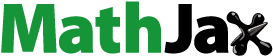
ABSTRACT
Consumers have begun to move their attention away from product functioning and toward value probably extracted from items. Companies have begun to use customer service systems (CSS) in response to this trend, which are business models that give clients with not solitary tangible items as well as intangible facilities. Even with substantial investigation on Smart CSS frameworks, rare of this frameworks considered customers active data producers actively creating data for the Smart CSS. Furthermore, the majority of them offered a generic remedy rather than a personalized one. To classify customer service systems, performance metrics, such as precision, accuracy, F1-score, Recall (Sensitivity), Specificity, Error rate, Computation time, and RoC are considered. The performance of AETGCN-NGOA-CSS approach attains 19.11%, 24.12% and 28.13% high specificity, 24.93%, 23.04%, and 9.51% lower computation time, 15.2%, 25.45% and 13.91% higher ROC and 8.45%, 20.98%, and 27.55% higher accuracy compared with existing methods, such as developing personalized recommendation system in smart product service system depend on unsupervised learning model (CSS-BERT), Cognitive Decision-Making approaches in Data-driven Retail Intelligence: Consumer Sentiments, Choices, Shopping Behaviors (CSS-CDMA), e-Commerce Online Intelligent Customer Service System under Fuzzy Control (CSS-FFNN), respectively.
Introduction
Higher income consumers prefer to spend 33% more on experience-based products these days, according to Deloitte’s consumer method. This insight shows that consumers have dramatically shifted their focus from the functioning of items to ancillary services (Chang et al. Citation2023; Chen, Ding-Bang, and Li Citation2023; Zhang et al. Citation2022). As a consideration of this mentality, Product Service System (PSS) has become increasingly common in modern society. The PSS business model is a novel one that combines unique products with planned services, with the service components often playing the most significant role (Dehghani, Hubálovský, and Trojovský Citation2021; Sharma et al. Citation2023). In order to provide clients even more value than previously, PSS is able to furnish them with high-quality intangible services in addition to physical items (Zhang et al. Citation2023). Due to its focus on services but not products, PSS is also possible to reduce resource consumption and waste levels Deep learning methods and big data analytics are also growing stars in the fields (Tseng et al. Citation2021). To provide customers with customized services depend on data created from their day-to-day lives, businesses today could create Smart Product Service Systems, include PSS, data-driven techniques (Zhang and Ming Citation2022a).
The study provides valuable insights into changing consumer preferences, specifically the shift toward experience-based products (Cong et al. Citation2023). By highlighting that, higher income consumers are willing to spend more on such products, the research acknowledges the evolving mind-set of consumers (Zhang et al. Citation2023). The study recognizes the increasing prevalence of PSS in modern society (Yuan, Chang, and Han Citation2023). It not only introduces the PSS business model but emphasizes the significance of planned services (Ren et al. Citation2023), within this model. This understanding contributes to a nuanced comprehension of how businesses can deliver value beyond physical products (Zhang and Ming Citation2022b). The research underlines the environmental benefits of PSS by reducing resource consumption and waste levels (Dong et al. Citation2023). This insight adds to the discourse on sustainable business practices, showcasing how service-oriented models can contribute to environmental conservation (Haber and Fargnoli Citation2021). The study identifies deep learning methods and big data analytics as pivotal components in the contemporary business landscape (Sakao and Neramballi Citation2020). The integration of these technologies is highlighted in Smart-PSS and CSS, emphasizing the role of data-driven techniques (Fargnoli and Haber Citation2023), including machine learning and deep learning models (Watanabe, Okuma, and Takenaka Citation2020). It presents a tangible example of a data-driven, individualized product – a wearable mask that evaluates fitness and monitors respiratory states. This showcases how Smart-PSS, combined with data-driven techniques, can lead to the creation of personalized and innovative solutions. Prospective application of Smart-PSS in auto maintenance and insurance (Iriarte et al. Citation2023). The idea of using GPS to alert repair businesses and employing text mining and picture analysis for quotes represents a forward-thinking approach in service innovation. A gap in current smart PSS research, highlighting that discussions mostly revolve around service distribution procedures rather than considering big data and client interactions. This recognition points toward areas that need further exploration. The aim is to encourage businesses to create its own smart PSS or gradually adapt existing models, thereby contributing to the evolution of business strategies in the digital age. The utilization of formless data and Natural Language Processing methods distribute modified services for consumers. This sheds light on the potential of advanced technologies in enhancing the customer service.
The typical customer service methods can be separated as three sets: (1) one to-one voice services for seats; (2) order schemes for several intersections; (3) mail box message. It is evident that the determination of typical customer service approaches has unable to keep up with ever expanding demands of company. Thus, to actively respond to the transformation of the customer service model, a new solution is desperately needed to increase customer satisfaction and response efficiency and the topic is “a new generation of intelligent customer service system research.”
Subsequently, in this work AETGCN-NGOA-CSS is incorporated for getting better results in Customer Service System. These motivate us to carry out this research work. Considering the brief background that was covered, it is clear that there is an increasing need for NLP to be used more widely across a range of industries. So, examining the effective NLP customer service systems across industries provides scholars, researchers, and business professionals with important information about NLP applications. The aim is to classify well suited customer service system in several business service schemes and offer future directions. The main contributions are listed as follows,
In this manuscript, introduce an Optimized Attention Enhanced Temporal Graph Convolutional Network, denoted as AETGCN-NGOA-CSS, designed for Intelligent Customer Service Systems employing Natural Language Processing (NLP) technology. The initial dataset is sourced from the Zhwiki Dataset, encompassing a Doc2vec model capable of capturing semantic meanings and the topics associated with input words.
