ABSTRACT
The complexity of ecosystem-based management (EBM) of natural resources has given rise to research frameworks such as integrated ecosystem assessments (IEA) that pull together large amounts of diverse information from physical, ecological, and social domains. Conceptual models are valuable tools for assimilating and simplifying this information to convey our understanding of ecosystem structure and functioning. Qualitative network models (QNMs) may allow us to conduct dynamic simulations of conceptual models to explore natural–social relationships, compare management strategies, and identify tradeoffs. We used previously developed QNM methods to perform simulations based on conceptual models of the California Current ecosystem's pelagic communities and related human activities and values. Assumptions about community structure and trophic interactions influenced the outcomes of the QNMs. In simulations where we applied unfavorable environmental conditions for production of salmon (Oncorhynchus spp.), intensive management actions only modestly mitigated declines experienced by salmon, but strongly constrained human activities. Moreover, the management actions had little effect on a human wellbeing attribute, sense of place. Sense of place was most strongly affected by a relatively small subset of all possible pair-wise interactions, although the relative influence of individual pair-wise interactions on sense of place grew more uniform as management actions were added, making it more difficult to trace effective management actions via specific mechanistic pathways. Future work will explore the importance of changing conceptual models and QNMs to represent management questions at finer spatial and temporal scales, and also examine finer representation of key ecological and social components.
Introduction
As ecosystem approaches to resource management take shape within real-world systems, scientists and managers are engaging in more frequent and informative communication about goals, strategies, constraints, and tradeoffs related to resource use. Through this discourse, scientists are coming to more fully realize the breadth and interplay of issues and needs that confront stakeholders and decision makers. Many aspects of applied ecosystem science, even in their most advanced state, focus primarily on a single resource sector (e.g., fisheries, forests, grasslands), even as we acknowledge that resources do not exist in “silos,” but rather overlap at many scales, have linked ecologies, and are valued by multiple stakeholder groups (Norse Citation2010). True ecosystem approaches for addressing these complex relationships and management goals require integrated analyses from physical, chemical, biological, ecological, economic, social, and political perspectives spanning multiple resource sectors (Ciannelli et al. Citation2014).
To help address this challenge, scientists have developed research frameworks that bring together theories and findings from multiple disciplines to support management objectives. One example is the integrated ecosystem assessment (IEA) approach, adopted by the US National Oceanic and Atmospheric Administration (NOAA) in support of ecosystem-based management (EBM) of marine resources (Levin et al. Citation2009; Samhouri et al. Citation2014). The IEA approach can be viewed as an iterative loop () with five steps: (1) defining the ecosystem goals and objectives of managers and stakeholders; (2) developing indicators that function as proxies for the ecosystem attributes that are the focus of management objectives; (3) assessing the status and trends of those indicators; (4) analyzing the risks posed to the ecosystem by natural and anthropogenic stressors; and (5) evaluating alternative management strategies to determine which ones are most likely to achieve management goals, and what the potential tradeoffs will be (Levin et al. Citation2009; Samhouri et al. Citation2014). Outcomes from step (5) should inform subsequent iterations of the IEA loop, as preferred management actions are applied and ecosystem responses are monitored.
Figure 1. The integrated ecosystem assessment (IEA) loop, outlining the general steps that an IEA follows in the course of iterative, adaptive management to meet the ecosystem-based management (EBM) goals defined at the outset. Source: adapted from NOAA IEA Program.
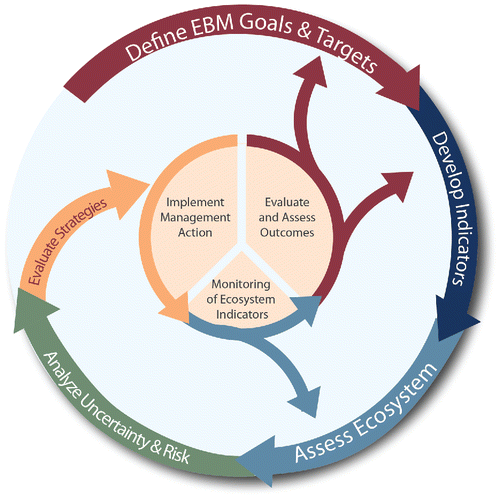
The IEA process hinges on effective communication and information transfer between scientists, managers, and stakeholders, especially early in the process when goals and objectives are defined and key ecosystem attributes and processes are identified and prioritized (Fletcher et al. Citation2014; Levin et al. Citation2009; Orians et al. Citation2012). To facilitate effective communication, the California Current IEA (CCIEA) team, which focuses on the California Current marine ecosystem along the West Coast of the USA (http://www.noaa.gov/iea/regions/california-current-region/), developed conceptual models that illustrate our understanding of the connections between key species, environmental drivers, habitats, and human systems. For example, shows the conceptual model centered on salmon (Oncorhynchus spp.). These simple yet elegant diagrams provide a general context within which we can frame and discuss more complex analyses, such as time series analyses (Andrews et al. Citation2015), risk assessment (Samhouri and Levin Citation2012), and ecosystem modeling (Kaplan, Gray, and Levin Citation2013b; Kaplan and Leonard Citation2012).
