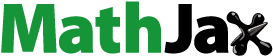
ABSTRACT
The phenomenon of economic globalization has led to a greater intermingling of economy and culture, which has brought about new demands for innovative talent cultivation in universities. To meet these demands, universities need to cultivate more students with high language ability who are capable of communicating and exchanging ideas between different cultures. This requires universities to innovate their language cultivation programs and improve students’ language ability and cultural literacy. The cultivation of international language ability, particularly in English language and literature, plays a vital role in enhancing students’ comprehensive language literacy. However, traditional teaching methods have been found to be less effective in achieving desired learning outcomes. As such, there is a need to adopt more innovative teaching methods that stimulate students’ interest in learning and facilitate their experience of English language and literature in authentic cultural contexts. One promising approach to achieve these objectives is through the integration of smart classroom teaching and artificial intelligence (AI) technology. This approach offers a range of benefits, including personalized learning experiences, real-time feedback, and interactive engagement. Furthermore, the application of AI technology can assist teachers in guiding students to experience the cultural background of English language and literature in English situations, helping students to exercise their English language application skills. This paper proposes a method and application assessment for designing a smart classroom for English language and literature based on AI technology. The primary aim of this approach is to help students become familiar with the English context and cultural background, thereby improving their overall language proficiency. The proposed approach seeks to facilitate a more engaging and effective learning experience for students, allowing them to develop their language skills and cultural literacy in a meaningful and impactful way.
Introduction
The teaching of English language and literature is a crucial aspect of education that aims to develop students’ high-quality and practical English language skills. By teaching the connotation and background of English literature, students can enhance their cognitive level of English language and culture, gain a deep understanding of social customs and practices of English-speaking countries, and become well-rounded individuals (Chen et al. Citation2022, Citation2022; Zhao et al. Citation2022). However, traditional English language and literature teaching modes often fail to engage students and hinder their active participation in learning. Furthermore, certain indoctrination teaching methods discourage students’ enthusiasm for learning. Therefore, the effectiveness of teaching mainly relies on the teacher’s professionalism and English language and literature teaching level (Hasan and Hasan Citation2019).
In recent years, the widespread application of artificial intelligence and smart classroom technology has provided a broader space for the modernization of education. These technologies contain rich resources and offer diverse and flexible forms that strongly promote the comprehensive renewal of teaching from concept to form. Thus, they create a theoretical and practical basis for promoting the development of English language and literature teaching (Chen et al. Citation2021, Citation2022; Cheng et al. Citation2011; Eser et al. Citation2014; Liang and Wang Citation2000). Under the macro background of informatization, English language and literature teachers should further strengthen the combination of artificial intelligence and smart classroom technology with English teaching. This focus would help to promote the scientific construction of teaching practice activities and the steady improvement of classroom teaching quality, which, in turn, would create a broader path for the exchange of Eastern and Western cultures. Ultimately, this would enable Chinese culture to be widely spread in the world.
The education department should actively introduce modern teaching concepts to provide English language and literature teachers with more room for development of their teaching activities. Artificial intelligence and smart classroom technologies promote a vibrant atmosphere for classroom teaching, enhance students’ interest in learning, and effectively promote innovation and progress in English language and literature teaching. illustrates a schematic diagram of pedagogical innovations in English language and literature, which can help teachers to adopt modern teaching concepts, implement them in the classroom, and enhance students’ learning outcomes.
The integration of artificial intelligence and smart classroom technology with English language and literature teaching is crucial for the modernization of education. English language and literature teachers should adopt modern teaching concepts, promote the scientific construction of teaching practice activities, and improve classroom teaching quality to create a broader path for the exchange of Eastern and Western cultures. Ultimately, this would enable Chinese culture to be widely spread in the world, and students to develop high-quality and practical English language skills.
Teachers play a critical role in promoting students’ interest in learning English language and literature. To achieve this goal, teachers should utilize a variety of artificial intelligence and smart classroom technologies to create a fun and engaging learning environment that fosters students’ subjective initiative and enthusiasm. Through the integration of AI and smart classroom technologies, teachers can provide students with novel and exciting learning experiences that promote their initial impression of English language learning and motivate them to explore the subject further (Kayad Citation2015).
The concept of a Smart Classroom refers to an educational environment that leverages technology to create a more interactive and personalized learning experience for students. There are several principles that underpin the Smart Classroom approach:
Interactive learning: The Smart Classroom encourages interactive and collaborative learning through the use of technology. This may include online discussion forums, virtual collaboration tools, and multimedia resources that allow students to engage with the course material in a more interactive and engaging way.
Personalized learning: The Smart Classroom approach recognizes that students have different learning needs and preferences. By leveraging technology such as AI-powered tools, educators can provide personalized feedback and support to students based on their individual needs and learning styles.
Flexibility: The Smart Classroom approach allows for flexibility in the delivery of course material and the scheduling of classes. This may include online classes or virtual office hours, which allow students to access course content and interact with their instructors at a time and place that is convenient for them.
