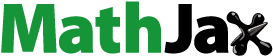
Abstract
Objective
24-hour ambulatory blood pressure monitoring (24ABPM) is state of the art in out-of-office blood pressure (BP) monitoring. Due to discomfort and technical limitations related to cuff-based 24ABPM devices, methods for non-invasive and continuous estimation of BP without the need for a cuff have gained interest. The main aims of the present study were to compare accuracy of a pulse arrival time (PAT) based BP-model and user acceptability of a prototype cuffless multi-sensor device (cuffless device), developed by Aidee Health AS, with a conventional cuff-based oscillometric device (ReferenceBP) during 24ABPM.
Methods
Ninety-five normotensive and hypertensive adults underwent simultaneous 24ABPM with the cuffless device on the chest and a conventional cuff-based oscillometric device on the non-dominant arm. PAT was calculated using the electrocardiogram (ECG) and photoplethysmography (PPG) sensors incorporated in the chest-worn device. The cuffless device recorded continuously, while ReferenceBP measurements were taken every 20 minutes during daytime and every 30 minutes during nighttime. Two-minute PAT-based BP predictions corresponding to the ReferenceBP measurements were compared with ReferenceBP measurements using paired t-tests, bias, and limits of agreement.
Results
Mean (SD) of ReferenceBP compared to PAT-based daytime and nighttime systolic BP (SBP) were 129.7 (13.8) mmHg versus 133.6 (20.9) mmHg and 113.1 (16.5) mmHg versus 131.9 (23.4) mmHg. Ninety-five % limits of agreements were [-26.7, 34.6 mmHg] and [-20.9, 58.4 mmHg] for daytime and nighttime SBP respectively. The cuffless device was reported to be significantly more comfortable and less disturbing than the ReferenceBP device during 24ABPM.
Conclusions
In the present study, we demonstrated that a general PAT-based BP model had unsatisfactory agreement with ambulatory BP during 24ABPM, especially during nighttime. If sufficient accuracy can be achieved, cuffless BP devices have promising potential for clinical assessment of BP due to the opportunities provided by continuous BP measurements during real-life conditions and high user acceptability.
Plain Language Summary
What is the context?
Hypertension is a major risk factor for cardiovascular and cerebrovascular end-organ damage, morbidity, and mortality world-wide.
Accurate measurement of blood pressure is essential for the diagnosis and management of hypertension.
What is new?
Cuffless blood pressure devices that allow measurement of blood pressure without a pressure cuff is a promising and novel method of blood pressure estimation.
The objective of this study is to assess whether pulse arrival time alone can be used to estimate blood pressure accurately during 24-hour ambulatory blood pressure monitoring, using a prototype cuffless device placed on the chest.
Our analysis shows that a general model based on pulse arrival time overestimated ambulatory blood pressure, especially during nighttime.
User acceptability was higher with the cuffless device compared to a conventional cuff-based oscillometric device during 24-hour ambulatory blood pressure monitoring.
What is the impact?
This study provides further evidence that accurate blood pressure estimations cannot be achieved by using pulse arrival time alone as a surrogate for blood pressure measurements.
Introduction
Accurate measurement of blood pressure (BP) is essential for the diagnosis and management of hypertension. Office BP has been used in every large outcome trial that has investigated the effects of lowering BP on cardiovascular outcomes, and thus remains the gold standard. However, office BP is unable to detect clinically important hypertension phenotypes such as white coat hypertension, masked hypertension, and nighttime hypertension. 24-hour ambulatory BP monitoring (24ABPM) has been shown to better predict hypertension-mediated organ damage, cardiovascular events and death compared to office BP, and is therefore considered to be the state-of-the-art method for out of office BP measurements (Citation1, Citation2). Standard ambulatory upper arm cuff-based oscillometric BP measurements have several limitations. The intermittent measurements from cuff devices only provide BP information during a certain, short time window, thereby ignoring BP fluctuations during real-life conditions. Furthermore, the cuff inflation itself may cause discomfort that can lead to both errors in measurements and falsely elevated BP (Citation2). Also, incorrect cuff-size can lead to over- or underestimation of BP (Citation3, Citation4), which is common in very thin and very obese patients (Citation3). Finally, repeated 24ABPM investigations are limited by patients’ high discomfort factor of cuff inflation, disturbed sleep and restriction of daily activities related to ambulatory BP monitoring (ABPM) (Citation5–7).
Several efforts have been made in recent years to develop wearable cuffless devices that track changes in BP after initial calibration with a cuff measurement (Citation8–14). In these devices, BP is predicted by measuring other physiological variables that are related to changes in BP and using algorithms, machine learning models or other data-driven techniques to convert these measurements to BP. Cuffless BP devices differ in several aspects (Citation15–17). First, they use different principles and technologies for estimating changes in BP. Both the physiological variable and the sensors used to measure these variables vary between devices. Second, they differ in anatomical sensor location, e.g. wrist (Citation18), chest (Citation19), ear (Citation14) and finger (Citation20). Finally, they have different intended use (continuous vs. intermittent) and are implemented in different types of devices, e.g., smartwatch, smartphones, dedicated devices. Most cuffless BP devices are based on pulse wave analysis (PWA) of a photoplethysmography (PPG) signal, pulse arrival time (PAT) or a combination of the two (Citation9–14, Citation20–23). Even though there has been an increasing amount of cuffless BP devices that claim to accurately estimate BP available on the market, none of these devices have yet been validated according to the recently released validation protocol for cuffless devices, ISO 81060-3:2022 standard, nor according to the European Society of Hypertension recommendations for the validation of cuffless blood pressure measuring devices (Citation24).
The present study is a pilot investigation of using PAT as a potential surrogate BP measurement to enable non-invasive cuffless 24ABPM, using a prototype cuffless multi-sensor device (cuffless device) placed on the chest. The study had three main aims. First, we aimed to compare BP predictions of the PAT-based BP model, derived from a general population cohort (Citation25), with the measurements of a conventional cuff-based oscillometric BP device (ReferenceBP) during 24ABPM in subjects with and without hypertension. Second, we aimed to compare the user acceptability of the cuffless device with the ReferenceBP device. Third, we aimed to test feasibility of the cuffless device to predict sleep times using a machine learning method based on self-reported sleep data.
