Abstract
A set of reusable ontologies has been developed as separate components within a knowledge representation (KR) system, to generically model a reef system. The ontology design, ranging from light to heavyweight, aims to leverage from the scalable characteristics of semantic technologies to allow for flexibility when posing domain and locality-specific hypotheses, such as predicting coral bleaching. The Semantic Reef Project is an eco-informatics application designed to test ecological hypotheses to derive information about environmental systems. The intention is to develop an automated data processing, problem-solving, and knowledge discovery system that will assist in developing our understanding and management of coral reef ecosystems.
Remote environmental monitoring (including sensor networks) is being widely developed and used for collecting real-time data across widely distributed locations. As the volume of raw data increases, it is envisaged that bottlenecks will develop in the data analysis phases because current data processing procedures still involve manual manipulation and will soon become unfeasible to manage. Ontologies provide a new approach and methodology for modulating this data overflow while also improving our ability to extract knowledge from the data collected.
INTRODUCTION
Eco-informatics is an emerging branch of e-Research whereby new information technology (IT) techniques and infrastructure are being developed to give ecologists far greater scope for problem-solving at otherwise impossible scales and with datasets of a size not previously available. With the predicted impact of climate change, and other environmental issues, these new cross-discipline tools are becoming crucial.
Modern data gathering methods have seen the emergence of highly connected and efficient scientific instruments that produce vast quantities of data, sometimes referred to as a “data deluge” (Hey and Trefethen, Citation2003). In the earth and marine sciences, environmental sensor networks are being deployed to gather data in real-time, across widely distributed areas, for applications such as environmental and seismic monitoring. The Integrated Marine Observing System (IMOS) – Great Barrier Reef Oceans Observing System (GBROOS) is one such infrastructure that uses sensor networks to remotely monitor chosen sites on the Great Barrier Reef (GBR) (IMOS, Citation2008). When fully implemented, it is expected GBROOS and similar sensor network deployments, will produce vast amounts of real-time streaming data from a variety of domain-specific sensors, including meteorological, chemical, and biological. This incalculable amount of sensed data is necessary in order to produce the new information needed to answer complex questions and expand knowledge that is vital for environmental management.
The Semantic Reef Project is an application of eco-informatics where collaborations between disparate disciplines and expertise have been effectively combined. This project aims to streamline the data analysis of remotely sensed data from the GBR. A primary goal is to balance the inevitable growth in sensed data streams with the capacity of individual researchers to benefit from the volume of data available. Currently, the platform combines emerging semantic web and scientific workflow technologies to access and infer information from unconnected datasets. On completion, the semantic reef model will produce a tool for hypothesis-driven research and problem-solving methods, allowing for more efficient investigation methods of disparate data streams. Ultimately, through coupling the disparate data to the ecological and environmental ontologies described herein, querying and deriving inferences may provide alerting for unusual domain-specific events.
One objective of the semantic reef is to improve the capacity to generate timely warnings of environmental conditions that are conducive to coral bleaching. A hierarchy of reusable lightweight to heavyweight domain ontologies and usable application ontologies has been developed to present marine scientists, from around the globe, tools to test hypotheses and probabilities through enlisting a semantic “richness on demand” environment. The level of granularity required, or the depth of the analysis, is flexible, scalable by design, and independent of location, reef type, or standing stock. The design purposefully separates the functionality and relationships, which are emulated in the integrated logic systems. In particular, it separates the description logics (DL) and propositional logics from the instance data that make up a reef ecosystem. Thus, the modularity permits changes of minor and major entities within the model to be made trivially, effectively supporting future propositions, queries, and even changes in the relationships themselves.
In this article, the domain-specific problems relating to reef ecosystems are described with particular attention to coral bleaching. The methodologies specific descriptions and justifications employed to create the hierarchy of ontologies that make up the knowledge base and detailed exemplar applications are presented. In conclusion, key lessons learned and their implication for future and related work is considered.
THE CORAL REEF DOMAIN
Globally, marine ecosystems face many pressures from both natural and human-induced stresses, and managing these threats requires major scientific insight and management coordination on a global scale. Figure depicts a domain expert's perspective of the core semantic constituents required to make up any arbitrary coral reef (Davey et al., Citation2008).
FIGURE 1 Identifying any coral reef – semantic building blocks (Davey, Holmes, and Johnstone, Citation2008).
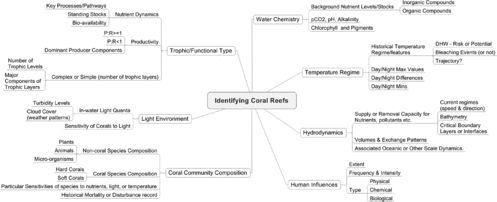
According to the Australian Institute for Marine Science (AIMS), threats to Australia's coral reefs fall into three categories (AIMS, Citation2007):
natural stresses that they have evolved to cope with for millions of years; | |||||
direct anthropogenic pressures, including sediment and nutrient pollution from land run-offs, over-exploitation, and damaging fishing practices, engineering, and modification of shorelines; | |||||
global climate change or variability. |
Through studying the manifestations of global climate change in coral reefs, such as increased coral bleaching and coral disease, it has become clear that many threats are closely linked and may exacerbate each other (AIMS, Citation2008).
The Great Barrier Reef is the largest continuous coral reef ecosystem in the world and is a large contributor to Australia's economy through fishing and tourism activities. Covering an area of approximately 348,000 square kilometers, and spanning ∼2300 kilometres of Australia's northeastern coast, the GBR is sometimes referred to as the single largest organism in the world; although in reality it is made up of many billions of tiny coral formations (GBRMPA, Citation2006). There are a number of major reef management and research facilities located in close proximity to the GBR. This proximity has allowed the authors convenient access to domain expertise when creating a use-case for trialing the semantic reef system. Furthermore, the outcome of a particular test, for example forecasting a coral bleaching event or a crown-of-thorns outbreak, provides material that can be directly assimilated as a useful tool for supporting management decisions on the GBR.
The collection of oceanographic and marine data is a complex and expensive process requiring significant scientific and technical infrastructure. In addition the decisions about where and when to collect data are also problematic. Accordingly, it is important that the collection is made of all data collected and that the data is made available for subsequent reuse.