Authentic learning experiences: The Smart Classroom approach emphasizes the importance of creating authentic learning experiences that expose students to real-world situations and contexts. This may include the use of virtual reality (VR) simulations, multimedia resources, and other tools that provide students with a more immersive and engaging learning experience.
Data-driven instruction: The Smart Classroom approach uses data to inform and improve instruction. This may include tracking student performance, identifying areas of weakness, and using data to adjust teaching strategies and course content to better meet the needs of students.
Teacher-led instruction: While the Smart Classroom approach leverages technology to create a more engaging and interactive learning experience, it is important to note that the role of the teacher remains crucial. Teachers are still responsible for guiding and supporting students, facilitating discussions, and providing feedback and guidance.
In conclusion, the Smart Classroom approach is based on principles that emphasize the importance of interactive and personalized learning, flexibility, authentic learning experiences, data-driven instruction, and teacher-led instruction. By leveraging technology in a thoughtful and intentional way, educators can create a more effective and engaging learning environment that better meets the needs of their students.
To ensure effective use of these technologies, teachers should reform their teaching philosophy and emphasize the role of students in the classroom. Effective communication with students is essential to understanding their personality and professional characteristics, and designing teaching methods that cater to their individual needs. Teachers should also eliminate students’ fear and resistance to learning English and use big data analysis methods to provide students with suitable English literature that further enhances their understanding of the subject (Liu and Wang Citation2008; Rodriguez, Wiles, and Elman Citation1999; Xia and Wang Citation2005; Xu, Ii, and Frank Citation2007).
Artificial intelligence and smart classroom technology can also be used to help students master the key and challenging aspects of English language and literature. Through the use of modern technologies, teachers can present complex cultural and historical knowledge in a vivid and engaging manner, using various multimedia forms such as video, audio, and images. By incorporating AI and smart classroom technologies into classroom teaching, teachers can provide students with an interactive and participatory learning environment that encourages exploration and builds a solid foundation of subject knowledge (Kim, Lewis, and Abdallah Citation1997; Xia and Wang Citation2005).
The use of artificial intelligence and smart classroom technology can significantly enhance English language and literature education (Du Citation2022). Through the integration of these technologies, teachers can create an engaging and stimulating learning environment that promotes students’ subjective initiative and enthusiasm, helps them master key and difficult contents, and builds a systematic and solid knowledge structure of the subject. Ultimately, this approach can lead to improved literacy and better academic outcomes for students.
In this paper, we adopt the SMART analysis framework to analyze the smart classroom teaching model of English language and literature. According to the SMART analysis framework, the smart teaching model can be summarized into five dimensions: content presentation, environment management, resource acquisition, timely interaction, and context perception, which are abbreviated as “S, M, A, R, T” conceptual model. According to the actual situation of the smart classroom teaching model, the five dimensions of the SMART conceptual model are standardized and improved, i.e., the college English smart classroom teaching model can be constructed from the five dimensions of content presentation, organization and management, resource acquisition, timely interaction and testing and evaluation, as shown in .
The main elements in are explained as follows.1) Content presentation (Showing): Content presentation requires the English smart classroom to be able to arrange the teaching content clearly and reasonably according to the students’ situation and teaching status. 2) Organizational management (Manageable): Organizational management mainly refers to the teacher’s wisdom management level of class activities, which can improve the management efficiency of the class. 3) Resource access (Accessible): Resources The classroom needs to be equipped with sufficient management tools and teaching resources, and students can freely access learning resources. 4) Real-time interactive: The classroom needs to be able to ensure that students can freely interact with teaching and learning, and the operation is convenient. 5) Testing: Testing Evaluation requires intelligent classroom support for teachers to provide timely feedback on classroom testing results, students can provide timely feedback on teaching suggestions, and support a diverse evaluation system (Barbounis et al. Citation2006; Lukosevicius and Jaeger Citation2009; Xiong and Jie Citation2005).
With the continuous research on artificial intelligence, several high-quality studies on generative automatic English language and literature teaching have started to appear in the field of English language and literature. After 2018, ELMo (Embeddings from Language Models), GPT (Generative Pretraining), and BERT (Bidirectional Encoder Representation from Transformers), emerged one after another, making the model of pre-trained language models + fine-tuning one of the practical application models in the field of natural language processing. The application of fully pre-trained language models to generative automatic English language and literature tasks requires only a small amount of model fine-tuning (Fine and Tune) to achieve performance comparable to that of the previous optimal model and has been used in GLUE (General Language Understanding Evaluation), SQuAD (Stanford Question Answering Dataset), RACE (Large Scale Reading Comprehension Dataset), and other downstream tasks in NLP (Takizawa et al. Citation2016).
The neural network model based on Transformer structure has also become one of the benchmark approaches in generative English language and literature generation models. Generative ELT techniques summarize the original document in two main ways: Sentence Compression, which removes words and phrases from sentences to reduce the length of individual utterances, and Sentence Fusion, which selects parts of several utterances separately and fuses them into one utterance. The difficulty of utterance compression is relatively small because the unimportant content of the utterance can be removed, and the grammatical semantics of the original sentence can still be maintained. In contrast, utterance fusion requires condensed generalization of several input utterances, which is more difficult and is the main performance bottleneck of the generative automatic textual English language and literature model.