Materials and Methods
Study Participants
We recruited 153 adults from Oslo University Hospital, Ullevål (n= 111) and from a general practitioner office, Sandefjord Helsepark (n= 42). At Oslo University Hospital, the subjects consisted of healthy volunteers, employees and patients registered in a local hypertension registry (HyReBi, REK 2017/477). At Sandefjord Helsepark, patients with an indication for 24ABPM were included. Inclusion criteria were age > 18 years and a written informed consent. Exclusion criteria were a history of cardiac arrhythmia, pregnancy, possible extreme uncontrolled hypertension (resting office BP ≥ 220/120 mmHg), interarm BP difference > 10 mmHg, age > 70 years, known cardiovascular or cerebrovascular disease, pulmonary disease, renal disease, or Diabetes Mellitus type I or II, or inability to measure BP on one or both arms. In addition, as we did not have interpreters, we excluded participants without sufficient Norwegian language skills to understand information, instructions, or questionnaires presented in Norwegian. Thus, the study aimed to include individuals that were either healthy or with uncomplicated hypertension. Participants recruited from the local hypertension registry were screened by examining the registry information in addition to their own knowledge of any new onset excluding disease history. Other participants were screened by their own knowledge of any excluding disease history. The study was approved by the Regional committee for medical and health research ethics (REK), project number 65844, prior to inclusion of the first study subject.
ABPM Measurements
ReferenceBP measurements were performed using a conventional ambulatory oscillometric BP device (Oscar 2, SunTech Medical, Morrisville, North Carolina, USA) (Citation26). The same ReferenceBP device was used at both centers. A detailed description of the prototype cuffless device, developed by Aidee Health AS, has been given previously (Citation25, Citation27, Citation28). In short, it is a wearable chest belt that measures electrophysiological and optical signals in form of an electrocardiogram (ECG) and a PPG and has an inertial measurement unit (IMU) consisting of a 3D accelerometer and a 3D gyroscope. A visualization of the prototype cuffless multi-sensor device is presented in . Cuffless BP predictions were obtained by using PAT calculated from the ECG and PPG signals. The model to obtain BP values from PAT estimations was developed from a different dataset, considered a general population cohort, during isometric exercise induced BP changes (Citation25). The model is a simple best-fit linear equation with an intercept term and a coefficient to determine either systolic BP (SBP) or diastolic BP (DBP) from measured PAT.
Both devices were mounted with the participants sitting in an upright position with back support and legs uncrossed. The dominant arm was fitted with an appropriately sized cuff. After 5 minutes of seated rest, inclusion BP measurements (office BPs) were taken on both arms starting with the dominant arm using the ReferenceBP device. Three consecutive measurements were taken with 1-minute intervals between measurements. If the difference between any of the first three BP measurements obtained on the dominant arm were > 7mmHg, two more measurements were taken. The first measurement taken was discarded and office BP calculated as the average of the remaining measurements. The process was then repeated on the non-dominant arm. Inter arm BP differences were calculated as the difference between the office BP on each arm.
The ReferenceBP was programmed for measurements every 20 minutes from 07:00 to 23:00 and every 30 minutes in the remaining period. The cuffless device measured signals continuously with 1000 Hz resolution. The PAT-based BP model was calibrated once using the inclusion brachial BP measurement (office BP) obtained by the ReferenceBP device on the non-dominant arm. Participants’ demographics were not used for additional calibration. To be able to compare measurements from the two devices, only PAT-based BP predictions corresponding to the timing of the cuff measurements were used. PAT-based BP predictions were estimated in 2-minute windows corresponding to a reference measurement. Individual self-reported diaries were used to define awake and asleep BP values. If a participants sleep diary was missing, the default sleep window of 23:00-07:00 was used, and the participant was excluded from the sleep analyses. The participants were instructed to engage in normal activities but stop any activity and keep the arm still at heart level during measurements and refrain from strenuous exercise, long car drives and taking the equipment off. Furthermore, participants were informed to stop moving and keep the arm still with the cuff at heart level when the cuff inflated.
A valid participant 24ABPM was defined as having at least 40% good measurements pairs where a good reference pair was defined as at least 40% valid data from the cuffless device within the 2-minute cuff measurement window. Valid sensor data was based on PPG and ECG quality criteria. Participants who did not meet these criteria were excluded. Furthermore, participants with less than 25 valid 24ABPM measurements and no valid night-time measurements were removed. Outliers predicted by the PAT-based algorithm were filtered in the following way: Within each participant extreme BP values defined as SBP above 280 mmHg or below 50 mmHg and/or DBP above 150 or below 30 mmHg. These values were considered non-physiological BP values.
Questionnaire
A self-developed questionnaire was used to evaluate the level of comfort, discomfort, activity, and sleep disturbance of both devices. Parts of the questionnaire were based on questions published in the “Device assessment form” from the British Hypertension Society BP device validation protocol (Citation29). These questions were translated into Norwegian and transformed to a 7-point Likert scale. In addition, we assessed discomfort and pain with a verbal numeric rating scale (VNRS) (Citation30, Citation31) and developed questions tailored to the chest belt design of the cuffless device.
Statistical Analysis
Data processing was performed offline using the Python programming language. Data processing included processing and filtering of the raw signals from the cuffless device and we implemented algorithms to classify signals from a heart cycle as valid or non-valid. Statistical analyses were performed using Stata v. 17.0 (Statacorp., Texas, USA). Variables were assessed for normality by visual inspection of histograms. Continuous data are presented as means (SD), or median (interquartile range (IQR) if non-normally distributed). Mean BP were normally distributed and compared using paired t-tests. The level of absolute agreement between the ReferenceBP and the PAT-based BP model for daytime, nighttime, and 24-hour SBP and DBP were evaluated using Bland-Altman plots with bias and 95% limits of agreement (LOA). Correlation analysis was performed using repeated measures correlation as proposed by Bland and Altman (Citation32). Within participant change in BP was calculated by taking the highest reported ReferenceBP measurement minus the lowest reported ReferenceBP measurement during 24ABPM. We performed separate subgroup analyses stratifying for hypertension. In these subgroups, mean of reference compared to predicted BP was calculated as well as repeated measures correlation coefficients. Hypertension strata and sex category interaction on accuracy of the PAT-based model was investigated both in a linear regression model with mean BPs and in a panel regression model to account for within participant repeated measures. Diagnostic accuracy of the cuffless device in detecting hypertension was investigated by computing 2 x 2 contingency tables to calculate sensitivity and specificity. ReferenceBP was considered the gold standard test and diagnostic thresholds were based on the European hypertension guidelines (Citation33).
Because of the inability of the PAT-based BP model to estimate nighttime BP dip, we investigated hourly changes of PAT during 24-hours and tested (post hoc) whether changes in the PPG waveform during ambulation could alter the PAT calculations. During data inspection we observed some variation of this slope during ambulation. We assumed that the steepness of the slope of the first upstroke of the PPG waveform could introduce errors in absolute PAT calculations. A steeper slope could cause PAT to be longer, corresponding to a lower BP prediction, and vice versa. We therefore hypothesized that a less steep slope during nighttime could cause an erroneous shortening of PAT calculations during nighttime. Average numeric values of the slope of the first upstroke of the PPG signal were calculated, i.e. the 1st derivative of the PPG waveform, in the same 2-minute windows as all BP measurements. Next, hourly averages were calculated and tested for changes over time using a one-way ANOVA repeated measures test. The slope values were log-transformed to achieve a normal distribution. A two-sided p value < 0.05 was considered statistically significant.