It is important to note that coral reef studies are highly disciplinary and significant data reuse occurs in the literature. Similarly, the range of problems currently facing coral reefs is necessitating the use of larger multi-disciplinary datasets and knowledge-building in the search for solutions. Many of the most influential articles in coral reef science of the past few years have been “synthesis” articles aggregating long-term observations into new hypotheses and conclusions. One well-known example of this new class of science would be the recent Intergovernmental Panel on Climate Change reports (IPCC) (IPCC, Citation2007).
The Coral Bleaching Phenomena
The impact of climate change on the GBR threatens this iconic wonder—climate change-induced warming of ocean temperatures is a major contributor to the coral bleaching phenomena (Hughes et al., Citation2003). Corals live in a symbiotic relationship with single-celled algae called zooxanthellae which live within the coral's tissue at very high densities. Coral bleaching results from a stress condition in coral that induces a breakdown of the symbiotic relationship between coral and zooxanthellae.
The long-term survival of both organisms is reliant on this symbiosis. The zooxanthellae reside in the coral animal's tissue and in exchange they provide energy-rich sugars via photosynthesis, and some nutrients that augment other potential food sources for the coral. Also, the photosynthetic pigments from the algae give corals their brilliant colors. Reef corals have a distinct thermal range for growth and survival such that they become stressed and may die when this is exceeded. When water temperatures exceed the upper thermal tolerance value for a species in a given habitat it can lead to stress, which causes the coral to expel the zooxanthellae leaving the white skeleton of the coral exposed, giving it the bleached white appearance. Bleaching is potentially fatal depending on the duration of the stress and the occurrence of other simultaneous influences such as light intensity, nutrients, sediments, and pollutants (Hughes et al., Citation2003).
BACKGROUND – THE SEMANTIC REEF PROJECT
The Semantic Reef Project is a platform designed as a hypothesis tool for research on reef ecosystems (Myers, Atkinson, and Lavery, Citation2007). This knowledge representation (KR) system employs semantic web methodologies and scientific workflow tools to access a range of datasets for automated processing (Figure ). The data is routed through the workflow and mapped to the ontologies contained in the knowledge base for querying and/or hypothesis testing. Ultimately, this system will assist in the automated synthesis and analysis of autonomously collected streams of data, for example, the volumes of data being produced by remote observing instruments. Our initial trials have involved first validating and then demonstrating the system.
To test and validate the semantic reef system, datasets were needed along with a well-researched topic. The coral bleaching problem was an appropriate test case to ground-truth the architecture, as the datasets relevant to the historic findings, and observations by experts in the field, were readily available. The validation entailed a reverse-hypothesis methodology that ground-truthed the knowledge base against known historic events, specifically the 1998 and 2002 mass bleaching occurrences. Datasets used in the prior research were imported to the knowledge base to hind-cast coral bleaching events using common bleaching indices, fashioned as inference rules or queries, to be compared to the actual historic outcomes (Myers, Atkinson, and Maynard, Citation2007). Then, expanding on the validation process, current data was used to predict a potential bleaching event.
The Semantic Web Component
Semantic web technologies allow for a flexible scalable environment to model worldly concepts in a way that is “understandable” to the machine (Antoniou and van Harmelen, Citation2004). Ontologies are the foundation of these technologies, and within the context of this article, are descriptions to represent both abstract and specific concepts. Each domain's vocabulary and the terms that describe the entities within (and the relationships they have with each other), are defined using axioms and restrictions that constrain the interpretation for use by the computer, making the concept machine-processable (Guarino, Citation1997).
Once data and information are interpretable by a machine Semantic technologies enable the machine to make intelligent decisions, based on conclusions derived through logic systems such as DLs and inference rules (Antoniou and van Harmelen, Citation2004).
Description logics are sets of logical statements to describe relationships and parameters of a concept. They can be used to reason over classes and individuals to infer equivalencies, assumptions, and subsumptions; for instance, between the reef communities standing stocks (e.g., Acropora from one database is equivalent to Staghorn coral in another). The DL reasoning engines used in this project are Pellet,Footnote 1 Racer PROFootnote 2 and FaCT+ + Footnote 3 as implemented through the Protégé ontology editors (Protégé, Citation2009).
An environment that supports suppositions is enabled with utilities such as the semantic web rules language (SWRL) and the SWRL query language (SQWRL pronounced “squirrel”). The SWRL manages inference using horn-like rules, which is a subset of predicate logic (first-order logic), and is orthogonal to description logic. The SWRL rules can define the hypotheses posed by marine researchers, as many hypotheses can be fashioned in the syllogistic format of a Horn clause. The SWRL rules are passed to the Jess inference engineFootnote 4 for inferring over the ontology instances, directly or using the “SWRL to Jess bridge” component of Protégé (O'Connor et al., Citation2005).
The SQWRL queries are used when there is a need for aggregation or selecting and counting functionality. The SWRL only supports monotonic rules and therefore cannot be used to modify information in an ontology, such as removing a value or updating a current value (commonly required for aggregation). If a SWRL rule modifies the current axioms already defined in an ontology, nonmonotonicity would ensue (O'Connor et al., Citation2007). Aggregation operators are problematic as they are not DL-safe constructs, so they cannot be used as antecedents in SWRL rules. The SQWRL is a query language that operates over OWL forms and is based on the notion of DL-safe rules (Motik et al., Citation2005).
The Web Ontology Language (OWL), which is the W3C's recommended specification (McGuinness and van Harmelen, Citation2004), has three different varieties: OWL Lite, OWL DL, and OWL Full, each providing different levels of logical expressiveness. All inferences available in an OWL-Lite or OWL DL ontology can be computed using the reasoning engine because the logical semantics of OWL DL (and Lite, which is a subset of DL) are based on DL. In contrast, ontologies in OWL Full are not decidable, and have insufficient application reasoning support available. Therefore, the ontology design within this project was maintained in OWL DL or Owl Lite to maximize the reasoning and inference functions of the logic systems.
The Scientific Work Flow Component
To date there have been few examples of semantic knowledge bases being connected to streaming data services. This is a consequence of the software implementations and not intrinsic to this model. For this purpose, the Kepler/Ptolemy workflow engine was employed to access streaming data services and populate the KR system (Atkinson et al., Citation2007). Scientific workflow technologies and tools, such as the Kepler system used here, are adaptive software programs to capture complex analyses in a flow of which the data is taken through one analytical step after another (Altintas et al., Citation2004). In the Kepler system each of these workflow steps are represented by an “actor.” The workflow actors provide access to the continually expanding amount of geographically distributed data repositories, computing resources, and workflow libraries and also compute menial operations such as determining averages or data manipulation prior to populating the knowledge base.
