ABSTRACT
The current research describes the use of e-mail reply suggestions from a socio-cultural perspective of some language-related variables. It also correlates how users perceive the use of e-mail reply suggestions to some variables: job, specialization, age, and gender. In this descriptive-inferential survey design, a self-developed questionnaire was distributed online to collect the data from a convenient sample of e-mail users. The analysis shows the study sample perceived medially the use of e-mail reply suggestions. There are statistically significant differences in the respondents’ means about using e-mail reply suggestions according to the variable of job for education, specialization for humanities, and gender for females. Also, ages of less than 30 and above 50 years are of significance in using e-mail reply suggestions. The study reveals a weak inverse correlation, however, statistical between using e-mail reply suggestions and the language of those suggestions. In addition, a statistically significant positive direct correlation is found between usability and culture and society of e-mail reply suggestions. In light of the findings, the study recommends the better implementation of artificial intelligence-mediated communication in e-mail reply suggestions in association with some sociolinguistic factors. Also, future related studies are suggested.
Introduction
Artificial intelligence (AI) is progressing fast and can enhance communications and interactions among human beings. Such communications and interactions can be boosted using AI in computer-mediated communication (CMC) (Hancock, Naaman, and Levy Citation2020). People have started trusting artificial intelligence-mediated communication (AI-MC) as it allegedly and arguably provides fast and useful information amid this accelerating world in which time is highly precious. One AI-MC application is e-mail reply suggestions (ERS), which provide users with automated responses in order to save their effort and time. The effectiveness of these systems is usually measured quantitatively using the users’ choices of these suggestions from a technical perspective (Hancock, Naaman, and Levy Citation2020; Henderson et al. Citation2017; Hohenstein and Jung Citation2018, Citation2020; Kannan et al. Citation2016; Moosa Citation2016). Yet, there is still much to know about how ERS service is perceived by users from social, cultural, and language perspectives (Hancock, Naaman, and Levy Citation2020; Henderson et al. Citation2017; Hymes Citation1972; Mieczkowski et al. Citation2021; Robertson et al. Citation2021). ERS poses some challenges concerning context, interpersonal relationships, agency, positivity, gender, language use, and religion. The novelty of the current study lies in describing the nature of how Gmail users perceive ERS and revealing the beneath factors that make them hesitant to use ERS. Therefore, the study aims to unveil users’ perceptions of ERS from a socio-cultural perspective of the English language. Also, it sheds lights on the relationship between the users’ perceptions of ERS and the variables of job, specialization, age, and gender. The study contributes to the existing knowledge as follows:
It describes and assesses the nature of how Gmail users perceive ERS.
It reveals the hidden factors making them hesitant to use ERS.
3. It presents guidelines to help those designing suggestions for automated e-mail replies.
Problem Description
E-mail has been an effective mean of communication. It is used in all sectors of life, including business, family, education, healthcare, etc. Also, it is used to create usernames for many applications and platforms. Google keeps updating its G-mail services using the most cutting edge technologies. Recently, it has added the service of reply suggestions to ease users’ work by saving time and effort. The ERS service analyzes automatically the received e-mail and accordingly suggests three short reply options. However, there has been a debate on using those suggestions, and the idea was not welcomed a lot among users to some extent due to various reasons concerning social, cultural, and language perspectives. Yet, some more factors are to be investigated such as the context, interpersonal relationships, agency, positivity, gender, language use, and religion. The current study assesses the nature of how Gmail users perceive ERS and explore the hidden factors making them hesitant to use ERS. Therefore, the problem of the study is reformulated in the following research questions:
1. To what extent do the users of Gmail perceive the usage of e-mail reply suggestions?
2. Is there any link between the respondents’ perceptions of e-mail reply suggestions and their job, specialization, age, and gender?
3. Is there a significant relationship (0.05) between the usability of e-mail reply suggestions (domain 1) and the language of suggestions (domain 2) and/or culture and society (domain 3)?
Background Work
Artificial Intelligence-Mediated Communication
A channel of CMC, AI-MC is defined as “mediated communication between people in which a computational agent operates on behalf of a communicator by modifying, augmenting, or generating messages to accomplish communication or interpersonal goals.” (Hancock, Naaman, and Levy Citation2020, 90). A few instances of AI-MC incorporate syntax adjustment, auto-completion, automated messages, auto-correct, prescient text, and brilliant answers (Hancock, Naaman, and Levy Citation2020). Clients commonly associate with these softwares through synthesis ideas, which are given as the client types or auto-replies, which give independent reactions. These ideas can assist clients with trying not to spell blunders, diminish keystrokes, and send brief answers with the snap of a button. (Arnold, Gajos, and Kalai Citation2016; Quinn and Zhai Citation2016). Users of Gmail, for instance, can use one of three reply suggestions that AI produces to respond to an e-mail. IA-MC includes five dimensions, as argued by Hancock, Naaman, and Levy (Citation2020): magnitude (changes made on messages by AI; media type (text, audio, and video); optimization goal (the goal for which AI optimizes message); autonomy (AI acts on the sender’s behalf); role orientation (AI enhances reply efficiency and assessment). IA-MC benefits that give ideas to Computer-Mediated Communication (CMC) including text, and e-mail – are presently implanted in items utilized by billions of individuals consistently (Chen et al. Citation2019; Hohenstein and Jung Citation2018; Kannan et al. Citation2016). Recent literature characterizes these suggestions as a type of CMC mediated by AI (Hancock, Naaman, and Levy Citation2020; Jakesch et al. Citation2019), consisting of “mediated communication between people in which a computational agent operates on behalf of a communicator by modifying, augmenting, or generating messages to accomplish communication or interpersonal goals” (Hancock, Naaman, and Levy Citation2020).