The input data undergoes a user identification process, capturing the customer service journey, including maps, account interactions, and customer emotions tied to various actions. Subsequently, an Explore Key is generated to encourage customer engagement, fostering activities such as commenting, posting, and providing feedback on social media platforms.
Following the generation of the Explore Key, the collected Doc2vec model is channeled into the Attention Enhanced Temporal Graph Convolutional Network (AETGCN). This network efficiently categorizes suitable Customer Service Schemes through NLP. To enhance the performance of the model, the weight parameters are optimized using the Northern Goshawk Optimization Algorithm (NGOA). This optimization step aims to refine the network’s capacity to classify and address customer service needs effectively.
Continual paper is structured as: section 2 portrays the literature survey, section 3 explains the proposed method, section 4 proves the result and discussion, section 5 gives the conclusion.
Literature Survey
Numerous researches were presented in the literature on deep learning basis Intelligent Customer Service System depend on NLP; a few recent works are revised below.
Chiu et al. (Citation2021) have presented Developing personalized recommendation system in smart product service system depending on unsupervised learning model. The approach includes few steps: unsupervised NLP assess user-providing data to close the research gap, (ii) a method for making recommendations that incorporates deep learning to provide clients with tailored solutions. Customers have a dual role as active data producers and service recipients, creating value in partnership with service providers. The advantages of the suggested strategy were supported by a case study of tourist praise. The primary impact of the investigation was creation of customized smart PSS approach that might produce a win-win outcome for all participants. It attains lower Error Rate. It attains higher Computation Time.
Kliestik, Kovalova, and Lăzăroiu (Citation2022) have presented Cognitive Decision-Making Algorithms in Data-driven Retail Intelligence: Consumer Sentiments, Choices, Shopping Behavior. Here, signifying how machine learning processes improve efficacy of data dispensation system, then the advance literature on cognitive policy-making processes in data-driven retail intelligence. A quantitative evaluation was conducted throughout the performance utilizing exploration phrases “data-driven retail intelligence” + “cognitive policy-making algorithm,” “consumer sentiment,” “consumer choice,” and “shopping behavior.” The selected 21 largely empirical sources by excluding sources with dubious conclusions, results that could not be replicated, inaccurate content, or sources with similar names. Here, PRISMA reporting quality evaluation tool was used for evaluating the methodological quality include AMSTAR, Distiller SR, MMAT, and ROBIS. It attains higher ROC. It attains lower Precision.
Wei and Zhou (Citation2021) has presented e-Commerce Online Intelligent Customer Service System depend on Fuzzy Control. It examines dialogue structure depend on speech action model, associations semantic vector model of question sentence depending on dialogue configuration to comprehend question sentence, aids in enhancing accurateness of feedback provided by Internet shopping customer service robot. It uses statistical techniques assess trial data validate effectiveness of online customer support method it has built. It attains higher accuracy. It attains lower ROC.
Kim, Lim, and Hsuan (Citation2023) have presented from technology enablers to circular economy: Data-driven understanding analysis of servitization and product – service systems in Industry 4.0. The exact themes were learned, recognized utilizing machine learning technique, important references suggested were carefully observed generate cutting-edge summary. Enabler-engineering-goal framework was generated, total of ten essential study parts were selected. The study stimulates future academic discussions and industrial transformation by contributing to the clarification of schematized view of scattered studies on servitization then PSSs in Industry 4.0 crosswise several disciplines. Around the world, product-based businesses make an effort to incorporate services into their products, using “servitization” as a primary approach. It attains higher recall. It attains lower F1-Score.
Tan et al. (Citation2021) have presented Research on Knowledge Driven Intelligent Question Answering System for Electric Power Customer Service. The construct knowledge graph in area of electric customer service, the approach creates a knowledge graph with more than 15,000 items and 20,000 links. Intelligent question-answering submission construction created using the knowledge graph and includes a number of functional modules, including those for business process processing, natural language processing and dialogue procedure setup. Increase the openness, effectiveness of information recovery services for electric customer care industry, as well as the sophistication of customer service inquiries. It attains higher specificity. It attains lower Precision.
Cong et al. (Citation2022) have presented machine learning-based iterative design method to automate user satisfaction degree calculation in smart product-service system. It was possible to intelligently determine the happiness level of each individual user by utilizing vast amounts of in-context data collected throughout the usage phase. Secondly, a data-driven approach allows for more flexible changes to be made to Smart PSS, which consists of mutually digitalized services, physical apparatuses. So that automate calculation of user satisfaction in the Smart PSS environment, here it suggests iterative enterprise technique depend on machine learning. Along with this suggested methodological framework, surgical robot utilized flexible ureteroscopy shown as an example, overcoming the problems with subjectivity and laborious expert assessment in traditional procedures. The industrial firms of today may benefit greatly from these principles as they construct Smart PSS. It attains higher ROC. It attains lower Recall.
Li et al. (Citation2021) have presented a data-driven reversible framework for achieving Sustainable Smart product-service systems. It examines the important components of the construction of maintainable smart PSS from broader perspective managing cyber-physical resources. To construct Sustainable Smart PSS, data-driven alterable architecture provided here to maintainable use high-value and context-dependent information and knowledge. To enable decision-making, optimization across extended else circular lifespan, four-step context-aware method in framework, containing obligation elicitation, solution suggestion, solution estimation, and knowledge evolution is implemented. Maintainable improvement of smart 3D printer is used as an illustration to show the benefits and viability of the suggested framework. It attains lower Computation Time. It attains higher Error Rate.