ONTOLOGIES TO REPRESENT ANY REEF ECOSYSTEM
The Importance of the Open World Assumption
Semantic technologies support the open world assumption (OWA), which allows for added flexibility to model concepts that can conceivably change, such as an ecosystem. The OWA means that what cannot be proven to be true is not automatically false; it assumes that the knowledge of the world is incomplete (Horrocks, Patel-Schneider, and van Harmelen, Citation2003). Therefore, what is not stated is considered unknown, rather than wrong; it simply assumes the extra information needed has not, as yet, been added to the knowledge base (Rector et al., Citation2004).
Science, in general, moves forward by maintaining an OWA, that is, nothing is false until explicitly proven thus. Traditional database implementations such as relational databases are required to maintain a closed world assumption, that is, everything that is known about the world exists within the boundaries of the database. This creates a mismatch between the researchers that needs to remain open to new discoveries and the current technological capabilities for flexibility and scalability (Page, Citation2008). When there is a change in relationships within a conceptual world due to a new discovery or fact, which is quite feasible in a marine biology domain, modifications to these relationships can be easily accomplished in OWL ontologies, as they are designed with an OWA.
In the marine biology domain, there is a great need for multiscalability. As the relationships within an ecosystem can change depending on research findings and/or defeasible current knowledge, the capacity for flexible modifications in lieu of new developments is very important. The OWA allows for a certain degree of flexibility when applying queries across domains where all is not explicitly proven knowledge. An OWA is considered implicit, that is, every tuple not explicitly contained in the ontology is implicitly assumed to represent a fact that is unknown, rather than false.
The Design Methodology
Concepts can be modelled through ontologies in a variety of ways and varying degrees of granularity. The most common types of ontologies are the general or common ontologies, top-level or upper-level ontologies, domain ontologies, task ontologies, domain-task ontologies, method ontologies, and application ontologies all serving different functions. The type of ontology to build is determined purely by the extensibility and expressiveness required, in conjunction with what information or knowledge the ontology is designed to produce. The more complex the ontology, the more prone it is to inconsistencies and the higher the restriction on flexibility and reusability independent of applications (Gomez-Perez, Corcho, and Fernandez-Lopez, Citation2004).
The semantic reef knowledge base consists of a hierarchy of ontologies, from lightweight through to heavyweight, in a modular design that is developed to describe coral reef ecosystems (Figure ). There are numerous published ontology engineering methodologies available to assist in the representation of knowledge. The choice of methodology or a combination of methodologies is dependent on the purpose of the knowledge base (Gomez-Perez et al., Citation2004; Corcho, Fernández-López, and Gómez-Pérez, Citation2003). The aim of the semantic reef ontological architecture was to maximize reusability and usability simultaneously through the separation of concept definitions, instance data, and rules.
A hybrid of design methodologies was adopted to create the knowledge base. The main elements include the seven step knowledge engineering methodology (Noy and McGuinness, Citation2001), Uschold and King's (Citation1995) three strategies to indentify concepts and the developing ontology-grounded methods and applications (DOGMA) approach (Jarrar and Meersman, Citation2008). The first and second are a generic set of guidelines to construct ontologies of any concept and were used for the intraontological development. The third offers a strategy that focuses on ontology reusability verses ontology usability and was adopted for the interontological development process employed here.
The Intraontology Development Methodology
The seven step ontology development guideline is a methodology for creating ontologies based on declarative knowledge representation systems (Noy and McGuinness, Citation2001). The systematic steps begin with the declaration of the scope and domain vocabulary and finish with the creation of classes, properties, property restrictions, and instances. As the individual ontologies were developed from the functional coral reef model in Figure , the steps in this method allowed for collaboration with domain experts.
The class hierarchies of the individual ontologies were developed using both middle-out and top-down approaches. The three strategies for identifying the main concepts in an ontology are: the top-down approach, which identifies concepts from the most generalized to the most specific; the bottom-up approach, which is the opposite (i.e., most concrete to the most abstract); and the middle-out approach, where the most relevant concept is identified first and then generalized and specialized (Uschold and King, Citation1995; Uschold and Gruninger, Citation1996).
The approach chosen to model the separate ontologies was dependant on the domain knowledge and the complexity of the reef ecosystem concept. For example, a top-down approach was taken when developing the “reef” plant and animal taxonomy because cataloguing from kingdom to species in both scientific and common names affords a natural rising. Other ontologies were designed using the middle-out approach, for instance, the “human influence” ontology, where the biological, chemical, or physical influence could be declared and systematically specialized (type, frequency, and intensity) and generalized (deliberate or accidental).
The Interontology Development Methodology
The DOGMA strategic approach was adopted for the interontology development. DOGMA is a methodological ontology engineering framework that divides an ontology into two specific axiomatization concepts: domain and application axiomatizations (Jarrar and Meersman, Citation2008). The domain axiomatization is concerned with characterizing the respective meaning of the domain vocabulary; in contrast, the application axiomatization is concerned with how the vocabularies are to be used. Specifically, the more emphasis put on application perspectives, the higher the usability and the more emphasis on independence from application requirements the higher the reusability.
The philosophy of the DOGMA methodology was adaptable to the goals of the semantic reef ontology base. According to the DOGMA approach, the separation of the domain knowledge from the application or domain tasks would maximize reuse and usability. Therefore, the ontologies within the semantic reef architecture are imported from the lowest layers upwards with two distinct levels: the reusable domain ontologies and the usable domain task and method ontologies (Figure ).
The implementation of the DOGMA philosophy effectively separated the domain (reusable) ontologies (i.e., the coral reef ontology) from the higher application (usable) domain-task ontologies (i.e., the specific reef hypotheses) (Figure ). The reusable components of the ontology base consist of the “Coral Reef” generic ontology, which imports all lower ontologies and maintains OWL DL axioms for richer relational descriptions that are indicative of any coral reef. A domain-task ontology (e.g., GBR.owl, Moorea.owl, Bahamas.owl, etc.) would import the “Coral Reef” ontology and be populated with instance data pertinent to the specific reef system and hypothesis. For example, posing questions of the sensor data fed from the GBROOS Davies Reef weather station on the GBR or the Coral Reef Environmental Observatory Network (CREON) group conducting research in the French Polynesians. The elements and classes of the generic “Coral Reef” domain ontology would be the same for either location; however, the instance data and rules of the application ontologies, at the usable level of the hierarchy, would differ (i.e., the “GBR” and “Moorea Island” application ontologies, respectively).
