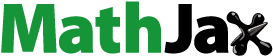
ABSTRACT
Risk management is essential to every organization’s management plan. It is the strategy by which organizations handle the risks involved with their actions to profit or avoid making decisions that will cost them financially in each activity. Identifying and mitigating potential digital threats and developing and implementing procedures to significantly reduce the likelihood of an organization being targeted by cyberattacks are at the core of effective risk management. This is especially true regarding the risks associated with an organization’s digital footprint. A particularly significant threat to reputation, but also at the same time indirect and direct costs, is the data breach that occurs when a security incident occurs about the data for which the organization is responsible, which results in a violation of confidentiality, availability, or the integrity of the data it manages. On the other hand, the rapid development of technology has transformed the agricultural industry, allowing for large-scale farming based on machine learning and other advanced tools. However, this transformation also exposes farms to cyber risks that can lead to costly data breaches. In this study, we propose a framework for incorporating explainable machine learning (exML) techniques into large-scale farming to enhance cyber risk analysis, mitigate cyber threats, and foster a sustainable rural economy. Specifically, given that an organization needs to implement the appropriate technical and organizational measures to avoid possible data breaches, this work presents an analytical stochastic modeling of risk with a multi-criteria objective function of low complexity, a factor in an incentive system, to model investment value and cost balancing. The motivation behind the proposed method is to improve cybersecurity, protect data and reputation, foster a sustainable rural economy, leverage explainable machine learning, and adapt to the changing technological landscape. By addressing these motivations, the method aims to provide a comprehensive and effective approach to cyber risk analysis in large-scale farming.
Introduction
Protecting sensitive information from being disclosed to unauthorized third parties has emerged as one of the most significant security challenges for businesses nowadays (Laube and Böhme Citation2017; Miao et al. Citation2021). Since the volume of data generated in the digital era is growing exponentially, data breaches are becoming more prevalent. In some contexts, theft or loss of digital media holding unencrypted data might be considered a data breach. This breach involves the violation of data and the interception of data, as well as the transfer of sensitive information to the information systems of a potentially hostile organization, such as a competitor or a foreign nation, where it may be decrypted and used in a manner not agreed upon by the entities that are the actual owners of the information in question. This breach also involves the transfer of sensitive information to the information systems of a potentially hostile organization, such as a competitor or a foreign nation (Zou et al. Citation2019).
In general, a security breach that results in the accidental or unlawful destruction, loss, alteration, unauthorized disclosure, or access to protected data transmitted, stored, or otherwise processed is one of the most severe modern problems, as it can result in high indirect or direct costs and irreparable problems in managing an organization’s reputation and solvency (Refsdal et al. Citation2015). To secure data, companies often must incur additional charges to take precautionary measures to prevent data breaches (Varshney et al. Citation2020)
Risk management processes have been designed ongoing throughout the project, particularly for all personnel called upon to manage sensitive data. Their use can be made in projects of any size, from very small (done by a single person) to very vast and complex (Telang Citation2015; Teymourlouei and Harris Citation2018) Although many problems can be addressed in advance, allowing the project manager to identify a specific path with distinct levels, risk management frequently fails to determine the exact cost of a data leak, resulting in the actual price being perceived a posteriori, which is often disastrous for an organization’s financial future (Lagazio, Sherif, and Cushman Citation2014).
According to this logic, risk analysis methods should include technology means for identifying prospective dangers and analyzing their capabilities so that the most significant ones may be controlled (Basallo, Estrada Senti, and Martinez Sanchez Citation2018; Refsdal et al. Citation2015). Also, have procedures for accurately identifying them on an ongoing basis so there can be resource allocation and assurance procedures corresponding to the associated risk level. In this spirit, this work presents an advanced cyber risk analysis prototype to prevent costly data breaches from business management information systems. It is analytical stochastic modeling of risk with a multi-criteria objective function of low complexity, which is set as a factor in an incentive system to model the balancing of investment and cost equilibrium (Liang et al. Citation2020; Torra and Torra Citation2017; Zhiru et al. Citation2021).
It is inspired by game theory (Akinwumi et al. Citation2017; Do et al. Citation2017; Mednikov et al. Citation2017), which deals with the study of elements that characterize situations of competitive interdependence with an emphasis on the decision-making process of more than one decision-maker opponent (Ahmadlou and Adeli Citation2010; Xinhe et al. Citation2008). Using simple calculations and logic, it is a scientific process that can study – and most likely predict – how individuals or groups of individuals make decisions in a competing field or environment.
The motivation behind the proposed method of incorporating explainable machine learning techniques into large-scale farming for cyber risk analysis is driven by several key factors:
Enhancing Cybersecurity: The primary motivation is to enhance cybersecurity in the agricultural industry. As the industry increasingly adopts advanced technologies and relies on digital systems, the risk of cyberattacks and data breaches becomes more significant. By integrating explainable machine learning techniques into risk analysis, the proposed method aims to identify and mitigate cyber threats effectively, thereby improving the overall cybersecurity posture of farms.
Protecting Data and Reputation: Data breaches can have severe consequences, including financial losses, reputational damage, and legal liabilities. The proposed method seeks to protect the data for which organizations are responsible and maintain the confidentiality, availability, and integrity of that data. By identifying potential vulnerabilities and implementing appropriate measures, the method aims to safeguard sensitive information and prevent costly breaches that could impact the reputation and trust of the organization.
Promoting Sustainable Rural Economy: A sustainable rural economy relies on the efficient and secure functioning of agricultural operations. By addressing cyber risks and enhancing cybersecurity practices, the proposed method aims to foster a sustainable rural economy. By reducing the potential financial losses and disruptions caused by cyber incidents, farmers and organizations can maintain operational continuity, preserve their economic viability, and contribute to the long-term sustainability of the agricultural sector.
