance inflation factors in regression models using latent variable modeling methods. Educ Psychol Meas. 2019;79(5):874–882. doi: 10.1177/0013164418817803.
- Zhang Z, Yang Z, Cheng Q, et al. Establishment and validation of a prognostic model for hepatitis B virus‑related acute-on-chronic liver failure patients with bacterial infection. Hepatol Int. 2022;16(1):38–47. doi: 10.1007/s12072-021-10268-6.
- Jindal A, Sarin SK. Epidemiology of liver failure in Asia-Pacific region. Liver Int. 2022;42(9):2093–2109. doi: 10.1111/liv.15328.
- Ramachandran P, Iredale JP, Fallowfield JA. Resolution of liver fibrosis: basic mechanisms and clinical relevance. Semin Liver Dis. 2015;35(2):119–131. doi: 10.1055/s-0035-1550057.
- Arroyo V, Moreau R, Kamath PS, et al. Acute-on-chronic liver failure in cirrhosis. Nat Rev Dis Primers. 2016;2(1):16041. (doi: 10.1038/nrdp.2016.41.
- Zaccherini G, Aguilar F, Caraceni P, et al. Assessing the role of amino acids in systemic inflammation and organ failure in patients with ACLF. J Hepatol. 2021;74(5):1117–1131. doi: 10.1016/j.jhep.2020.11.035.
- Arroyo V, Angeli P, Moreau R, et al. The systemic inflammation hypothesis: towards a new paradigm of acute decompensation and multiorgan failure in cirrhosis. J Hepatol. 2021;74(3):670–685. doi: 10.1016/j.jhep.2020.11.048.
- Zanetto A, Pelizzaro F, Campello E, et al. Severity of systemic inflammation is the main predictor of ACLF and bleeding in individuals with acutely decompensated cirrhosis. J Hepatol. 2023;78(2):301–311. doi: 10.1016/j.jhep.2022.09.005.
- Arroyo V, Moreau R, Jalan R, et al. Acute-on-chronic liver failure: a new syndrome that will re-classify cirrhosis. J Hepatol. 2015;62(1 Suppl.):S131–S143. doi: 10.1016/j.jhep.2014.11.045.
- Ruiz-MARGáIN A, Pohlmann A, Ryan P, et al. Fibroblast growth factor 21 is an early predictor of acute-on-chronic liver failure in critically ill patients with cirrhosis. Liver Transpl. 2018;24(5):595–605. doi: 10.1002/lt.25041.
- Matsushima K, Yang D, Oppenheim JJ. Interleukin-8: an evolving chemokine. Cytokine. 2022;153:155828. doi: 10.1016/j.cyto.2022.155828.
- Baggiolini M, Clark-Lewis I. Interleukin-8, a chemotactic and inflammatory cytokine. FEBS Lett. 1992;307(1):97–101. doi: 10.1016/0014-5793(92)80909-z.
- Li P, Liang X, Luo J, et al. Predicting the survival benefit of liver transplantation in HBV-related acute-on-chronic liver failure: an observational cohort study. Lancet Reg Health West Pac. 2023;32:100638. doi: 10.1016/j.lanwpc.2022.100638.
- Zhu Y, Li H, Wang X, et al. Hepatitis B virus reactivation increased the risk of developing hepatic failure and mortality in cirrhosis with acute exacerbation. Front Microbiol. 2022;13:910549. (doi: 10.3389/fmicb.2022.910549.
- Kumar A, DAS K, Sharma P, et al. Hemodynamic studies in acute-on-chronic liver failure. Dig Dis Sci. 2009;54(4):869–878. doi: 10.1007/s10620-008-0421-9.