Xie, Li, and Wang (Citation2022) have presented digital smart product service system and case study of mining industry: MSPSS. The smart product-service system for mining sector, included smart product sub system, stake holders, smart service sub system, smart policy-making sub system. For policy-making, product collection, operation, preservation, Analytical Hierarchy Process (AHP), Virtual Reality (VR) were utilized. Utilizing AHP and VR for decision-making, the system offers digital enterprise, virtual imitation, planning, debugging. Iterative optimization produces interactive design outputs, providing a robust, trustworthy solution for challenging mining situations. It attains higher Specificity and lower Precision.
Ngai et al. (Citation2021) have suggested an intelligent knowledge-based chatbot for customer service. A prototype system was built in real-world setting. The outcomes of system prototypeevaluation were reasonable and aid the contention that the system was effectual. It discussed about the difficulties encountered, the lessons discovered, and the theoretical and management ramifications of system installation. The approach achieves a higher specificity but a lower Precision, indicating its success in accurately identifying relevant information while maintaining openness in responses.
Nie, Wang, and Xiong (Citation2021) have suggested Research on intelligent service of customer service system (CSS). CSS have progressed from manual to intelligent, capable of transmitting files and videos. Simultaneously, the system implements a knowledge base under the processing of handling issues with customers. The CSS undertakes the task of processing control within the enterprise. The objective was to leverage customer data to produce corporate goods and leverage the knowledge base to comprehend consumer needs. Network architecture of intelligent CSS was introduced to present a reference for the construction. It provides greater precision with greater computational time.
Li et al. (Citation2021) have introduced Application with Development of NLP Service in Intelligent Customer Service System. Key technologies of intelligent CSS, like speech recognition, machine learning, NLP, and introduce the application status of NLP service technology in intelligent CSS. It provides higher sensitivity with lower accuracy.
William et al. (Citation2023) have suggested Framework for design and implementation of chat support system using natural language processing. A customer support chatbot named “Anjore” (pronounced as Λn.d3o:r) means “light” was developed from the Chhattisgarh State Power Distribution Company Limited. The chatbot was established in django web frame work with spaCy NLP library for Python to recognize utterances in English language. It provides lower computational time with lower accuracy.
Proposed Methodology
In this section, Attention Enhanced Temporal Graph Convolutional Network espoused Research of Intelligent Customer Service System depend on NGOA (AETGCN-NGOA-CSS) is discussed. The block diagram of the proposed AETGCN-NGOA-CSS is portrayed in . It comprises Dataset, Preprocessing, Classification, and Optimization. The comprehensive discussion about this is given below.
Data Collection
In this section datas are collected from Zhwiki Dataset (https://www.kaggle.com/datasets/a24998667/zhwiki-cleaned). The Dataset chooses to use the Doc2vec model, which can capture semantic meaning and topic of input words, to turn reviews then users’ needs to great dimensional vectors after gathering the necessary data in the previous stage. This work used the emerging approach genism, trained the model on 340,000 Wikipedia articles (zhwiki dataset) over training period. Model accomplished utilizing 40 epochs, output dimension is set at 50. The input phrases are fed into this model during the inference phase and yield matching 50-dimensional vectors immediately.
Identify User Requirements from Existent CSS
The service companies may use customer journey maps to study the thoughts that go through consumers’ minds when they use their goods or services (Chandraraju and Jeyaprakash Citation2022). A technique to visualizing the customer journey is the customer journey map. It makes use of images and procedural techniques to illustrate how customers may feel when using items or receiving services (Zhou et al. Citation2022). The customer journey map enables businesses to examine their services from the viewpoint of their clients before reviewing their current lack of customer service procedures as a benchmark for development. Updated customer journey map to assist service providers in looking at the steps consumers take. First, service providers should break down the service process into its several steps and act as if they are one of their clients. Second, the customer journey map must also take into account the important activities and touch points that consumers will experience. Third, consumers may have emotions related to each action they participated in, and service providers must put themselves in the customers’ place to understand those emotions of customer.
Explore Key Data
After determining the consumers’ needs, the service providers must decide whether to address those needs directly or adapt their present services to do so. When service providers identify their customers’ pain spots, they may begin looking for the data require. Source of the data originate from a variety of areas, such as comments and postings on social media sites, quick consumer feedback, available data from governmental else instructive organizations. Because of ease the internet, a lot of data from online sources frequently well-ordered, making it affordable for service providers to obtain. However, it’s possible that internet data isn’t as complete as what service provider’s need. Services provider’s urged to gather required data on their peculiar deal with this problem. For instance, one may collect restaurant evaluations by writing appraisal information on Google Maps. Benefit of gathering data independently that appropriately satisfies their needs, but doing so also takes time and costs money for service providers.
