Abstract
The conceptual approach used in this study incorporates spatial analysis techniques for data integration and analysis to perform reconnaissance-scale mineral prospectivity mapping for iron oxide copper – gold (IOCG) mineralisation in Finland. The known IOCG occurrences in Finland are characterised by the following features: (i) an epigenetic magnetite-rich host-rock; (ii) an association of Fe – Cu – Au ± Co ± U; (iii) ore minerals comprising magnetite, chalcopyrite, pyrite or pyrrhotite, and native gold; (iv) a gangue dominated by Ca-amphibole ± diopside, albite and biotite; (v) enrichment in Ag, Au, Bi, Ca, CO2, Cu, Fe, S, Te ± As, Ba, Cl, Co, K, LREE, Mo, Na, Pb, Rb, Sb, Se, U; (vi) multi-stage alteration; (vii) formation in the P – T range of 400 – 600°C, 150 – 350 MPa; and (viii) a distinct structural control in regions that have experienced both extensive compression and extension. The datasets used for the prospectivity analysis include a 1:1 000 000 scale geological map, high-resolution airborne geophysics, regional-scale multi-element till-geochemistry data, and a mineral occurrence database. The derived parameters used in the conceptual analysis include: (i) proximity to the craton margin; (ii) intersecting fault structures; (iii) presence of granitic intrusions particularly those with compatible and incompatible element enrichment; (iv) Cu, Co and Fe concentrations in till samples; (v) presence of hematite; and (vi) airborne magnetic highs and radiometric U data. A conceptual fuzzy-logic model was used to predict and locate the most prospective or favourable areas for IOCG exploration in the study area using the above-mentioned data layers. The models identify several permissive and high-potential areas within a significantly reduced potential exploration area. Validation of the modelling was conducted by quantifying the spatial association between the predicted endowment as favourability classes on the prospectivity map and the known mineral deposit sites with IOCG affinities using the Bayesian weights-of-evidence method.
Introduction
The amount of digital geoscientific data available in mineral exploration is increasing rapidly, and the technologies for storing, maintaining and analysing these data have been developing equally fast during recent years. The spatially referenced geoscience data are especially suitable for rapid quantitative analysis using a Geographic Information System (GIS) in order to assist in the decision-making process or strategic management and planning in an organisation involved in mineral exploration. As stated by Luo and Dimitrakopoulos (Citation2003), these quantitative methods are often used to: (i) extract the maximum amount of information from the data; (ii) effectively combine data from diverse information sources; (iii) provide tools to quantify inherent uncertainties within the data; (iv) rank potential targets (mineral sites); and (v) reduce data processing and evaluation time.
In this paper, a prospectivity (or endowment) map is presented for iron oxide copper – gold (IOCG) deposits in the northern Fennoscandian Shield, northern Finland () at 1:1 000 000 reconnaissance scale. The IOCG deposits, first defined by Hitzman et al. (Citation1992), are a diverse group of mineral deposits described as forming a continuum from Cu – Au deposits to Cu- and Au-free Kiruna-style magnetite – apatite deposits (Hitzman et al. Citation1992; Pollard Citation2000; Williams et al. Citation2005). Thus, their precise characteristics in terms of geological, geophysical and geochemical data vary, making a prospectivity analysis a challenging exercise. Bonham-Carter (Citation1994) divided spatial analysis into two main categories: empirical or data-driven vs conceptual or knowledge-driven. In Finland, there are only a few known mineral occurrences which have features broadly in common with the IOCG deposit type (Eilu et al. Citation2003). Hence, the lack of a sufficient number of training sites precludes the use of empirical (data-driven) modelling techniques to produce a IOCG prospectivity map. Instead, a conceptual fuzzy-logic overlay method was employed to create a prospectivity map. The 11 prospects or deposits with IOCG affinities in the study area were used to validate the prospectivity maps. The validation was accomplished by calculating categorical weights for the favourability classes of the IOCG prospectivity map using the weights-of-evidence method (Bonham-Carter Citation1994).
Figure 1 Simplified geological map of the Fennoscandian Shield (modified from Koistinen et al. Citation2001). Boundaries of the major geologic units from Lahtinen et al. (Citation2005). Filled circles represent deposits and showings with IOCG affinities (Eilu Citation1999; Niiranen et al. Citation2002; Edfelt et al. Citation2005; Weihed et al. Citation2005). Filled diamonds define the location of kimberlites (Lehtonen Citation2005). A thick grey line was used in the modelling to represent the Archean craton margin defining the study area together with the border of Finland. Kainuu suture interpreted from Peltonen et al. (Citation2003) and Peltonen (Citation2005). Numbers refer to occurrences listed in .
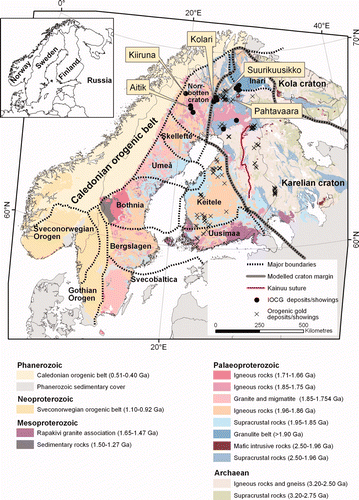
In the northern part of the Fennoscandian Shield, deposits with broadly IOCG affinities define a continuum from apatite – Fe (e.g. Kiruna) and epigenetic Cu – Au to intrusion-related Cu – Au deposits with very few Fe oxides (e.g. Aitik) as discussed by Nyström & Henriquez (Citation1994), Edfelt et al. (Citation2005), Wanhainen et al. (Citation2005) & Niiranen et al. (Citation2007). These deposits occur in Archean to Proterozoic crust along the rifted southwest margin of the Archean Karelian craton where they are temporally associated with calc-alkalic to alkalic magmatism. This rifted margin contains other ores and volatile-rich magmas, including: (i) a large number of epigenetic occurrences with an unusual metal association (variable combinations of Au, Co, Cu, F, Fe, LREE, Nb, Ni, Pd, P, Th, U, and Zr) (Weihed et al. Citation2005); (ii) the Outokumpu Cu – Co – Zn – Ni – Au massive-sulfide deposits (Gaál et al. Citation1975; Kontinen et al. Citation2005); (iii) the Siilinjärvi carbonatite-hosted apatite deposit (Puustinen & Kauppinen Citation1989); (iv) lamprophyres (O'Brien et al. Citation2005); and (v) kimberlites (Lehtonen Citation2005; O'Brien et al. Citation2005). The craton margin has been recognised as one of the most significant metallogenic belts in the Fennoscandian Shield, resulting in decades of debate over possible genetic connections between the ore types located there (Hietanen Citation1975; Gaál & Gorbatschev Citation1987; Peltonen Citation2005). It is this craton margin that is investigated here.