The resulting hierarchical formation of the knowledge base is a bottom-up physical architecture (Figure ), not to be confused with Uschold and King's (Citation1995) strategy for design. The physical flow consists of the lower lightweight ontologies being imported to the more complex DL ontologies and then the task-specific application ontologies employ the reusable coral reef ontology base beneath, where finally, finely detailed inference rules can be written to the system as propositions to infer conclusions about a specific issue on a particular reef, regardless of location (e.g., a coral bleaching watch on the GBR or coral spawning events on the Moorea Island reefs).
The Ontologies
The reuse of existing ontologies was considered and applied where appropriate. Initially, the strategy here was to structure the semantic reef knowledge base on the model supplied by the domain experts (Davey et al., Citation2008). The functional coral reef model (Figure ) was utilized as semantic “building blocks” when applying the methodological steps to build the intra- and inter-ontology framework. To date, the temporal ontology developed at Stanford University for use in the SWRL rules is implemented (O'Connor, Shankar, and Das, Citation2006) and the future development of the ontology knowledge base will incorporate other common ontologies for integration to the system.
Currently, the individuals of the knowledge base are temporal instances of a reef, with quantifiable data asserted for that moment in time and place. Therefore, data-type properties are the appropriate choice in expressing the quantifiable values of the environmental elements (e.g., SST, pH, etc.). Many pre-existing ontologies were not suitable for usage at this stage of development. For example, the common ontologies investigated to describe quantifiable concepts such as units of measurement express the variety of units as individuals and not data-type properties (NASA, Citation2009; SEEK, Citation2009).
The Lightweight Ontologies
The base level “reef” ontology is designed for maximum reusability. It describes a taxonomy written in OWL-Lite that lists the plant and animal vocabulary of the coral reef domain in both scientific and common names. The only constraints used in this ontology are the equivalency and subclass axioms, which explicitly state the equality relationship and taxonomic hierarchy between the phylum and family names with the common names. Adding in the structured scientific names and the common names is required for flexibility when using disparate datasets to populate the knowledge base with standing stock. That is, accessing prewritten species databases that are listed in different perspectives (i.e., common names versus phylum, etc.) will be inconsequential, as the reasoning engine will subsume instances to multiple superclasses. For example, “Scaridae” in one database, such as the CSIRO Data Centre (CSIRO, Citation2008) might be “parrotfish” in another, where upon classification, the instances will belong to both classes automatically. The end users perspectives, needs, and knowledge of the domain, and the types of queries and inferences required, could be vastly different or unknown, at this time; therefore it is important to bridge disparate schemas.
Organizations exist where the primary focus is the standardization of data and metadata produced in marine research. A seminal example is the Marine Metadata Interoperability (MMI) project, which is a National Science Foundation (NSF) funded initiative that aims to “promote the exchange, integration and use of marine data through enhanced data publishing, discovery, documentation and accessibility” (Stocks et al., Citation2009). The MMI lays a foundation, via a comprehensive set of guides, for scientists and data managers to follow when creating metadata. The MMI site hosts a list of accessible controlled vocabularies pertaining to biological taxonomies concepts and there are e-Science endeavors to defragment the biological taxonomies that are currently spread both online and through ink-on-article publications (Page, Citation2006; Clark et al., Citation2009). Currently, there are millions of biological names indexed by the Universal Biological Indexer and Organiser (uBio),Footnote 5 for example, over 1,140,000 in the Animalia Kingdom alone. In Fishbase,Footnote 6 a global information system about fish, there are 31,100 species and 276,100 common names logged. A noncomprehensive list of species was declared to form the controlled vocabulary as a sample of the available taxonomies. To date, there are 360 classes of scientific and common names, which is extendable with simple additions to the KB.
The three main quantifiable environment elements are the temperature regime, water chemistry, and light quanta. To describe factors that are significant to these environmental regimes requires only simple data-type properties (i.e., integer, floats, strings, etc.) that state, for example, Sea Surface Temperature (SST), humidity percentile, pH level, among others. The elements are defined in the informal OWL Lite ontologies in order for the Kepler workflow to populate with historic or real-time data, for instance, SST taken from the GBROOSFootnote 7 sensors or salinity from the Australian Bureau of Meteorology's BLUElink,Footnote 8 at a given time stamp.
The Heavyweight Ontologies
To describe the intricate, multiscale relations involved in a coral reef ecosystem, such as human influences or the trophic layers, OWL-DL was used. There are many principles within the semantic technology standards that must be adhered to in order to employ all of the complex logic levels available within the languages and current tools. For example, if an ontology is to remain straightforward, for reasons of reusability, without the added complexity of the logic systems, it is prudent to maintain a lightweight or informal ontology (i.e., written using RDF or OWL-Lite). However, due to the limitations in expressiveness available at this knowledge representation level, the reasoning capacity of the classifiers is diminished. Richer descriptions of concepts, using axiomizations and quantifications, is made available in DL and used by the classifiers to automate the inferred subsumption of classes and/or instances.
The “Human Influence” ontology illustrates one such complex ontology. The development of an ontology to describe human influences on coral reefs was not a trivial task, especially when designing to maximize scalability and flexibility for future unknown queries, propositions, or additions. Coral reefs can be affected by a variety of human influences, which can be categorized as biological, physical, or chemical. The “Human Influence” ontology was fashioned as a matrix consisting of occurrences and the levels of severity for each of these influence types. The main factors of each influence type are intensity, frequency, and extent, which were expressed so each instance could be categorized and subsumed to a degree of extent from minimal to maximal severity.
Specifically, the “Intensity,” “Frequency,” and “Influence_Type” classes of the “Human Influence” ontology were created as enumerated classes. Where “Intensity” and “Frequency” were filled with individuals from low to high, and the “Influence_Type” class was filled with predefined individuals of biological, physical, and chemical. Subsequently, the minimal to maximal affects were created as subclasses of “Influence_Extent,” where class axioms of necessary and necessary and sufficient conditions were explicitly stated to allow for the automated reasoning. For example, to belong to the class “Chemical_Biological_Affect_Bad,” a subclass of “Maximal_Affect” the conditions state:
Chemical_Biological_Affect_Bad ⊑ hasHumanInfluence ∃ Biological_Influence hasHumanInfluence ∃ Chemical_Influence hasHumanInfluence ∀ (Biological_Influence ⊔ Chemical_Influence) hasInfluenceType ∋ Biological hasInfluenceType ∋ Chemical hasFrequencyOf ∋ High_Frequency HasIntensityOf ∋ High_Intensity
On populating the Influence class with instances, properties such as “hasIntensityOf” and “hasFrequencyOf” are filled, dependant on the preprocessing triggers from the Kepler workflow (e.g., a highly frequent value can be determined as the data is presented in real-time). Therefore, following classification by the reasoning engine, all influence instances (such as a dredging, scientific experiment, pollution, flumes, etc.) will be automatically categorized to the severity of the affect.