Figure 2. Nested conceptual model of the California Current ecosystem, centered on salmon; overview (top left) and relationships to key environmental drivers (top right); key ecological interactions (bottom left); and key human activities (bottom right).
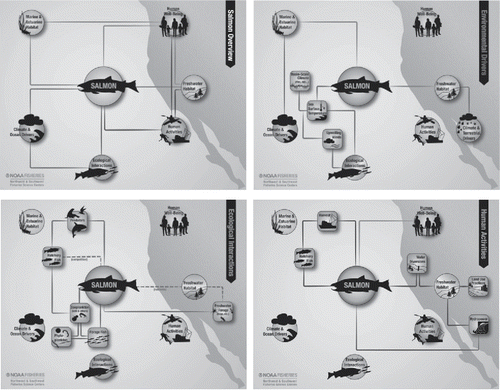
As members of the CCIEA team began incorporating these models into communication with different partners, we came to more clearly realize two of their other potential benefits, which would dramatically increase their value above just being effective communication tools. First, we realized that the conceptual models could be reasonable organizing frameworks for our thinking within the CCIEA project: they need not merely be coarse simplifications of the system, but rather should be “maps” of the indicators derived from the IEA indicator screening process (Harvey et al. Citation2014; Levin and Schwing Citation2011). In other words, each symbol or line in the model should have one or more indicators associated with it, in order to convey the context and importance of those indicators to scientists, managers, and stakeholders alike.
Our second, parallel realization was that the conceptual models were reminiscent of box-and-arrow plots that are often used to describe food webs and complement quantitative food web models (Field, Francis, and Strom Citation2001; Gaichas, Aydin, and Francis Citation2010; Smith et al. Citation2015). Our conceptual models include diverse processes, human activities, societal constructs, and units of measure that would not be easy to incorporate into a single quantitative framework. However, qualitative network models (QNMs; Melbourne-Thomas et al. Citation2012), which link system components by the signs of the relationships between them (−, 0, +), could provide a means to operationalize conceptual models. QNMs are well-suited to data-poor systems, such as poorly studied food webs (Melbourne-Thomas et al. Citation2012; Reum et al. Citation2015), and to complex socioecological networks and governance systems (Metcalf et al. Citation2014). Thus they could be applied to our conceptual models, where we are unsure of the precise quantitative relationships that link many components. Converting conceptual models into QNMs should allow us to test our assumptions about conceptual model structure, link multiple models together, and run simple scenarios to examine relationships across different ecosystem components.
Our goal is to evaluate CCIEA conceptual models as the basis for modeling ecological and social dynamics in the California Current ecosystem. To do so, we develop a QNM based on conceptual models of the pelagic portion of the ecosystem. We examine different configurations of the QNM to compare model behavior, relative to expectations derived from our understanding of how indicators are correlated with and/or respond to one another. Finally, we conduct some simple perturbations of the system to see how different components respond directly and indirectly, and if there are obvious tradeoffs across human activity sectors.
Methods
Development of conceptual models
The CCIEA team is made up of ∼50 scientists from multiple West Coast institutions; most team members are in the fields of oceanography and climate science, biology, ecology, fisheries, economics, sociology, and cultural anthropology (Levin, Wells, and Sheer Citation2013). Since 2010, the team has been developing comprehensive lists of ecosystem indicators related to the status and trends of species groups, habitats, human activities and wellbeing, and stressors (Harvey et al. Citation2014; Levin and Schwing Citation2011; Levin et al. Citation2013). All indicators were rigorously screened with methods developed by Kershner et al. (Citation2011). The team developed conceptual models in parallel, in order to ensure key ecosystem attributes, components, and processes were represented by the indicator sets (Orians et al. Citation2012). Conceptual models were built by consensus over the course of multiple workshops and follow-up discussions. The conceptual models centered around key species groups (coastal pelagic species (CPS), salmon, groundfishes, seabirds, and marine mammals), with humans present in each model. Each model is nested: an overview model depicts general ecosystem relationships, and sub-models provide greater detail on the species' relationship to other ecosystem components ().