The fuzzy-logic overlay method has been used widely in mineral potential assessments in various ways and scales. An et al. (Citation1991) used fuzzy logic to combine geophysical and geological maps for prospectivity analysis of base-metal and iron deposits using expert knowledge to define the fuzzy membership values. Bonham-Carter (Citation1994) gave textbook examples on using fuzzy-logic overlay for defining gold potential and landfill suitability. D'Ercole et al. (Citation2000) defined a fuzzy-logic approach to make a prospectivity analysis of Mississippi Valley-type deposits using geochemical, stratigraphic, geophysical and structural criteria. Knox-Robinson (Citation2000) applied vector algebra in fuzzy modelling, where favourability was expressed as a vector whose direction is defined by fuzzified prospectivity, and magnitude is defined by fuzzified confidence value. Carranza & Hale (Citation2001) applied fuzzy logic to map gold potential using derivatives of geological maps as inputs. Porwal et al. (Citation2003) incorporated a data-driven approach in fuzzy modelling, and described a new membership function simulating human reasoning for deriving fuzzy membership values in a knowledge-driven model. Luo & Dimitrakopoulos (Citation2003) used fuzzy analysis in order to produce a mineral favourability index, and introduced a new weighted average-type aggregation operator resembling the data-driven approaches. Tangestani & Moore (Citation2003) applied fuzzy-set theory to prospecting porphyry copper deposits using geochemical, geophysical and geological data. Subjective geological knowledge was used by Brown et al. (Citation2003) in prospectivity mapping by using fuzzy membership input layers in neural network models. Porwal et al. (Citation2004) were also combining fuzzy logic together with neural networks in their hybrid neuro-fuzzy modelling approach. Nykänen et al. (Citation2005) used fuzzy-logic spatial modelling to integrate high-resolution geophysics and regional-scale till geochemistry at the target scale. Fuzzy logic was also used by Rogge et al. (Citation2006) to integrate geological maps, geochemistry, aeromagnetic data and satellite radar data for shear-hosted gold mineralisation potential. These papers give an overview of different approaches and datasets used in fuzzy prospectivity mapping. The validation process appears to be crucial in many cases. The other knowledge-driven methods, like the evidential belief function (Moon Citation1990), the decision-tree approach (Reddy & Bonham-Carter Citation1991) and the Dempster – Shafer model (Tangestani & Moore Citation2002; Rogge et al. Citation2006), have also been used for mineral potential assessment studies. It is obvious that the results of the modelling, regardless of the method, are heavily dependent on the quality of the input data.
The basic data from which parameters to define IOCG deposit-type favourability are derived, and hence used in the prospectivity analysis in this paper, are the geoscientific regional datasets () collected, compiled and maintained by the Geological Survey of Finland. High-resolution airborne geophysical surveys flown at a low-altitude (40 m), with an average line spacing of 200 m and measurement points every 10 – 50 m, cover almost 100% of the land surface of Finland (Airo 2005). For the regional geochemical survey covering the whole country, till c-horizons were sampled, with one sample per 4 km2, and assayed for 27 elements, including Au, Te and Pd in some parts of the survey area (Salminen Citation1995). The bedrock map of Finland at 1:1 000 000 scale (Korsman et al. Citation1997) was also used as a source of information, as well as the mineral occurrences database including about 9800 records of mineralised boulders and outcrops in Finland (Saltikoff & Tarvainen Citation1990). This is a rare case where almost continuous layers of geoscientific data provided by government are available for conceptual targeting and prospectivity analysis.
Table 1 Fuzzification parameters for the input layers used in the modelling.
Study area
The study area is located in the northern Fennoscandian Shield (), which is the northwesternmost part of the East European craton. The Archean bedrock in the Fennoscandian Shield is divided into three adjacent cratonic nuclei, Norrbotten, Karelia and Kola, which may have originated separately, were fragmented between 2.51 and 2.4 Ga, and finally were amalgamated by ca 1.9 Ga (Alapieti & Lahtinen Citation2002; Lahtinen et al. Citation2005). Between 1.9 and 1.8 Ga, a number of juvenile volcanic arcs and microcontinents were accreted to the Archean craton, mostly on its southwestern margin. This resulted in the formation of the present Fennoscandian Shield by cratonisation by 1.77 Ga (Lahtinen et al. Citation2005).
The northern region of the Fennoscandian Shield is recognised as an economically important metallogenic province dominated by generally small Fe oxide and Au – Cu deposits, some with IOCG affinities (Niiranen et al. Citation2002; Edfelt et al. Citation2005; Niiranen Citation2005; Weihed et al. Citation2005). These deposits mainly occur within or close to (<100 km) the margin of the Archean craton (), above Archean continental crust and subcontinental lithospheric mantle, and are hosted by juvenile Paleoproterozoic volcano-sedimentary sequences formed during rifting of the Archean craton (Weihed et al. Citation2005). As summarised by Weihed et al. (Citation2005), geochronological studies indicate that Paleoproterozoic hydrothermal mineralisation was multiphase, probably occurring at least over 160 million years from 1.9 to 1.76 Ga.