Classification by Attention Enhanced Temporal Graph Convolutional Network (AETGCN)
In this sector, Intelligent Customer Service System Based on Attention Enhanced Temporal Graph Convolutional Network is discussed. Attention mechanisms allow the model to assign varying levels of importance to different temporal graph nodes or edges. This improves the network’s ability to focus on the most relevant information for a given task, leading to more accurate and context-aware predictions. Temporal Graph Convolutional Networks (TGCNs) are adept at capturing temporal dependencies in data. The attention enhancement can further refine the model’s ability to understand and leverage complex temporal dynamics, making it more suitable for time-series data. Attention mechanisms can contribute to personalized recommendations or predictions by emphasizing individual preferences or patterns within the temporal graph. This is particularly beneficial in customer service systems or smart-product service systems where personalization is crucial for user satisfaction. Intelligent customer service system is based on AETGCN method, which may be enhanced to become a customer service classification recognition method. The label information from the authentic and fake comments is integrated into a data suppression and fixed-real-time convergence to the AETGCN. Furthermore, compared to exponential or finite time convergence, fixed-real-time convergence generate with conditional information. Then, it is adjusted to the output type of AETGCN. This is expressed in Equationequation (1)(1)
(1) ,
where represents feature of node on graph
;
signifies weighting operation that generates weight vector depending on input;
is the normalizing term, which is equal to the matching subset’s cardinality and
is a node’s graph convolution output
. This is revealed in Equationequation (2)
(2)
(2) ,
where symbolizes adjacency matrix of label
;
signifies adjacency matrix of label;
set to 0.001 to evade empty lows natural languages in customer service;
is a weight matrix made up of weight vectors of several output channels;
denotes node’s graph convolution output and
represents node feature on the graph. Modeling of temporal dependency is yet another important issue for customer service systems. Currently, AETGCN is mostly utilized neural network model for processing sequence data. Therefore, in order to extract implied information from time intervals, by using GRU cell as fundamental network. By utilizing the ATGCN, which is based on GRU and can simultaneously examine temporal dynamics and spatial correlation of nodes. This is represented in Equationequation (3)
(3)
(3) ,
where signifies hidden state at time
afterward customer service in language layer;
denotes the information which is gained from customer serviced at time;
denotes memory con- tent storing at time
;
represents the graph convolution process;
catching gait information of customer service instant;
to capture historical customer dynamics, exploit natural language information at same time and
is the information from the prior state is integrated with the present status. Then, the formula is derived as Equationequation (4)
(4)
(4)
where denotes the
frame of input sequences;
denoted internal operator of ATGCN is graph convolutional design;
denoted as a hidden states’ capacity for discrimination, a spatial attention layer used;
denotes identification of recognition, and
denotes the identification of emotion recognition. By taking the hidden state at time, the ATGCN determines the gait status at time
. The update gate controls how much status data from a past time is integrated into the present state. Then, the data kept in memory at the moment
. The proposed customer service system model able to exploit different parts of significance in natural language attention on customer service language. Then the formula is derived as Equationequation (5)
(5)
(5)
where signifies hidden state at time
afterward customer service in language layer;
denoted as reflection noise scalars of every task, respectively;
for indicating the importance of the
term and
denoted as the Bias Vector. Then the Equationequation (5)
(5)
(5) is elaborated and then the formula is derived as Equationequation (6)
(6)
(6)
where the scores for signifying importance of
joints;
to capture historical customer dynamics, exploit natural language information at same time;
denotes hidden state at time
before language attention layer;
symbolizes hidden state at time
afterward customer service in language layer;
,
are the learnable parameter matrixes;
,
are the bias vectors. Here
term is considered to be normalized of the function term. By considering the
term, the formula is derived as Equationequation (7)
(7)
(7)
where signifies regularized diagonal matrix then
set to 0.001 to evade empty lows; scores
for signifying the significance of
joints and
denoted as significance of information providing altered frames.
Develop Smart CSS
The service providers previously identified problems that their clients have and the best model to use to address those issues using the data that has been gathered. The final phase of the suggested technique is to motivate service providers to create comprehensive solution for clients. Solution might take many different forms, such web pages else other software submissions, entirely common interfaces used by service providers to give services to clients. Finally, AETGCN describes the natural language processing in customer service. Owing to its convenience, pertinence, the artificial intelligence-basis optimization algorithm is considered in the AETGCN classifier. In this work, NGOA is applied to enhance the AETGCN, and also tuning the weight with bias parameter of AETGCN. The Step-by-step processing of NGOA is given below.
Optimization Using Northern Goshawk Optimization Algorithm
Weight parameter of proposed AETGCN is optimized using the proposed NGOA. It is a novel nature-inspired algorithm. If the algorithm is designed to efficiently explore the solution space, it may have an advantage in finding global optima, especially in complex and multi-dimensional problem spaces. Efficient convergence is a key factor in optimization algorithms. If the Northern Goshawk Optimization Algorithm demonstrates faster convergence compared to other algorithms, it can be considered advantageous, particularly in real-time or resource-constrained applications. The algorithm’s robustness in handling noisy or dynamic environments can be an advantage. If it can adapt well to changes in the optimization landscape, it may outperform other algorithms. It was inspired from Northern Goshawk hunting behavior and attacking techniques’ toward its variety of prey. The northern goshawk has a two-part hunting technique. In the first stage is approaches prey quickly afterward spotting it then second stage is pursues the victim for brief period of time.
Stepwise Process of NGOA
Here, step by step processing is defined to get individual value of AETGCN based on NGOA. Initially, NGOA makes the equally distributing populace to optimize the optimum parameterof AETGCN. The optimal solution is updated by NGOA and the associated flowchart is given in .
Step 1: Initialization
The initial populace of NGOA is, initially generated through randomness. Then, the formula is derived as Equationequation (5)(5)
(5)
where denotes vector of attained objective function values;
signifies objective function value attained by
proposed solution;
denotes population of northern goshawks;
signifies count of populace members,
Considered as the
proposed solution.