Sociolinguistics and Computer-Mediated Communication
Language and language use through the Internet has specific and distinct features that differ from those in real life. They are governed by the medium constraints (e.g., decreased conversational coherence, disrupted turn adjacency, and relevance norms) and affordance (e.g., easiness, speed, economy) (Herring, Stein, and Virtanen Citation2013). Also, the language of CMC is inadequately contrasted with language in spoken and written forms. It underscores the shortfall of a few sign sorts, for example, non-verbal, hearable, olfactory, gustatory, and material, and the utilization of compensational elements like emojis, graphical gadgets, redundancies, and omissions (Herring, Stein, and Virtanen Citation2013). Herring, Stein, and Virtanen (Citation2013) argued that sociolinguistics can add to CMC by showing the effect of language use and etymological changeability in the development of relational connections and social characters on the Internet. Also, people’s ways of writing and interpreting messages may be changed amid the emergence of AI-MC. AI-MC can shape human language and thought as argued by Hancock, Naaman, and Levy (Citation2020). Studying the interaction between language-related variables and computer-mediated communication is represented in linguistic peculiarities that newbies should check whether they need to join, how orderly these highlights are, and the number of accents that can be recognized (Androutsopoulos Citation2006).
Research on the relationship between sociolinguistics and computer-mediated communication started in the mid of 1990s (Herring, Stein, and Virtanen Citation2013). This relationship includes aspects of language choice and code-switching, usage variation concerning users’ status, gender, dialects, and mode of CMC. The research has resulted in three main phenomena: classical core pragmatic, CMC-specific (emoticons, nicknames, netspeak), and CMC types (chats, blogs, wikis) (Herring, Stein, and Virtanen Citation2013). Crystal (Citation2001) used the term Netspeak to refer to the use of language in computer-mediated communication. Netspeak is a type of language “displaying features that are unique to the Internet” (Crystal Citation2001: 22, 94). One instance is that e-mails are considered as a language variety or new linguistic medium that comprises “functionally distinct elements” that are “central for the identification of e-mail as a linguistic variety,” such as headers, signatures, greetings, and responsive quotations, as well as “more local points of stylistic significance,” for instance, spelling variation” (Crystal Citation2001, 122).
According to Androutsopoulos (Citation2006), sociolinguistics in CMC includes some issues related to language variation, social interaction, online ethnography, social identity and language, and multi-lingualisim. Language elements that have been evaluated and thought about across or inside web-based networks incorporate emojis, unusual spellings, communicated highlights of spoken language, territorial vernacular elements, code-exchanging, and vulgarity. These highlights were found to have a connection with age, gender, and the region as reviewed by Androutsopoulos (Citation2006).
Social interaction is also another important aspect of sociolinguistics in computer-mediated communication. This work proposes that in-between language and intercultural studies are expected to comprehend the exchange of transcultural and socially certain powers in the molding of social connection in CMC. The literature investigated individual or public collaboration through e-mail, newsgroups, or conversation channels about the foundation of interactional cognizance and support structure, pleasantness in conversations, language play, and execution, tongue stylization, style-moving, and code-exchanging (Androutsopoulos Citation2006). Online ethnography concerns the study of cultures online to examine the online communities’ formation and events that take place concerning offline communities. It incorporates the investigation of the connection between language, personality, and online social design among clients, offline and online communication (Androutsopoulos Citation2006). Social identity and language started by linking the use of language and gender in asynchronous and synchronous settings. Multilingualism on the Internet includes the strength of English as the most widely used language of transnational correspondence and the portrayal of language variety on the web. It is this strand of CMC research that makes unequivocal connections to the globalization hypothesis and analyzes the exchange of worldwide and nearby powers in the improvement of language variety online (Androutsopoulos Citation2006).
Literature Review
E-Mail Reply Suggestions Service
In 2017, Google created a smart reply for its e-mail use, Gmail, utilizing AI to check approaching e-mails and foresee potential reactions. Smart reply not just computerizes sending the e-mail reaction yet predicts potential reactions and suggests the client with three reply options. ERS suggests three short responses in English (Jehan Citation2021). Gmail clients can utilize automated answers to react to their messages much faster and with the assistance of straightforward expressions. Kannan et al. (Citation2016) argued that the responses should be customized to suit whatever the e-mail message is. The users choose to respond with a single-click smart reply. Marcelis and MacMillan (Citation2018) reported that promptly after Google carried out Smart Reply as a default setting for all of its 1.4 billion dynamic Gmail accounts, 10% of all Gmail reactions sent were produced by Smart Reply. ERS aims to simplify or accelerate correspondence among users (Robertson et al. Citation2021). It saves the client’s time in writing a reaction in situations where one of the three anticipated answers is adequate. Be that as it may, the client should, in any case, choose whether to send an anticipated reaction or to write one straightforwardly (Agrawal, Gans, and Goldfarb Citation2019). Hancock, Naaman, and Levy (Citation2020) and Hohenstein and Jung (Citation2018) argued that Gmail’s excessively positive language replies can move language standards and assumptions in any event, when interactors are not utilizing these services, and produce long haul language change after some time. Also, smart replies must be good “quality in style, tone, diction, and content” (Kannan et al. Citation2016).