Fuzzy-logic methodology
Among the many spatial modelling techniques, the fuzzy-logic model (in the knowledge-driven group of Bonham-Carter Citation1994) provides a convenient way to interpret and transfer expert knowledge into a quantitative spatial analysis in a GIS platform in a stepwise practice as expressed in the flow chart in . It is based on fuzzy-set theory first introduced by Zadeh (Citation1965). Whereas in classical set theory, the membership of a set is defined as true or false (one or zero), in fuzzy-set theory, the membership of a set is defined on a continuous scale from true (full membership or one) to false (full non-membership or zero). A fuzzy set of A is a set of ordered pairs:
where X is a collection of objects and μ A (x) is the membership function (degree of truth) of x in A: that is, μ A (x) quantifies the grade of membership of x in A. The membership function can be anything from linear to non-linear or non-continuous (Carranza & Hale Citation2001). The membership is usually used to reflect a statement of the problem that has been addressed. In mineral exploration, the statement could be: ‘definition of favourable location for mineralisation.’ As stated by An et al. (Citation1991), the fuzzy membership function is generally different from the traditional probability concept. Experts can assign the memberships to each input dataset according to governing physical or chemical principles and the scientists' expertise. In this paper, the proposition of the fuzzy membership is an ‘indication of favourable location for an IOCG deposit,’ and the specific membership functions used here (‘Large’ and ‘Small’) were adopted from Tsoukalas & Uhrig (Citation1997). The expression for the membership function is:
where μ(x) is the membership corresponding to the value of variable x, f 1 is the spread, and f 2 is the mid-point where the membership is 0.5. For the Small function, the spread is positive, whereas for the Large function, the spread is negative. The shape of the function is controlled by these variables, and for this study, the mid-point was set to the mean of each dataset, and the spread was given an intermediate value of 5 to maintain the simplicity of the model. In order to tune the model, one could change these parameters to achieve a somewhat skewed distribution of the fuzzy membership values. The assignment of fuzzy memberships by using the membership function, of which the above-mentioned Large and Small are examples, is also called a fuzzification process. The fuzzification parameters for each data layer are given in . The ultimate purpose of the fuzzification procedure is to transfer expert knowledge of the importance of each individual data source into one common scale so that the data can be integrated.
After assigning the fuzzy membership values based on expert knowledge, and using the above-described methods, the datasets are in a common scale from 0 to 1 (full non-membership to full membership of the statement ‘favourable location for an IOCG deposit’). The next step is to integrate all this information into a single-output fuzzy set, that is a prospectivity map (also known as a favourability or endowment map), by using a variety of fuzzy operators (). As in classical set theory, set-theory operations can be applied to fuzzy sets. There are several operations available, some of them commonly used in GIS, including union and intersection. An et al. (Citation1991), Bonham-Carter (Citation1994) and Carranza & Hale (Citation2001) described the five operators used in mineral exploration-related datasets. These are the Fuzzy AND, Fuzzy OR, Fuzzy algebraic product, Fuzzy algebraic sum and Fuzzy Gamma (γ) operator (). In this paper, the minimum operator, Fuzzy AND, the maximum operator, Fuzzy OR, and the Fuzzy Gamma operator, which is a combination of Fuzzy algebraic sum and Fuzzy algebraic product, were used. The γ parameter in the Fuzzy Gamma function has values from 0 to 1. When the γ parameter is one, the combination equals the Fuzzy algebraic sum, and when the γ parameter is zero, the combination equals the Fuzzy algebraic product.
Table 2 Fuzzy operators as described by An et al. (Citation1991), Bonham-Carter (Citation1994), and Carranza & Hale (Citation2001).
The fuzzification and data integration using the fuzzy operators described above as well as the validation calculations were carried out using an ArcGIS™ extension called Arc-SDM or Spatial Data Modeller for ArcGIS described by Raines et al. (Citation2000) and more recently by Raines and Bonham-Carter (Citation2006). The code is freely downloadable from <http://www.ige.unicamp.br/sdm/default_e.htm> and may be used for examining spatial associations between spatial data layers and mineral sites, or other locations of interest.
Iron oxide Cu – Au characteristics
Iron oxide Cu – Au (IOCG) deposits were first defined as a distinctive class of mineral deposits by Hitzman et al. (Citation1992) after discovery and research on the Olympic Dam deposit in the Gawler Craton of South Australia (Oreskes & Einaudi Citation1990, Citation1992). The category IOCG now includes a variety of deposits enriched in iron oxides, either magnetite or hematite, some of which contain Cu and Au, whereas others contain only Cu or Au, and yet others are iron-oxide-rich deposits with accessory P (e.g. Kiruna) or REE (e.g. Bayan Obo). A recent review is provided by Williams et al. (Citation2005).
In this paper, IOCG is used as a term to describe those deposits placed in the broader grouping that are iron-oxide-rich deposits with significant Cu – Au grades. These have been varyingly considered to be of non-magmatic (Barton & Johnson Citation1996) to magmatic – hydrothermal (Pollard Citation2000, Citation2006) derivation, although the large economic examples (e.g. Olympic Dam; Carajas deposits such as Igarapé Bahia) provide overwhelming evidence for a magmatic origin.
Such IOCG deposits are characterised by: (i) a metal association of Fe – Cu – Au ± Co, U, REE, Ba, F; (ii) low sulfur concentration; (iii) extensive metasomatism including localised, but repeated, Fe – K ± Na, Cl, Ca metasomatism; (iv) moderate to high salinity, oxidising, aqueous ore fluids; (v) a spatial association with crustal-scale faults; and (vi) an extensional tectonic setting (Hitzman et al. Citation1992; Williams et al. Citation2005). The economic examples are in terranes with abundant alkalic to A-type granitoids.
Groves & Vielreicher (Citation2001) suggested that most IOCG deposits (especially, the largest deposits in the class) are located close to a craton margin or other major lithospheric boundary, where decompression melting of metasomatised mantle produces volatile-rich alkaline magmas rich in REE, P, F and other incompatible elements such as S, Cu and Au. These magmas have associated hydrothermal activity, which produces deposits with an element association compatible with their source, including variable combinations of Au, Co, Cu, F, Fe, LREE, Ni, P, Pd, P, Th and U. The IOCG deposits produced are commonly large pipe-like breccia bodies, with dimensions similar to diamondiferous kimberlite pipes that contain S-poor assemblages enriched in incompatible elements. They are commonly controlled by structural intersections in the vicinity of crustal-scale lineaments (Groves & Vielreicher Citation2001).