In the present study, we chose three conceptual models, which focus on species that co-occur in pelagic waters of the California Current ecosystem: salmon (specifically Chinook salmon Oncorhynchus tshawytscha and coho salmon O. kisutch), CPS, and marine mammals (, S1, and S2). We simplified the three models further and merged them into a single QNM (; ) to determine if changes centered within one conceptual model could extend to other conceptual models, and thus provide an assessment of direct and indirect connectivity between the models and the species, processes, and human systems that they represent.
Figure 3. Combined conceptual models (salmon, coastal pelagic species (CPS) and forage fish, marine mammals, and human systems) used for qualitative network models (QNMs). FW, freshwater. Lines indicate links between components; an arrow indicates a positive effect (+) on the terminal group, while a dot indicates a negative (−) effect on the terminal group. A link with no arrow or dot is neutral (0) for the terminal group. The configuration of this QNM is for the “mixed control” system outlined in Scenario 1.
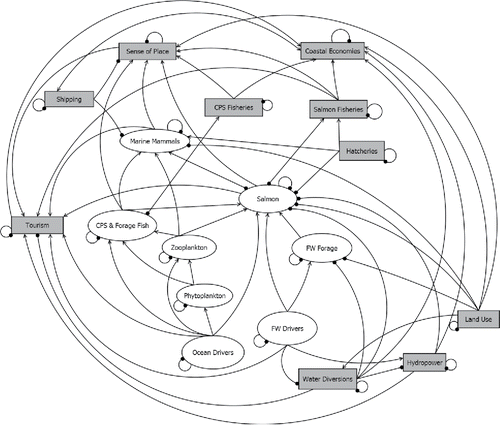
Table 1. Basic description of each node in the qualitative network models (QNMs).
Qualitative network models
Our use of QNMs generally follows the methods of Melbourne-Thomas et al. (Citation2012), who used a probabilistic approach for estimating uncertainty in the signs of responses of system components following perturbations. Our approach most closely follows the Melbourne-Thomas et al. (Citation2012) framework as outlined by Reum et al. (Citation2015). The conceptual models described above were reconfigured as a signed directed graph (i.e., a signed digraph; ), in which system nodes (described in ) are linked by lines that represent the signs of direct effects (positive, negative, or neutral) of the components on one another (Puccia and Levins Citation1985). Because they are mathematical equivalents, a signed digraph is analyzed in the same manner as a community interaction matrix, wherein the rate of change of an ecosystem component is a continuous function of one or more other components in the system (Levins Citation1974; Puccia and Levins Citation1985). The dynamics of n interacting components (environmental drivers, species, human activity sectors, etc.) can be described as a series of differential equations:where the rate of change in variable xi is a function fi of the other n − 1 variables and their rate parameters c (Raymond et al. Citation2011). Pair-wise interaction coefficients aij describe the effect of a small change in the level of component j on the level of component i, as defined by the partial derivative
evaluated at the equilibrium (Melbourne-Thomas et al. Citation2012; Raymond et al. Citation2011). The community matrix A is made up of elements aij, and the negative inverse of the community matrix (−A−1) yields estimated changes in the equilibrium abundances of each component x as a function of a sustained (press) perturbation of one or more rate parameters c (Puccia and Levins Citation1985; Raymond et al. Citation2011).
In practice, quantitative estimates of aij are often difficult to obtain and quantitative predictions from the community matrix can be highly sensitive to small errors in aij (Dambacher, Li, and Rossignol Citation2002). As an alternative, the models can be analyzed qualitatively. In the QNM approach of Melbourne-Thomas et al. (Citation2012), the community matrix A is generated by first specifying the signs of pair-wise links (positive, negative, or zero), and then by randomly drawing the aij values for each non-zero link from specified distributions. The predicted quantitative response of the community to a press perturbation is converted to a qualitative sign response, which is then summarized over many simulations to generate probabilities of sign outcomes (Melbourne-Thomas et al. Citation2012; Raymond et al. Citation2011).
For each scenario outlined below, we used the following simulation protocol: (1) the signs of the elements aij in A were derived from the interactions illustrated in the conceptual model; (2) the absolute values of each aij were randomly drawn from a uniform distribution that spanned two orders of magnitude (0.01–1), except for self-interaction coefficients, which were drawn from a uniform distribution spanning −0.25 to −1 (Raymond et al. Citation2011); and (3) the resulting matrix A was evaluated against system stability criteria (Melbourne-Thomas et al. Citation2012). If A was stable, the sign responses of each node to the given system perturbation were calculated. We repeated this procedure until we had 10,000 stable model structures for a given analysis, from which we summarized each node's distribution of responses (positive or negative) to the perturbation. Following the approach of Raymond et al. (Citation2011), we assumed a “sign consistency” threshold of 70%, that is, we would only conclude that a node's response was consistently positive if it was positive ≥70% of the time (or consistently negative when negative ≥70% of the time).