Literature on e-mail smart replies has centered on the technical perspectives of their execution that represented punctuation, spelling, grammar, and mechanics of excessively natural composition, and casual composition. Likewise, technical methodologies, for example, frequently use measurements like transformation rates – the quantity of snaps an idea gets – as an intermediary for reply quality (Henderson et al. Citation2017). Albeit valuable, such measurements summarize how suggested replies are capable and uncover minimal with regards to how friendly settings and different elements may add to that insight. The further effect of AI-MC on language might be in the way that individuals adjust. In addition, Toma (Citation2014) debated that interpersonal variation, or altering one’s practices to conform to a correspondence accomplice, is basic to social collaboration. This interaction might be disturbed or heightened when AI-MC is incorporated. What will happen if AI-MC assumes a part in proposing or creating messages by a communicator? Research is expected to examine the effect of these auto smart replies and other AI-MC language patterns, from pragmatic-minded peculiarities (e.g., politeness) to enlist peculiarities (gender patterns, provincial accents, and bunch dialectical languages utilized in internet-based networks), which could all be affected by AI. The execution of AI-MC applications can additionally gather or discriminate language practices by permitting or covering individual or gathering-based varieties. Additionally, the content and style highlights of language are the guideline channels for interpersonal information in CMC. However, AI generating and modifying messages sent through technology may raise issues related to the loss of diagnosticity for interpersonal information (Hancock, Naaman, and Levy Citation2020). Self-representation, evaluation of others, and trustiness are other important issues that are to be impacted by AI.
Research on using automated replies has revealed various findings. Concerning the interpersonal dynamics, Hohenstein and Jung (Citation2020) examined how trust and agency were perceived by 113 US university users when AI-MC was applied. The researcher compared the degree of success between the use of traditional replies and smart replies. The use of AI-MC enhanced trust among communicators, however, some interactions were not successfully attributed to the aspect of AI-MC agency that caused some moral collapse. As for technicality, Moosa (Citation2016) sought to understand friend and classmate users’ perspectives toward suggesting smart instant messages suggested by Google Allo through a conversation and a survey in terms of knowledge and familiarity. While the findings showed a disagreement on the representation of Allo’s suggestions for the participants’ intention or their personality, the suggestions were found enjoyable, but unhelpful. The usefulness of Allo’s suggestions was rated moderate. Also, Quinn and Zhai (Citation2016) conducted an experiment using suggestions on mobile keyboards on 17 participants. Suggestions were either always present, never present, or gated by a probability threshold. The results showed that although the increase in suggestion confirmation reduced the number of keyboard actions for text entry and was personally preferred, no benefits were indicated by time due to the cost of interacting with the interface. In a similar context, Arnold, Chauncey, and Gajos (Citation2020) evaluated the impact of prescient text on composing content involving an inside-subject exploration in which 111 members from the US and Canada composed subtitles for pictures. It was shown that the inscriptions composed with suggestions were briefer and that they had limited words that the application did not anticipate. The suggested options likewise helped text passage speed with less advantage for quicker typists. Hohenstein and Jung (Citation2018) analyzed discussions between 36 college dyads utilizing AI-aided Google Allo and standard texting applications and evoked subjective input from the clients of the AI-aided Google Allo texting application through interviews in the United States. The findings showed the suggested responses were hardly ever used and were not especially connected to the task completion. The findings were attributed to the task nature accompanied by the positive slant of the recommended options playing a role in changing their direction of reply from negative to positive.
From a pragmatic perspective, Economidou-Kogetsidis (Citation2015) investigated the politeness level in six real messages (e-mails) composed by Greek-Cypriot college understudies in English to their English native lecturers. Politeness was perceived as significant in writing e-mails. Yet, it was recommended that English as a second language speakers’ awareness be raised. Similarly, Edstrom and Ewald (Citation2017) examined 60 US participants’ 81 English auto-reply messages in English from 60 participants in the United States of America. The results showed that greetings and closings were mostly missing from the messages, and this could impose issues related to politeness, intimacy, and personality among communicators. Robertson et al. (Citation2021) examined the impact of social context on e-mail reply suggestions using interviews and experiments. Gender-loaded replies, dissonant, Over-positive, contextual politeness, and cultural concerns were shown. Also, social ties and salutations impacted the participants’ perceptions of e-mail correspondence. Overall, Robertson et al. (Citation2021) study showed that the current text suggestion software for e-mail and other CMC innovations remains inadequately nuanced to mirror the accurate differences between genuine social connections and correspondence needs. Much more work on studying the link between the use of ERS service in association with the variables of job, specialization, age, language, and religion is highly needed to enrich the existing literature on the interaction between AI-MC and human beings.