Leveraging Explainable Machine Learning: The use of explainable machine learning techniques is motivated by the need to understand and interpret the risk analysis results effectively. By incorporating techniques that provide transparent and interpretable insights, farmers and stakeholders can better comprehend the factors contributing to cyber risks and make informed decisions regarding risk mitigation strategies. Explainability also promotes trust and acceptance of the risk analysis process among users, making it more accessible and actionable.
Adapting to Technological Advancements: The proposed method acknowledges the transformation of the agricultural industry through technological advancements, such as machine learning. By embracing these advancements and leveraging them for cyber risk analysis, the method aims to align risk management practices with the evolving digital landscape. It recognizes the need to adapt and utilize advanced tools and techniques to effectively address the emerging cyber threats that arise from increased connectivity and automation in large-scale farming.
The scientific innovation of the proposed framework lies in the integration of explainable machine learning (exML) techniques into large-scale farming to enhance cyber risk analysis and mitigation. While the agricultural industry has witnessed the transformation brought about by technology, including machine learning and advanced tools, it has also become vulnerable to cyber risks and data breaches. Therefore, the framework aims to address this specific challenge by introducing novel methodologies and approaches.
Incorporating exML Techniques: The integration of explainable machine learning techniques into the framework allows for transparent and interpretable risk analysis. By utilizing exML models, the framework can provide insights into the decision-making process of the system, enabling organizations to understand how risks are identified, assessed, and managed. This enhances the overall transparency and trustworthiness of the risk analysis process.
Analytical Stochastic Modeling of Risk: The framework introduces analytical stochastic modeling to quantify and assess cyber risks in large-scale farming. This modeling approach takes into account various factors and uncertainties associated with cyber threats, enabling a more comprehensive and realistic risk assessment. By incorporating stochastic modeling, the framework provides a more robust and accurate representation of risk probabilities and potential impacts.
Multi-Criteria Objective Function: The framework utilizes a multi-criteria objective function to balance investment value and cost in risk management decisions. This approach considers multiple criteria, such as the value of assets at risk, the potential financial impact of data breaches, and the cost-effectiveness of mitigation measures. By incorporating these criteria into the decision-making process, organizations can make informed choices that optimize resource allocation and risk reduction efforts.
Sustainable Rural Economy Focus: The proposed framework highlights the importance of fostering a sustainable rural economy through effective cyber risk management. By safeguarding sensitive agricultural data and protecting against data breaches, the framework contributes to building resilience and maintaining trust in the agricultural industry. This focus on sustainability aligns with broader societal goals of economic stability and longevity in rural areas.
The scientific innovation of this framework lies in the combination of exML techniques, analytical stochastic modeling, multi-criteria decision-making, and a focus on sustainable agriculture. By integrating these elements, the framework offers a novel and comprehensive approach to addressing cyber risks in large-scale farming, enhancing risk analysis, and promoting a sustainable rural economy.
The following sections of the paper are structured as follows: the second section of this article discusses the research studies that are related to the topic at hand; the third section is an in-depth analysis of the Cyber Risk Analysis Prototype; the fourth section presents the findings of the proposed method, and the final section the research is summed up in the conclusion section.
Related Research
A growing number of people in the Information Systems community and economic worlds are concerned about how best to spend their money on cyber security (Bates and Ul Hassan Citation2019; Musman and Turner Citation2018; Zhiru et al. Citation2021). There have been numerous previous studies, but none give a framework that combines risk analysis, user behavior, and big data analytics to provide a holistic approach to risk estimation and cyber insurance computation.
For example, Akinwumi et al (Akinwumi et al. Citation2017) surveyed game-theoretic models for managing cyber security risk. In this article, the challenges associated with modeling and some studies on the topic are discussed, as is the requirement for a game-theoretic approach to the management of cyber risk. The review outcomes illustrated the superior qualities and traits that set each model apart. In addition to this, it has come to light that the algorithms are in their infancy and require a large amount of development and improvement. The survey’s conclusions are presented on several existing models for a game-theoretic approach to the management of cybersecurity, with particular attention paid to the models’ advantages, disadvantages, opportunities, and threats. According to the findings, these systems are still in their early stages and require substantial further development. In addition, a synopsis of recent research on cyber risk management based on these methodologies is presented, emphasizing the motivations, targets, methods, and related restrictions. Because of the threats associated with cyber security change and the introduction of new technologies, it is essential to review and update the cyber security plan continually. Therefore, the primary focus of study in the future should be on experimentally validating these models and constructing models that address both actual and expected concerns or hazards to cyber security and some of the deficiencies of the works that have been examined.
Also, Musman and Turner (Musman and Turner Citation2018) introduced the Cyber Security Game, a strategy realized in software that quantifies cyber protection risks and utilizes this measure to find the ideal security solutions to deploy for any given expenditure level. Decreasing mission risk increases a system’s capacity to function in today’s disputed cyber environment. The grade is arrived at by first estimating the impacts of cyber events through a project impact model and then combining those results with the probability that assaults will be successful. Their game considers the widespread interconnectivity of cyber systems, in which defenders are required to protect against all multi-step attack paths. In contrast, attackers are simply the only ones to follow. It takes a game-theoretic approach by formulating a game that finds defensive options for mitigating the utmost cyber risk.