Step 2: Random generation
The input weight parameter developed randomness utilizing NGOA after initialization.
Step 3: Fitness Function
It creates random solution from the initialized values. This is calculated using optimizing parameter and is represented in Equationequation (9)(9)
(9) ,
Step 4: Exploration Phase
The feasible search space of the problem begins to shrink down toward their best prey’s best location. It entails the feasible new location of the problem which begins to shrink down the prey’s best location. In this phase, NGOA will approach in searching of prey’s movement location by observing their movements. Additionally, because the new location is determined at random, NGOAs exploration power is increased. Then, the formula is derived as Equationequation (10)(10)
(10) and (Equation11
(11)
(11) )
where denoted as its
dimension;
signifies random count 1 or 2;
are random parameter to generate randomness of NGOs behavior in search and update;
denotes positioning of prey for ith northern goshawk and
implies objective function. By elaborating Equationequation (10)
(10)
(10) , the formula is derived as Equationequation (11)
(11)
(11) ,
where signifies new denoted status for
proposed solution;
is denoted as objective function value depend on
phase of NGO;
signifies
proposed solution,
is its objective function value.
Step 5: Exploitation phase for optimizing:
It entails NGOA hunting strategy from one place to other by observing the movement toward their prey. The NGOA in this area will search best area toward their global best new location along with the leaders. NGOA would move to their new position according the preys. Then the algorithm provides a better solution for their prey’s best location, then the potential increases during the exploitation phase. So, the NGOAs moving position is derived mathematically. Then, the formula is derived as Equationequation (12)(12)
(12) , (Equation13
(13)
(13) ) and (Equation14
(14)
(14) ).
where denotes
dimension;
is implicit that hunting closed to an attack location through radius;
signifies random parameter of numbers which used to generate randomness of NGOs behavior in search and
update and
variable indicated by ith solution. This is expressed in Equationequation (10)
(10)
(10) ,
where signifies iteration counter;
denotes maximum number of repetitions,
implies hunting near to attack location through radius. By elaborating Equationequations (9)
(9)
(9) and (Equation10
(10)
(10) ), the formula is derived as Equationequation (11)
(11)
(11)
where denotes new denoted status for
proposed new generated solution;
signifies objective function with respect to 2nd phase of NGO,
denotes
proposed solution.
Step 6: Termination
The weight parameter value of generator from Attention Enhanced Temporal Graph Convolutional Network is optimized by utilizing NGOA; and it will repeat step 3 until it obtains its halting criteria
. Then AETGCN-NGOA-CSS effectively assesses the quality of customer service system in natural language with higher accuracy by diminishing the computational period and error.
Result and Discussion
The experimental results of AETGCN-NGOA-CSS method are discussed in this section. The simulation is activated in Python using PC through Intel Core i5, 2.50 GHz CPU, 8GB RAM, Windows 7 under zhwiki dataset. The obtained outcomes of the proposed AETGCN-NGOA-CSS approach is analyzed with existing systems like, Developing personalized recommendation system in smart product service system depend on unsupervised learning model (CSS-BERT) (Chiu et al. Citation2021), Cognitive Decision-Making approaches in Data-driven Retail Intelligence: Consumer Sentiments, Choices, Shopping Behaviors (CSS-CDMA) (Kliestik, Kovalova, and Lăzăroiu Citation2022), e-Commerce Online Intelligent CSS under Fuzzy Control (CSS-FFNN), respectively.
Data Description
Zhwiki is a noted Chinese data set for domain finding derived from Wikipedia. It comprises texts from 7 fields: “Business and Commerce” (BUS), “Government and Politics” (GOV), “Physical and Mental Health” (HEA), “Law and Order” (LAW), “Lifestyle” (LIF), “Military” (MIL), and “General Purpose” (GEN). It has 26,280 documents separated as training, validation and testing. The dataset contain the textual content of Wikipedia articles in Chinese. This can include information on a wide range of topics. Information such as article titles, categories, revision history, and other metadata are included. While the primary language is Chinese, articles that include content in multiple languages, especially the topic involves international subjects. This datasets include information about the revision history of articles, allowing users to track changes over time.
Performance Measures
The performance of proposed approach is examined utilizing the given performance metrics.
Accuracy
It defines identification rate, which are correctly categorized. This is depicted in Equationequation (15)(15)
(15) ,
where TP symbolizes True Positive, TN symbolizes True Negative, FP signifies False Positive, and FN signifies False Negative.
Precision
This estimates positive result count while detecting the intrusion and is expressed in Equationequation (16)(16)
(16) ,
F1-Score
The performance equation is provided in and the evaluation parameter of F1-score is analyzed. This is exhibited in Equationequation (17)(17)
(17) ,
Sensitivity
Recall is a performance metric commonly used in binary classification tasks, such as medical tests, where the goal is to correctly identify the negative cases (nonevents or healthy individuals). This is represented in Equationequation (18)(18)
(18) ,
Error Rate
The rate of error is said to be the ratio of true positive cases to true negative cases that is incorrectly forecasted. Then, the formula is derived as Equationequation (20)(20)
(20)
Performance Analysis
The simulation result of AETGCN-NGOA-CSS is shown in . The performance of AETGCN-NGOA-CSS is analyzed with existing CSS-BERT, CSS-CDMA and CSS-FFNN models.