To conclude, ERS is an important product of AI-MC having emerged recently. Its use of automated reply suggestions is governed by some factors concerning technical, social, cultural, and language issues. The conceptual frameworks of language centered on language competence and failed to capture communicative competence. Hymes (Citation1972) emphasized the role of language use and referred to it as appropriateness in communicative competence without which grammar rules are helpless. The review of the existing research highlights the technical effectiveness of ERS in terms of simplicity, easiness, time, speed, economy, relatedness to the content of the message, and language features. Yet, so that the ERS service is alleviated and highly appreciated by users, factors concerning the effect of users’ usability, cultural, social, and language perceptions on using ERS need to be investigated in the light of some variables. Therefore, the uniqueness of the study appears in examining the relationship between the users’ utilization of ERS in connection with the variables of job, specialization, age, and gender.
Methodology
The study applies the descriptive-inferential design to collect the required data from a convenient sample of e-mail users about their views on using e-mail automated reply suggestions in terms of usability, language, and culture and society. Also, the respondents’ perceptions are correlated in terms of job, specialization, gender, and age.
System Model Description
Inbox by Gmail’s Smart Reply service, which employs machine learning to recommend e-mail responses, was introduced by Google in 2017. Since its original release, Smart Reply has seen a sharp increase in usage, now accounting for around 12% of replies in Inbox on mobile. Designers have developed a new version of Smart Reply for Gmail based on the investigation into the use of Smart Reply in Inbox and our theories regarding how people learn and utilize language. This update improves algorithmic efficiency and the proportion of usable suggestions. Designers utilized hierarchies of modules of which humans can learn, retain, and recognize a sequential pattern, as inspiration for how people understand languages and concepts.
Language’s structure, which progresses from letters to words to phrases to sentences to paragraphs to sections to chapters to books to authors to libraries, etc., reflects the profound hierarchical nature of language’s content. Many of these minute discrepancies can be found using a machine learning approach if language examples are provided in sufficient numbers. Furthermore, the hierarchical structure of language lends itself nicely to a hierarchical approach to learning. This method, designers have discovered, is effective for recommending potential e-mail responses. Similar to how humans comprehend voice and language, designers employ a hierarchy of modules, each of which takes into account qualities that correspond to sequences at various temporal scales.
Figure 1. Process of Email Reply Suggestions (adopted from Strope and Kurzweil Citation2017).
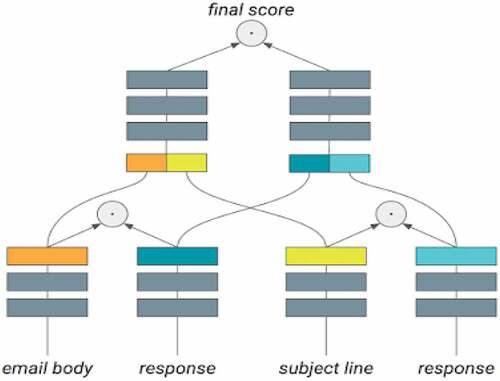
Each module performs input processing and outputs altered versions of the inputs (which are, in turn, available for the next level). The repeating structure in the Smart Reply system, and has two levels of hierarchy. Both the first and second combine these traits to make them effective as indicators of the outcome. The second, by definition, employs a more abstract representation and takes into account a longer timeframe.
In contrast, the first version of Smart Reply used a long-short-term memory (LSTM) recurrent neural network to encode incoming e-mails word-by-word, and another LSTM to decode suggested replies. Even with Google infrastructure, this kind of modeling is quite efficient in many situations, but it’s a method that uses a lot of computational power. By addressing the problem more thoroughly all at once and comparing a straightforward hierarchy of vector representations of various features corresponding to larger time spans, we discovered an effective and extremely fast method as opposed to working word-by-word. Designers have also thought about whether these vector representations’ mathematical spaces contain implicit semantics. In order to decide which inputs and answers belong together, do the hierarchical network representations show a rough “knowledge” of their true meaning, or do they show more dependable syntactical patterns? Designers discovered that the networks are surprisingly successful and efficient in generating representations that satisfy the training requirements given a large number of real examples of which couples go together and, perhaps more crucially, which do not.
Method
Population and Sample of the Study
The population of the study included users of Gmail programs that suggest automated replies. The sample of the study was conveniently chosen as the survey was created online and distributed to the users of Gmail through groups on social networks such as Facebook, WhatsApp, Gmail, and outlook. The link to the questionnaire was made available for respondents over two weeks. Wide coverage of users was attempted to reach out through the researchers’ friends, acquaintances, colleagues, students, and relatives. Based on the received responses, the details of the respondents (N = 1507) were categorized according to job, specialization, gender, and age as shown in .
Table 1. Distribution Frequencies of the Study Sample according to Variables.