Do et al (Do et al. Citation2017) surveyed current game-theoretic methods for cyber security and privacy, classifying them as security or privacy approaches. They chose to research three significant applications of game theory in cyberspace security and anonymity to demonstrate how it is applied: cyber-physical security, authentication protocols, and privacy. They discussed the chosen works’ game concepts, characteristics, solutions, and their pros and disadvantages, from design through execution of the defensive systems. Additionally, they recognized some new patterns and research issues for future study. They aimed to familiarize the reader with cutting-edge research and diverse game-theoretic approaches to online security and privacy challenges. They studied the lengthy history of the development of some problems, such as survivability, denial of service, and data forwarding. They concluded that more research is necessary, given the fast evolution of communication technology (Sullivan Citation2019). Additionally, they reviewed new security topics such as cyber-physical safety, survivability, information exchange, and steganography, which have received less attention in the literature but are gaining interest lately. Finally, they discussed increasing risks in cyberspace and suggested future avenues for game-theoretic techniques.
Feng et al (Feng et al. Citation2018) suggested a framework for risk management in the blockchain service industry by offering cyber-insurance to shield the blockchain provider against double-spending assaults financially. They selected cyber insurance as a cost-effective method of mitigating cyber risks associated with assaults on blockchain networks. They evaluated a market for blockchain services consisting of infrastructure providers, blockchain providers, cyber-insurers, and customers. The blockchain provider acquires computer resources required to sustain the blockchain consensus from the infrastructure supplier, for example, a cloud, and then sells blockchain services to consumers. Additionally, they ran comprehensive simulations to ascertain the market entities’ performance at equilibrium. They intended further to research the long-run rivalry between blockchain providers and cyber-insurers (Piprani Citation2006).
Stephen Grey presented standard approaches for analyzing project contingencies, highlighting severe flaws in those that are only focused on risk occurrences or line-item ranges. He described the risk factor technique, eliminating these flaws and making the procedure more realistic and less time-consuming. He demonstrated how a conscientious approach might reduce the complexity of generating estimates of unknown numbers and more accurate evaluations of the range of likely outcomes. He explained how a principled approach might reduce the complexity of developing estimates of unknown numbers. It is predicated on paying close attention to the evaluation context and utilizing procedures that minimize the possibility of bias. The presentation contains graphics illustrating how He may implement the idea in several projects, including information technology and construction. When risk factor modeling and context-based range estimation are coupled, the pressure on analysts and their clients is reduced while producing more realistic and informative findings. depicts a risk factor modeling scenario (Cost and Schedule Risk Assessment – Risk Factor Modelling (Broadleaf 2022).
Finally, Panou et al (Panou, Ntantogian, and Xenakis Citation2017) proposed the RiSKi framework, a cyber investment management structure that incorporates detection and continuous monitoring of insiders’ social behavior, to the extent permitted by law, to proactively resolve implied anomalies and threats and their potential business impact and risks. Furthermore, it provides access to publicly available security incident data to assist organizations in improving their understanding of security and capacity to understand the threats and penalties connected with cyber breaches, allowing for a faster recovery period following an incidence. Furthermore, using a web crawler provides direct access to publicly available data on security incidents, allowing the company to advance its cybersecurity knowledge and understanding of the threats and implications associated with cyber breaches, ultimately allowing for rapid recovery from an event.
Cyber Risk Analysis Prototype
The proposed implementation is based on creating an objective function related to system modeling that simulates a conventional medium-sized enterprise (Schmitz and Pape Citation2020). This organization manages sensitive personal data related to health. The model aims at the optimal long-term design of the system risk analysis (Chua, Sheng Teh, and Herbland Citation2021; Torra and Torra Citation2017; Yun-Jie, Wen-Qi, and Ling Citation2018). This includes finding new investments in network business resources and the appropriate integration of units in the system. With the given technological constraints or strategy constraints, the maximization of the profit function – minimization of the cost function is achieved. The objective part of the model is to maximize the present value of the profit by balancing the solutions of securing valuable information with the calculation of costs in case of data leakage (Hassanzadeh, Biddle, and Marsen Citation2021; Joseph Citation2017). The quantity demanded and the equilibrium value is the locus of the points that arise because of the problem of optimizing the organization’s utility. To simulate the locus, we considered a linear function with parameters
.
The cost function is an ascending and non-linear function.
In the short-term study, the organization has contracted investments of a given range from which it is obliged to repay whether to use them. If they need bigger ones, the company is looking for them. Therefore, finding additional investments is more complicated and involves more significant investment efforts (Akinwumi et al. Citation2017; McCord et al. Citation2020; Xenya and Quist-Aphetsi Citation2019). On the other hand, in the medium-long-term horizon, the company can evaluate various benefits that offset operating costs. In addition, through the optimization problem, the company can change its required investments in the long run. Therefore, the cost curve is increasing but smoother than the short-term horizon. The system is studied from 2010 (base year) to 2030. For the first years of study, in which it is difficult to find investments, the cost functions resemble the short-term horizon, while as the years go by, the cost functions resemble the long-term horizon (Laube and Böhme Citation2017; McCord et al. Citation2020; Zhiru et al. Citation2021). In conclusion, the factors that affect the value of the objective function are:
Revenue is calculated as follows:
And the costs at which they add up:
The cost of purchasing equipment:
The variable cost of storage units, which changes as the amount of data stored changes:
The fixed cost of data centers:
The capital cost of investments in new data centers (is the initial expenditure required for new data center construction):
In the renovation of old data centers:
The capital cost of investments in cloud infrastructure:
The cost of using the network infrastructure:
So, the final objective function that describes the optimal long-term risk analysis design of the system is (Bhoyar and Yadav Citation2017; Do et al. Citation2017; Refsdal et al. Citation2015):
The above function represents the present value of cost investment balance for implementing the cyber risk analysis prototype to prevent costly data breaches from the business management information system (Myklebust and Ove Båtevik Citation2022; Xenya and Quist-Aphetsi Citation2019).