Sleep Analyses
Sleep features
The accelerometer-based features Euclidean Norm Minus One (ENMO) and Locomotion Inactivity During Sleep (LIDS) were calculated as described by Sundararajan and colleagues (Citation32). In addition, three new accelerometer and gyroscope-based features were defined: ChangeGravity, AccumRot and SleepPosture. ChangeGravity was defined as the angle between average accelerometer vectors in two successive epochs. This is an indication of posture change between two epochs. AccumRot was defined as the root-sum-square rotation about all three gyroscope axes. This serves as an indicator of overall rotational movement of the truncus within an epoch. Lastly, SleepPosture was defined as 1 if pose was classified as laying prone, supine or on the side. Heart rate (HR) and HR variability features were calculated from the ECG-signal after normalization of the RR-intervals, as described by Aktaruzzaman et al (Citation33). Time-domain features were mean RR interval (meanRR) and standard deviation of RR-intervals (SDNN). Frequency-domain features were the low-frequency to high-frequency ratio (LF/HF).
Sleep wake classification
An automated sleep/wake classification algorithm was trained using the participants’ self-reported sleep diaries as a reference. The algorithm was a decision tree classifier which used features derived from IMU data and R peak to R peak measurements from the ECG to derive HR frequency and HR variability measurements. The features were calculated over 5-minute epochs and are described in paragraph above. Optimal decision tree depth was determined by evaluating depths from 1 to 25 using leave-one-out cross validation of 10 subjects. Finally, decision tree classifiers with optimal depth were trained for each subject using leave-one-out cross validation. This process was repeated for all participants, so that each model was tested on a single participant that was excluded from the training data. This method entails the development of a unique model for each individual participant, utilizing data from all the remaining participants in the dataset. Thus, the method provides a robust assessment of the model’s performance and generalizability across the entire participant cohort. Classifier performance was evaluated by overall accuracy score (fraction of correctly classified epochs) and Cohen’s kappa (Citation35).
Sleep window determination and sleep efficiency
Sleep window was determined by finding the continuous time window that maximized sleep epochs and minimized wake epochs. Specifically, setting s = 1 in epochs classified as sleep and s = -1 in epochs defined as wake, the time points for sleep window onset (slept) and offset (woke) was determined by the following equation:
Sleep efficiency was defined as the fraction of epochs classified as sleep during the time spent in bed. Time spent in bed was defined from the last epoch with a non-sleep pose directly prior to onset of the sleep-time window, until the first epoch of non-sleep pose after the end of the sleep-time window.
Results
Participant Selection, General Characteristics and Blood Pressures
A flow chart of participant inclusion is presented in . Twenty-one observations were defined as outliers predicted by the PAT-based algorithm and removed. A total of 4010 measurement pairs were included in the analyses. All participants reported sleep times. Eight participants did not report sleep quality and was excluded from those parts of the sleep analyses. General characteristics are presented in . Median (IQR) age was 49.0 (39.0 – 61.0) years, 42.1% were female and approximately half of the included subjects had a previous history of hypertension (47.4%). Baseline mean (SD) SBP and DBP, defined as the average of the office BP measurements on the non-dominant arm excluding the first measurement, were 127.0 (15.2) mmHg and 78.4 (10.6) mmHg. Comparisons between ReferenceBP and PAT-based mean 24-hour, daytime and nighttime BP showed that the PAT-based model overestimated BP (). Mean (SD) 24-hour ReferenceBP SBP was 125.4 (14.8) mmHg compared to the PAT-based SBP of 133.3 (21.1) mmHg (p <0.001) and mean nighttime ReferenceBP SBP was 113.1 (16.5) mmHg compared to the PAT-based SBP of 131.9 (23.4) mmHg (p <0.001). The results were similar regarding DBP and in the subgroups of participants with or without a prior history of hypertension (). To exemplify more result details, we included time series plots from a few selected participants in . The plots defined as mediocre agreement (Panel A, B and C) were considered to be among the best and those defined as poor agreement (D, E and F) were considered representative of the majority of participants.
Figure 3. Time series plots from six different participants of systolic blood pressure (SBP) and diastolic blood pressure (DBP) from the cuff-based oscillometric device (ReferenceBP) and PAT-based blood pressure (BP) model (Predicted BP). Three subjects with mediocre agreement (A + B + C) and three subjects with poor agreement (D + E + F).
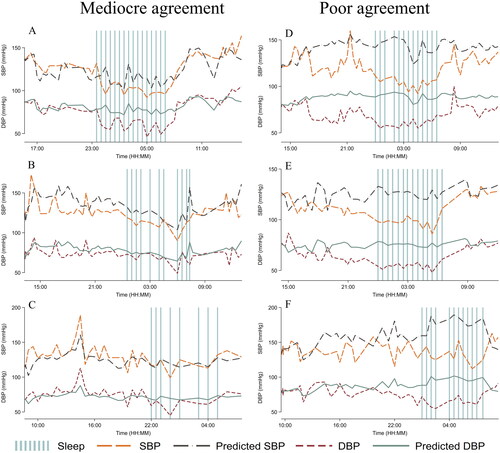
Table 1. General characteristics of included participants.
Table 2. Comparison between conventional cuff-based oscillometric device (ReferenceBP) measurements and PAT-based blood pressure measurements. SBP, systolic blood pressure. DBP, diastolic blood pressure.
Agreement
Agreement between the PAT-based BP model and ReferenceBP is presented with Bland Altman plots with bias and 95% LOA (). Regarding SBP, bias [LOA] were 7.9 mmHg [-23.3, 39.2 mmHg], 3.9 mmHg [-26.7, 34.6 mmHg] and 18.8 mmHg [-20.9, 58.4 mmHg] for 24-hour, daytime, and nighttime respectively. Corresponding results for DBP were 6.9 mmHg [-10.8, 24.5 mmHg], 3.3 mmHg [-14.3, 20.9 mmHg] and 16.7 mmHg [-6.1, 39.5 mmHg]. Repeated measures correlation coefficients were 0.06 (p < 0.001) and -0.01 (p = 0.509), demonstrating a weak but statistically significant correlation regarding 24-hour SBP but no correlation regarding 24-hour DBP. Repeated measures correlation coefficients for daytime and nighttime SBP were 0.06 (p = 0.003) and 0.029 (p = 0.388). Corresponding results for DBP were -0.030 (p = 0.111) and -0.075 (p = 0.025). The results were similar when stratifying for hypertension and sex category. There was no interaction regarding hypertension strata in a linear regression model on aggregated mean 24-hour BPs (p = 0.16 for SBP and p = 0.25 for DBP), and no interaction in a panel regression model (p = 0.81 for SBP and p = 0.68 for DBP). There was also no interaction regarding sex category in a liner regression model (p = 0.0.45 for SBP and p = 0.074 for DBP) and no interaction in a panel regression model (p = 0.1 for SBP and p = 0.88 for DBP).