QNM scenarios of California Current pelagic system
All scenarios were run with the R language (R Core Team Citation2013), using the simulation.r, tree.r, dia.r, and community.r code adapted from Melbourne-Thomas et al. (Citation2012). The conceptual models for QNM analysis () were designed in the open source diagramming software Dia (ver. 0.97.2; http://dia-installer.de).
The first scenario was a test of the role of trophic functioning in shaping model outputs. We compared outcomes from four alternative, hypothetical food web configurations: (1) a system in which all food web interactions were “donor controlled,” that is, a trophic link had a positive direct effect on the predator but no direct effect on the prey; (2) a “joint controlled” system in which all trophic interactions had a direct positive effect on the predator and a direct negative effect on the prey; (3) a “wasp-waist” system in which most trophic interactions were donor controlled, but the intermediate trophic levels (CPS and zooplankton) also exerted top-down control on their prey (Griffiths, Olson, and Watters Citation2013); and (4) a “mixed control” system in which designations of direct trophic control were based on the assumption that the pelagic food web of the California Current is mostly a bottom-up (donor-controlled) system (Ware and Thomson Citation2005), but that marine mammals could also exert top-down predation control on salmon, as has been observed for pinnipeds (Keefer et al. Citation2012; Moore, Berejikian, and Tezak Citation2013; Wright et al. Citation2007). This system is illustrated in . The signs (−, 0, +) for all configurations are shown in Table S1. In the model, we included nodes to correspond to climate and environmental conditions, which generally correlate with system productivity (, ): “ocean drivers” include factors like coastal upwelling, nutrients, and upper water column temperatures, while “freshwater drivers” include snowpack, stream flow, and stream temperatures. For each simulation in this scenario, we imposed a “productive” climate scenario for salmon, that is, positive press perturbations to freshwater drivers (≈ high snowpack, high spring/summer stream flow, cool temperatures) and ocean drivers (strong upwelling, high nutrient availability, cool temperatures) (Crozier and Zabel Citation2006; Peterson et al. Citation2014).
In the second scenario, we imposed management actions in order to mitigate the effects of poor environmental conditions on salmon populations. We ran four simulations: in the first, we imposed negative environmental conditions (i.e., negative press perturbations on freshwater and ocean drivers, ). The next three simulations featured different management actions to determine which was most effective in countering the negative environmental impacts: we reduced fishing mortality on salmon and their prey (i.e., negative perturbations to salmon fisheries and CPS fisheries); we reduced fishing mortality and also reduced adverse impacts of other human activities on salmon and their habitats (negative perturbations on hatcheries, hydropower, water diversions, and land use); and we reduced all of the above activities and also decreased biomass of marine mammals (negative perturbation on marine mammals), analogous to actions used to reduce pinniped predation on protected salmon stocks (Keefer et al. Citation2012). We applied these actions to the “mixed control” food web from Scenario 1.
In the third scenario, we examined the effects of the simulations in Scenario 2 on “sense of place,” a human wellbeing attribute related to the connections and identity that people derive from a place's physical setting, environmental features, and cultural, societal and psychological activities, qualities and values (Larson, De Freitas, and Hicks Citation2013). This social attribute is more difficult to track or model in a quantitative sense than, say, economic attributes. To determine which conceptual model components most affect sense of place, we used a boosted regression tree (BRT) method (Elith, Leathwick, and Hastie Citation2008; Melbourne-Thomas et al. Citation2012) to estimate and rank the relative effects of each pair-wise link on the sign response of sense of place. We used the tree.r code of Melbourne-Thomas et al. (Citation2012) for this analysis. Within the code, we fixed the learning rate at 0.001, which always resulted in final BRT models with well over 1,000 trees, as recommended by Elith et al. (Citation2008). We examined the influence of different links on sense of place using the four management simulations from Scenario 2. Because initial simulations showed evidence of a food web configuration effect on the influence of pair-wise links, we included BRT analysis for both the “mixed control” and “wasp-waist” system configurations.
Results
In Scenario 1, the configuration of the food web had a strong effect on the response of the QNM to the perturbation. In the donor-controlled food web, forcing the freshwater and ocean drivers to a productive state caused most ecosystem components to respond positively in the clear majority of simulations (). Most components had positive responses with high sign consistency (positive between 89% and 100% of the time). Only water diversions responded negatively with high consistency (i.e., 100% of the time). Marine mammals and sense of place responded negatively with low sign consistency (negative 55–60% of the time); the failure of these nodes to respond strongly positively appears largely due to the influence of increased shipping. Hatcheries and land use showed no response because they were linked neither directly nor indirectly to the increases in production (). In the joint-controlled food web (), responses to productive conditions were more complex: CPS and forage fish, salmon, both types of fisheries, hydropower, tourism, shipping, and coastal economies responded positively with sign consistencies >70%, while water diversions and marine mammals exhibited clear negative responses (sign consistency 100% and 73%, respectively). Other responses were ambiguous, falling below 70% sign consistency. The wasp-waist configuration () yielded qualitatively similar results to the joint-controlled configuration, although CPS and forage fish, CPS fisheries, and freshwater forage were more strongly positive in the wasp-waist configuration (>96% sign consistency) because they were not controlled by predators, as they were in the joint-controlled configuration (). The mixed control configuration () was qualitatively similar to the donor-controlled configuration ().