To make this implementation more legible for audiences, let’s delve into a detailed explanation:
Objective Function: An objective function is a mathematical expression that defines the goal to be maximized or minimized in a system. In this case, the objective function is designed to capture the objectives and constraints specific to a convention-sized enterprise. These objectives can include maximizing profits, minimizing costs, optimizing resource allocation, or achieving a desired level of customer satisfaction.
System Modeling: System modeling refers to the process of creating a mathematical representation of the convention-sized enterprise. This model encompasses various components, such as operational processes, resources, constraints, and relationships among different elements within the enterprise. The model captures the dynamics and interactions of these components to simulate the behavior and performance of the enterprise.
Simulation: By incorporating the objective function into the system model, the proposed implementation allows for simulation of the convention-sized enterprise. Simulation involves running the model using input data and parameters to simulate the enterprise’s operations over time. This simulation generates outputs that reflect the enterprise’s performance under different scenarios and conditions.
Analysis and Optimization: The simulation outputs are then analyzed using the objective function. The objective function serves as a quantitative measure to evaluate the performance of the enterprise based on the simulation results. It enables the identification of optimal solutions or decisions that align with the enterprise’s goals and constraints. This analysis can help identify potential areas for improvement, uncover bottlenecks, or optimize resource allocation for better outcomes.
Decision Support: The objective function, combined with the simulation outputs and analysis, provides decision support for the convention-sized enterprise. It assists in making informed decisions by quantifying the trade-offs between different objectives and considering the impact of various factors on the enterprise’s performance. Decision-makers can use this information to identify strategies, policies, or interventions that can enhance the enterprise’s overall performance and achieve desired outcomes.
By employing the proposed objective function and system modeling approach, the implementation enables a quantitative analysis of a convention-sized enterprise. It provides decision-makers with a structured and data-driven framework to evaluate and optimize the enterprise’s operations. This approach offers insights into the enterprise’s performance, facilitates scenario testing, and supports evidence-based decision-making.
It’s important to note that the specifics of the objective function and system model would need to be defined in accordance with the unique characteristics and goals of the convention-sized enterprise. This would involve considering relevant factors such as revenue streams, cost structures, resource capacities, customer demands, and any other pertinent variables that drive the enterprise’s performance.
For the prototype in question, it must be proved that there is a solution’s uniqueness to demonstrate its application’s usefulness.
The Cyber Risk Analysis Prototype is a detailed tool that aims to assist organizations in assessing and managing cyber risks effectively. It provides a comprehensive framework for analyzing potential threats, vulnerabilities, and their associated impacts, allowing organizations to make informed decisions about risk mitigation and resource allocation. The prototype incorporates various components and methodologies to achieve its objectives:
Threat Identification: The prototype begins by identifying potential cyber threats relevant to the organization’s digital footprint and operations. This involves conducting a thorough analysis of the threat landscape, considering both external and internal threats. External threats may include malicious actors, hacking attempts, or social engineering attacks, while internal threats may involve insider threats or accidental data leaks.
Vulnerability Assessment: Once the threats are identified, the prototype performs a vulnerability assessment to determine the weaknesses and vulnerabilities within the organization’s systems, infrastructure, and processes. This assessment may involve conducting penetration testing, vulnerability scanning, or security audits to identify potential entry points for cyber attacks.
Risk Quantification: The prototype then quantifies the identified cyber risks by assessing the probability of occurrence and the potential impact of each risk. It takes into account factors such as the likelihood of successful attacks, the value of assets at risk (e.g., sensitive data, intellectual property), and the potential financial, operational, or reputational consequences of a successful breach. By assigning quantitative values to these factors, the prototype provides a means to compare and prioritize risks based on their severity.
Risk Mitigation Strategies: Based on the identified risks and their associated impact, the prototype recommends risk mitigation strategies. These strategies may include technical measures such as implementing firewalls, intrusion detection systems, or encryption protocols, as well as organizational measures like employee training, incident response plans, and access controls. The prototype provides insights into the effectiveness and cost-effectiveness of various mitigation options to aid decision-making.
Cost-Benefit Analysis: To assist organizations in making informed decisions about risk mitigation investments, the prototype incorporates a cost-benefit analysis. It evaluates the potential costs of implementing mitigation measures against the expected benefits in terms of risk reduction and potential cost savings from averting or minimizing cyber incidents. This analysis helps organizations strike a balance between the investment required for mitigation and the potential impact of a cyber breach.
Monitoring and Adaptation: The prototype emphasizes the importance of continuous monitoring and adaptation to evolving cyber risks. It provides mechanisms for organizations to track changes in the threat landscape, update vulnerability assessments, and reassess risk levels periodically. This ensures that the risk analysis remains up to date and aligned with the dynamic nature of cyber threats.
Reporting and Visualization: The prototype offers reporting and visualization capabilities to present the results of the risk analysis in a clear and understandable manner. This includes generating visual representations of risk levels, heat maps highlighting areas of high vulnerability, and comprehensive reports that summarize the identified risks, recommended mitigation strategies, and cost-benefit analysis.
The Cyber Risk Analysis Prototype integrates these components into a cohesive toolset, providing organizations with a systematic and structured approach to assess, prioritize, and mitigate cyber risks. By leveraging this prototype, organizations can enhance their overall cybersecurity posture, reduce the likelihood and impact of cyber incidents, and make informed decisions to protect their digital assets and reputation.
Modeling and Simulation
A function is convex if and only if it holds (Akinwumi et al. Citation2017; Zhiru et al. Citation2021):
All constraints of the causal model are linear functions, so it is concluded that they are convex. In addition, the sets x resulting from a convex function as:
it is also convex.