Figure 4. Bland-Altman plots. Mean of measurements from the cuff-based oscillometric device (ReferenceBP) and PAT-based blood pressure (BP) model (x-axis) plotted against the difference between ReferenceBP and PAT-based BP model (y-axis). Horizontal lines indicate bias and upper and lower 95% limits of agreement. Systolic blood pressure (SBP; left panels) and diastolic blood pressure (DBP; right panels) for the entire 24-hour measurement period (top panels), daytime measurements (mid panels) and nighttime measurements (bottom panels).
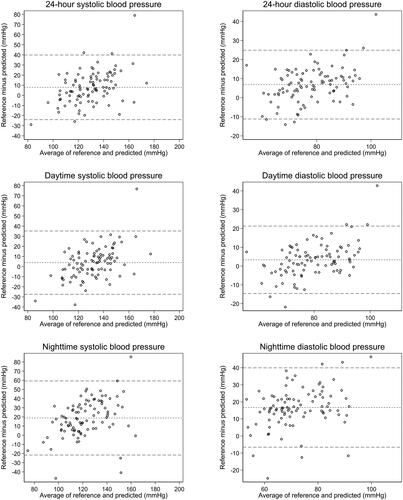
Diagnostic Accuracy
The sensitivity and specificity of the PAT-based BP model compared to ReferenceBP are presented in . Because of the tendency to overestimate BP, sensitivity was good regarding the PAT-based BP model. However, specificity was poor, only 44.9% and 23.3% for SBP and DBP during nighttime respectively.
Table 3. Sensitivity and specificity of the PAT-based blood pressure (BP) model compared to conventional cuff-based oscillometric device (ReferenceBP) as the gold standard.
Ambulatory Changes in Pulse Arrival Time
Hourly averages of PAT showed an opposite ambulatory variation compared to expected (). Contrary to our hypothesis that the slope would be less steep during nighttime and steeper during daytime, potentially confounding the PAT calculations, our analyses showed the opposite (). There was a statistically significant hourly variation in slope (p < 0.001), as calculated by ANOVA repeated measures.
User Acceptability
Ninety-two (97%) of the 95 participants answered the questionnaire. Results are presented in . Questions related to comfort during 24ABPM of the cuffless device yielded high acceptance ratings with 58 (63%) agreeing or strongly agreeing versus only 4 (4%) for the ReferenceBP device. Response regarding disturbance of both daily routine and sleep also showed the cuffless device superior to the ReferenceBP device, 83 (90%) versus 17 (18%) subjects and 76 (83%) versus 15 (16%) responding with strongly disagree/disagree to being disturbed. Furthermore, the cuffless multi-sensor device had a higher rating for long term adherence with 85 (92%) of the study participants answering that they would choose cuffless multi-sensor device as the monitoring method for their next 24ABPM.
Sleep Detection
Eighty-seven (92%) of the 95 participants answered the sleep diary. The decision tree classifier accuracy was 91 ± 5% and Cohen’s kappa = 0.77 ± 0.13, of which 1 is perfect agreement and 0 the expected agreement due to chance alone. Reported versus predicted sleep windows for all participants are presented visually in . The distribution of deviations between predicted sleep window and diary sleep window is presented as boxplots in . There was a median 10-minute overestimation of predicted sleep window compared to the sleep diaries, with the 25th and 75th percentile ranging from -32 to 43 minutes (, “SW duration”). The sleep window onset was more challenging to detect than the end of the sleep window (IQR 67 minutes vs 28 minutes, respectively, “SW onset” and "SW end" in ). Sleep efficiency (fraction of epochs classified as sleep during the time spent in bed) was on average 85 ± 12% and was unrelated to sleep quality in the diaries ().
Figure 7. (A) Reported (i.e. diary, black lines) versus predicted (gray lines) sleep windows (SW) for each participant. Numbering on horizontal axis represent participants. (B) Distribution of deviations between predicted SW and reported SW. The boxplots show interquartile range (box height) and median deviation (gray lines). “SW onset” and "SW end” indicates deviation between predicted and reported sleep window onset and end, respectively. “SW duration” indicate the deviation between predicted and reported sleep window duration. (C) Sleep efficiency (i.e. the fraction of time in bed classified as sleep) for the three self-reported sleep quality categories (“good”, “medium” and “poor”). “NA” indicates no response from the participant (N = 8 occasions).
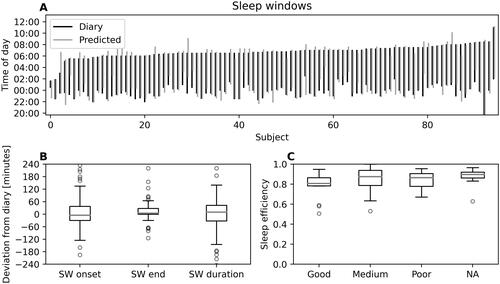
Discussion
Our findings together with previously published studies suggests that PAT alone is not sufficient as a cuffless surrogate measurement to predict BP measurements accurately in 24ABPM. The PAT-based algorithm used to estimate BP, which was measured with a prototype cuffless device and derived from general population cohort, showed unsatisfactory agreement for 24-hour ambulatory BP, with the largest discrepancy observed during nighttime. However, user acceptability was higher with the cuffless device compared to the ReferenceBP device and it showed promising results towards automatic sleep detection as an additional feature.
Our BP results are comparable to most similar studies that have investigated accuracy in a cuffless BP device compared to conventional cuff BP in adults for 24ABPM (Citation11, Citation22, Citation36–40). Nyvad et al investigated a PAT-based device in 51 adults with essential hypertension and found that BP was generally overestimated with the largest discrepancies observed during nighttime (Citation36). Compared to our subgroup of 45 patients with a hypertension diagnosis, nighttime SBP bias was smaller (21.6 mmHg compared to 11.6 mmHg) and LOA similar (± 48.0 mmHg compared to ± 47.1 mmHg). In our subgroup without a hypertension diagnosis, nighttime bias regarding SBP was 16.2 mmHg with LOA of ± 30 mmHg. Similar results were reported in other studies investigating 24ABPM accuracy of the same PAT-based device as Nyvad et al (Citation22, Citation37). Tan et al investigated a PWA-based cuffless wrist band in 41 normotensive and hypertensive adults and also found that particularly nighttime BP was overestimated (Citation39). Nighttime SBP bias and LOA were 15.3 [-8.7, 39.3] mmHg. In contrast, Proença et al found good agreement between a cuffless wrist or upper arm worn device based on PWA of the PPG signal and conventional cuff measurements in 67 adults including healthy and hypertensives (Citation23). Their daytime and nighttime SBP bias and LOA were -1.5 [-14.4, 11.4] mmHg and 0.4 [-14.4, 15.1] mmHg. Nachman et al investigated PWA of the PPG signal in combination with pulse wave transit times (PAT or pulse transit time not clearly specified) incorporated in a watch-like device in 28 participants both healthy and with stable chronic disease (Citation8). This study is the only one to date to report high precision and accuracy with SBP LOA of [-6.9, 3.3 mmHg] and [-1.7, 2.6 mmHg] during daytime and nighttime respectively. All the cuffless devices in these studies were calibrated once when mounted using a brachial BP measurement.