The concept of Points of Correspondence (PoC) is used to study the phenomenon of utterance fusion in English language and literature texts. Quantitative analysis of the generated texts by methods such as Pointer Generator Networks and comparison with manually generated reference English language and literature reveals that although the texts generated by the previous methods can achieve good performance in ROUGE and other the percentage of the texts generated by the preexisting method is much lower than the human average, although they can achieve good results in ROUGE. To address this problem, two customized models based on the idea of pre-trained language models are designed to improve the number and quality of the generated texts using utterance fusion methods, and thus the quality of the generated English language and literature texts.
Related Work
English Language and Literature Smart Classroom
Teachers can use modern smart classroom technology to create authentic contexts for teaching activities and guide students to fully appreciate the cultural connotations of English language and literature in the process of language use and to build excellent intercultural communication skills. As an important goal of English language and literature teaching, the cultivation of high standards of students’ intercultural communication skills should occupy a large proportion of the overall teaching activities. Teachers need to attach great importance to the cultivation of students’ language comprehension ability, effectively change the situation of certain traditional English language and literature teaching that emphasizes expression but not comprehension, lead students to comprehensively and deeply comprehend the knowledge and cultural connotations in language, promote the construction of students’ language system on the basis of their language comprehension ability and social and cultural cognitive ability, and strengthen the all-roundedness of students’ multiple abilities in listening, speaking, reading, writing and translating English (I, I, and M (Keiko and Akiko Citation2016; Li, Qu, and Scherpereel Citation2016)). The purpose of the program is to promote the construction of students’ language systems and to strengthen students’ listening, speaking, reading, writing, and translating skills. In practice, teachers can use smart classroom technology to assist in the creation of simulations of communicative situations to help students engage their minds in the immersive experience of applying language and literature to understand each other’s expressions and to increase their own sensitivity to English perception by appreciating the emotions in literary works. Daily English communication activities are used to reduce students’ nervousness and stress in language use, develop their confidence, and lay a solid foundation for smooth and accurate cross-cultural communication.
Teachers should apply smart classroom technology in a targeted manner to provide students with personalized knowledge assessment and corrective guidance. The teaching innovation of English language and literature cannot be separated from the post-class check to consolidate the learning effectiveness, and high-quality review is a necessary means to improve the teaching results. Teachers should scientifically use smart classroom technology to assign students review tasks of corresponding quantity and difficulty, such as using software to create knowledge mind maps, write English sequels of character stories, introduce the life of the main character in a literary work, or watch film and television adaptations of literary works, etc., to help students improve the knowledge structure system of English literature and achieve a deeper understanding of the main idea and cultural meaning of the work. This helps students improve their knowledge structure of English literature, achieve a deeper understanding and mastery of the main idea and cultural meaning of the works, and enhance their professionalism and understanding of English language and literature (Scott and Gilmour Citation2022; Stone, Mcmillan, and Hazelton Citation2015). At the same time, teachers can also provide students with a space for cultural exchange in English through activities such as lectures, debates, sequels, and seminars on English literature to help students summarize their learning experiences and complement their strengths and weaknesses and promote the improvement of their overall English language comprehension and expression skills.
Teachers should rely on smart classroom technology and focus on improving their professionalism and their ability to apply smart classroom technology to provide students with higher quality English language and literature teaching. In the context of artificial intelligence and smart classroom technology, education authorities should systematically analyze the impact of smart classroom technology on English language and literature teaching, reconstruct English teaching models and teaching requirements, and promote the improvement of students’ English language and literature comprehension and application skills with more flexible and targeted teaching methods. At the same time, schools should make use of the convenience of smart classroom technology to enhance the ability of English teachers, strengthen teachers’ ability to apply smart classroom technology and integrate it with professional knowledge through thematic training, improve teachers’ innovative thinking and ability to sort out and integrate knowledge, and invite senior scholars and industry leaders to participate in subject teaching seminars to expand teachers’ professional vision. We also invite senior scholars and industry leaders to participate in subject teaching seminars to expand teachers’ professional horizons, update their professional cognition and enhance their practical abilities, so as to lay the foundation for the construction of English language and literature classrooms with artificial intelligence and smart classroom technology. In addition, inter-school collaboration should also serve as an important means to improve teachers’ professionalism. Schools in the region and prestigious schools across the country can establish an efficient collaborative platform through smart classroom technology to pool high-quality teachers, strengthen the development and utilization of educational resources, enhance teachers’ professional knowledge application capabilities, and optimize the results of English talent cultivation (Kafipour Citation2014).
At the technical level, the smart classroom approach utilizes the MapReduce framework, which is a programming model introduced by Google. MapReduce is designed to process and analyze large data sets on a distributed system of servers. The framework consists of two primary functions: Map and Reduce. The Map function processes the input data and generates a local intermediate result in the form of a Key/Value pair. The Reduce function then processes these intermediate results remotely, using the scheduling of the Master (host) to combine results with the same key to produce the final output.