For two convex sets, it holds that their intersection is a convex set, so it follows that the space of feasible solutions as the intersection of the convex sets defined by each constraint is a convex set.
In this model, the optimization concerns the maximization of the objective function. When the space of feasible solutions is convex and the problem to be solved is to find the maximum, then it is enough for the objective function to be genuinely concave or, respectively, its first derivative to be genuinely decreasing so that the solution is unique. The objective function calculates the equilibrium value of cost investments. This results from subtracting the cost function from the business revenue function. The company’s income as a function of the requested value is a hollow function with the result that the first derivative is decreasing. The first derivative of the cost function is incremental, as can be deduced from the addition of genuinely increasing variable and capital cost functions (Akinwumi et al. Citation2017; Refsdal et al. Citation2015, OTM et al. Citation2006).
Without damage to the generality, there are q1, q2 with q1 < q2 such that:
Otherwise, the company would have negative profits, not a long-term steady state.
The first derivative of the objective function is genuinely decreasing, and given its strength, there is a period such that the company’s profits are positive. In addition, there is a point where the first derivative of the revenue function is equal to the cost function, that is, such that:
This point is a unique solution to the objective function since its first derivative is genuinely monotonous.
To simulate and draw the conclusions of the proposed methodology, we will show that the expected total costs are equal to the typical total investment, thus creating a value for equalizing cost investments. To reach this conclusion, we must first prove a proposition that states that the expected payout and the expected virtual benefit of a strategic player are equal. Specifically, in each environment of a unique parameter with distributions of personal value F1, F2, …, Fn each mechanism Strategy Incentive Compatible (SIC) (x, p), for each strategic player i and each personal value v − i from the rest strategic players apply (Hassanzadeh, Biddle, and Marsen Citation2021; Laube and Böhme Citation2017):
We employ a specific theory inspired by game theory to prove the following thesis. Games are a mathematical approach to studying problems relating to making decisions in conflict and cooperative settings. The fundamental premise is that “intelligent” and “reasonable” action exists. A player is described as “intelligent,” which means he knows exactly how to play the game, and “reasonable,” which means he plays intending to maximize his benefit. It is critical to underline that each player’s profit in the game is dependent not only on his own choices but also on the choices of the other players (who are not necessarily treated as his opponents). In the suggested game, two or more rational players with competing aims choose modes of action, resulting in situations of competitive interdependence (Chen and Bo-Han Citation2018; Hou, Driessen, and Sun Citation2015).
To apply and simulate the proposed template, according to Myerson, the amount of payment for a strategic player i in a SIC mechanism with a distribution rule x continuous and generable for the vector of personal values, the following relation gives v (Bhoyar and Yadav Citation2017; Laube and Böhme Citation2017):
Let a random strategic player i. We will have:
Changing the order of completion, we have how:
Then we perform factor integration and receive:
So, it becomes evident that for z that follows Fi distribution, it holds that:
With the help of the above proposal, we will prove the equilibrium value of cost investments. Specifically, we must confirm that in any environment of a unique parameter with distributions of personal value F1, F2, …, Fn and for each SIC mechanism, it holds that:
So for the proof of the above proposal, we have:
Due to the linearity of the average value, we have:
The second term of equality is the most easily maximized mathematical expression. In practice, we observe that it is as if we have replaced vi with ϕi(vi), which proves the truth of the hypothesis about the equilibrium value of investment investments that we assumed (Basallo, Estrada Senti, and Martinez Sanchez Citation2018; Mednikov et al. Citation2017).
The options approach, in which business risk preferences are incorporated through the use of a utility function, is the methodology that provides the most accurate simulation of the behavior of an organization. This utility function can replicate either a greater aversion to risk, as in the approach for maximizing the chance of avoiding injury, or a lesser aversion to risk, as in the method that uses the value at risk. Since there are no limits that are inherently present in the evaluation research or the prototype presented and developed, organizations may effectively use this method to solve problems in extended prototype models.
Discussion
The ability of the proposed approach to generalize to other scientific fields depends on several factors. While the core principles and methodologies of incorporating explainable machine learning techniques into risk analysis can be applied across different domains, there are considerations to be aware of regarding generalization:
Domain-specific Considerations: Different scientific fields have unique characteristics, data types, and specific cybersecurity challenges. Therefore, it is important to carefully consider and adapt the proposed approach to the specific requirements and nuances of each domain. This may involve adjusting the risk analysis models, incorporating domain-specific data sources, and accounting for sector-specific regulations and standards.
Data Availability and Quality: The availability and quality of data play a crucial role in the generalization of any machine learning approach. While the proposed approach emphasizes the importance of data for risk analysis, different domains may vary in terms of the availability and quality of relevant data. It is necessary to assess the data landscape in each field and determine whether sufficient data exists to support effective risk analysis and the application of machine learning techniques.
Contextual Understanding: Generalization requires a contextual understanding of the unique characteristics and requirements of each scientific field. It is essential to collaborate with domain experts and stakeholders to gain insights into the specific challenges, risk factors, and industry practices within the field. This collaboration can help tailor the approach to the specific needs of the domain and ensure that the risk analysis models and techniques align with the domain-specific context.
Interpretability and Explainability: The proposed approach emphasizes the use of explainable machine learning techniques, which are valuable for understanding and interpreting the risk analysis results. However, the interpretation of results may vary depending on the specific domain and the stakeholders involved. It is important to consider the interpretability requirements and preferences of each field and develop approaches that provide meaningful and actionable insights for decision-making within that context.