From the characteristic features of IOCG deposits, it is possible to define those that may be visible in geological and geophysical map data and other databases. Theses features include: (i) proximity to craton margin; (ii) intersecting fault structures; (iii) presence of granitic intrusions, particularly those that may be enriched in both compatible and incompatible elements; (iv) magnetic highs related to magnetite concentrations in the deposit; (v) high-U radiometric signatures; (vi) presence of hematite; and (vii) anomalous Cu, Au or Fe and incompatible elements associated with mineralisation. Fortunately, all these parameters that are indicative of IOCG mineralisation can be derived from the various geoscience datasets collected over Finland.
Primary datasets for input parameters
The datasets used to define the input parameters based on the critical characteristics defined above were high-resolution airborne geophysics, regional till geochemistry and their derivatives, together with derivatives of the 1:1 000 000 scale bedrock map of Finland, all available from the Geological Survey of Finland. In the following sections, the primary datasets are described, followed by short descriptions of the methodologies to generate the input parameters for the modelling in GIS.
Bedrock geological map and derived structural interpretations
The derivatives of the digital 1:1 000 000 scale bedrock map of Finland (Korsman et al. Citation1997) were used as the conceptual inputs in the modelling. The map defines geological units on a broad scale and is heavily generalised from the existing higher resolution geological bedrock maps. Selected spatial analysis functions within the GIS, including proximity analysis and density analysis, were applied to generate the input map parameters (i.e. evidence maps) corresponding to the conceptual IOCG model.
Lineament density
Density maps can be created from point or line data. The user-defined circular search area determines the distance to search for features to calculate a density value for each cell in the output raster. Lineament density is measured in length of lines per unit area (defined by the search radius). For this study, the lineament dataset was derived from the 1:1 000 000 scale bedrock map combined with the interpretations of the airborne geophysics and digital elevation model (A. Kuivamäki unpubl. data). A density grid was calculated by using a 20 km search radius. The search radius was selected empirically so that the resulting grid would represent a regional aspect rather than a local-scale feature. The fuzzy membership values were assigned by using the Large function and, a mean lineament density value of 0.38 km/km2 was used as a mid-point ().
Craton margin and Kainuu suture
The location of craton margins was derived from Lahtinen et al. (Citation2005), and a proximity grid was calculated using a 200 × 200 m cell size, as the IOCG deposits have been shown to be located near craton margins (Groves & Vielreicher Citation2001). The Kainuu suture, containing mantle lithosphere, was derived from the 1:1 000 000 scale bedrock map of Finland (Korsman et al. Citation1997) by interpreting the results of Peltonen et al. (Citation2003) and Peltonen (Citation2005), and a proximity grid was then calculated. The fuzzy membership values were assigned using the Small function with midpoints set to 100 km for both ().
Igneous activity (1.9 – 1.7 Ga)
Proximity to granitoids emplaced between 1.9 and 1.7 Ga derived from the 1:1 000 000 scale bedrock map of Finland was calculated, since IOCG deposits in the Fennoscandian Shield have been suggested to be related to igneous activity during this time span. The mid-point parameter was set at 15 km, and the Small function was applied to assign the fuzzy membership values for this proximity map ().
Hematite showings in mineral occurrence database
The mineral occurrence database has been compiled at the Geological Survey of Finland to store mineral occurrences from different sources. The database includes some 9800 entries of mineralised boulders and outcrops. From this dataset, all the occurrences of hematite in the study area were selected, and a proximity grid to those locations was calculated. According to Salonen (Citation1986), the average glacial transport distance for surface boulders in Finland varies from 0.8 to 10 km in cover moraine areas and from 5 to 17 km in drumlin assemblages. The study area of the current paper is dominantly being mapped as cover moraine. Considering the uncertainty of the source location due to glacial transport, the mid-point parameter was set at 24.2 km, and the Small function was applied to assign the fuzzy membership values for the map ().
Till geochemistry
The countrywide regional till-geochemical survey (referred to as ‘regional till’ geochemistry in ) was conducted in the 1980s (Salminen Citation1995), with a sampling density of one sample per 4 km2. Most samples were collected as a composite of three to five sub-samples, from an average depth of 1.5 m, taken with a portable percussion drill equipped with a through-flow bit. The sampled material was chemically unaltered parent till. The samples were dried and the <0.06 mm fraction sieved for analysis. Hot aqua regia digest was used, and Al, Ba, Ca, Co, Cr, Cu, Fe, K, La, Li, Mg, Mn, Mo, Ni, P, Pb, Sc, Sr, Th, Ti, V, Y, Zn and Zr were determined by ICP-AES. In addition, Au, Pd and Te were analysed with graphite furnace AAS (Kontas Citation1981; Kontas et al. Citation1990). As Th and U assays in the regional till dataset were not at an adequate level in terms of quality, the assays for these elements were taken from another, much broader-scale survey with one sample per 300 km2 (Koljonen Citation1992), referred to as ‘Atlas till’ geochemistry in . For that survey, the sampling depth was 0.5 – 2 m, and the samples were taken from non-weathered till, mostly from the upper part of the C-horizon. Composite samples of five subsamples were collected from an area of 300 × 1000 m in southern Finland, whereas in northern Finland, the samples were prepared from an earlier survey (Bølviken et al. Citation1986) at a density of one sample per 30 km2. Total element concentrations of U and Th were determined by neutron activation analysis.