Survey
The study utilized an initially self-developed questionnaire based on the review of the literature. The questionnaire was administered to gather data on the respondents’ perceptions of the use of e-mail reply suggestions in their correspondences. It also collected data about the respondents’ personal information for correlating the users’ perceptions to their background information. Therefore, it included two main sections: background information (job, specialization, age, and gender) and users’ perceptions of e-mail reply suggestions. The perceptions of users on using e-mail reply suggestions totaled 36 items and were divided into three domains: usability [5,6,7,8,9,10,11,12,13,14], language [15,16,17,18,19,20,21,22,23,24,25,26], and culture and society [27,28,29,30,31,32,33,34,35,36,37,38,39,40].
The questionnaire was administered to e-mail users online. It was created online using Microsoft Forms. Then, the link was shared with users through some channels such as Facebook, WhatsApp, Gmail, and Outlook. The questionnaire was open for two weeks to guarantee a good number of replies.
The issue of validity was maintained by getting the questionnaire reviewed by 10 experts in the field of artificial intelligence and sociolinguistics. They were asked to check whether the questionnaire can measure the e-mail users’ perceptions toward the use of reply suggestions service in light of social, cultural, and language variables. Also, they were asked to rate the questionnaire out of 100%. The rate means of the experts reached 91%. In addition, they suggested issues related to language editing, organization, changing items from questions to statements, and the use of a Five-point Likert scale (never, rarely, sometimes, often, & always). Also, the validity of the questionnaire was confirmed consistently by administering it to a sample of 25 e-mail users other than those who participated in the main study. The internal consistency of the study in which the relationship between items and domains as well as domains and the whole scale was calculated by Pearson Correlation.
The means value of the study sample’s responses was calculated according to the number of rating options in the questionnaire. The range of means was calculated using the following equation: 4÷5 = 0.80. The standards of extracting data and interpreting means were the following: 1–1.80= very low level, <1.80–2.60= low level, <2.60–3.40= medium level, <3.40–4.20= high level, <4.20–5= very high level.
The results of Pearson correlation between the items and domains of the scale were significant at (0.01). The values of Pearson correlation ranged between (0.548–0.922). Also, the results revealed that the values of Pearson correlation between the domains and the scale as a whole were significant at (0.01). The values of the first (Usability), second (Language), and third (Culture & society) domains were (0.986, 0.978, 0.952) respectively. These results indicate the validity of the scale to measure what it was prepared for.
The reliability was measured using Cronbach’s Alpha equation. Also, the reliability was computed using the test-retest method of the questionnaire that was applied twice in two weeks. shows the results of the analysis.
Table 2. Coefficients of Cronbach’s Alpha and Test-retest (Pearson).
shows that the coefficient of Cronbach’s Alpha scored high (0.96). Also, the coefficient of Pearson came high (0.95). These results indicate that the questionnaire enjoys a high validity and reliability.
The collected data were analyzed using SPSS, v23. Two analyses were computed: descriptive and inferential. While the descriptive analysis extracted means, standard deviation, frequencies, and percentages, the inferential analysis ran t-tests to infer any statistically significant differences between the users’ background information and their perceptions of using e-mail reply suggestions.
Results
G-Mail users’ Perceptions of ERS
The means and standard deviations of the study sample’s responses were calculated for the items, the domains, and the whole scale. and depict the results.
Table 3. Descriptive Statistics.
shows that the users perceived the use of e-mail reply suggestions medially (M = 3.25, SD = 0.329). To illustrate, the domain of usability of e-mail reply suggestions was highly perceived (M = 3.54, SD=.837). The second and third domains (language, culture & society) scored medium (M = 3.24, 3.09, SD=.417, 335) in order.
Job
The study sample’s responses were analyzed using the one-way analysis of variance (ANOVA) to extract any significant differences in using e-mail reply suggestions according to the variable of job. displays the results.
Table 4. Variance Analysis of Responses according to Job.
shows statistically significant differences in the means of the respondents about using e-mail reply suggestions according to the variable of job on the overall scale and the domains of language and culture and society except for the first domain (usability) (0.041). The dimensional comparisons using Scheffe’s method were used to show whether the differences are significant for usability.
shows a statistically significant difference at (0.05) between the jobs of education and industry in favor of education (0.029). This result means that those who study or work in educational fields had more significant and higher perceptions toward using e-mail reply suggestions than those who work in other fields, namely, trade and health.
Table 5. Multiple Comparisons - Scheffe.
Specialization
T-test analysis was used to extract any significant differences between the participants’ responses about using e-mail reply suggestions attributed to the variable of specialization. shows the results.
Table 6. T-Test Analysis of Responses according to Specialization.
As shown in , significant differences appeared in the overall domains of usability and culture and society at (0.000). However, the language domain had no statistical difference. The statistical differences came in favor of those participants who specialize in humanities.
Age
The study sample’s responses were analyzed using the one-way analysis of variance (ANOVA) to extract any significant differences in the use of e-mail reply suggestions according to the variable of age. depicts the results.