Validation and Benchmarking: To ensure the generalizability of the approach, it is essential to validate its effectiveness and performance across different scientific fields. Conducting rigorous evaluations, comparing results against existing methodologies, and benchmarking against domain-specific standards can provide evidence of the approach’s generalizability and its ability to address cybersecurity risks effectively.
While the proposed approach has the potential to be generalized to other scientific fields, it requires careful consideration of domain-specific factors, data availability, and the contextual understanding of each field. Collaborative efforts with domain experts, thorough validation, and adaptability to specific requirements will contribute to successfully applying the approach in different scientific domains. The core principles and benefits of the approach can be adapted and extended to address cyber risk management in various domains. Here are a few examples:
Healthcare: The healthcare industry deals with sensitive patient data and faces significant cybersecurity challenges. Applying the proposed approach can help healthcare organizations identify and mitigate cyber threats, protect patient privacy, and maintain the integrity of medical records. Explainable machine learning techniques can aid in understanding the risk factors and decision-making processes related to cybersecurity in healthcare.
Finance: The financial sector handles vast amounts of sensitive data and faces constant threats of cyberattacks. The proposed approach can assist financial institutions in analyzing and managing cyber risks, protecting customer financial information, and ensuring the integrity of financial transactions. By integrating explainable machine learning techniques, risk analysis results can be interpreted and used to enhance cybersecurity measures in financial systems.
Energy and Utilities: The energy and utilities sector relies on critical infrastructure that is increasingly connected and susceptible to cyber threats. Implementing the proposed approach can help identify vulnerabilities and potential points of attack in energy systems. It can aid in assessing the risks associated with power grids, smart grids, and other utility networks, enabling organizations to prioritize investments in cybersecurity and develop effective risk mitigation strategies.
Manufacturing and Industrial Systems: The industrial sector is undergoing a digital transformation with the adoption of technologies such as Industrial Internet of Things (IIoT) and automation. These advancements increase the complexity of cybersecurity risks. The proposed approach can be utilized to analyze cyber risks in manufacturing processes, supply chains, and industrial control systems. Explainable machine learning techniques can provide insights into potential vulnerabilities and support decision-making for risk mitigation in these settings.
Transportation and Logistics: The transportation and logistics industry heavily relies on interconnected systems and networks for efficient operations. This dependency exposes the industry to cyber threats. The proposed approach can be applied to analyze and mitigate cyber risks in transportation infrastructure, autonomous vehicles, supply chain management, and other related areas. Explainable machine learning can provide valuable insights into the security of these systems and aid in designing robust cybersecurity measures.
These are just a few examples, but the applicability of the proposed approach extends to other scientific fields where cyber risk management is crucial. By adapting the principles of incorporating explainable machine learning techniques into risk analysis, organizations in various domains can enhance their cybersecurity practices, mitigate risks, and safeguard sensitive data and critical systems.
Conclusions
Uncertainties regarding the external factors that affect investments are addressed by working through different scenarios and doing sensitivity analysis. The causal models utilized in risk analysis studies are considered the most relevant. They are unable to calculate the actual costs associated with a data breach. It will likely realize the actual price of such a leak a posteriori, which can sometimes be detrimental to the organization’s future financial prospects. In this work, we suggested a unique prototype for cyber risk analysis to prevent expensive data breaches from occurring in business management information systems. Analytical stochastic modeling of risk with a low complexity multi-criteria objective function is used to model investment value and cost balancing. This modeling technique is a component of an incentive system.
Organizations will be able to simplify further the model proposed in the future if they return to the single object procedures of the objective function and assume that all of the strategic objectives follow independent and regular equilibrium distributions. This will allow them to use an equilibrium distribution rule that provides value to the organization and a higher positive virtual valuation. Also, according to the allocation rule, they will handle the item for which the virtual valuation drops to zero. As a result, they may bring the projected costs into parity with one another such that the numbers follow a lognormal distribution.
While the proposed approach of incorporating explainable machine learning techniques into large-scale farming to enhance cyber risk analysis has its merits, it is important to consider its limitations as well. Here are some potential limitations of the approach:
Complexity and Implementation Challenges: Implementing an analytical stochastic modeling framework that incorporates explainable machine learning techniques can be complex and resource-intensive. It may require significant expertise and technical infrastructure, which could pose challenges for adoption, especially for smaller farms with limited resources.
Availability and Quality of Data: The effectiveness of machine learning algorithms heavily relies on the availability and quality of data. In the agricultural sector, obtaining comprehensive and reliable data for risk analysis might be challenging. Data collection and management processes need to be carefully established to ensure the accuracy and completeness of the data used in the analysis.
Interpretability and Explainability: While the focus on explainable machine learning is commendable, achieving true interpretability and explainability in complex machine learning models can be difficult. Some advanced machine learning algorithms, such as deep learning models, often operate as black boxes, making it challenging to understand and explain their decision-making processes. Ensuring that the generated insights are understandable and actionable for farmers and stakeholders may require additional efforts.
Evolving Cyber Threat Landscape: The field of cybersecurity is continuously evolving, and new threats and attack vectors emerge regularly. Developing a framework that can effectively adapt to these evolving threats and stay up-to-date with the latest cybersecurity practices and technologies can be a significant challenge. Regular updates and continuous monitoring would be necessary to keep the risk analysis and mitigation strategies relevant.
Cost and Affordability: Implementing sophisticated cybersecurity measures and integrating machine learning techniques can involve substantial costs. This could potentially limit the accessibility and affordability of the proposed approach for smaller farms or organizations with limited budgets.