The present study is the only one to date to report correlation coefficients that account for repeated measures within individuals. An important factor when evaluating accuracy of cuffless BP estimations is the degree of BP change within each individual after this initial calibration. A small intraindividual change in BP during data collection will result in a small bias and narrow LOA. Our participants had high within-subject variations in BP with mean SBP (SD) 55.6 (12.8) (). Within-subject variation in BP was not described in detail in the above-mentioned studies, but since they all describe standard 24ABPM settings we assume that within-subject variation is similar to our results, and thus, if the predicted BP values were accurate, high repeated measures correlation coefficients can be expected. In the present study, correlations corrected for repeated measurements within individuals showed no correlation for SBP or DBP. Other studies reported correlations of mean predicted BP vs mean ReferenceBP for each individual. In this way, predicted BP versus ReferenceBP show good correlation because of calibration at start of the measurement period and subsequently because there is a range in mean BP between individuals. We hypothesize that other studies that show discrepancies between reference and predicted BP that are similar to ours, also have poor repeated measures correlation. Even though the generalized PAT-based BP model did not achieve high accuracy results overall, the model was able to predict ambulatory BP changes in selected participants as displayed in .
A concerning finding in our results, as well as in most previous studies evaluating cuffless devices compared to conventional cuff-based 24ABPM, except from the study by Proença et al and Kachel et al, was the inability of the PAT-based BP model to predict nighttime BP (Citation8, Citation22, Citation36, Citation39, Citation40). As shown above, agreements tended to be poorer for nighttime averages compared to daytime. A cuffless device utilizing the radial artery tonometry method also failed to detect nighttime dipping (Citation38). Other PWA-devices, such as smart watches, showed a systematic bias toward a calibration point, overestimating low BPs and underestimating high BPs, in 40 normotensive and hypertensive subjects (Citation11). Combining PWA and pulse wave transit time analyses seems to manage to overcome these difficulties and accurately predict nighttime BP (Citation8). However, the findings from Kachel et al are yet to be reproduced in a manufacturer-independent clinical research study.
Our post-hoc analyses of ambulatory changes in the PPG signal did not reveal any possible explanatory mechanism towards our unexpected results (). Contrary to our hypothesis that a less steep slope during nighttime could cause shorter PAT calculations, we found that the slope steepness increased from daytime to nighttime. Thus, we interpret the slope of the PPG signal to not affect the PAT calculations. The pre-ejection period (PEP) is a known potential confounder. PAT includes PEP, defined as the electromechanical delay from the electrical R wave to the actual onset of the pulse wave when the aortic valve opens (Citation41). PEP is repeatedly shown to lengthen during sleep (Citation42). Thus, PEP cannot explain the observed shortening of PAT during sleep in our study. The shortcomings of PAT as cuffless surrogate measurement remain incompletely understood. Both PAT-based and PWA-based devices have been shown to accurately predict BP in subjects under controlled conditions in the laboratory. Sola et al demonstrated accurate BP predictions in the most common body positions of daily life of a PWA based bracelet compared to auscultation in 91 subjects (Citation18) and Bilo et al showed similar results in 33 adults in the sitting position using a PAT-based device (Citation43). There are, however, many potential sources of error. It is well known that the contact pressure of the PPG sensor markedly alters the PPG waveform amplitude (Citation44, Citation45). This issues a clinically relevant problem since sensor movement can result in altered PPG wave amplitude and shape due to changes in contact pressure and thereby alter the PAT measurement (Citation46). The theoretical relationship between changes in PAT and BP is based on the Moens-Korteweg equation, in which changes in pulse wave propagation times arise from pressure induced changes in vessel wall stiffness if diameter and wall thickness are held relatively constant (Citation47). Although this may be true in central elastic arteries, wall thickness and vessel diameter changes frequently in the peripheral circulation due to both systemic autonomic signals and local autoregulatory blood flow demands. HR is also suggested to affect pulse wave propagation times. Increased HR is shown to cause increased wave propagations independently of BP (Citation48, Citation49). We believe these issues make PAT-based BP estimations extremely challenging or even impossible.
Previous studies have found 24ABPM to cause significant discomfort and sleep disturbances (Citation50, Citation51). This can lead to both errors in measurements and falsely elevated BP (Citation52, Citation53), and poor acceptance for repeated 24ABPM due to discomfort has been shown (Citation2). Our questionnaire results showed that the cuffless device was more comfortable for participants and less disturbing during both daily activities and sleep compared to the ReferenceBP device during 24ABPM. The cuffless device showed promising results in limiting the impact of discomfort associated with 24ABPM measurements, especially during sleep. These findings agree with previous studies evaluating user acceptability of cuffless BP devices (Citation8, Citation14, Citation36, Citation54, Citation55). The 28 participants in the study by Nachmann et al (Citation8) reported the wrist monitor significantly more comfortable, less disturbing and had a significantly higher rating for long-term adherence. Similar results were found by Nyvad et al (Citation36), Zeng et al (Citation55) and by McGillon et al (Citation14).
As an additional feature we investigated the ability of the device to predict sleep times. To the best of our knowledge, this is the first study to investigate this as an additional feature in a wearable cuffless BP device. Predicted sleep times from the model trained on one part of the dataaset showed high accuracy. Sleep time is important to correctly distinguish between daytime and nighttime BP values. However, in clincal practise default sleep time between 23 and 07 is often used regardless of the patients actual sleep time. Although diaries to adjust for acutal sleep and awake times are recommended, they are often only utilized in 24ABPM in research settings. Thus, automated features could improve sleep and awake classifications.
Strengths and Limitations
A strength of the present study is the 24-hour recording time under routine clinical conditions. This allows for an interpretation of clinical utility and feasibility. Our study provides, to the best of our knowledge, the highest number of study participants to date with 95 individuals included in the analysis and a data set with a total of 4010 BP measurement pairs. The ability to automatically detect sleep window by measuring movement, HR and HRV is an advantage of the cuffless device compared to the ReferenceBP device. Furthermore, we evaluated both healthy individuals and individuals with a prior hypertension diagnosis (47.4%). This is important because any novel method must show satisfactory accuracy in both groups. The gender distribution was relatively equal (42.1% females).