The IOCG deposits are associated with several geochemical indicators, and the regional till geochemical survey of Geological Survey of Finland has been widely used as a reconnaissance-scale exploration tool. For modelling, the selected element data were interpolated into grids with 200 × 200 m cell size using inverse distance weighting (IDW). The IDW parameters were: power = 2; variable search radius; number of points = 12. These parameters were selected from empirical knowledge. Furthermore, the interpolated grids were generalised by calculating a median value over a 4 km radius around each cell in the original grid, resulting in 2500 × 2500 m cell size grids used as input in the modelling. The generalisation was done in order to equalise the spatial accuracy of the data with the other geochemical dataset, called ‘Atlas till,’ which was not generalised, but the same interpolation parameters were applied. The elements used were Co, Cu and Fe, reflecting a sulfide source. Chrome, Ni and Ti were used to represent compatible elements, and Ba, K, La, Li, P, Th and U to represent incompatible element enrichment, which may be present in both source granitoid and the IOCG orebody itself. The mean value for each element was used as a mid-point parameter in the fuzzification process when using the Large function as described above (). The incompatible and compatible element groups of elements were combined using the Fuzzy OR operator, and the resulting two grids were combined by using the Fuzzy AND operator (). The elements reflecting sulfidic sources were combined together using the minimum operator, Fuzzy AND ().
Airborne geophysics
Almost the entire land surface of Finland is covered by high-resolution, multi-component, airborne geophysical surveys using 40 m flight altitude and 200 m line spacing (Airo 2005). Airborne magnetic measurements were derived from a cesium magnetometer on the rear boom of the aircraft. Secular and other magnetic field variations were corrected according to data from a base station registration. The line data were levelled using an in-house program, and final levelling was done using tie lines.
Two datasets from the multi-component airborne survey were selected as input parameters in the modelling. Because the IOCG deposits are typically located within or close to magnetic anomalies, the magnetic-field total-intensity data were used to define point-like anomalies in the study area. This was accomplished by using neighbourhood (focal) statistics to calculate the mean and standard deviation (σ) around a 4 km moving window across the study area. Then, those areas, which are above 3σ or below −3σ of mean value, were selected, and finally a proximity grid was created for the clusters with more than two pixels as an input layer in the modelling.
The uranium channel of the gamma-radiation survey was the other component from the airborne survey used in the modelling. The original grid was generalised to be closer to the resolution of the rest of the data using neighbourhood statistics with a 2 km moving window to calculate mean value. The output was stored as a 200 × 200 m cell size grid. Neither the magnetic data nor the U data were used alone. They were combined using the Fuzzy AND operator, resulting in a grid used as input in the modelling ().
Data integration into the prospectivity map
The integration of parameters into a single prospectivity map was completed using the fuzzy-logic overlay method described by Bonham-Carter (Citation1994). The inference network in is a concise statement of the exploration model for IOCG deposits by defining the combination procedure. The integration was done in steps, and the intermediate maps, together with some of the original maps, and the final prospectivity map are shown in and .
The four geological input layers shown in were used to give the geological input into the exploration model. The assumption was that the high density of linear features () reflects deep structures which would be favourable for IOCG deposits, as well as near presence of 1.9 – 1.7 Ga granitoids () and hematite showings (). As stated earlier (Groves & Vielreicher Citation2001), the IOCG deposits tend to be located near craton margins or other major lithospheric boundaries. Thus, the proximity to the Karelian craton margin and proximity to the Kainuu suture were combined into the ‘proximity to boundaries’ map in using the Fuzzy OR operator.
For the geochemical evidence layers, the maximum operator, Fuzzy OR, and the minimum operator, Fuzzy AND, were used to combine the individual element groups. The compatible element combination and the incompatible element combination were both carried out using the minimum operator, Fuzzy OR. The resulting combination of these two groups of elements was then achieved by using the minimum operator, Fuzzy AND (), to be a sign of favourable magmatic environment. The elements Co, Cu and Fe in till, were combined by using the minimum operator, Fuzzy AND (), to indicate sulfidic sources.
Proximity to radiating magnetic anomalies () was achieved by integrating the proximity to magnetic point anomalies together with the uranium radiation anomalies using the Fuzzy AND operator.
The final combination was done using the Fuzzy Gamma operator with a γ parameter value of 0.75. The selection of the γ parameter value is a subjective decision, and several values were tested. The selection of the fuzzification parameters, together with the combination operators and their parameters, are the factors driving the output, but are difficult to control when there are numerous input layers as in this study. The Fuzzy OR operator can be described as a maximum operator, since it returns the maximum value of any of the input layers and is a logical union of these. On the other hand, Fuzzy AND could be described as a minimum operator, since it returns the minimum value of any of the input layers and is a logical intersection of these. When we require a conservative estimate of a set membership, we use Fuzzy AND, whereas using Fuzzy OR would be applicable when the presence of any positive evidence to suggest favourability is desired (Bonham-Carter Citation1994).
Validation
Validation of the modelling is an essential part of a prospectivity mapping analysis. However, validation can be challenging for a conceptual model, because these are typically used when there are no known mineralised sites to be used as validation sites. In this study, 11 mineral deposits or showings () with IOCG affinities were used as validation sites employing categorical weight calculations based on the weights-of-evidence method (Bonham-Carter Citation1994) to quantify the spatial association, as measured by the contrast between these sites and the final prospectivity map (). Weights-of-evidence modelling is based on the Bayesian conditional probability concept, where the first step is to estimate the prior probability (P prior). Taken that the study area is divided into a number of N(T) units cells containing N(D) pixels including only one prospect or training site (D), the P prior that a randomly selected pixel would contain a prospect (D) can be estimated as N(D)/N(T), which actually is the training site density in the study area. Suppose that, within the study area there are N(B) and N([Bbar]) pixels where spatial evidence B is present and absent, respectively: in this case, a positive weight (W+) and a negative weight (W−) can then be estimated for N(B) and for N([Bbar]), respectively, given the presence N(D) and absence N([Dbar]) of D. The weights can be estimated from likelihood ratios as:
Table 3 Mineral deposits and occurrences with iron oxide copper – gold affinities within the study area used as validation sites.
Table 4 Categorical weights calculated for the five prospectivity classes in the final prospectivity map in .
The prospectivity map () was first generalised into five classes with equal intervals, and the distribution of the validation sites on those map classes was then measured. The contrast in for each class is the measure of correlation. It appears that 54% of the validation sites are located within 3% of the study area with an acceptable confidence level (confidence >1.97) and that only one of the 11 validation sites is located in the lowest prospectivity class. It may be noted that the validation sites are occurrences with IOCG-like features, not necessarily classic IOCG deposits. Thus, the validation done by using these sites could be somewhat misleading. However, a recently confirmed IOCG deposit at Kolari (Niiranen et al. Citation2007), currently owned and explored by Northland Resources Inc., is sited within a high favourability domain in the IOCG prospectivity map described in this paper.