Figure 4. Percent of positive versus negative responses of ecosystem components to positive perturbations in freshwater and ocean drivers in the QNM of the California Current pelagic system (n = 10,000 iterations per QNM run). Light shading, negative response; dark shading, positive response; open, no response. Panels represent different configurations of food web control in the system: (A) donor control; (B) joint control; (C) wasp-waist control; and (D) mixed control.
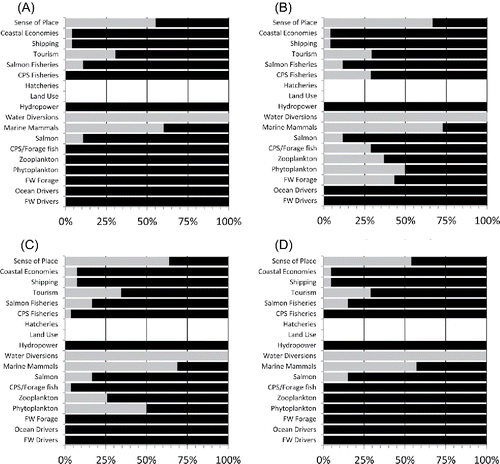
In Scenario 2, model outcomes indicated that under negative environmental conditions in the mixed control configuration, mitigating declines in salmon through management actions would be very difficult. The baseline simulation, with negative forcing to freshwater and ocean drivers, caused most nodes to decline in a majority of model runs, with sign consistency >70% (). Reducing fishing on salmon and CPS had little effect on the system (), perhaps because both fisheries had already been heavily constrained by the negative environmental forcing (). Salmon sign consistency was even greater under this scenario (93%) than in the baseline (85%), likely because of the increase in marine mammals fueled by the small improvement in CPS. Implementing further restrictions on hatcheries, water diversions, land use, and hydropower () improved conditions for freshwater forage, such that they responded positively with 72% sign consistency, but that effect only modestly mitigated the environmental effects on salmon, which responded negatively with low sign consistency (67%). Adding marine mammal control () resulted in the salmon response being even more indeterminate (negative with 57% consistency).
Figure 5. Percent of positive versus negative responses of ecosystem components to four simulations run in the QNM of the California Current pelagic system (n = 10,000 iterations per QNM run). Light shading, negative response; dark shading, positive response; open, no response. Panels represent the four simulations: (A) “environment” (poor freshwater and ocean environmental conditions); (B) “environment + fishing” (same as (A) plus fisheries reductions); (C) “environment + fishing + habitat” (same as (B) plus reductions in land use, water diversions, hydropower, and hatcheries); and (D) “environment + fishing + habitat + mammals” (same as (C) plus reductions in marine mammals).
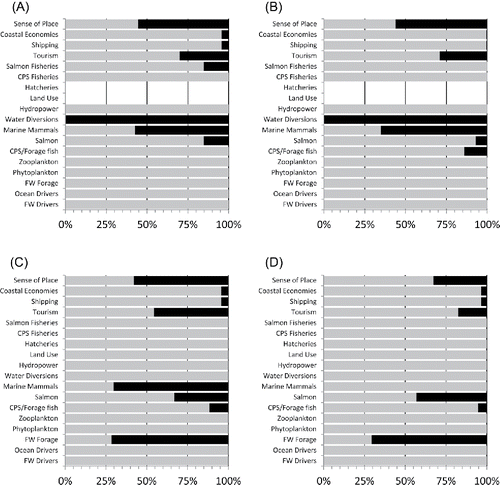
In Scenario 3, the relative influence of different model links on sense of place was dependent upon the number of perturbations included, and also on food web configuration (mixed control vs. wasp-waist control). In all cases, sense of place had low sign consistency (33–67%) across all perturbations and system configurations (to emphasize that, depicts one example out of the four model configuration options). In the mixed control model (n = 75 links), the sign response of sense of place was primarily attributed to the interaction strengths of a small subsets of links in the simulation with poor environmental conditions (). As management controls were added (reducing fishing, protecting habitat, controlling marine mammals), the relative influence of individual links declined, but only slightly. The ten most influential links on sense of place in the baseline “environment” simulation were generally related to coastal economies, shipping, hydropower, and tourism (). The coastal economies–shipping link was the most influential link on sense of place, but its influence dropped slightly as management actions were implemented. In general, the most influential links on sense of place were indirect, although the direct link between shipping and sense of place was relatively influential (). Also, most of the influential links were links between two social elements rather than links involving natural ecosystem components.