Another popular open-source project, Hadoop, also implements the MapReduce mechanism along with the HDFS distributed file system, which has been widely adopted by many companies worldwide for large-scale distributed data processing. Similarly, Lucene, another open-source project developed by Doug Cutting, provides a comprehensive set of APIs for full-text search. By using Lucene, developers can create and optimize index files to facilitate efficient querying of large data sets.
However, the internal mechanisms of Lucene can lead to performance issues when dealing with large data sets. In particular, when the index file grows beyond a certain size, a large amount of result data is loaded into memory, which can cause frequent garbage collection in the JVM and ultimately lead to a serious bottleneck in system query performance.
Artificial Intelligence Technology
With the advent of artificial intelligence, access to information is no longer a difficult task. People are in a state of information explosion all the time, with news breaking, celebrity news, political turmoil, and economic changes flooding people. With all this information, it is an urgent problem to quickly distinguish what information is needed and what is not. English language and literature generation technology can be a good solution to this problem, as it can effectively bring together the important information in long texts to create summary texts for people to read, and thus better distinguish the merits of the information. In this paper, we improve the existing model pointer generation network model to obtain better word embedding representation on Chinese summary and have good improvement in the feature representation of text (A and A (Bland Citation2013)).
The concept of utterance fusion is widely recognized as a crucial element of textual English language and literature, and has been subject to much discussion and investigation within the academic community. However, current textual English language and literature datasets lack labeled information regarding utterance fusion within their training data. In response, drawing on previous research on information fusion in English grammar, we propose a new approach to address this limitation. Specifically, we suggest annotating the CNN dataset based on PoC (point of coherence) information, and subsequently outsourcing the annotation and disclosing the dataset to facilitate further research on utterance fusion.
To operationalize this approach, we classify the PoCs relevant for utterance fusion into five types, including pronoun referent, nominal referent, common noun referent, repetition, and event-driven. Each sample in the dataset comprises two source utterances and one summary utterance that contains PoCs, with each PoC type and its specific occurrences in both the source and summary utterances labeled.
By addressing the gap in labeled data on utterance fusion in existing textual English language and literature datasets, this approach has the potential to advance research in this area and improve our understanding of the role of utterance fusion in English language and literature.
Self-supervised training means that the model can learn directly from unlabeled data without labeling the data. As a type of self-supervised training, pre-trained English language and literature models refer to learning a uniform English language and literature representation on large-scale unlabeled text, facilitating the use of downstream NLP tasks, and avoiding the need to train new models for new tasks from scratch. The core of self-supervised training lies in how to automatically generate labels for the data (Kayad Citation2015). The training tasks of pre-trained English language and literature models are basically English language and literature model tasks or various variants, so the training data labeling methods of different PTMs are also specific to their own training tasks. In terms of training tasks, PTMs can be divided into causal English language and literature models and mask English language and literature models (CLMs are also called autoregressive models), and the representative models include GPT series models, CTRL, Transformer-XL, Reformer.
After the popularity of deep learning, the current mainstream solutions with better performance on textual English language and literature datasets are based on deep neural network models, and most of them use Seq2Seq structure as the basic model framework. Taking the release of BERT as the boundary, these approaches can be further divided into pre-pre-training era models and post-pre-training era models, i.e., various approaches based on pre-training English language and literature models for summary English language and literature wisdom classrooms (Jiang and Wang Citation1993). Most of the pre-pre-training era models are based on recurrent neural networks (RNNs), including long- and short-term memory networks, threshold recurrent networks, and other RNN variants (Noori and Mazdayasna Citation2014). Various models in the post-pre-training era are basically based on Transformer structures.
Methods
The research methodology for designing and assessing a smart classroom for English language and literature based on AI technology would involve several key steps.
First, a comprehensive literature review would be conducted to identify the current state of the art in AI-powered language education and the potential benefits and challenges of using this approach. This review would involve analyzing research studies, articles, and reports in relevant fields such as education, computer science, linguistics, and psychology.
Second, the research would involve the development of a conceptual framework that outlines the key elements of the smart classroom approach and the expected outcomes. This framework would include the selection of appropriate AI tools and technologies, the development of effective teaching strategies, and the creation of a supportive learning environment.
Third, a pilot study would be conducted to test the effectiveness of the smart classroom approach in improving students’ language proficiency and cultural literacy. This study would involve selecting a group of students and dividing them into a control group and an experimental group. The control group would receive traditional classroom instruction, while the experimental group would receive instruction in the smart classroom using AI-powered tools and technologies.
Fourth, a range of metrics would be used to assess the effectiveness of the smart classroom approach, including student performance on language proficiency tests, engagement levels in classroom activities, and feedback from students and teachers. These metrics would be analyzed using statistical methods to determine whether the smart classroom approach has a significant impact on student outcomes.