Knowledge Base Statistics
Currently the Semantic Reef KB is comprised of the reusable domain ontology (Coral Reef) and domain-task ontology for the GBR (Coral Reef GBR) and the current statistics for these ontologies is depicted in Table .
TABLE 1 Current Statistics for the Reusable and Usable Ontology Base Used to Demonstrate Hypothesis Testing on the GBR
USE CASE EXAMPLES
The Validation of the Semantic Reef Architecture Using Thermal Stress Indices
In the late summer (February and March) of 1998 and 2002, two mass bleaching events occurred in the GBR and Coral Sea off the coast of Australia. The historical records of the remotely sensed SST were measured by Advanced Very High Resolution Radiometer (AVHRR) from the satellite platforms of the U.S. National Oceanic and Atmospheric Administration (NOAA) (Gleeson and Strong, Citation1995) and the historic analyses conducted using these records were to determine the extent to which bleaching severity could be attributed to the different measures of thermal stress.
The work and studies carried out on these events offered relevant building blocks and information in which to populate the ontologies used herein. In particular, the stress factor most commonly associated with bleaching of elevated sea surface temperature (Marshall and Schuttenberg, Citation2006), so data was acquired through the Great Barrier Reef Marine Park Authority (GBRMPA) for use in the initial validation test.
Two common metrics currently used to forecast possible bleaching events include, among others, the SST anomaly (SST+) and Degree Heating Days (DHD) (Berkelmans, De'ath, Kininmonth, and Skirving, Citation2004; Maynard et al., Citation2008). They are as follows: where SST +is calculated as the number of°C above the long-term mean summer temperature (LMST) for that location and a DHD describes the accumulation of thermal stress; where one DHD is calculated as one degree above the local LMST for 1 day and two DHDs is either two degrees above the local LMST for 1 day, or one degree above LMST for 2 days and so forth.
The validation entailed a reverse-hypothesis method that ground-truthed the outcome of the system against actual historical events. The SWRL rules were created to simulate the SST+ metric and infer a bleaching event based on the degree of the anomaly. The SST anomaly ranges from category one to category five depending on the number of degrees above LMST. Therefore, the antecedents of the rules compared the daily average SST against the LMST and inferred any temporal instance belonging to a class of bleach watch categories. The SQWRL queries were required to determine the summer DHDs because aggregation was necessary for this metric to sum up the summer anomaly values.
The results from the rules and the queries were compared to the data and research from the 1998 and 2002 mass bleaching events (Myers, Atkinson, and Maynard, Citation2007). The coral reef instances that had been inferred, through rules, to belong to a particular categorical bleach alert class, and the outcome of the DHD SQWRL queries were matched to the historic events to confirm accuracy. On completion of this experiment, it was found that the instances that were expected to be members of the bleach risk classes were in fact correctly allotted. For example, the individuals from late January 1998 were sent to the lower risk bleach alert classes, then as the temperature intensified through the February period these individuals were inferred to the bleach watch categories. The system can now act as an alternative method for forecasting bleach alerts based on spatial SST.
Predicting a Bleaching Event with Remotely Sensed Data
The capabilities of the semantic reef system are presently being tested to infer a coral bleach warning with current SST data. The data used includes spatial data from NOAA (Gleeson and Strong, Citation1995) and real-time SST data being streamed from the Davies Reef, Cleveland Bay, and Myrmidon Reef monitoring sites (Kininmonth et al., Citation2004). The data was available from the weather observing system, which is accessed through the AIMS data access portal. Data quality is checked via the AIMS data center processes and if there are gaps found in the data feed, representational data is substituted. Figure depicts a workflow created to stream SST data from AIMS, tag it with a URI, then map the data to the domain-specific “GBR” ontology, which includes the subclasses of “Sensor Temperature” and “Spatial Temperature” via the imported “Coral Reef” ontology. The workflow actors are then directed to populate the designated reef instances with date/time and temperature data-type property values.
The inference rules from the validation, which are based on the coral bleaching metrics SST +and DHDs, are applied to detect a problematic area. The rules infer any instances of a particular reef to belong to a categorized bleach watch class automatically, dependant on the rise in temperature above average and the susceptibility of the coral community to bleaching. To illustrate a simplified rule, written in SWRL to assess the rising SST and the presence of zooxanthellae in the coral species (i.e., hermatypic), was as follows:
The rules were applied to the recent 2008/2009 summer period and resulted in a bleach watch alert for Davies Reef. Although there were a number of warmer times during that summer period, from the 11th to the 22nd of February, Davies Reef experienced extremely high temperatures and the outcome form the rules derived a Category 2 bleaching alert.
The outcome of the inferred bleach risk instances coincided with the established coral bleaching alert systems. Specifically, the National Oceanic and Atmospheric Administration (NOAA) Coral Reef Watch's Satellite Bleaching Alert (SBA) system issued a bleaching watch alert on the 16 February 2009 (NOAA, Citation2009) for the same region.
The advantages of a semantic system extend past the ability to extract specific instances based on asserted data properties. Characteristics of semantic technologies include capabilities in data integration and automatically linking latent connections through reasoning. When the relationships are known, statistical inference is efficient and appropriate; however, when the relationships are not all known semantic inference can be used to disclose phenomena in the data. The cumulative mix of contributing factors that makes up the tipping point between a healthy reef and a bleached dead reef is just such an unknown relationship.
An Example as a Hypothesis Tool – Finding the Coral Bleaching Tipping Point
Coral bleaching is not uniform, it occurs in discreet reefs across the many that comprise the reef systems of the world. While our understanding of the causal factors of bleaching has some limitations, it is widely accepted that sea temperatures are clearly involved (Berkelmans, Citation2009). Notably however, research is still being conducted to determine why some coral species bleach in some areas but not in others, and why some corals of the same genera adapt to higher SSTs given time (Hughes et al., Citation2003).