Figure 6. Relative influence of pair-wise interactions (links) between model nodes on sense of place, as driven by four environmental/management simulations (simulations as shown in ). Links are ranked from most to least influential. Relative influence is unitless and sums to 100 for a given simulation. (A) Relative influences for each link in the mixed control system. (B) Changes in relative influence for the 10 highest-ranked links from the “Environment” simulation of the mixed control simulation. (C) Same as (A), but for the wasp-waist controlled system. (D) Same as (B), but for the wasp-waist controlled system.
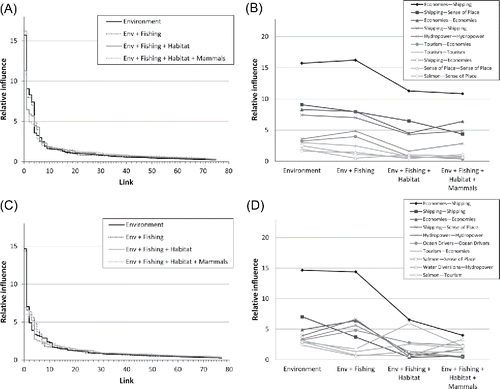
The wasp-waist system (n = 77 links) had some important differences from the mixed control system. Once again, a relatively small number of links had disproportionate influence on sense of place, and the relative influence of links became more evenly distributed as progressively more management actions were added to the simulations (). Once again, the coastal economies–shipping link was the most influential link on sense of place in the “environment” simulation, that is, poor environmental conditions but no management intervention (). However, as management actions were progressively added, the influence of the coastal economies–shipping link dropped sharply and eventually reached levels that were comparable to many other links. Again, most of the influential links involved social nodes (economies, shipping, hydropower, tourism), but natural components (ocean drivers and salmon) were more common in the wasp-waist system than in the mixed control system ().
Discussion
Simulating ecosystems using QNMs and conceptual models
This study presents a means by which conceptual models can evolve from their already great value—for illustrating, organizing, and communicating general knowledge—to the even greater value of a simulation modeling framework. Enabling researchers, managers, and stakeholders with the ability to run simulations with a QNM derived from a conceptual model allows rapid testing of the assumptions that the model embodies and the signs of the direct and indirect relationships that it contains. There is further power in that conceptual models and QNMs can be easily updated to reflect changing conditions, new information, or alternative hypotheses about system structure. The speed and adaptability of QNMs, along with the intuitive nature and visual appeal of conceptual models, makes this approach suitable to “gaming” workshops in which scientists, decision-makers, and stakeholders collaborate to explore various approaches to management under different environmental conditions (Dambacher, Rothlisberg, and Loneragan Citation2015; Metcalf et al. Citation2014). Furthermore, the qualitative nature of the analysis makes it amenable for use in data-poor systems, or in cases where the functional forms of complex relationships, for example, between natural components and human components, are not as well defined as the signs (positive, negative, or neutral) of those relationships (Dambacher et al. Citation2009; Levins Citation1998). Ideally, this approach could be used in IEAs or other ecosystem management frameworks to complement quantitative, mechanistic methods that may provide more detailed information and complete the IEA loop (), but that can also take longer to fully develop (Reum et al. Citation2015).
Another strength of this approach is the ability to link ecological and social components under a common framework; such relationships can be abstract and difficult to quantify or data-poor at this point of their study (Metcalf et al. Citation2014). Here, we demonstrated the inclusion of human wellbeing, represented in our QNM by sense of place. Sense of place is a multifaceted, subjective, and often qualitative attribute (Larson et al. Citation2013), and individual facets of sense of place (e.g., personal or community-level values for place heritage, place aesthetics, spiritual qualities, biogeographic characteristics; see Poe, Donatuto, and Satterfield Citation2016) mean different things to different stakeholder groups. Diverse ecosystem components may interact with these facets independently of the others. Given these complexities, QNMs may be a suitable tool for exploring dynamic connections between sense of place and other ecosystem components. Moreover, the ability to explore relationships between human wellbeing attributes and other components of an ecosystem is particularly important given emerging mandates to consider human wellbeing as a part of natural resource management. For example, the U.S. Magnuson-Stevens Fishery Conservation and Management Act includes several National Standards (NS) that require federal fisheries managers to consider aspects of human wellbeing as essential aspects of fisheries management decisions, including equitable resource allocation (NS4); efficiency of harvesting, processing, marketing, and other uses of fishery resources (NS5); operational contingencies specific to different fisheries (NS6); avoiding unnecessary regulation and management costs (NS7); preserving long-term sustainability of fishery participation and economic conditions in coastal communities (NS8); and promoting safety at sea (NS10) (http://www.fisheries.noaa.gov/sfa/laws_policies/national_standards/index.html).