Human Factors and User Adoption: Successful implementation of any risk management framework requires user buy-in and adoption. Ensuring that farmers and relevant stakeholders understand the value and benefits of the proposed approach, and are willing to adopt and follow the recommended practices, is crucial for its effectiveness. Adequate training, education, and support may be necessary to facilitate user acceptance.
It’s important to consider these limitations while evaluating the proposed approach and to address them appropriately to enhance its effectiveness and applicability in real-world agricultural settings. In addition, there are several avenues for future research to further enhance and refine this approach. Some potential areas for future research include:
Integration of Real-Time Monitoring: Investigate the integration of real-time monitoring systems and sensors in large-scale farming operations to enhance the detection and response capabilities to cyber threats. This could involve exploring how data from various sensors, such as weather, soil moisture, or equipment performance, can be analyzed in conjunction with cyber risk analysis to provide a comprehensive and proactive defense against cyberattacks.
Incorporation of Threat Intelligence: Explore the incorporation of threat intelligence sources and frameworks into the risk analysis process. This would involve leveraging external sources of information on emerging cyber threats specific to the agricultural industry and integrating them with the risk analysis framework. By continuously monitoring and updating threat intelligence, farmers and organizations can better understand and mitigate evolving cyber risks.
Scalability and Adaptability: Investigate methods to ensure that the proposed approach remains scalable and adaptable to different farming systems, sizes, and geographical locations. Developing flexible models and frameworks that can accommodate variations in data availability, farm infrastructure, and risk profiles would make the approach more accessible and applicable to a wider range of agricultural contexts.
Privacy-Preserving Techniques: Address the challenges related to privacy and data protection in the context of implementing machine learning techniques for cyber risk analysis. Explore privacy-preserving methods, such as federated learning or differential privacy, to protect sensitive data while still enabling effective risk analysis. This would ensure that farmers’ and organizations’ data remains secure and confidential throughout the analysis process.
User-Friendly Interfaces and Decision Support Systems: Develop user-friendly interfaces and decision support systems that present the results of the risk analysis in a clear and actionable manner. This would aid farmers and stakeholders in understanding the findings, making informed decisions, and implementing appropriate risk mitigation strategies effectively. The interfaces should be designed to accommodate different levels of technical expertise and be accessible across different devices and platforms.
Economic Impact Assessment: Conduct studies to evaluate the economic impact and return on investment of implementing the proposed approach. Assess the cost-effectiveness of different risk mitigation strategies, taking into account the potential financial losses due to cyber incidents and the benefits gained from enhanced security measures. This would provide stakeholders with valuable insights into the economic viability and benefits of adopting the proposed approach.
By addressing these research areas, we can further advance the understanding and implementation of cyber risk analysis in large-scale farming, ensuring robust cybersecurity practices and supporting the sustainable growth of the agricultural industry in the digital era.
Disclosure Statement
No potential conflict of interest was reported by the author(s).
Additional information
Funding
References
- Ahmadlou, M., and H. Adeli. 2010. Enhanced probabilistic neural network with local decision circles: A robust classifier. Integrated Computer-Aided Engineering 17 (3):197–2165. doi:10.3233/ICA-2010-0345.
- Akinwumi, D. A., G. B. Iwasokun, B. K. Alese, and S. A. Oluwadare. 2017. A review of game theory approach to cyber security risk management. Nigerian Journal of Technology 36 (4):1271–85. doi:10.4314/njt.v36i4.38.
- AWeSOMe, CAMS, COMINF, IS, KSinBIT, MIOS-CIAO, MONET, OnToContent, ORM, PerSys, OTM Academy Doctoral Consortium, RDDS, SWWS, and SeBGIS. 2006. Montpellier, France October 29-November 3, 2006. Proceedings, Part II.
- Basallo, Y. A., V. Estrada Senti, and N. Martinez Sanchez. 2018. Artificial intelligence techniques for information security risk assessment. IEEE Latin America Transactions 16 (3):897–901. doi:10.1109/TLA.2018.8358671.
- Bates, A., and W. Ul Hassan. 2019. Can data provenance put an end to the data breach? IEEE Security & Privacy 17 (4):88–93. doi:10.1109/MSEC.2019.2913693.
- Bhoyar, D. G., and U. Yadav. 2017. Review of jamming attack using game theory. Paper read at 2017 International Conference on Innovations in Information, Embedded and Communication Systems (ICIIECS), Coimbatore, India.
- Chen, T.-S., and W. Bo-Han 2018. Gateway selection based on game theory in internet of things. Paper read at 2018 International Conference on Electronics Technology (ICET), Chengdu, China.
- Chua, H. N., J. Sheng Teh, and A. Herbland. 2021. Identifying the effect of data breach publicity on information security awareness using hierarchical regression. IEEE Access 9 (9):121759–70. doi:10.1109/ACCESS.2021.3107426.
- Do, C. T., H. Nguyen, C. H. Tran, A. K. Charles, K. A. Kwiat, E. Blasch, S. Ren, N. Pissinou, and S. Sitharama Iyengar. 2017. Game theory for cyber security and privacy. ACM Computing Surveys 50 (2):1–37. doi:10.1145/3057268.
- Feng, S., W. Wang, Z. Xiong, D. Niyato, P. Wang, and S. Shuxun Wang. 2018. On cyber risk management of blockchain networks: A game theoretic approach. IEEE Transactions on Services Computing 14 (5):1492–504. doi:10.1109/TSC.2018.2876846.
- Hassanzadeh, Z., R. Biddle, and S. Marsen. 2021. User perception of data breaches. IEEE Transactions on Professional Communication 64 (4):374–89. doi:10.1109/TPC.2021.3110545.