Ecological hypothesis validation across wide geographic domains is still mostly in development stages. The KR system described here would enable such propositional testing by using SWRL inference rules. In this case, domain task ontologies are introduced, at the highest level of the hierarchy, importing all lower ontologies and effectively separating the instance data from the inference rules. Then, tasks are performed such as queries or posing hypotheses, for any reef, with any mix of standing stock composition, hydrodynamics, environmental elements, or external human influences. To easily modify or add new relational information to the ontologies is an advantage of the semantic technologies and making changes such as chemical factors like nitrogen levels, biological factors such as fresh water flumes, or new discoveries as they develop, will enable scientists to explore at levels not possible before.
The inference rules can be designed to define observational hypotheses that are in syllogistic form. The SWRL supports inductive and deductive rules written as a Horn clause, which is an appropriate form for most quantitative observational hypotheses. For instance, in exploring the unknown casual effects of a coral bleaching event, the researcher can declare the factors believed to be responsible for the occurrence as antecedents in the rule. Accordingly, inductive reasoning would then produce a conclusion that could be proven or disproved through in situ observation.
Importantly, the researcher is not required to have a predetermined hypothesis prior to data collection and populating the KB. Rather, the questions can be as flexible and may evolve as new data becomes available or as ideas grow and/or epiphanies emerge. To illustrate, the researcher may initially populate the knowledge base with sensed data and scheduled dredging activities; then the following basic inference rule checks for any instances that have a higher than average SST, critical salinity level or whether there is a human influence involved, which in this example is dredging:
Then, new rules or more information can be introduced to enable modifications in the hypotheses. Simple modifications to the antecedents within the rule, such as the SST anomaly, can change the hypothesis and disclose other temporal instances for observation. Also, introducing data to the knowledge base that is accessible from disparate sources, via the workflow, can potentially disclose phenomena and correlations in the data. For example, adding data pertaining to a reef system from disconnected sources, such as cloud cover from the Bureau of Meteorology, Chlorophyll and PAR levels from AIMS, CO2 levels from NOAA, human population from the Australian Bureau of Statistics, etc. the questions posed of the system will disclose specific temporal instances to then validate in situ.
Classifying Coral Reefs by Reef Type
Representational data and/or defined reef types are used in the different methodologies of marine research (IPCC, Citation2007). Data representation is a technique used in marine research to apply data from one reef to be indicative of other unmonitored reef systems (Maynard et al., Citation2008). The semantic reef system can automate the linking of reef-type concepts (e.g., by location, community make-up, biomass, etc.), using explicit assertions and axioms in DL. In many cases, an individual reef would belong to a number of reef types simultaneously and this classification can be automatically accomplished through reasoning. All relevant reefs that fit the specified criteria would be automatically inferred to belong to that reef class prior to running the inference rules.
Then, questions can be posed of reef types instead of individual reefs. For example, a hypothesis to draw out instances of reefs types where the environment is similar but the reef type is not or vice versa. If the outcome of this hypothesis is confirmed, then the bleaching phenomena could be predicted by the “type” of reef and not just environmental factors.
To date the “Coral Reef” ontology includes relationship axioms and classes that define certain reef types. To illustrate, the make-up of a reef type by its community composition and the sensitivity to heat stress can be described in the following statements (AIMS Data Centre, Citation2008):
Type A reef – is a reef with a high percentage of slow-growing coral and is therefore thermally tolerant, whereas, | |||||
Type B reef – is a reef with a high percentage of fast-growing coral and is therefore thermally sensitive. |
These assumptions can be defined in an ontology as “necessary and sufficient” axioms of a Type A and Type B reef (i.e., slow- and fast-growing reefs, respectively). The axioms are written as:
The Boolean data type property “isSlowGrowing” is set to true or false as a preset value for each coral species. When instances of coral species are asserted as a part of the community make up of a specific coral reef, as described by the community composition information available from GBRMPA and AIMS, the reasoning engine will subsume all coral reefs to belong to the “fast growth,” “medium growth,” or “slow growth” classes automatically (Figure ).
FIGURE 5 Reef types classified by the Pellet Reasoner to belong to the respective “reef type” model – grid location, a fast growth composition, and shelf location.
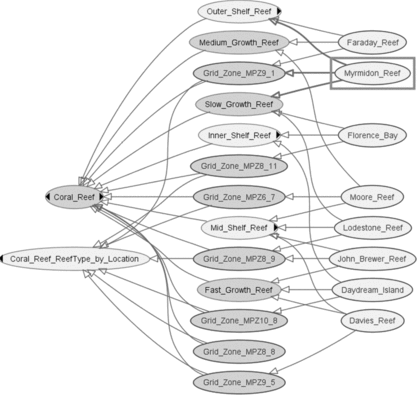
The reef type, by location, relies heavily on the range reasoning function because the proximity boundaries are defined by longitude and latitude coordinates of a gridded region in a reef system. Currently, the “GBR” domain ontology defines the reef types by grid location as classes with asserted “necessary and sufficient” property restrictions, that is, the longitude and latitude data-type properties (Figure ). On reasoning over the knowledge base, the specific reefs of the GBR are subsumed to different reef types, which effectively add other dimensions when posing questions of the system.
Development in the emerging OWL standards is extending many functional capabilities for defining concepts. Currently in OWL 1.1, there is no support for advanced data-type restrictions such as testing for a range of asserted data-type properties. In OWL 2.0, this functionality has been added and has been implemented in Protégé 4 (Protégé, Citation2009). These new developments offer richer descriptions and flexibility in restrictions over data-type properties and will add extra functionality, particularly in automating the linkages between reefs by type.
CONCLUSIONS AND FUTURE WORK
The demand for automatic data analysis and hypothesis testing is emerging in the ecological sciences. The need for autonomy grows increasingly vital as the escalating range of data-gathering devices and instruments are deployed. Presently, it is becoming increasingly difficult for individual researchers to deal with the growing amount of data available to them and, simultaneously, to be able to extract the key knowledge resources from data at a rate consummate with the need of managers. However, assimilating the mass data being gathered is vitally important for the management and research of our reef systems if they are to be resilient to, or even survive, the effects of disturbance including climate change. Coral bleaching is one impact of climate change and although the stress factor most commonly associated with bleaching is elevated sea temperature, for which there are a variety of instruments deployed to gather this spatially varied data, there are a number of other causal factors. These additional stressors, such as high light intensity, low salinity, and pollutants, are known to exacerbate coral bleaching; however, the information to confirm these findings is limited by the finite historical data available (Hughes et al., Citation2003)—hence the requirement for real-time data in combination with efficient processing tools.