Interpreting California Current QNM results
Our first scenario clearly demonstrated the influence of QNM structure on the sign response of system variables. The four food web configurations that we explored resulted in two groups of model outputs. In one group, represented by the donor control and mixed control models, model components responded fairly uniformly to changes in environmental drivers: improving conditions for productivity in the freshwater and ocean systems led to increases in most biological and social components. In the other group of outputs, represented by the joint control and wasp-waist models, the responses to improved environmental conditions were more nuanced due to the suppressing effect of predators on prey populations. In this example, the different model configurations were based on aspects of food web theory, for which a broad literature exists. However, other aspects of socioecological system structure, such as the signs of links between social nodes or between social nodes and natural nodes, may be far more difficult to derive or divine from literature or consensus. The QNM approach can provide a rapid screening tool for testing the sensitivities of model structures to different assumptions (e.g., of trophic configuration) and elucidating particularly sensitive linkages or sources of structural uncertainty upon which future research should be focused (Melbourne-Thomas et al. Citation2012).
The second scenario illustrated how the QNM approach can be used to run qualitative scenarios that compare different management actions related to ecosystem goals. In this case, the management actions were intended to mitigate declines in salmon caused by poor environmental conditions. Given the structure of our QNM, the most stringent management regime produced only modest mitigation of the salmon decline (i.e., salmon moved from high, negative determinacy to low, negative determinacy). We should emphasize that these simulations only imply that salmon biomass “decreased” in most of the stable model structures; we can draw no further quantitative or qualitative conclusions about the extent of the decreases. Similarly, the status of other components can only be said to be likely to increase, likely to decrease, or uncertain. Thus, while QNM scenarios can illustrate some likely tradeoffs (e.g., exerting restrictions during poor environmental regimes may only yield minor salmon mitigation, but will almost certainly decrease many human activities), further analyses would be required to fully understand the nature and extent of those tradeoffs.
The third scenario illustrated the capacity of QNM methods to connect specific elements of a model (e.g., links between nodes) to desired outcomes (e.g., a strong sense of place). For example, we saw that sense of place in this system was more strongly connected to social components than to natural components, and that indirect connections were more influential on sense of place than were direct links from other components to sense of place. However, we also found that as more and more management actions were added to simulations, the distribution of relative influences flattened—particularly with the wasp-waist food web configuration—such that a steadily larger number of links had similar levels of influence on sense of place. That may imply that when complex combinations of management strategies are applied across multiple resource sectors and societal objectives, it becomes much more difficult for a QNM to identify which management strategies are most likely to achieve a particular goal. This result is likely sensitive to the complexity of the underlying conceptual model, and future applications of this approach in the CCIEA framework will require careful sensitivity analysis of model structure to ensure QNM outputs are being interpreted reasonably.
Next steps
There are many directions in which to move forward with applying QNMs to conceptual models. For example, we have yet to develop a conceptual model for seabirds, which play multiple roles as predators and scavengers in the pelagic region of the California Current ecosystem. Similarly, lumping all marine mammals into a single group likely oversimplifies their tremendous functional diversity; groups such as pinnipeds, baleen whales, and toothed whales have very different ecosystem roles and human interactions. In the same manner, simulating sense of place as one monolithic node belies its complexity and the distinct ways that factors like individual biography, community heritage, place aesthetics, and spirituality affect human behavior and decision-making. It also belies the fact that in an ecosystem with so many uses, any ecosystem component could be viewed as a positive, negative, or neutral aspect of sense of place, depending on the perspective of the stakeholder (Poe et al. Citation2016).
Another consideration is that conceptual models and QNMs are site- and scale-dependent: key components and linkages very likely differ as we move from a coast-wide scale to a regional or local scale. For example, it is reasonable to expect that a conceptual model designed to represent a region of the coast with a very small human population would have very different components and links from a model designed to represent waters near a major port city, or a model designed to represent the entire coast. A model in a region with a large pinniped haul-out area would have different linkages from one where pinnipeds were scarce. Additionally, our models do not account for strongly nonlinear dynamics that involve thresholds or produce transitions in system state (Reum et al. Citation2015), which could be caused by strong perturbations at some spatiotemporal scales; addressing such dynamics would likely require building separate models for each alternate system state. The models presented here are founded at a very general level at a broad spatial scale, and would require refinement in order to be scaled appropriately for specific questions or applications.