Finally, based on the findings of the pilot study, the smart classroom approach would be refined and scaled up for broader implementation. This would involve developing a comprehensive implementation plan that addresses key issues such as teacher training, infrastructure requirements, and sustainability.
Overall, the research methodology for designing and assessing a smart classroom for English language and literature based on AI technology would involve a multi-disciplinary approach that combines insights from education, computer science, and social sciences, and emphasizes a data-driven and iterative process of development and evaluation.
Model Structure
The English language and literature wisdom classroom evaluation method based on multi-granularity features consists of two main parts: the multi-granularity semantic representation part and the multi-granularity feature matching part, as shown in . The multi-granularity semantic representation part includes a sub-word semantic representation module, a syllable semantic representation module and a phrase semantic representation module. First, to obtain semantic feature representations of different granularities, MBERT is used to vectorize the sub-word sequences of the reference and generated sentences. Based on this, the syllable semantic representation vectors and the phrase semantic representation vectors are obtained by combining the sub-word combinations that constitute syllables and phrases. Next, the semantic feature vectors of the reference and generated sentences at different granularities are matched with maximum cosine similarity respectively, so that the model considers the semantic correlation between the two sentences at multiple granularities.
Encoder-Decoder Framework
Encoder-Decoder is a training framework, which is divided into encoder and decoder. The function of the encoder is to transform a realistic problem into a mathematical problem, such as characterizing an input text, picture, or audio as a vector. The function of the decoder is to solve the mathematical problem based on the result of the encoder and translate it into a real-world solution. The implementation of both encoder and decoder functions relies on deep learning networks, the choice of which depends on the needs of the application scenario. Since LSTM has the advantage of processing sequence data and solves the problem of gradient disappearance and gradient explosion generated by RNN when facing long sequences, LSTM is chosen to build the encoder-decoder model in this paper. The input sequence is encoded by LSTM to obtain the state
of the implicit layer, The state of the implied layer at t-1 is determined by the current input
and the implied layer state
at the moment t-1, and the calculation process is as follows:
After obtaining the implied layer state, the intermediate semantic vector is obtained by aggregating them, and the computation process is as follows:
The decoding process is based on the intermediate semantic vector and the output sequence to predict the output of the next moment, and the calculation process is as follows:
In the LSTM, the equation can be expressed as follows:
Where, denotes the nonlinear activation function, which is essentially equivalent to the LSTM network action and then processed by the SoftMax function,
denotes the decoder output value at the moment t-1, C denotes the decoder state at the moment, and denotes the intermediate semantic vector.
Content-Based Attention Mechanism
When using the Encoder-Decoder framework, the attention mechanism is often used to form the intermediate semantic vector in the aggregated implicit layer, which solves the problem that the Encoder part must compress the information of the whole input sequence into a fixed-length context, thus also solving the possible missing information of a long input sequence and the possible redundant information of a short input sequence. The basic idea is to calculate the similarity between the output value of the implicit layer at each moment and the state of the decoder at each moment to determine the weight of each part of the implicit layer in the process of aggregation to the intermediate semantic vector:
Where denotes the weight of the state
of the implicit layer at the moment t,
denotes the state of the implicit layer at the decoder side at the moment t, and the function
is to calculate the similarity of the sum. After obtaining the weights of each implicit layer hi at time t, the weights are multiplied with the corresponding implicit layers, and the final sum is obtained as the intermediate semantic vector under the content-based attention mechanism.
Copy Mechanism
When using the Encoder-Decoder framework, there is another problem that the words in the structured data are not fully utilized. Specifically, many words in structured data are very suitable for use in the final generated text, but they are often neglected due to their low frequency of occurrence. Therefore, the codec model often uses the copy mechanism to solve this problem, which allows the model to combine both generate and copy, and the model will choose whether to select the words to be generated from the vocabulary according to the probability or copy them directly from the input data in the decoding stage. The probability of copying is calculated as follows:
Where represents the result of the words in the input data after the encoding stage, and
indicates the state of the decoder. The other part needs to calculate the probability of generation from the vocabulary, which is calculated as in the formula, where
represents the state of the decoder at t. Finally, the two probabilities are added together and processed by the SoftMax function to form a new lexical probability distribution that expands the words in the input data into the original vocabulary, which is calculated:
Selection Mechanism
To solve the task of generating analytical texts for numerical data in a more targeted way, the proposed model incorporates the coarse-to-fine aligner selection mechanism and linked-based attention mechanism. The coarse to-fine aligner selection mechanism adds a pre-selection function for the content part of the structured data [field,content] to the content-based attention mechanism, which optimizes the selection of the descriptive content in the generated text. The linked-based attention mechanism models the relationship between the field part of the structured data [field,content], so that the model can maintain a reasonable description order when generating text.
When generating text based on structured data, whether using the traditional rule-based template approach or the data-driven end-to-end deep learning approach, the optimization is based on the same three parts: content planning, i.e., selecting the fields and contents to be described in the structured data; sentence planning, i.e., determining the description order of the selected descriptions in the generated text; and sentence implementation, i.e. based on the planning of the first two steps to generate the corresponding text.