depicts the analysis of Accuracy. AETGCN-NGOA-CSS method attains 8.45%, 20.98%, and 27.55% higher Accuracy compared to the existing method CSS-BERT, CSS-CDMA, and CSS-FFNN, respectively.
depict analysis of Precision. AETGCN-NGOA-CSS method attains 20.69%, 29.64%, and 21.786% higher precision compared to the existing methods CSS-BERT, CSS-CDMA, and CSS-FFNN, respectively.
depicts the analysis of F1-Score. AETGCN-NGOA-CSS method attains 28.34%, 22.78%, and 27.7%greater F1-Score compared to the existing methods CSS-BERT, CSS-CDMA, and CSS-FFNN, respectively.
shows the Recall analysis. AETGCN-NGOA-CSS method attains 25.98%, 21.98%, and 24.25% higher Recall compared to the existing methods CSS-BERT, CSS-CDMA, and CSS-FFNN, respectively.
portrays the specificity analysis. AETGCN-NGOA-CSS method attains 19.11%, 24.12% and 28.13% higher specificity compared to the existing methods CSS-BERT, CSS-CDMA and CSS-FFNN respectively.
shows the error rate analysis. AETGCN-NGOA-CSS method attains 23.23%, 26.45%, and 22.19% lower Error Rate compared to the existing methods CSS-BERT, CSS-CDMA, and CSS-FFNN, respectively.
depicts analysis of computation Time. AETGCN-NGOA-CSS method attains 24.93%, 23.04%, and 9.51% lower Computation Time compared to the existing CSS-BERT, CSS-CDMA, and CSS-FFNN, respectively.
depicts the analysis of ROC. AETGCN-NGOA-CSS method attains 15.2%, 25.45%, and 13.91% lower ROC compared to the existing CSS-BERT, CSS-CDMA, and CSS-FFNN models, respectively.
Discussion
This study proposes a smart PSS method based on deep learning and used a modified customer traveling map to identify user necessities. The casestudy pertaining to taxi operatives is also included to illustrate the procedure’s phases, and questionnaire experiments are conducted to confirm the effectiveness of the proposed recommendation system. A novel product service system type with platform-oriented product service system was created in the existing approaches, emphasizing the presentation of individualized solutions along a virtual platform that permits communication amongst service providers and clients. Emulating this approach, the proposed structure is a platform for passengers, taxi drivers with the goal of generating market demand and producing win-win scenario for aforementioned roles. For dependable service design, an evolving Smart PSS cannot rely only on machine or human intelligence. Three main obstacles must be overcome for a Smart PSS to be developed and implemented: (i) hybrid design, (ii) hybrid intelligence, (iii) hybrid value. When designing a Smart PSS, the self-adaptability with context-alertness must be deemed. Smart PSS addresses linking, gathering, calculation, communications and co-creation, these are termed as 5Cs. There are two ways that the proposed structure can integrate people into the system. Firstly, this method inspires service providers to determine target customer wants by using service design tools. Second, the system makes advantage of information supplied by the user to offer a personalized recommendation system. The 5Cs are met in the proposed architecture when users add to their customized services. The proposed approach can benefit both tourists and taxi operators, and also companies close to the tourist places. The proposed approach allows passengers to travel from the standpoint of taxi operators for the entire day and allows them to plan their routes, which allows them to more effectively assign their drivers. Additionally, this technology could simplify consumer demand forecasts and significantly lower the idle rate of taxis. From the perspective of surrounding retailers, they might not be included in many blog posts or television shows that offer recommendations, but they nonetheless provide excellent services or products. If Google Maps reviews of nearby attractions are closely aligned with users’ interests, then users can find them with certainty while looking for them. The manuscript highlights the integration of cutting-edge technologies, specifically the Optimized AETGCN and the Northern Goshawk Optimization Algorithm (NGOA). This amalgamation reflects a forward-thinking approach to leveraging artificial intelligence and optimization techniques in the realm of customer service. The incorporation of the Zhwiki Dataset, coupled with the utilization of the Doc2vec model, showcases a data-driven methodology. This not only emphasizes the importance of large-scale data for training models but also signifies a commitment to capturing semantic meanings and topics from input words. The research addresses the user identification requirement by delving into the entire customer service process. By exploring customer journeys through maps, accounts, and emotions related to actions, the study takes a holistic view, acknowledging the multifaceted nature of user interactions. The generation of the Explore Key introduces an interactive element, encouraging customer engagement in various online activities such as commenting, posting, and providing feedback on social media platforms. This demonstrates an understanding of the importance of customer participation in shaping service experiences. The transmission of the collected Doc2vec model to the Attention Enhanced Temporal Graph Convolutional Network (AETGCN) reflects the application of advanced neural network architectures. AETGCN, as introduced, is employed for the effective classification of well-suited Customer Service Systems using Natural Language Processing. The optimization of weight parameters using the Northern Goshawk Optimization Algorithm (NGOA) contributes to the efficiency and fine-tuning of the model. This optimization step is crucial for enhancing the performance of the Customer Service System. The research employs a comprehensive set of performance metrics. This thorough evaluation aims to identify the most effective model for Customer Service Systems based on NLP, providing a quantitative basis for comparison. The overall objective of the research is to contribute to the development of a highly effective Customer Service System, informed by NLP and advanced machine learning techniques. The focus on metrics such as accuracy and precision underscores the commitment to creating a reliable and precise system.