Table 7. Variance Analysis of Responses according to Age.
shows statistically significant differences at (0.05) in the means of the respondents about using e-mail reply suggestions on all the domains of the scale (usability, language, culture & society) and the scale as a whole. The dimensional comparisons using Scheffe’s method were used to show whether the differences are significant for usability.
shows statistically significant differences in the first domain (usability) at (0.05) between the participants who are less than 30 years old and those who are above 50 years old in favor of less than 30 years. This means that those whose age is less than 30 years had perceived higher perceptions toward using e-mail reply suggestions than those whose age is above 50 years. Also, there were significant differences between those whose ages ranged between 30–50 and above 50 for the group of 30–50 years old. As for the second domain (language), significant differences were shown between those less than 30 years old and above 50 in favor of those less than 30. In addition, differences were scored between those who ranged between 30–50 and above 50 for the benefit of the group from 30–50 years old. The third domain (culture & society) had significant differences between those whose age is less than 30 years compared to 31–50 in favor of 31–51 years old. In total, the respondents had significant differences between less than 30 and 31–50 years old in favor of the latter. Also, significant differences came for the group with ages ranging from 30–50 compared to those above 50 years old.
Table 8. Multiple Comparisons - Scheffe.
Gender
T-test was used to show the significance of means differences in the study sample’s responses according to the variable of gender as displayed in .
Table 9. T-test Analysis of Responses according to Gender.
According to , significant differences at (0.05) are shown in the overall domains of usability and culture and society at (.000) except for the second domain (language). The statistical differences came in favor of the female participants. This result indicates that female users of Gmail do consider the issues of culture and society when using e-mail reply suggestions. Unlikely, male users make no line between the use of e-mail reply suggestions and the cultural and social aspects of those suggestions.
Usability of E-Mail Reply Suggestions and Language of Suggestions
Pearson correlation coefficient between the use of e-mail reply suggestions (domain 1) and the language of the suggestions (domain 2) was extracted as presented in .
Table 10. Pearson Correlation between Usability and Language.
shows a weak inverse correlation, however, statistical between using e-mail reply suggestions and the language of those suggestions. That is to say, the increase in the use of e-mail reply suggestions means a decrease in the users’ consideration of language issues such as cohesion, coherence, writing styles, politeness, tone, etc. This hints at the increasing trust that results from Gmail users in using reply suggestions. Language issues are marginalized when correlated with increasing the degree of using e-mail-reply suggestions.
Usability of E-Mail Reply Suggestions and Culture and Society
Also, the Pearson correlation coefficient between the use of e-mail reply suggestions (domain 1) and culture and society (domain 3) was extracted as presented in .
Table 11. Pearson Correlation between Usability, and Culture and Society.
shows a statistically significant positive direct correlation between the first domain (usability) and the third domain (culture & society). This means that the more the respondents use e-mail reply suggestions, the more cultural and social considerations about using these suggestions there are. If any indication, the users of e-mail reply suggestions do consider the cultural and social issues when using those suggestions.
Also, the respondents were asked to indicate the categories where they used e-mail reply suggestions. shows the results.
shows that the respondents use e-mail reply suggestions mostly for work (No = 1232, 48%) followed by study (No = 781, 30%). This indicates that about 78% of using e-mail reply suggestions is used in formal settings such as work and study. Also, the respondents use e-mail reply suggestions in their contact of friends (No = 352, 14%) and family members (No = 220, 8%). That is to say, around 22% respondents use e-mail reply suggestions in informal settings with friends and family members. This result may be justified with the less efficiency of using e-mail in general and reply suggestions in specific by users at the personal level in their contact of friends and family members amid the spread of social networking applications such as WhatsApp, Facebook, Twitter, etc. In addition, the respondents indicated the useful perspectives of e-mail reply suggestions as shown in the following figure.
shows that the respondents’ use of e-mail reply suggestions would help them save time (No = 759, 28%), effort (No = 616, 22%), get new ideas (No = 627, 27%), and improve their language (No = 726, 27%).
Remarks
An open-ended question on the respondents’ remarks about e-mail reply suggestions. The relevant answers were analyzed and categorized under main themes and positive and negative aspects. Some respondents see that e-mail reply suggestions have more positive sides in terms of saving time and effort. Some other think that they are very helpful, useful and necessary when users on the go. However, some other respondents indicated that e-mail reply suggestions sometimes lead the receiver to misunderstand the sender’ intention. They might cause confusion because they are emotionless. Also, they are still inaccurate and need editing.
Discussion
The current study explored the Gmail users’ perceptions of e-mail reply suggestions in terms of usability, language, and culture and society. Also, the users’ perceptions of using e-mail reply suggestions were correlated with some variables: job, gender, specialization, and age. The analysis revealed that the respondents perceive medially the use of e-mail reply suggestions. While the domain of usability is highly perceived, language and culture and society has medium scores. Also, there are statistically significant differences in the means of the respondents about using e-mail reply suggestions according to the variable of job for education, specialization for humanities, and gender for females. In addition, the variable of age has significant differences in favor of less than 30 and above 50 years old. These differences are interpreted in that users of Gmail are not all at the same degree of using reply suggestions. Their jobs, specialization, gender, and age control their degree of using Gmail reply suggestions. To detail, those who work in the education sector have higher perceptions toward using Gmail reply suggestions than those who work in other sectors such as trade, industry, and health. Also, users who specialize in humanities more highly perceive the use of Gmail reply suggestions than those who specialize in sciences. In addition, gender is a key variable in determining the users’ degree of using Gmail reply suggestions. Female users have higher perceptions than male users. Further, age is significant in using Gmail reply suggestions. Users with ages less than 30 or above 50 years old perceive the use of Gmail reply suggestions more highly than those ages ranging between 30–50. Practically, these results indicate a need that the programmers who apply artificial intelligence in producing reply suggestions should consider the variations of the users’ jobs, specialization, gender, and age to ensure high efficiency and usability among different types of users.