In the content planning part, models based on structured data to text generation often use only content-based attention mechanism, coarse-to-fine selection mechanism assigns a probability to select the part based on calculating the attention weight of each part at the implicit layer, thus achieving the optimization of content selection. The field part of the data is not different from other types of data, while the content part is mostly or even entirely numerical data, which makes it impossible for the model to focus on and describe certain items of data during the training process. Therefore, the model proposed in this paper incorporates the coarse to fine selection mechanism.
Based on the content-based coarse degree of attention mechanism, each implicit layer is given the probability of being selected, and the fine degree of attention weight is obtained by calculating the product of the probability of selecting each part and the weight of each part under the content-based attention mechanism. Based on the characteristics of the content part of numerical data, this paper makes an adaptive change in the process of implementing this mechanism, firstly, the Embedding of both field and content parts also namely and is concatenated to get [;
], after getting the state of the implicit layer by Encoder, the implicit layer will enter the pre Coarse aligner calculates the attention weights of the two parts based on the embedding of the field part and the encoding vector of the sum to calculate the attention weights of the two parts respectively, which is calculated as in equation (9). The final output of coarse aligner is obtained by combining the two parts of weights and doing SoftMax processing, which is calculated as:
Experiments and Results
Experimental Setup
The current publicly available ELT datasets are fitted to tasks such as sentiment classification and text summarization, which will exclude certain ELT features for the task requirements and are not suitable for training domain-adapted language models in this paper. Learning the training idea of GPT2 model, this paper obtains about 200 M English language and literature teaching data (MR dataset and AR dataset) through an English education institution in China, where the data is processed in JSON format, one line represents one piece of data, and only simple pre-processing work is performed on the initial cluttered data in order to retain the integrity of the original features of the data, including the removal of emoticons and short texts with 5 characters. In order to retain the integrity of the original features of the data, only simple pre-processing work is performed on the initial cluttered data, including the removal of emoticons and short texts below 5 characters.
The model in this paper mainly contains a generator and a discriminator model, and the specific parameter settings are shown in . The training process is mainly divided into a pre-training phase and a confrontation training phase. In the pre-training phase, the MLE is used to pre-train the generator to a better state, and the Adam optimization algorithm is used for learning, with the learning rate set to 0.05 and the total number of rounds set to 150; in the adversarial training phase, the Adam optimization algorithm is also used to update the network parameters of the generator and discriminator, with the learning rate set to 0.0001 and the maximum number of rounds set to 2000. The whole network was implemented using the Tensorflow 1.5.0 deep learning framework, and the model was trained and tested on a workstation with an Intel(R) Xeon(R) Gold5115 CPU, 64 GB RAM and a single card NVIDIA(R) GTX2080Ti GPU.
Table 1. Parameter settings in the experiment.
Experimental Results
To verify the effectiveness of the method proposed in this paper, we conducted experiments on the MR dataset and the AR dataset respectively, and show the experimental results of the model on the real dataset MR and the real dataset AR, respectively. The results of each evaluation index are taken as the summed average of the corresponding results of all categories, and the average results of multiple experiments are taken as the results. Among them, CatGAN-AL denotes a generative adversarial network composed of a dual attention-based generative network and a discriminative network based on LSTM and CNN; RealGAN-AL denotes a generative adversarial network composed of a generative network based on RealFormer and a discriminative network based on LSTM and CNN.
From the experimental results, compared with the benchmark method, the method in this paper can better capture the contextual dependencies of the text in order to make the generated text with higher fluency. And it can alleviate the problem of text tending to be consistent when the text quality is improved to a certain extent and maintain the diversity of text.
To verify the effectiveness of each module of the model, the ablation experiments on MR dataset and AR dataset are conducted in this paper. Among them, w/o AOA denotes the removal of the dual attention module in the CatGAN-AL model generator; w/o LSTM denotes the removal of the LSTM-based global semantic feature extraction module in the CatGAN-AL model discriminator. The results of each evaluation metric are taken as the summed average of the corresponding results of all categories, and the average result of multiple experiments is taken as the result. show the results of the ablation experiments of the CatGAN-AL model on the 2 datasets, respectively.
The ablation experiments show that the joint introduction of the dual attention module and the global feature extraction module enables the model to fetch a better performance. The dual attention module can maintain the text diversity while the text quality is improved and prevent the generated text from tending to be consistent; the LSTM-based global feature extraction can improve the BLEU values of the generated samples, especially BLEU-4 and BLEU-5, indicating that the global feature extraction module can make the model pay attention to the contextual dependencies of the text and achieve the purpose of improving the fluency of the generated text. From the overall results, the method in this paper can maintain a certain amount of text diversity while improving the fluency of generated text, proving the effectiveness of the dual attention module and global feature extraction module in the model.