Conclusion
An Optimized Attention Enhanced Temporal Graph Convolutional Network espoused Research of Intelligent Customer Service System under NGOA is successfully executed in this paper. The proposed AETGCN-NGOA-CSS method is activated in Python with zhwiki dataset. This study used human-provided data along deep learning algorithms to create a smart product service system concept. In quickly evolving big data epoch, the proposed system is capable of handling unstructured data (text). The four-step process starts with using a customized customer journey map to identify the target consumers’ hidden pain points. This map is meant to help service providers put themselves in the consumers’ shoes, reconsider the initial procedure, depict the customers’ emotional journey, and identify any pain points. After, service providers investigate semantic data including appropriate methods to build a better service procedure. Lastly, the pain points must guide the development of the solution. This research offers a smart product service system strategy that involves value co-creation among the users and service providers. To manage unstructured data, it also integrates deep learning techniques. A case study with questionnaire investigate is included to confirm the efficacy of proposed approach. In spite of the benefits, this research still has some spaces for enhancements. Firstly, this approach use service designing tools to identify user’s pain points, but no data objective to aid the arguments. Secondly, this approach use un-supervised learning approaches to store the labeling data cost but it is feeble for scaling the presentation of the method, because lack of ground truth. Thirdly, the amount to which users improved was not calculated in this study, and a model is necessary to assess changes before and after utilizing the proposed approach. The performance of proposed AETGCN-NGOA-CSS approach contains 20.69%, 29.64%, and 21.786% high precision; 28.34%, 22.78%, and 27.7% high F-1 Score; 23.23%, 26.45% and 22.19% low error rate; 25.98%, 21.98%, and 24.25% high Sensitivity when analyzed to the existing methods like CSS-BERT, CSS-CDMA, and CSS-FFNN respectively. Application of the AETGCN-NGOA-CSS method on classifying customer service system has lessened the error through 18–24% for most of the data sets. In the future, this study will be enhance using the given steps: (ii) to identify the original service’s pain points, this study will employ text mining techniques or questionnaire experiments to identify real-world pain points, making them more credible and convincing, (ii) this study will use great supervised learning approaches, like BERT, XLM-Roberta to create user-input sentences representation ideally, also train in Google Map reviews, (iii) an additional experiment will be carried out as part of this study to compare user satisfaction levels before and after the proposed system is used. If the aforementioned procedures are followed, the proposed approach might be effective as well as tailored to the users, this will help the service providers.
Disclosure statement
No potential conflict of interest was reported by the author(s).
Data availability statement
Data sharing does not apply to this article as no new data has been created or analyzed in this study.
References
- Chandraraju, T. S., and A. Jeyaprakash. 2022. Categorization of breast masses based on deep belief network parameters optimized using chaotic krill herd optimization algorithm for frequent diagnosis of breast abnormalities. International Journal of Imaging Systems and Technology 32 (5):1561–24. doi: 10.1002/ima.22718.
- Chang, D., F. Li, J. Xue, and L. Zhang. 2023. A TRIZ-inspired knowledge-driven approach for user-centric smart product-service system: A case study on intelligent test tube rack design. Advanced Engineering Informatics 56:101901. doi: 10.1016/j.aei.2023.101901.
- Chen, T., L. U. H. Ding-Bang, and X. S. Li. 2023. Building product family platform and product service system based on extension innovation method.
- Chiu, M. C., J. H. Huang, S. Gupta, and G. Akman. 2021. Developing a personalized recommendation system in a smart product service system based on unsupervised learning model. Computers in Industry 128:103421. doi: 10.1016/j.compind.2021.103421.
- Cong, J., C. H. Chen, X. Meng, Z. Xiang, and L. Dong. 2023. Conceptual design of a user-centric smart product-service system using self-organizing map. Advanced Engineering Informatics 55:101857. doi: 10.1016/j.aei.2022.101857.
- Cong, J., P. Zheng, Y. Bian, C. H. Chen, J. Li, and X. Li. 2022. A machine learning-based iterative design approach to automate user satisfaction degree prediction in smart product-service system. Computers & Industrial Engineering 165:107939. doi: 10.1016/j.cie.2022.107939.
- Dehghani, M., Š. Hubálovský, and P. Trojovský. 2021. Northern goshawk optimization: A new swarm-based algorithm for solving optimization problems. Ieee Access 9:162059–80. doi: 10.1109/ACCESS.2021.3133286.
- Dong, L., M. Ren, Z. Xiang, P. Zheng, J. Cong, and C. H. Chen. 2023. A novel smart product-service system configuration method for mass personalization based on knowledge graph. Journal of Cleaner Production 382:135270. doi: 10.1016/j.jclepro.2022.135270.
- Fargnoli, M., and N. Haber. 2023. A QFD‐based approach for the development of smart product‐service systems. Engineering Reports e12665. doi: 10.1002/eng2.12665.
- Haber, N., and M. Fargnoli. 2021. Sustainable product-service systems customization: A case study research in the medical equipment sector. Sustainability 13 (12):6624. https://www.kaggle.com/datasets/a24998667/zhwiki-cleaned.
- Iriarte, I., M. Hoveskog, H. N. Ngoc, I. Legarda, M. Uranga, M. Nazabal, and A. Atxa. 2023. Service design for digital servitization: Facilitating manufacturers’ advanced services value proposition design in the context of industry 4.0. Industrial Marketing Management 110:96–116. doi: 10.1016/j.indmarman.2023.02.015.
- Kim, M., C. Lim, and J. Hsuan. 2023. From technology enablers to circular economy: Data-driven understanding of the overview of servitization and product–service systems in industry 4.0. Computers in Industry 148:103908. doi: 10.1016/j.compind.2023.103908.