Discussion
The major challenge in completing a prospectivity analysis in GIS for IOCG deposits is the nature of this diverse deposit type. There are no characteristic features that could be applied solely to distinguish these deposits using common geoscientific data. Instead, these deposits tend to vary in shape, size and composition, forming a continuum between the end members at different crustal depths and in different host-rock sequences. There are, however, several factors that can be used to make a conceptual assessment of IOCG, as is shown in this paper. Direct observations of indicator minerals and certain geophysical and geochemical parameters are useful in a prospectivity analysis.
The lack of known IOCG deposit sites in the study area is another disadvantage. This makes it difficult to validate the results, when the number of validation sites is small, as it is in the Fennoscandian Shield. It is also unclear whether or not some of the validation sites belong to the IOCG deposit type or simply resemble it in several characteristics.
In the fuzzy-logic approach used in this paper, it is difficult to judge the magnitude of the effect of classification (i.e. how the fuzzy membership values are assigned) and also the effect of choice of the fuzzy operators used in data integration. These issues have not been reviewed in the literature to any extent, so that the effects remain unclear.
Conclusions
A fuzzy-logic overlay method was used to make an assessment of IOCG potential in the northern Fennoscandian Shield, Finland. The combined datasets were: (i) derivatives of a 1:1 000 000 scale bedrock map; (ii) high-resolution airborne geophysics; (iii) regional till geochemistry; and (iv) a mineral occurrences database. From these datasets, the following parameters were derived to be used as input in the modelling: (i) proximity to craton boundary; (ii) proximity to Kainuu suture; (iii) density of lineaments; (iv) proximity to granitic intrusions (1.9 – 1.7 Ga); (v) proximity to hematite showings; (vi) anomalous levels of incompatible elements (Ba, K, La, Li, P, Th and U), together with anomalous levels of compatible elements (Cr, Ni and Ti) in till; (vii) anomalous levels of elements (Co, Cu and Fe) indicating sulfidic sources in till; and (viii) proximity to magnetic anomalies with high U radiation levels.
Data integration using a GIS resulted in the following main conclusions with respect to IOCG exploration potential in the Fennoscandian shield.
(1) Based on the GIS-based prospectivity analysis, there is considerable potential for IOCG deposits along the Archean craton margin, and several prospective areas can be defined.
(2) Deposits could be related to alkalic to A-type granitoids derived from metasomatised subcontinental lithospheric mantle adjacent to the craton margin.
(3) Most of the known deposits with at least some IOCG characteristics lie within the conceptually predicted target zones, as do other magnetite-rich deposits with a metal association that is anomalous for syngenetic iron deposits.
(4) Importantly, the recently confirmed IOCG deposit at Kolari (Niiranen et al. Citation2007), currently being explored by Northland Resources Inc. is sited within a high favourability domain in the IOCG prospectivity map.
Acknowledgements
We would like to thank Gary Raines from the US Geological Survey and Jeff Harris from the Geological Survey of Canada, for their constructive and thorough reviews. Their comments and corrections helped us to improve the paper significantly. Petri Peltonen and Hugh O'Brien contributed ideas in the early stages of the project.
References
- Airo M-L. ed. 2005. Aerogeophysics in Finland 1972 – 2004: Methods, system characteristics and applications. Geological Survey of Finland Special Paper 39
- Alapieti , T. and Lahtinen , J. 2002 . “ Platinum-group element mineralization in layered intrusions of northern Finland and the Kola Peninsula, Russia ” . In The Geology, Geochemistry, Mineralogy and Mineral Beneficiation of Platinum-Group Elements Edited by: Cabri , L. J. 507 – 546 . CIM Special Volume 54
- An , P. , Moon , W. M. and Rencz , A. 1991 . Application of fuzzy set theory to integrated mineral exploration . Canadian Journal of Exploration Geophysics , 27 : 1 – 11 .
- Barton , M. D. and Johnson , D. A. 1996 . Evaporitic source model for igneous-related Fe oxide – (REE – Cu – Au – U) mineralization . Geology , 24 : 259 – 262 .
- Bølviken , B. , Bergström , J. , Björklund , A. , Kontio , M. , Lehmuspelto , P. , Lindholm , T. , Magnusson , J. , Ottesen , R. T. , Steenfelt , A. and Volden , T. 1986 . Geochemical Atlas of Northern Fennoscandia (Scale 1: 4 000 000) , Uppsala : Geological Survey of Sweden .
- Bonham-Carter , G. F. 1994 . Geographic Information Systems for Geoscientists – Modelling with GIS , New York : Pergamon Press . (Computer Methods in the Geosciences 13).
- Brown , W. , Groves , D. , Gedeon , T. and Kouda , R. 2003 . Use of fuzzy membership input layers to combine subjective geological knowledge and empirical data in a neural network method for mineral-potential mapping . Natural Resources Research , 12 : 183 – 200 .
- Carranza , E. J. M. and Hale , M. 2001 . Geologically constrained fuzzy mapping of gold mineralization potential, Bauio District, Philippines . Natural Resources Research , 10 : 125 – 136 .
- D'Ercole , C. , Groves , D. I. and Knox-Robinson , C. M. 2000 . Using fuzzy logic in a Geographic Information System environment to enhance conceptually based prospectivity analysis of Mississippi Valley-type mineralisation . Australian Journal of Earth Sciences , 47 : 913 – 927 .
- Edfelt , Å. , Armstrong , R. N. , Smith , M. and Martinsson , O. 2005 . Alteration paragenesis and mineral chemistry of the Tjårrojåkka apatite – iron and Cu ( – Au) occurrences, Kiruna area, northern Sweden . Mineralium Deposita , 40 : 409 – 434 .