The study has several limitations. First, as with other conventional cuff-based 24ABPM devices, our ReferenceBP device (Oscar 2, SunTech Medical, Morrisville, North Carolina, USA) is only validated according to ISO 81060-2:2013 standard in the seated position, and with stable, relaxed conditions. Accordingly, this and other cuff-based BP devices are not validated in an ambulatory setting. Therefore, the accuracy of these cuff-based BP devices during 24ABPM is unknown, still, they are currently accepted as the best ambulatory method to collect BP readings and are found to be part of the standard of care for hypertensive patients. Second, we excluded many individuals (38%) and cannot rule out selection bias. However, the majority were related to quality criteria for minimum acceptable good measurement pairs within an individual and reflect issues mainly related to signal quality of the prototype cuffless device. The signals from the prototype chest belt had unforeseeable amounts of noise in combination with loss of ECG electrode contact during free ambulatory living. The reason for this seemed to be issues with the flexible belt, such as stiff material, which later has been improved in addition to loss of signal from the first generation of ECG electrodes which were changed. Originally, the quality criteria regarding a valid participant 24ABPM were defined a priori to be at least 70% good measurement pairs, of which “a good pair” was defined as at least 70% valid data within the 2-minute cuff measurement window. However, as these criteria only yielded seven valid participants, the criteria were modified. We did not include very old individuals or very obese individuals. Furthermore, we used the ReferenceBP device as the calibration measurement for the PAT-based BP model. The findings regarding PAT as a cuffless surrogate measurement must be interpreted in light of PAT being measured at chest level, which could differ from PAT measured at different anatomical locations or by other signals.
There are also important methodological considerations regarding the questionnaire to evaluate the user acceptability and the sleep analyses. A limitation was the lack of validation of the survey. Additionally, evaluating the two methods together may affect the user evaluation (Citation56, Citation57). This means that the user evaluates each of the products in relation to each other to a greater extent than evaluating the individual method. If the participant perceives belt monitoring as more positive than cuff monitoring, there is thus a possibility that belt monitoring is given a higher score, or gives the cuff a lower score, than if they had been evaluated separately. Finally, as measurements were carried out with both devices mounted at the same time, we cannot conclude that patient sleep would be significantly better if measurements were carried out only with the chest belt. The sleep/wake classifier was trained using self-reported sleep diaries, which is a limitation. The sleep diaries did not include nighttime wake-periods nor daytime sleep-periods. It is likely that the sleep/wake classifier can be improved if it is trained on objective reference measurements of sleep/wake-state.
Conclusion
In the present study, we demonstrated that a general PAT-based BP model measured by a prototype cuffless multi-sensor device on the chest significantly overestimated 24-hour and nighttime ambulatory BP in normotensive individuals and individuals with a prior hypertension diagnosis compared to conventional cuff measurements. We interpret this as a need for more data to develop robust and more complex models that can accurately estimate BP across differing postures and daily activities. The prototype multi-sensor device was reported to be more comfortable and less disturbing during daily activities and sleep compared to the reference device. Furthermore, the prototype device showed promising results in automatic sleep detection. If sufficient accuracy can be achieved, cuffless BP devices have promising potential for clinical assessment of BP due to high user acceptability and the ability to estimate BP continuously during real-life conditions in relation to context data, rather than providing mere snapshots of the dynamic BP profile.
Author Contributions
SH, TMS, ESB, FEMFE and BWG contributed to conception and design of the study. ESB designed the questionnaire. SH, HJG and KN performed the data collection. SH, KGBR, AS, ØG and ESB organized the database. SH and in part CH performed the data analysis and statistical analysis. CH and SH wrote the first draft of the manuscript. ØG and ESB wrote sections of the manuscript. All authors contributed to manuscript revision, read, and approved the submitted version.
Conflict of Interest
TMS, KGBR and AS are employees of the company behind the prototype cuffless multi-sensor device. The remaining authors have no conflicts of interest to declare.
Data Availability Statement
BP predictions from the PAT-based model and the reference measurements can be made available upon a reasonable request. Raw signals and data regarding model development may not be disclosed.
Additional information
Funding
References
- Williams B, Mancia G, Spiering W, Agabiti Rosei E, Azizi M, Burnier M, et al. 2018 ESC/ESH Guidelines for the management of arterial hypertension. Eur Heart J. 2018;39(33):1–16. doi:10.1093/eurheartj/ehy339.
- O’Brien E, Parati G, Stergiou G, Asmar R, Beilin L, Bilo G, et al. European Society of Hypertension position paper on ambulatory blood pressure monitoring. J Hypertens. 2013;31(9):1731–68. doi:10.1097/HJH.0b013e328363e964.
- Olsen MH, Angell SY, Asma S, Boutouyrie P, Burger D, Chirinos JA, et al. A call to action and a lifecourse strategy to address the global burden of raised blood pressure on current and future generations: the Lancet Commission on hypertension. Lancet. 2016;388(10060):2665–712. doi:10.1016/S0140-6736(16)31134-5.
- Palatini P, Benetti E, Fania C, Malipiero G, Saladini F. Rectangular cuffs may overestimate blood pressure in individuals with large conical arms. J Hypertens. 2012;30(3):530–6. doi:10.1097/HJH.0b013e32834f98a0.
- Mengden T, Weisser B. Monitoring of Treatment for Arterial Hypertension–The Role of Office, Home, and 24 h Ambulatory Blood Pressure Measurement. Dtsch Arztebl Int. 2021;118(27-28):473–8.
- Nasothimiou EG, Karpettas N, Dafni MG, Stergiou GS. Patients’ preference for ambulatory versus home blood pressure monitoring. J Hum Hypertens. 2014;28(4):224–9. doi:10.1038/jhh.2013.104.
- Ringrose JS, Bapuji R, Coutinho W, Mouhammed O, Bridgland L, Carpenter T, et al. Patient perceptions of ambulatory blood pressure monitoring testing, tolerability, accessibility, and expense. J Clin Hypertens (Greenwich). 2020;22(1):16–20. doi:10.1111/jch.13760.
- Nachman D, Gilan A, Goldstein N, Constantini K, Littman R, Eisenkraft A, et al. Twenty-Four-Hour Ambulatory Blood Pressure Measurement Using a Novel Noninvasive, Cuffless, Wireless Device. Am J Hypertens. 2021;34(11):1171–80.
- Vybornova A, Polychronopoulou E, Wurzner-Ghajarzadeh A, Fallet S, Sola J, Wuerzner G. Blood pressure from the optical Aktiia Bracelet: a 1-month validation study using an extended ISO81060-2 protocol adapted for a cuffless wrist device. Blood Press Monit. 2021;26(4):305–11. doi:10.1097/MBP.0000000000000531.
- Ahn JH, Song J, Choi I, Youn J, Cho JW. Validation of Blood Pressure Measurement Using a Smartwatch in Patients With Parkinson’s Disease. Front Neurol. 2021;12:650929. doi:10.3389/fneur.2021.650929.