The value of λ in the evaluation function is a weighted sparsity that balances the quality and diversity of the generated text. To the effect of λ taking value on the model performance, we try to experiment on CatGAN-AL model using different λ values in the interval of [0.0001,0.1] on 2 datasets respectively, and the experimental results are shown in .
Table 2. Results of the parametric analysis of the model on the MR dataset.
Table 3. Parametric analysis results of the model on the AR dataset.
From the above experiments, as the λ value increases, the model pays more attention to the diversity of the generated text and achieves better results. However, it also weakens the focus on text quality and decreases in BLEU values. Therefore, to balance the diversity and fluency of generated text, λ is set to 0.01 in this paper is experiments.
Conclusion
Artificial intelligence and smart classroom technology have brought new development opportunities for English language and literature teaching, and in the process of teaching innovation and practice, teachers should actively improve their teaching concepts and teaching methods by combining the development trend of the times with a high degree of professional ethics and profound professionalism.
In this paper, we propose a smart classroom model of English language and literature that integrates local semantic features and global semantic features. By extracting local features and global features of the text, the method makes it possible to focus on the contextual dependencies of the text while obtaining key information of English language and literature, enhance the discriminator’s attention to the contextual dependencies of English language and literature, force the generator to focus on the overall semantics and generate fluent The proposed approach provides students with a good opportunity to develop their own English language and literature. The proposed method provides students with a good learning atmosphere and learning guidelines, enhances students’ enthusiasm and autonomy through various smart classroom technologies, helps students break through learning difficulties and master English learning skills, and thus improves students’ English language and literature comprehension and application skills, and nurtures many excellent talents for the increasingly frequent and close international exchanges.
While the proposed approach of integrating smart classroom teaching and AI technology in English language and literature education has many potential benefits, there are also several limitations that should be considered. These limitations include:
Access to technology: The use of AI technology and smart classroom teaching requires access to appropriate technology, which may not be available in all educational settings or for all students. This may create a digital divide, where some students have access to advanced learning resources while others do not.
Quality of AI tools: The effectiveness of AI-powered tools and technologies is highly dependent on the quality of the algorithms and data used to train them. Poorly designed or biased algorithms may produce inaccurate or irrelevant feedback, which could undermine the effectiveness of the smart classroom approach.
Overreliance on technology: While technology can be a powerful tool for enhancing learning, it should not replace the role of teachers in the classroom. Overreliance on AI tools and technologies may reduce the opportunities for personal interaction and communication between students and teachers, which is a crucial aspect of language learning.
Cultural appropriateness: The use of AI technology to simulate cultural contexts and experiences may not always be culturally appropriate or sensitive. It is important to ensure that the content and tools used in the smart classroom approach are respectful of diverse cultural perspectives and do not reinforce stereotypes or biases.
Ethical concerns: The use of AI technology in education raises ethical concerns around issues such as privacy, data security, and algorithmic bias. It is important to carefully consider these issues and develop appropriate safeguards to protect the rights and interests of students and educators.
In conclusion, while the smart classroom approach using AI technology has many potential benefits for English language and literature education, it is important to consider the limitations and potential challenges associated with this approach. By addressing these issues, educators can develop a more effective and inclusive approach to language learning that leverages the power of technology while respecting the cultural diversity and individual needs of their students.
Disclosure statement
No potential conflict of interest was reported by the authors.
Data Availability statement
Data will be provided upon request to the corresponding authors.
Additional information
Funding
References
- Barbounis, T. G., J. B. Theocharis, M. C. Alexiadis, and P. S. Dokopoulos. 2006. Long-term wind speed and power forecasting using local recurrent neural network models. IEEE Transactions on Energy Conversion 21 (1):273–1575. doi:10.1109/TEC.2005.847954.
- Bland, J. 2013. Children’s literature and learner empowerment. Children and Teenagers in English Language Education (CONCLUSION). International Research in Children S Literature 8 (1):93–95.
- Cheng, L., Z. G. Hou, Y. Lin. 2011. Recurrent neural network for non-smooth convex optimization problems with application to the identification of genetic regulatory networks. IEEE Transactions on Neural Networks. 22(5):714–26. doi:10.1109/TNN.2011.2109735.
- Chen, C., J. Jiang, R. Fu, L. Chen, C. Li, and S. Wan. 2021. An intelligent caching strategy considering time-space characteristics in vehicular named data networks. IEEE Transactions on Intelligent Transportation Systems 23 (10):19655–67. doi:10.1109/TITS.2021.3128012.
- Chen, C., J. Jiang, Y. Zhou, N. Lv, X. Liang, and S. Wan. 2022. An edge intelligence empowered flooding process prediction using internet of things in smart city. Journal of Parallel and Distributed Computing 165:66–78. doi:10.1016/j.jpdc.2022.03.010.
- Chen, C., H. Li, H. Li, R. Fu, Y. Liu, and S. Wan. 2022. Efficiency and fairness oriented dynamic task offloading in internet of vehicles. IEEE Transactions on Green Communications and Networking 6 (3):1481–93. doi:10.1109/TGCN.2022.3167643.