- Kliestik, T., E. Kovalova, and G. Lăzăroiu. 2022. Cognitive decision-making algorithms in data-driven retail intelligence: Consumer sentiments, choices, and shopping behaviors. Journal of Self-Governance and Management Economics 10 (1):30–42.
- Li, Y., T. Wang, S. Chen, and X. Zhang. 2021. Application and development of natural language processing service in intelligent customer service system. In 3D Imaging Technologies—Multidimensional Signal Processing and Deep Learning, 157–62. Singapore: Springer.
- Li, X., Z. Wang, C. H. Chen, and P. Zheng. 2021. A data-driven reversible framework for achieving sustainable smart product-service systems. Journal of Cleaner Production 279:123618. doi: 10.1016/j.jclepro.2020.123618.
- Ngai, E. W., M. C. Lee, M. Luo, P. S. Chan, and T. Liang. 2021. An intelligent knowledge-based chatbot for customer service. Electronic Commerce Research and Applications 50:101098. doi: 10.1016/j.elerap.2021.101098.
- Nie, J., Q. Wang, and J. Xiong. 2021. Research on intelligent service of customer service system. Cognitive Computation and Systems 3 (3):197–205. doi: 10.1049/ccs2.12012.
- Ren, M., L. Dong, Z. Xia, J. Cong, and P. Zheng. 2023. A proactive interaction design method for personalized user context prediction in smart-product service system. Procedia CIRP 119:963–968. doi: 10.1016/j.procir.2023.01.021.
- Sakao, T., and A. Neramballi. 2020. A product/service system design schema: Application to big data analytics. Sustainability 12 (8):3484. doi: 10.3390/su12083484.
- Sharma, A. K., B. Bajpai, R. Adhvaryu, S. D. Pankajkumar, P. P. Gordhanbhai, and A. Kumar. 2023. An efficient approach of product recommendation system using NLP technique. Materials Today: Proceedings 80:3730–43. doi: 10.1016/j.matpr.2021.07.371.
- Tan, Y., H. Xu, Y. Wu, Z. Zhang, Y. An, Y. Xiong, and F. Wang. 2021. Research on knowledge driven intelligent question answering system for electric power customer service. Procedia Computer Science 187:347–352. doi: 10.1016/j.procs.2021.04.072.
- Tseng, M. L., T. D. Bui, S. Lan, M. K. Lim, and A. H. M. Mashud. 2021. Smart product service system hierarchical model in banking industry under uncertainties. International Journal of Production Economics 240:108244. doi: 10.1016/j.ijpe.2021.108244.
- Watanabe, K., T. Okuma, and T. Takenaka. 2020. Evolutionary design framework for smart PSS: Service engineering approach. Advanced Engineering Informatics 45:101119. doi: 10.1016/j.aei.2020.101119.
- Wei, D., and M. Zhou. 2021. E-commerce online intelligent customer service system based on fuzzy control. Journal of Sensors 2021:1–11. doi: 10.1155/2021/4867222.
- William, P., G. R. Lanke, V. N. R. Inukollu, P. Singh, A. Shrivastava, and R. Kumar 2023, May. Framework for design and implementation of chat support system using natural language processing. 2023 4th International Conference on Intelligent Engineering and Management (ICIEM), 1–7. London, United Kingdom: IEEE.
- Xie, J., S. Li, and X. Wang. 2022. A digital smart product service system and a case study of the mining industry: MSPSS. Advanced Engineering Informatics 53:101694. doi: 10.1016/j.aei.2022.101694.
- Yuan, W., D. Chang, and T. Han. 2023. A context-aware smart product-service system development approach and application case. Computers & Industrial Engineering 183:109468. doi: 10.1016/j.cie.2023.109468.
- Zhang, X., L. Chen, G. Sheng, X. Lu, and X. Ming. 2023. An innovation service system and personalized recommendation for customer-product interaction life cycle in smart product service system. Journal of Cleaner Production 398:136470. doi: 10.1016/j.jclepro.2023.136470.
- Zhang, K., K. Y. Lin, J. Wang, Y. Ma, H. Li, L. Zhang, K. Liu, and L. Feng. 2023. UNISON framework for user requirement elicitation and classification of smart product-service system. Advanced Engineering Informatics 57:101996. doi: 10.1016/j.aei.2023.101996.
- Zhang, X., and X. Ming. 2022a. Comprehensive understanding of smart product service system from multi-dimension and multi-perspective: An innovative service model for customer-product interaction life cycle (CILC). Advanced Engineering Informatics 52:101619. doi: 10.1016/j.aei.2022.101619.
- Zhang, X., and X. Ming. 2022b. Implementation path and reference framework for Industrial Internet Platform (IIP) in product service system using industrial practice investigation method. Advanced Engineering Informatics 51:101481. doi: 10.1016/j.aei.2021.101481.
- Zhang, X., X. Ming, Y. Bao, and X. Liao. 2022. Industrial internet platform (IIP) enabled smart product lifecycle-service system (SPLSS) for manufacturing model transformation: From an industrial practice survey. Advanced Engineering Informatics 52:101633. doi: 10.1016/j.aei.2022.101633.
- Zhou, T., Z. Chen, Y. Cao, R. Miao, and X. Ming. 2022. An integrated framework of user experience-oriented smart service requirement analysis for smart product service system development. Advanced Engineering Informatics 51:101458. doi: 10.1016/j.aei.2021.101458.