- Eilu , P. 1999 . FinGOLD—a public database on gold deposits in Finland . Geological Survey of Finland, Report of Investigation , 146 Online <http://en.gtk.fi/ExplorationFinland/Commodities/Gold/gtk_gold_map>
- Eilu , P. , Sorjonen-Ward , P. , Nurmi , P. and Niiranen , T. 2003 . A review of gold mineralization styles in Finland . Economic Geology , 98 : 1329 – 1353 .
- Gaál , G. and Gorbatschev , R. 1987 . An outline of the Precambrian evolution of the Baltic shield . Precambrian Research , 35 : 15 – 52 .
- Gaál , G. , Koistinen , T. and Mattila , E. 1975 . Tectonics and stratigraphy of the vicinity of Outokumpu, North Karelia, Finland: including a structural analysis of the Outokumpu ore deposit . Geological Survey of Finland Bulletin , 271
- Groves , D. I. and Vielreicher , N. M. 2001 . The Phalabowra (Palabora) carbonatite-hosted magnetite – copper sulphide deposit, South Africa: an end-member of the iron-oxide copper – gold – rare earth element deposit group . Mineralium Deposita , 36 : 189 – 194 .
- Hietanen , A. 1975 . Generation of potassium poor magmas in the northern Sierra Nevada and the Svecofennian of Finland . Journal of Research of the US Geological Survey , 3 : 631 – 645 .
- Hitzman , M. W. , Oreskes , N. and Einaudi , M. T. 1992 . Geological characteristics and tectonic setting of Proterozoic iron oxide (Cu – U – Au – REE) deposits . Precambrian Research , 58 : 241 – 287 .
- Knox-Robinson , C. M. 2000 . Vectorial fuzzy logic: a novel technique for enhanced mineral prospectivity mapping, with reference to the orogenic gold mineralisation potential of the Kalgoorlie Terrane, Western Australia . Australian Journal of Earth Sciences , 47 : 929 – 941 .
- Koistinen T. Stephens M. B. Bogatchev V. Nordgulen O. Wennerström M. Korhonen J. Geological Map of the Fennoscandian Shield, Scale 1:2 000 000 Geological Survey of Finland Espoo 2001
- Koljonen T. The Geochemical Atlas of Finland, Part 2: Till Geological Survey of Finland Espoo 1992
- Kontas , E. 1981 . Rapid determination of gold by flameless atomic absorption spectrometry in the ppb and ppm ranges without organic solvent extraction . Atomic Spectroscopy , 2 : 59 – 61 .
- Kontas , E. , Niskavaara , H. and Virtasalo , J. 1990 . Gold, palladium and tellurium in South African, Chinese and Japanese geological reference samples . Geostandards Newsletter , 14 : 477 – 478 .
- Kontinen , A. , Sorjonen-Ward , P. , Peltonen , P. and Kuronen , U. Some new constraints on hydrothermal alteration and deformation of the Paleoproterozoic serpentinite-hosted Outokumpu Cu – Co – Ni – Zn – Au deposits, Finland . Mineral Deposit Research: Meeting the Global Challenge: Proceedings of the Eighth Biennial SGA Meeting, Beijing, China, 18 – 21 August 2005 . Edited by: Mao , J. and Bierlein , F. P. Berlin : Springer . vol. 1, pp. 639 – 642
- Korsman K. Koistinen T. Kohonen J. Wennerström M. Ekdahl E. Honkamo M. Idman H. Pekkala Y. Bedrock Map of Finland 1:1 000 000 Geological Survey of Finland Espoo 1997
- Lahtinen , R. , Korja , A. and Nironen , M. 2005 . “ Palaeoproterozoic tectonic evolution ” . In Precambrian Geology of Finland—Key to the Evolution of the Fennoscandian Shield (Developments in Precambrian Geology 14 ) , Edited by: Lehtinen , M. , Nurmi , P. A. and Rämö , O. T. 481 – 532 . Amsterdam : Elsevier Science .
- Lehtonen , M. 2005 . Kimberlites in Finland: Information about the Mantle of the Karelian Craton and Implications for Diamond Exploration , Espoo : Geological Survey of Finland .
- Luo , X. and Dimitrakopoulos , R. 2003 . Data-driven fuzzy analysis in quantitative mineral resource assessment . Computers and Geosciences , 29 : 3 – 13 .
- Moon , W. M. 1990 . Integration of geophysical and geological data using evidential belief function . Institute of Electric and Electronic Engineering Transactions on Geoscience and Remote Sensing , 28 : 711 – 720 .
- Niiranen , T. 2005 . Iron Oxide – Copper – Gold Deposits in Finland: Case Studies from the Peräpohja Schist Belt and the Central Lapland Greenstone Belt , Helsinki : University of Helsinki . Academic Dissertation. Publications of the Department of Geology D6
- Niiranen , T. , Hanski , E. and Eilu , P. 2002 . General geology, alteration, and iron deposits in the Palaeoproterozoic Misi region, northern Finland . Geological Society of Finland Bulletin , 75 : 69 – 92 .
- Niiranen , T. , Poutiainen , M. and Mänttäri , I. 2007 . Geology, geochemistry, fluid inclusion characteristics, and U – Pb age studies on iron oxide – Cu – Au deposits in the Kolari region, northern Finland . Ore Geology Reviews , 30 : 75 – 105 .
- Nykänen , V. , Salmirinne , H. , Hyvönen , E. , Hulkki , H. and Airo , M. Fuzzy logic spatial modelling as targeting tool for orogenic gold mineralization in the Central Lapland greenstone belt, Northern Fennoscandian Shield, using high-resolution multi-element airborne geophysical data . Symposium 2005. Window to the World. Symposium Proceedings . Edited by: Rhoden , H. N. , Steininger , R. C. and Vikre , P. G. Reno : Geological Society of Nevada . vol. II, pp. 1159 – 1167
- Nyström , J. O. and Henriquez , F. 1994 . Magmatic features of iron ores of the Kiruna-type in Chile and Sweden: ore textures and magnetite geochemistry . Economic Geology , 89 : 820 – 839 .
- O'Brien , H. E. , Peltonen , P. and Vartiainen , H. 2005 . “ Kimberlites, carbonatites, and alkaline rocks ” . In Precambrian Geology of Finland—Key to the Evolution of the Fennoscandian Shield (Developments in Precambrian Geology 14 ) , Edited by: Lehtinen , M. , Nurmi , P. A. and Rämö , O. T. 605 – 644 . Amsterdam : Elsevier Science .