- Chen, C., Y. Zeng, H. Li, Y. Liu, and S. Wan. 2022. A multi-hop task offloading decision model in MEC-enabled internet of vehicles. IEEE Internet of Things Journal 10 (4):3215–30. doi:10.1109/JIOT.2022.3143529.
- Du, T. T. 2022. Teaching and learning literature in the english language curriculum in vietnamese university education: Problems and solutions. Journal of Psychology Research 12 (5):10. doi:10.17265/2159-5542/2022.05.005.
- Eser, J., P. Zheng, J. Triesch, and D. Durstewitz. 2014. Nonlinear dynamics analysis of a self-organizing recurrent neural network: chaos waning. PLos One 9 (1):e86962. doi:10.1371/journal.pone.0086962.
- Hasan, A. M., and Z. F. Hasan. 2019. Students’ perception towards literature integration in the english language departments at duhok and zakho universities. Footscray VIC: Australian International Academic Centre PTY, LTD. http://journals.aiac.org.au/index.php/alls/index
- Jiang, D., and J. Wang. 1993. A recurrent neural network for real-time semidefinite programming. Applied Mathematics and Computation 55 (1):89–100. doi:10.1016/0096-3003(93)90007-2.
- Kafipour, R. 2014. International journal of english language and literature studies. Aessweb Com 3 (3):99–116.
- Kayad, F. G. 2015. Teacher education: English language and literature in a culturally and linguistically diverse environment. Education Research & Perspectives 42: 286–328.
- Keiko, O. Z. A., and W. A. Akiko. 2016. Late night meals and obesity: A systematic review using english-language literature. Japanese Journal of Health Education and Promotion 24 (4):205–16.
- Kim, Y. H., F. L. Lewis, and C. T. Abdallah. 1997. A dynamic recurrent neural-network-based adaptive observer for a class of nonlinear systems. Automatica 33 (8):1539–43. doi:10.1016/S0005-1098(97)00065-4.
- Liang, X. B., and J. Wang. 2000. A recurrent neural network for nonlinear optimization with a continuously differentiable objective function and bound constraints. IEEE Transactions on Neural Networks 11 (6):1251–62. doi:10.1109/72.883412.
- Li, H., S. Y. Qu, and C. M. Scherpereel. 2016. Research progress in alliance stability: A content and comparative analysis of the english- and Chinese-language literature. International Journal of Strategic Business Alliances 5 (2):110–32. doi:10.1504/IJSBA.2016.079384.
- Liu, Q., and J. Wang. 2008. A one-layer recurrent neural network with a discontinuous hard-limiting activation function for quadratic programming. IEEE Transactions on Neural Networks 19 (4):558–70. doi:10.1109/TNN.2007.910736.
- Lukosevicius, M., and H. Jaeger. 2009. Reservoir computing approaches to recurrent neural network training. Computer Science Review 3 (3):127–49. doi:10.1016/j.cosrev.2009.03.005.
- Noori, M., and G. Mazdayasna. 2014. A triangulated study of target situation needs of iranian undergraduate students of english language and literature. Procedia - Social & Behavioral Sciences 98 (1):1374–79. doi:10.1016/j.sbspro.2014.03.555.
- Rodriguez, P., J. Wiles, and J. L. Elman. 1999. A recurrent neural network that learns to count. Connection Science 11 (1):5–40. doi:10.1080/095400999116340.
- Scott, H., and R. Gilmour. 2022. Badenglish: Literature, multilingualism and the politics of language in contemporary Britain. Review of English Studies. (310): 310.
- Stone, T. E., M. Mcmillan, and M. Hazelton. 2015. Back to swear one: A review of english language literature on swearing and cursing in western health settings. Aggression & Violent Behavior 25:65–74. doi:10.1016/j.avb.2015.07.012.
- Takizawa, Y., T. Fujita, and H. Kawai. 2016. Toward defining teacher research in english language teaching in Japan: A review from literature (Theoretical studies and experimental studies, projects, The 45th CELES Conference in Wakayama). Journal of the Chubu English Language Education Society 45 .
- Xia, Y., and J. Wang. 2005. A recurrent neural network for solving nonlinear convex programs subject to linear constraints. IEEE Transactions on Neural Networks 16 (2):379–86. doi:10.1109/TNN.2004.841779.
- Xiong, Z., and Z. Jie. 2005. A batch-to-batch iterative optimal control strategy based on recurrent neural network models. Journal of Process Control 15 (1):11–21. doi:10.1016/j.jprocont.2004.04.005.
- Xu, R., D. W. Ii, and R. Frank. 2007. Inference of genetic regulatory networks with recurrent neural network models using particle swarm optimization. IEEE/ACM Transactions on Computational Biology & Bioinformatics 4 (4):681–92. doi:10.1109/TCBB.2007.1057.
- Zhao, M., C. Chen, L. Liu, D. Lan, and S. Wan. 2022. Orbital collaborative learning in 6G space-air-ground integrated networks. Neurocomputing 497:94–109. doi:10.1016/j.neucom.2022.04.098.