The results showed a weak inverse correlation, however, statistical between using e-mail reply suggestions and the language of those suggestions. This means that the degree of using Gmail reply suggestions is linked weakly to the language of those suggestions. To illustrate, users do not consider language when using Gmail reply suggestions. Finally, a statistically significant positive direct correlation was scored between the first domain (usability) and the third domain (culture & society). That is to say, the users’ degree of using Gmail reply suggestions is linked to the cultural and social aspects that those suggestions include. In order words, the higher the degree of using Gmail reply suggestions is, the higher the considerations of cultural and social aspects are. These results emphasize the necessity to highly take into account the aspects of language such as clarity, logic, relevance, accuracy, writing styles, discourse, creativity, and politeness and culture and society such as interpersonal relationships, agency, communication, gender, religion, and dominance when programming Gmail reply suggestions.
Also, the results of open questions indicated that the respondents use e-mail reply suggestions mostly in formal settings such as work and study. Around 22% respondents use e-mail reply suggestions in informal settings with friends and family members. This result may be justified with the less efficiency of using e-mail in general and reply suggestions in specific by users at the personal level in their contact of friends and family members amid the spread of social networking applications such as WhatsApp, Facebook, Twitter, etc. In addition, it was shown that the use of e-mail reply suggestions would help users save time and effort, learn new ideas, and improve their language. Further, the remark section revealed the positive effectiveness of e-mail reply suggestions in saving time and effort. They are very helpful, useful, and necessary when users on the go. However, negative remarks on miscommunication and misunderstanding of intentions were unveiled some other respondents indicated that e-mail reply suggestions sometimes lead the receiver to misunderstand the sender’ intention. They might cause confusion because they are emotionless. Also, they are still inaccurate and need editing.
Conclusion
The current study sheds lights on artificial intelligence-mediated communication (AIMC). It explored Gmail users’ use of e-mail reply suggestions in terms of usability, language, culture, and society. Also, the users’ perceptions of using e-mail reply suggestions were examined concerning some variables: job, gender, specialization, and age. Given the current results, the study implicates the importance of considering the issues of language and culture and society when producing e-mail reply suggestions by artificial intelligence for better effectiveness of use. Future studies that make use of mixed designs on considerations when using e-mail reply suggestions are recommended. Applicable research that addresses issues such as usability, language, culture and society in e-mail reply suggestions in light of factors such as job, specialization, gender, and age is also suggested.
Improving the Efficiency of E-Mail Reply Service
The provider of e-mail reply suggestions may work on neutralizing the English language in a sense that keeps it away from cultural and social inferences which may affect the use of e-mail reply suggestions. Also, Google can work on providing the service of e-mail reply suggestions in other languages such as Spanish, French, Arabic, and Chinese over stages. This may improve the service and make users more satisfied. In addition, the users’ job, gender, specialization, and age should be well-analyzed while producing such suggestions due to the impact of using e-mail reply suggestions. The idea that one thing may fit all people no longer exists. More in-depth analysis and further considerations about the above issues individually should be of priority to ensure guaranteed continuity and trust among users in the developers’ persuasion to make to improve the service of reply suggestions. Google can ask on signing in or up for more information about the users’ job, gender, specialization, and age to improve the reply suggestions service. Doing so will help artificial intelligence to better analyze e-mails in light of users’ background information and suggest smarter replies. These recommendations may increase the percentage of generated e-mail reply suggestion services on mobiles from 12% to a higher rate to save effort and time and guarantee a quick response to e-mails.
Disclosure statement
No potential conflict of interest was reported by the authors.
Additional information
Funding
References
- Agrawal, A., J. S. Gans, and A. Goldfarb. 2019. Artificial intelligence: The ambiguous labor market impact of automating prediction. Journal of Economic Perspectives 33 (2):31–50.. doi:10.1257/jep.33.2.31.
- Androutsopoulos, J. 2006. Introduction: Sociolinguistics and computer‐mediated communication. Journal of Sociolinguistics 10 (4):419–38.. doi:10.1111/j.1467-9841.2006.00286.x.
- Arnold, K. C., K. Chauncey, and K. Z. Gajos. 2020. Predictive text encourages predictable writing. In Proceedings of the 25th International Conference on Intelligent User Interfaces, (128–470). doi: 10.1145/3377325.3377523.