- Falter M, Scherrenberg M, Driesen K, Pieters Z, Kaihara T, Xu L, et al. Smartwatch-Based Blood Pressure Measurement Demonstrates Insufficient Accuracy. Front Cardiovasc Med. 2022;9:958212. doi:10.3389/fcvm.2022.958212.
- Nair D, Tan SY, Gan HW, Lim SF, Tan J, Zhu M, et al. The use of ambulatory tonometric radial arterial wave capture to measure ambulatory blood pressure: the validation of a novel wrist-bound device in adults. J Hum Hypertens. 2008;22(3):220–2. doi:10.1038/sj.jhh.1002306.
- Sayer G, Piper G, Vorovich E, Raikhelkar J, Kim GH, Rodgers D, et al. Continuous Monitoring of Blood Pressure Using a Wrist-Worn Cuffless Device. Am J Hypertens. 2022;35(5):407–13. doi:10.1093/ajh/hpac020.
- McGillion MH, Dvirnik N, Yang S, Belley-Cote E, Lamy A, Whitlock R, et al. Continuous Noninvasive Remote Automated Blood Pressure Monitoring With Novel Wearable Technology: A Preliminary Validation Study. JMIR Mhealth Uhealth. 2022;10(2):e24916. doi:10.2196/24916.
- Mukkamala R, Shroff SG, Landry C, Kyriakoulis KG, Avolio AP, Stergiou GS. The Microsoft Research Aurora Project: Important Findings on Cuffless Blood Pressure Measurement. Hypertension. 2023;80(3):534–40. doi: 10.1161/HYPERTENSIONAHA.122.20410.
- Stergiou GS, Mukkamala R, Avolio A, Kyriakoulis KG, Mieke S, Murray A, et al. Cuffless blood pressure measuring devices: review and statement by the European Society of Hypertension Working Group on Blood Pressure Monitoring and Cardiovascular Variability. J Hypertens. 2022;40(8):1449–60. doi:10.1097/HJH.0000000000003224.
- Mukkamala R, Stergiou GS, Avolio AP. Cuffless Blood Pressure Measurement. Annu Rev Biomed Eng. 2022;24:203–30. doi: 10.1146/annurev-bioeng-110220-014644.
- Sola J, Vybornova A, Fallet S, Polychronopoulou E, Wurzner-Ghajarzadeh A, Wuerzner G. Validation of the optical Aktiia bracelet in different body positions for the persistent monitoring of blood pressure. Sci Rep. 2021;11(1):20644. doi:10.1038/s41598-021-99294-w.
- Kachel E, Constantini K, Nachman D, Carasso S, Littman R, Eisenkraft A, et al. A Pilot Study of Blood Pressure Monitoring After Cardiac Surgery Using a Wearable, Non-invasive Sensor. Front Med (Lausanne). 2021;8:693926. doi:10.3389/fmed.2021.693926.
- Schukraft S, Haddad S, Faucherre Y, Arroyo D, Togni M, Barison A, et al. Remote blood pressure monitoring with a wearable photoplethysmographic device in patients undergoing coronary angiography: the senbiosys substudy. Blood Press Monit. 2022;27(6):402–7. doi:10.1097/MBP.0000000000000615.
- Nachman D, Gepner Y, Goldstein N, Kabakov E, Ishay AB, Littman R, et al. Comparing blood pressure measurements between a photoplethysmography-based and a standard cuff-based manometry device. Sci Rep. 2020;10(1):16116. doi:10.1038/s41598-020-73172-3.
- Krisai P, Vischer AS, Kilian L, Meienberg A, Mayr M, Burkard T. Accuracy of 24-hour ambulatory blood pressure monitoring by a novel cuffless device in clinical practice. Heart. 2019;105(5):399–405.
- Proença M, Ambühl J, Bonnier G, Meister TA, Valentin J, Soria R, et al. Method-comparison study between a watch-like sensor and a cuff-based device for 24-h ambulatory blood pressure monitoring. Sci Rep. 2023;13(1):6149. doi:10.1038/s41598-023-33205-z.
- Stergiou GS, Avolio AP, Palatini P, Kyriakoulis KG, Schutte AE, Mieke S, et al. European Society of Hypertension recommendations for the validation of cuffless blood pressure measuring devices: European Society of Hypertension Working Group on Blood Pressure Monitoring and Cardiovascular Variability. J Hypertens. 2023.
- Heimark S, Rindal OMH, Seeberg TM, Stepanov A, Boysen ES, Bøtker-Rasmussen KG, et al. Blood pressure altering method affects correlation with pulse arrival time. Blood Press Monit. 2022;27(2):139–46. doi:10.1097/MBP.0000000000000577.
- Jones SC, Bilous M, Winship S, Finn P, Goodwin J. Validation of the OSCAR 2 oscillometric 24-hour ambulatory blood pressure monitor according to the International Protocol for the validation of blood pressure measuring devices. Blood Press Monit. 2004;9(4):219–23. doi:10.1097/00126097-200408000-00007.
- Seeberg TM, Orr JG, Opsahl H, Austad HO, Roed MH, Dalgard SH, et al. A Novel Method for Continuous, Noninvasive, Cuff-Less Measurement of Blood Pressure: Evaluation in Patients With Nonalcoholic Fatty Liver Disease. IEEE Trans Biomed Eng. 2017;64(7):1469–78. doi:10.1109/TBME.2016.2606538.
- Heimark S, Eitzen I, Vianello I, Bøtker-Rasmussen KG, Mamen A, Hoel Rindal OM, et al. Blood Pressure Response and Pulse Arrival Time During Exercise Testing in Well-Trained Individuals. Front Physiol. 2022;13:863855. doi:10.3389/fphys.2022.863855.
- O’Brien E, Petrie J, Little W, de Swiet M, Padfield PL, Altma DG, et al. Short report: An outline of the revised British Hypertension Society protocol for the evaluation of blood pressure measuring devices. Journal of Hypertension. 1993;11(6):677–9. doi:10.1097/00004872-199306000-00013.
- Holdgate A, Asha S, Craig J, Thompson J. Comparison of a verbal numeric rating scale with the visual analogue scale for the measurement of acute pain. Emerg Med. 2003;15(5-6):441–6. doi:10.1046/j.1442-2026.2003.00499.x.
- Marquié L, Duarte LR, Mariné C, Lauque D, Sorum PC. How patients and physicians rate patients’ pain in a French emergency department using a verbally administered numerical rating scale and a visual analog scale. Acute pain: international journal of acute pain management. 2008;10(1):31–7. doi:10.1016/j.acpain.2008.01.003.
- Myles PS, Cui J. Using the Bland-Altman method to measure agreement with repeated measures. Br J Anaesth. 2007;99(3):309–11. doi: 10.1093/bja/aem214.
- Mancia Chairperson G, Kreutz Co-Chair R, Brunström M, Burnier M, Grassi G, Januszewicz A, et al. 2023 ESH Guidelines for the management of arterial hypertension The Task Force for the management of arterial hypertension of the European Society of Hypertension Endorsed by the European Renal Association (ERA) and the International Society of Hypertension (ISH). J Hypertens. 2023.