- Oreskes , N. and Einaudi , M. T. 1990 . Origin of rare earth element-enriched hematite breccias at the Olympic Dam Cu – U – Au – Ag deposit, Roxby Downs, South Australia . Economic Geology , 85 : 1 – 28 .
- Oreskes , N. and Einaudi , M. T. 1992 . Origin of hydrothermal fluids at Olympic Dam: preliminary results from fluid inclusion and stable isotopes . Economic Geology , 87 : 64 – 90 .
- Peltonen , P. 2005 . “ Ophiolites ” . In Precambrian Geology of Finland—Key to the Evolution of the Fennoscandian Shield (Developments in Precambrian Geology 14 ) , Edited by: Lehtinen , M. , Nurmi , P. A. and Rämö , O. T. 237 – 278 . Amsterdam : Elsevier Science .
- Peltonen , P. , Mänttäri , I. , Huhma , H. and Kontinen , A. 2003 . Archaean zircons from the mantle: the Jormua ophiolite revisited . Geology , 31 : 645 – 648 .
- Pollard , P. J. 2000 . “ Evidence of a magmatic fluid and metal source for Fe-oxide Cu – Au mineralization ” . In Hydrothermal Iron Oxide Copper – Gold & Related Deposits: A Global Perspective , Edited by: Porter , T. M. 27 – 41 . Adelaide : PCG Publishing .
- Pollard , P. J. 2006 . An intrusion-related origin for Cu – Au mineralization in iron oxide-copper – gold (IOCG) provinces . Mineralium Deposita , 41 : 179 – 187 .
- Porwal , A. , Carranza , E. J. M. and Hale , M. 2003 . Knowledge-driven and data-driven fuzzy models for predictive mineral potential mapping . Natural Resources Research , 12 : 1 – 25 .
- Porwal , A. , Carranza , E. J. M. and Hale , M. 2004 . A hybrid neuro-fuzzy model for mineral potential mapping . Mathematical Geology , 36 : 803 – 826 .
- Puustinen , K. and Kauppinen , H. 1989 . “ The Siilinjärvi Carbonatite Complex, Eastern Finland ” . In Phosphate Deposits of the World. Vol. 2: Phosphate Rock Resources , Edited by: Notholt , A. J. G. , Sheldon , R. P. and Davidson , D. F. 394 – 397 . Cambridge : Cambridge University Press .
- Raines , G. L. and Bonham-Carter , G. F. 2006 . “ Exploratory spatial modelling: demonstration for Carlin-type deposits, central Nevada, USA, using Arc-SDM ” . In GIS for the Earth Sciences Edited by: Harris , J. R. 23 – 52 . Geological Association of Canada Special Publication 44
- Raines , G. L. , Bonham-Carter , G. F. and Kemp , L. D. 2000 . Predictive probabilistic modelling using Arcview GIS . ArcUser , 3 : 45 – 48 .
- Reddy , R. K. T. and Bonham-Carter , G. F. 1991 . A decision-tree approach to mineral potential mapping in Snow Lake area, Manitoba . Canadian Journal of Remote Sensing , 17 : 191 – 200 .
- Rogge , D. M. , Halden , N. M. and Beaumont-Smith , C. 2006 . “ Application of data integration for shear-hosted Au mineralisation potential modelling: Lynn Lake Greenstone Belt, northwestern Manitoba, Canada ” . In GIS for the Earth Sciences Edited by: Harris , J. R. 191 – 210 . Geological Association of Canada Special Publication 44
- Salminen R. (Editor) 1995. Geochemical mapping in Finland in 1982 – 1994. Geological Survey of Finland Report of Investigation 130 (in Finnish with English summary)
- Salonen , V.-P. 1986 . Glacial transport distance distributions of surface boulders in Finland . Geological Survey of Finland Bulletin , 338
- Saltikoff , B. and Tarvainen , T. 1990 . “ Explorational data bases at the Geological Survey of Finland ” . In Computer Applications in Resource Estimation—Prediction and Assessment for Metals and Petroleum , Edited by: Gaál , G. and Merriam , D. F. 409 – 420 . Oxford : Pergamon Press .
- Tangestani , M. H. and Moore , F. 2002 . The use of Dempster – Shafer model and GIS in integration of geoscientific data for porphyry copper potential mapping, north of Shahr-e-Babak, Iran . International Journal of Applied Earth Observation and Geoinformation , 4 : 65 – 74 .
- Tangestani , M. H. and Moore , F. 2003 . Mapping porphyry copper potential with fuzzy model, northern Shahr-e-Babak, Iran . Australian Journal of Earth Sciences , 50 : 311 – 317 .
- Tsoukalas , L. H. and Uhrig , R. E. 1997 . Fuzzy and Neural Approaches in Engineering , New York : Wiley .
- Wanhainen , C. , Billström , K. , Martinsson , O. , Stein , H. and Nordin , R. 2005 . 160 Ma of magmatic/hydrothermal activity in the Gällivare area: Re – Os dating of molybdenite and U – Pb dating of titanite from the Aitik Cu – Au – Ag deposit, northern Sweden . Mineralium Deposita , 40 : 435 – 447 .
- Weihed , P. , Arndt , N. , Billström , K. , Duchesne , J.-C. , Eilu , P. , Martinsson , O. , Papunen , H. and Lahtinen , R. 2005 . Precambrian geodynamics and ore formation: the Fennoscandian Shield . Ore Geology Reviews , 27 : 273 – 322 .
- Williams , P. J. , Barton , M. D. , Johnson , D. A. , Fontboté , L. , de Haller , A. , Mark , G. , Oliver , N. and Marschik , R. Iron oxide copper – gold deposits: geology, space – time distribution, and possible modes of origin . Economic Geology 100th Anniversary Volume . pp. 371 – 405 .
- Zadeh , L. A. 1965 . Fuzzy sets . Institute of Electric and Electronic Engineering, Information and Control , 8 : 338 – 353 .