The modular ontology design, depicted in Figure , used within the project may assist streamlining the data analysis phase. Here, diverse backgrounds and expertise were combined effectively in a collaboration to answer domain-specific and locality-specific hypotheses. As user-driven propositions change due to new findings, information, or queries, and new data sources become available, the open world nature of semantic technologies, in particular OWL, allows for additions and eliminations, such as new discoveries, to be trivially met.
The ontologies were created as separate concepts based on the domain experts model in Figure . The hierarchical ontology base design was adopted as clearly reefs are highly complex, interdependent ecosystems. The use of a single complex ontology would not be simple to create, implement, nor maintain and reusability would not be feasible as the number of variables and multiscale relationships in a single ontology would be immense. Instead, a modular design, that includes both intra- and inter-ontological development methodologies, was chosen with the aim of maximizing the advantages semantic web technologies offer, such as flexibility, scalability, and rich computer-understandable descriptive languages.
The Semantic Reef Project is a new approach to such data analysis and interpretation. The project is extendable to hypothesize over many issues of concern in environmental monitoring and climate change. The importance of research on these issues is vital given the sustainability, and survival of our reef ecosystems are indicators to the future of the global ecosystem (IPCC, Citation2007).
To achieve these important goals, cross-discipline collaborations are imperative. Eco-informatics applications by definition imply the binding of diverse disciplines and there are many hurdles in bridging the collective knowledge required. The obvious solution to overcoming this multidisciplinary dilemma is adopting and fostering the collaborative research model. Here, disparate backgrounds and expertise have been combined effectively, and in this case, potential end users: the GBRMPA and AIMS were involved. Notably, the development and the ultimate use of systems such as that described here foster collaboration between marine scientists and information technologists seeking to solve a common problem.
The authors wish to thank Scott Bainbridge and Stuart Kininmonth from the Australian Institute of Marine Science (AIMS) and Sally Holbrook and Russell Schmitt from the Coral Reef Environmental Observatory Network (CREON) group for their interest, helpful discussions, and feedback and the Climate Change Response group at the GBRMPA for their role. We also thank the expert reviewers for their valuable comments, recommendations, and suggestions.
Notes
http://clarkparsia.com/pellet/
http://www.racer-systems.com
http://owl.man.ac.uk/factplusplus/
http://herzberg.ca.sandia.gov/jess/
http://www.ubio.org
http://www.fishbase.org
http://www.imos.org.au/nodes/great-barrier-reef-observing-system.html
http://www.bom.gov.au/bluelink/
REFERENCES
- AIMS . 2007 . Threats to coral reefs. Australian Institute of Marine Science. http://www.aims.gov.au/docs/research/biodiversity-ecology/threats/threats.html. Last accessed January 2009.
- AIMS . 2008 . Marine Blueprint – Climate change and the fate of the Great Barrier Reef. Australian Institute of Marine Science. http://www.aims.gov.au/docs/research/climate-change/position-article.html. Last accessed January 2009.
- AIMS Data Centre . 2008 . The Future of the Reef. http://data.aims.gov.au/reefstate/sci/reefstate/. Last accessed January 2009.
- Altintas , I. , C. Berkley , E. Jaeger , M. Jones , B. Ludäscher , and S. Mock . 2004 . Kepler: An extensible system for design and execution of scientific workflows . In: Proceedings of the 16th International Conference on Scientific and Statistical Database Management (SSDBM'04) , June , Santorini Island , Greece .
- Antoniou , G. , and F. van Harmelen . 2004 . A Semantic Web Primer. Cambridge , MA : The MIT Press .
- Atkinson , I. M. , D. du Boulay , C. Chee , K. Chiu , P. Coddington , A. Gerson , T. King , D. McMullen , R. Quilici , P. Turner , A. Wendelborn , M. Wyatt , and D. Zhang . 2007 . Developing CIMA-based cyberinfrastructure for remote access to scientific instruments and collaborative e-research . In: Proceedings of the Fifth Australasian Symposium on ACSW Frontiers , January, Ballarat , Australia .
- Berkelmans , R. 2009 . Bleaching and mortality thresholds: How much is too much? In: Coral Bleaching . Berlin/Heidelberg , Germany : Springer . pp. 103 – 119 .
- Berkelmans , R. , G. De'ath , S. Kininmonth , and W. J. Skirving . 2004 . A comparison of the 1998 and 2002 coral bleaching events on the Great Barrier Reef: Spatial correlation, patterns, and predictions . Coral Reefs 23 ( 1 ): 74 – 83 .
- Clark , B. R. , H. C. J. Godfray , I. J. Kitching , S. J. Mayo , and M. J. Scoble . 2009 . Taxonomy as an eScience . Phil. Trans. R. Soc. A 367 ( 1890 ): 953 – 966 .
- Corcho , O. , M. Fernández-López , and A. Gómez-Pérez . 2003 . Methodologies, tools and languages for building ontologies: Where is their meeting point? Data Knowl. Eng. 46 ( 1 ): 41 – 64 .
- Commonwealth Scientific and Industrial Research Organisation (CSIRO) . 2008 . Divisional Data Centre, Australia. http://www.marine.csiro.au/datacentre. Last accessed March 2008 .
- Davey , M. , G. Holmes , and R. Johnstone . 2008 . High rates of nitrogen fixation (acetylene reduction) on coral skeletons following bleaching mortality . Coral Reefs 27 ( 1 ): 227 – 236 .
- Great Barrier Reef Marine Park Authority (GBRMPA) . 2006 . Australian Government. http://www.gbrmpa. gov.au/. Last accessed May 2006 .
- Gleeson , M. W. , and A. E. Strong . 1995 . Applying MCSST to coral reef bleaching . Advances in Space Research 16 ( 10 ): 151 – 154 .
- Gomez-Perez , A. , O. Corcho , and M. Fernandez-Lopez . 2004 . Ontological Engineering with Examples from the Areas of Knowledge Management, e-Commerce and the Semantic Web. First Edition (Advanced Information and Knowledge Processing). London : Springer .
- Guarino , N. 1997 . Understanding, building and using ontologies . International Journal of Human-Computer Studies 46 ( 2–3 ): 293 – 310 .