- Williams B, Mancia G, Spiering W, Agabiti Rosei E, Azizi M, Burnier M, et al. 2018 ESC/ESH Guidelines for the management of arterial hypertension. Eur Heart J. 2018;39(33):3021–104. doi:10.1093/eurheartj/ehy339.
- Cohen J. Weighted kappa: nominal scale agreement with provision for scaled disagreement or partial credit. Psychol Bull. 1968;70(4):213–20. doi: 10.1037/h0026256.
- Nyvad J, Christensen KL, Buus NH, Reinhard M. The cuffless SOMNOtouch NIBP device shows poor agreement with a validated oscillometric device during 24-h ambulatory blood pressure monitoring. J Clin Hypertens (Greenwich). 2021;23(1):61–70. doi:10.1111/jch.14135.
- Socrates T, Krisai P, Vischer AS, Meienberg A, Mayr M, Burkard T. Improved agreement and diagnostic accuracy of a cuffless 24-h blood pressure measurement device in clinical practice. Sci Rep. 2021;11(1):1143. doi: 10.1038/s41598-020-80905-x.
- Miranda Hurtado M, Reyes Vasquez J, Rodriguez-Fernandez M. Comparison of a tonometric with an oscillometric blood pressure monitoring device over 24 hours of ambulatory use. Blood Press Monit. 2021;26(2):149–55. doi: 10.1097/MBP.0000000000000511.
- Tan I, Gnanenthiran SR, Chan J, Kyriakoulis KG, Schlaich MP, Rodgers A, et al. Evaluation of the ability of a commercially available cuffless wearable device to track blood pressure changes. J Hypertens. 2023.
- Almeida TP, Cortes M, Perruchoud D, Alexandre J, Vermare P, Sola J, et al. Aktiia cuffless blood pressure monitor yields equivalent daytime blood pressure measurements compared to a 24-h ambulatory blood pressure monitor: Preliminary results from a prospective single-center study. Hypertens Res. 2023. doi:10.1038/s41440-023-01258-2.
- Zhang G, Gao M, Xu D, Olivier NB, Mukkamala R. Pulse arrival time is not an adequate surrogate for pulse transit time as a marker of blood pressure. J Appl Physiol (1985). 2011;111(6):1681–6. doi:10.1152/japplphysiol.00980.2011.
- Forouzanfar M, Baker FC, Colrain IM, Goldstone A, de Zambotti M. Automatic analysis of pre-ejection period during sleep using impedance cardiogram. Psychophysiology. 2019;56(7):e13355. doi:10.1111/psyp.13355.
- Bilo G, Zorzi C, Ochoa Munera JE, Torlasco C, Giuli V, Parati G. Validation of the Somnotouch-NIBP noninvasive continuous blood pressure monitor according to the European Society of Hypertension International Protocol revision 2010. Blood Press Monit. 2015;20(5):291–4. doi:10.1097/MBP.0000000000000124.
- Mascaro SA, Asada HH. Photoplethysmograph fingernail sensors for measuring finger forces without haptic obstruction. IEEE Transactions on Robotics and Automation. 2001;17(5):698–708. doi:10.1109/70.964669.
- Hsiu H, Hsu CL, Wu TL. Effects of different contacting pressure on the transfer function between finger photoplethysmographic and radial blood pressure waveforms. Proc Inst Mech Eng H. 2011;225(6):575–83. doi:10.1177/0954411910396288.
- Chandrasekhar A, Yavarimanesh M, Natarajan K, Hahn JO, Mukkamala R. PPG Sensor Contact Pressure Should Be Taken Into Account for Cuff-Less Blood Pressure Measurement. IEEE Trans Biomed Eng. 2020;67(11):3134–40. doi:10.1109/TBME.2020.2976989.
- Mukkamala R, Hahn JO, Inan OT, Mestha LK, Kim CS, Töreyin H, et al. Toward Ubiquitous Blood Pressure Monitoring via Pulse Transit Time: Theory and Practice. IEEE Trans Biomed Eng. 2015;62(8):1879–901. doi:10.1109/TBME.2015.2441951.
- Haesler E, Lyon X, Pruvot E, Kappenberger L, Hayoz D. Confounding effects of heart rate on pulse wave velocity in paced patients with a low degree of atherosclerosis. J Hypertens. 2004;22(7):1317–22. doi:10.1097/01.hjh.0000125447.28861.18.
- Tan I, Butlin M, Spronck B, Xiao H, Avolio A. Effect of Heart Rate on Arterial Stiffness as Assessed by Pulse Wave Velocity. Curr Hypertens Rev. 2018;14(2):107–22. doi:10.2174/1573402113666170724100418.
- van der Steen MS, Lenders JW, Thien T. Side effects of ambulatory blood pressure monitoring. Blood Press Monit. 2005;10(3):151–5. doi:10.1097/00126097-200506000-00007.
- Beltman FW, Heesen WF, Smit AJ, May JF, Lie KI, Meyboom-de Jong B. Acceptance and side effects of ambulatory blood pressure monitoring: evaluation of a new technology. J Hum Hypertens. 1996;10 Suppl 3:S39–S42.
- Sheshadri V, Tiwari AK, Nagappa M, Venkatraghavan L. Accuracy in Blood Pressure Monitoring: The Effect of Noninvasive Blood Pressure Cuff Inflation on Intra-arterial Blood Pressure Values. Anesth Essays Res. 2017;11(1):169–73. doi:10.4103/0259-1162.181430.
- Charmoy A, Würzner G, Ruffieux C, Hasler C, Cachat F, Waeber B, et al. Reactive rise in blood pressure upon cuff inflation: cuff inflation at the arm causes a greater rise in pressure than at the wrist in hypertensive patients. Blood Press Monit. 2007;12(5):275–80. doi:10.1097/MBP.0b013e3282c9ac9a.
- Gnanenthiran SR, Liu H, Tan I, Chan J, Schlaich MP, Schutte AE. Cuffless blood pressure devices: the gap between patient acceptability and need for validation. J Hypertens. 2022;40(11):2317–9. doi:10.1097/HJH.0000000000003274.
- Zeng WW, Chan SW, Tomlinson B. Patient preferences for ambulatory blood pressure monitoring devices: Wrist-type or arm-type? PLoS One. 2021;16(8):e0255871. doi:10.1371/journal.pone.0255871.
- Basu S, Savani K. Choosing Among Options Presented Sequentially or Simultaneously. Curr Dir Psychol Sci. 2018;28(1):97–101. doi: 10.1177/0963721418806646.
- Hsee CK, Leclerc F. Will Products Look More Attractive When Presented Separately or Together? Journal of Consumer Research. 1998;25(2):175–86. doi:10.1086/209534.