- Arnold, K. C., K. Z. Gajos, and A. T. Kalai. 2016. On suggesting phrases vs. predicting words for mobile text composition. In Proceedings of the 29th Annual Symposium on User Interface Software and Technology, (603–08). doi: 10.1145/2984511.2984584
- Chen, M. X., B. N. Lee, G. Bansal, Y. Cao, Y. Zhang, J. Lu, J. Tsay, Y. Wang, A. M. Dai, Z. Chen, et al. 2019. Gmail smart compose: Real-time assisted writing. In Proceedings of the 25th ACM SIGKDD International Conference on Knowledge Discovery & Data Mining (2287–95). doi: 10.1145/3292500.3330723
- Crystal, D. 2001. Language and the Internet. Cambridge: Cambridge University Press.
- Economidou-Kogetsidis, M. 2015. Teaching e-mail politeness in the EFL/ESL classroom. ELT Journal 69 (4):415–24.. doi:10.1093/elt/ccv031.
- Edstrom, A., and J. D. Ewald. 2017. “Out of the office”: Conveying Politeness through Auto-Reply E-mail Messages. Language@ Internet 14 (4). doi: 10.1016/j.techsoc.2019.01.005.
- Hancock, J. T., M. Naaman, and K. Levy. 2020. AI-mediated communication: Definition, research agenda, and ethical considerations. Journal of Computer-Mediated Communication 25 (1):89–100.. doi:10.1093/jcmc/zmz022.
- Henderson, M., R. Al-Rfou, B. Strope, Y. Sung, L. Lukacs, R. Guo, S. Kumar, B. Miklos, and R. Kurzweil. 2017. Efficient Natural Language Response Suggestion for Smart Reply. arXiv preprint arXiv:170500652. doi: 10.18653/v1/d17-1235.
- Herring, S. C., D. Stein, and T. Virtanen. 2013. Introduction to the pragmatics of computermediated communication. In Pragmatics of computer-mediated communication, De Gruyter Mouton, 3–32. doi: 10.1515/9783110214468.3.
- Hohenstein, J., and M. Jung. 2018. AI-supported messaging: An investigation of human-human text conversation with AI support. In Extended Abstracts of the 2018 CHI Conference on Human Factors in Computing Systems, (1–6). doi: 10.1145/3170427.3188487
- Hohenstein, J., and M. Jung. 2020. AI as a moral crumple zone: The effects of AI-mediated communication on attribution and trust. Computers in Human Behavior 106:106190.. doi:10.1016/j.chb.2019.106190.
- Hymes, D. 1972. On communicative competence. In Sociolinguistics: Selected readings, ed. J. B. Pride and J. Holmes, 269–93. England: Harmondsworth.
- Jakesch, M., M. French, X. Ma, J. T. Hancock, and M. Naaman. 2019. AI-mediated communication: How the perception that profile text was written by AI affects trustworthiness. In Proceedings of the 2019 CHI Conference on Human Factors in Computing Systems, (1–13). doi: 10.1145/3290605.3300469
- Jehan, N. 2021. Smart Reply Suggestion for an E-mail in Multiple Languages Data and Method [ Doctoral dissertation]. COMSATS University Islamabad.
- Kannan, A., K. Kurach, S. Ravi, T. Kaufmann, A. Tomkins, B. Miklos, G. Corrado, L. Lukács, M. Ganea, P. Young, et al. 2016. Smart reply: Automated response suggestion for e-mail. In Proceedings of the 22nd ACM SIGKDD International Conference on Knowledge Discovery and Data Mining (955–64). doi: 10.1145/2939672.2939801
- Marcelis, D., and D. MacMillan. 2018. “Is this article worth reading? Gmail’s Suggested reply: ‘Haha, Thanks!’” Wall Street Journal. Retrieved from https://www.wsj.com/articles/very-interesting-awesome-love-it-gmailusers-confront-chipper-smart-reply-1537282569.
- Mieczkowski, H., J. T. Hancock, M. Naaman, M. Jung, and J. Hohenstein. 2021. AI-mediated communication: Language use and interpersonal effects in a referential communication task. Proceedings of the ACM on Human-Computer Interaction 5 (CSCW1):1–14.. doi:10.1145/3449091.
- Moosa, J. M. 2016. How smart is the “smart reply” feature of Google Allo: From users’ viewpoint. Retrieved from www.academia.com.
- Quinn, P., and S. Zhai. 2016. A cost-benefit study of text entry suggestion interaction. In Proceedings of the 2016 CHI conference on human factors in computing systems, (83–88). doi: 10.1145/2858036.2858305
- Robertson, R. E., A. Olteanu, F. Diaz, M. Shokouhi, and P. Bailey. 2021. “I Can’t Reply with That”: Characterizing Problematic E-mail Reply Suggestions. In Proceedings of the 2021 CHI Conference on Human Factors in Computing Systems, (1–18). doi: 10.1145/3411764.3445557
- Strope, B., and R. Kurzweil. 2017. Efficient Smart Reply, now for Gmail. Retrieved from https://ai.googleblog.com/2017/05/efficient-smart-reply-now-for-gmail.html
- Toma, C. L. 2014. Towards conceptual convergence: An examination of interpersonal adaptation. Communication quarterly 62 (2):155–78.. doi:10.1080/01463373.2014.890116.