- Hey , T. , and A. E. Trefethen . 2003 . The data deluge: An e-Science perspective . In: Grid Computing – Making the Global Infrastructure a Reality , eds. F. Berman , G. C. Fox , and A. J. G. Hey . West Sussex , UK : Wiley and Sons .
- Horrocks , I. , P. F. Patel-Schneider , and F. van Harmelen . 2003 . From SHIQ and RDF to OWL: The making of a web ontology language . Journal of Web Semantics 1 ( 1 ): 7 – 26 .
- Hughes , T. P. , A. H. Baird , D. R. Bellwood , M. Card , S. R. Connolly , C. Folke , R. Grosberg , O. Hoegh-Guldberg , J. B. C. Jackson , J. Kleypas , J. M. Lough , P. Marshall , M. Nystrom , S. R. Palumbi , J. M. Pandolfi , B. Rosen , and J. Roughgarden . 2003 . Climate change, human impacts, and the resilience of coral reefs . Science 301 ( 5635 ): 929 – 933 .
- Integrated Marine Observing System (IMOS) . 2008 . http://imos.org.au/. Last accessed June 2008 .
- Intergovernmental Panel on Climate Change (IPCC) . 2007 . Climate change 2007: The physical basis . In: Contribution of Working Group I to the Fourth Assessment Report of the Intergovernmental Panel on Climate Change. Cambridge , UK : Cambridge University Press .
- Jarrar , M. , and R. Meersman . 2008 . Ontology engineering – The DOGMA approach . In: Advances in Web Semantic. Berlin/Heidelberg : Springer .
- Kininmonth , S. , S. Bainbridge , I. Atkinson , E. Gilla , L. Barrald , and R. Vidaude . 2004 . Sensor networking the Great Barrier Reef . Spatial Sciences Qld 1 : 34 – 38 .
- Marshall , P. , and H. Schuttenberg . 2006 . A Reef Manager'S Guide to Coral Bleaching . G.B.R.M.P. Authority (eds.), Great Barrier Reef Marine Park Authority, Townsville, Australia .
- Maynard , J. , K. Anthony , P. Marshall , and I. Masiri . 2008 . Major bleaching events can lead to increased thermal tolerance in corals . Marine Biology 155 ( 2 ): 173 – 182 .
- McGuinness , D. L. , and F. van Harmelen . 2004 . OWL Web Ontology Language Overview [W3C Recommendation]. http://www.w3.org/TR/owl-features/. Last accessed February 2009.
- Motik , B. , U. Sattler , and R. Studer . 2005 . Query answering for OWL-DL with rules . Web Semantics: Science, Services and Agents on the World Wide Web 3 ( 1 ): 41 – 60 .
- Myers , T. S. , I. M. Atkinson , and W. J. Lavery . 2007 . The Semantic Reef: Managing complex knowledge to predict coral bleaching on the Great Barrier Reef . In: Proceedings of the Fifth Australasian Symposium on ACSW Frontiers . Ballarat , Australia .
- Myers , T. S. , I. M. Atkinson , and J. Maynard . 2007 . The Semantic Reef: An eco-informatics approach for modelling coral bleaching within the Great Barrier Reef . In: ERE Environmental Research Event. Cairns , Queensland .
- NASA . 2009 . Semantic Web for Earth and Environmental Terminology (SWEET). Jet Propulsion Laboratory, California Institute of Technology. http://sweet.jpl.nasa.gov/ontology/. Last accessed July 2009 .
- National Oceanic and Atmospheric Administration (NOAA) . 2009 . Coral Reef Watch – Methodology and description. http://coralreefwatch.noaa.gov/ satellite/methodology/methodology.html. Last accessed May 2009 .
- Noy , N. F. , and D. L. McGuinness . 2001 . Ontology development 101: A guide to creating your first ontology . Knowledge Systems Laboratory 1 – 5 .
- O'Connor , M. , R. Shankar , and A. Das . 2006 . An ontology-driven mediator for querying time-oriented biomedical data . In: Proceedings from the 19th IEEE International Symposium on Computer-Based Medical Systems . (CMBS 2006), 22–23 June , Salt Lake City , UT .
- O'Connor , M. J. , H. Knublauch , S. W. Tu , B. Grossof , M. Dean , W. E. Grosso , and M. A. Musen . 2005 . Supporting rule system interoperability on the Semantic Web with SWRL . In: Fourth International Semantic Web Conference (ISWC-2005) , Galway , Ireland .
- O'Connor , M. J. , S. W. Tu , C. I. Nyulas , A. K. Das , and M. A. Musen . 2007 . Querying the Semantic Web with SWRL . In: The International RuleML Symposium on Rule Interchange and Applications (RuleML2007) , Orlando , FL .
- Page , R. D. M. 2006 . Taxonomic names, metadata, and the semantic web . Biodiversity Informatics 3 : 1 – 15 .
- Page , R. D. M. 2008 . Biodiversity informatics: The challenge of linking data and the role of shared identifiers . Briefings in Bioinformatics 9 ( 5 ): 345 – 354 .
- Protégé . 2009 . The ontology editor and knowledge acquisition system. Stanford University. http://protege.stanford.edu/. Last accessed April 2009.
- Rector , A. , N. Drummond , M. Horridge , J. Rogers , H. Knublauch , R. Stevens , H. Wang , and C. Wroe . 2004 . OWL Pizzas: Practical experience of teaching OWL-DL: Common errors and common patterns . In: Proceedings of the European Conference on Knowledge Acquistion (EKAW 2004) , Northampton , UK .
- Science Environment for Ecological Knowledge (SEEK) . 2009 . http://seek.ecoinformatics.org/. Last accessed March 2009 .
- Stocks , K. I. , C. Neiswender , A. W. Isenor , J. Graybeal , N. Galbraith , E. T. Montgomery , P. Alexander , S. Watson , L. Bermudez , A. Gale , and K. Hogrefe . 2009 . The MMI Guides: Navigating the world of marine metadata. http://marinemetadata.org/guides. Last accessed April 2009.
- Uschold , M. , and M. Gruninger . 1996 . Ontologies: Principles, methods and applications . Knowledge Engineering Review 11 : 93 – 136 .
- Uschold , M. , and M. King . 1995 . Towards a methodology for building ontologies . In: International Joint Conference on Artificial Intelligence (IJCAI-95) , December 1996 , Montreal , Canada .