- Hou, D., T. Driessen, and H. Sun. 2015. The Shapley value and the nucleolus of service cost savings games as an application of 1-convexity. IMA Journal of Applied Mathematics 80 (6):1799–807. doi:10.1093/imamat/hxv017.
- Joseph, R. C. 2017. Data breaches: Public sector perspectives. IT Professional 20 (4):57–64. doi:10.1109/MITP.2017.265105441.
- Lagazio, M., N. Sherif, and M. Cushman. 2014. A multi-level approach to understanding the impact of cyber crime on the financial sector. Computers & Security 45 (45):58–74. doi:10.1016/j.cose.2014.05.006.
- Laube, S., and R. Böhme. 2017. Strategic aspects of cyber risk information sharing. ACM Computing Surveys 50 (5):1–36. doi:10.1145/3124398.
- Liang, X., Q. Taiyue, Z. Jin, S. Qin, P. Chen, and Y. Liu. 2020. Risk assessment system based on fuzzy composite evaluation and a backpropagation neural network for a shield tunnel crossing under a river. Advances in Civil Engineering 2020 (2020):1–14. doi:10.1155/2020/8840200.
- McCord, M., P. Davis, J. McCord, M. Haran, and K. Davison. 2020. An exploratory investigation into the relationship between energy performance certificates and sales price: A polytomous universal model approach. Journal of Financial Management of Property and Construction 25 (2):247–71. doi:10.1108/JFMPC-08-2019-0068.
- Mednikov, M. D., N. A. Sokolitsyna, A. S. Sokolitsyn, and V. P. Semenov. 2017. Game theory model of forming enterprise development strategy in market environment uncertainty. Paper read at 2017 XX IEEE International Conference on Soft Computing and Measurements (SCM), St. Petersburg, Russia.
- Miao, Y., C. Chen, L. Pan, Q.-L. Han, J. Zhang, and Y. Xiang. 2021. Machine learning–based cyber attacks targeting on controlled information: A survey. ACM Computing Surveys 54 (7):1–36. doi:10.1145/3465171.
- Musman, S., and A. Turner. 2018. A game theoretic approach to cyber security risk management. The Journal of Defense Modeling and Simulation 15 (2):127–46. doi:10.1177/1548512917699724.
- Myklebust, J. O., and F. Ove Båtevik. 2022. Special needs provision and economic independence among young adults with disabilities: A longitudinal study. European Journal of Special Needs Education 37 (5):715–28. doi:10.1080/08856257.2021.1974552.
- Panou, A., C. Ntantogian, and C. Xenakis. 2017. RiSKi: A framework for modeling cyber threats to estimate risk for data breach insurance. Paper read at Proceedings of the 21st Pan-Hellenic Conference on Informatics, Larissa Greece.
- Piprani, B. 2006. Using orm-based models as a foundation for a data quality firewall in an advanced generation data warehouse. Paper read at On the Move to Meaningful Internet Systems 2006: OTM 2006 Workshops: OTM Confederated International Workshops and Posters
- Refsdal, A., B. Solhaug and K. Stølen. 2015. Cybersecurity. Cyber-Risk Management 4: 29–32.
- Schmitz, C., and S. Pape. 2020. LiSRA: Lightweight security risk assessment for decision support in information security. Computers & Security 90 (90):101656. doi:10.1016/j.cose.2019.101656.
- Sullivan, C. 2019. EU GDPR or APEC CBPR? A comparative analysis of the approach of the EU and APEC to cross border data transfers and protection of personal data in the IoT era. Computer Law & Security Review 35 (4):380–97. doi:10.1016/j.clsr.2019.05.004.
- Telang, R. 2015. Policy framework for data breaches. IEEE Security & Privacy 13 (1):77–79. doi:10.1109/MSP.2015.12.
- Teymourlouei, H., and V. E. Harris. 2018. Organization risk management on network vulnerability and potential data breach. Paper read at 2018 International Conference on Computational Science and Computational Intelligence (CSCI), Las Vegas, NV, USA.
- Torra, V., and V. Torra. 2017. Privacy models and disclosure risk measures. Data Privacy: Foundations, New Developments and the Big Data Challenge 28: 111–89.
- Varshney, S., D. Munjal, O. Bhattacharya, S. Saboo, and N. Aggarwal. 2020. Big data privacy breach prevention strategies. Paper read at 2020 IEEE International Symposium on Sustainable Energy, Signal Processing and Cyber Security (iSSSC), Gunupur Odisha, India.
- Xenya, M. C., and K. Quist-Aphetsi. 2019. Decentralized distributed blockchain ledger for financial transaction backup data. Paper read at 2019 International Conference on Cyber Security and Internet of Things (ICSIoT), Accra, Ghana.
- Xinhe, X., Y. Wang, J. Liu, and X. Zhang. 2008. Analysis on the achievement milestones and limitations of Game Theory. Paper read at 2008 Chinese Control and Decision Conference, Yantai, China.
- Yun-Jie, J., C. Wen-Qi, and H. Ling. 2018. Risk identification and simulation based on the bayesian inference. Paper read at 2018 4th Annual International Conference on Network and Information Systems for Computers (ICNISC), Wuhan, China.
- Zhiru, L., X. Wei, H. Shi, Y. Zhang, Y. Yan, and D. Gong. 2021. Security and privacy risk assessment of energy big data in cloud environment. Computational Intelligence and Neuroscience 2021 (2021):1–11. doi:10.1155/2021/2398460.
- Zou, Y., S. Danino, K. Sun, and F. Schaub. 2019. YouMight’Be affected: An empirical analysis of readability and usability issues in data breach notifications. Paper read at Proceedings of the 2019 CHI Conference on Human Factors in Computing Systems, Glasgow Scotland Uk.