Fortunately for all of the above caveats, conceptual models and QNMs are very easy to reconfigure if necessary. However, investigators should carefully consider the complexity of their models as they make configuration decisions. It is certainly possible to build and analyze highly complex QNMs, but increasing complexity can eventually challenge computing power. Moreover, model complexity affects model dynamics, possibly in ways that unrealistically bias QNM outputs. Finally, model complexity may interact with scenario complexity to render model outputs very difficult to interpret, as we experienced with the most complex management simulations and their effects on sense of place. Exploring the sensitivity of QNMs to model structural complexity will be an essential exercise in judging QNM usefulness and reliability (Link et al. Citation2012).
As with any model, the QNM conceptual model should be validated, ideally against empirical data or more mechanistic models, such as food web models for the California Current region (Field et al. Citation2001; Harvey and Kareiva Citation2005; Kaplan et al. Citation2013a). This validation would provide support for some model structures over others, and may provide greater confidence in QNM predictions about relatively data-poor components of the system. Some components or links of any conceptual model are more likely to have data than others, and these data should be used throughout QNM development, including when the model is being structured. For example, early iterations of our QNMs included hatchery salmon as interactive ecological components, rather than as an external driver as depicted in . Hatchery salmon experienced the same relationships with ecological and human components as “wild” salmon, and also had direct negative interactions with wild salmon. Under this model structure, wild salmon were insensitive to every scenario we imposed; increases and decreases were nearly equally likely following nearly every perturbation simulated. This was implausible because there is abundant evidence that juvenile and adult wild salmon respond strongly to many environmental changes (Beamish et al. Citation1999; Burke et al. Citation2013; Greene et al. Citation2005) and human activities (e.g., McClure et al. Citation2003; Paulsen and Fisher Citation2001; Schaller, Petrosky, and Langness Citation1999). While a full validation of our QNM against empirical data is beyond the scope of this paper, which merely attempts to present the essence of the approach, we will certainly test the robustness of QNM behavior relative to data as we operationalize conceptual models within the CCIEA. For instance, time series analysis may offer information on the sign response of one or more system variables to a given press perturbation. Such information will be used to screen the randomly drawn community interaction matrices to only those that reproduce the known behavior (Melbourne-Thomas et al. Citation2012; Raymond et al. Citation2011). Further, if system responses are partially known, the relative likelihood of multiple competing models can be compared under a Bayesian framework (Melbourne-Thomas et al. Citation2012).
Conclusions
The emphasis on EBM of natural resources continues to grow. Concurrently, ecosystem science as a field is focusing ever-greater attention upon social sciences that relate humans to ecosystems. In many cases, we lack data sets, monitoring programs, or quantitative models to keep pace with these developments or to properly measure their trends and explore their dynamics. Those research capacities will take time and resources to develop. In the meantime, conceptual models are important tools that can help natural scientists, social scientists, managers, and policymakers to build understanding and consensus around major ecosystem components, processes, states, and goals (Orians et al. Citation2012; Reum et al. Citation2015). QNMs are a means by which conceptual models can be further operationalized into a dynamic simulation framework that connects natural and social components (Metcalf et al. Citation2014). While much work remains to be done in understanding QNM behavior and sensitivity, QNMs hold great promise as a tool that can be used for qualitative, integrative ecosystem assessments, and a complement to quantitative tools as they evolve.
Supplementary_materials.zip
Download Zip (441.4 KB)Acknowledgments
This paper is dedicated to the memory of our friend and colleague Mark Plummer. We gratefully acknowledge the work of the entire CCIEA team in the painstaking task of developing the conceptual models, with particular thanks to Mindi Sheer, Brian Wells, Dawn Noren, Sharon Melin, and Phil Levin. Brian Wells, Ric Brodeur, Eric Bjorkstedt, Phil Levin, Leif Anderson, and two anonymous reviewers provided valuable comments and discussion that improved this paper considerably. All conceptual model images for the California Current IEA project are available from the authors.
Funding
Research was supported by funds from the NOAA Fisheries IEA Program.
References
- Andrews, K. S., G. D. Williams, J. F. Samhouri, K. N. Marshall, V. Gertseva, and P. S. Levin. 2015. The legacy of a crowded ocean: Indicators, status, and trends of anthropogenic pressures in the California Current ecosystem. Environmental Conservation 42 (2):139–151.
- Beamish, R. J., D. J. Noakes, G. A. McFarlane, L. Klyashtorin, V. V. Ivanov, and V. Kurashov. 1999. The regime concept and natural trends in the production of Pacific salmon. Canadian Journal of Fisheries and Aquatic Sciences 56 (3):516–526.
- Burke, B. J., W. T. Peterson, B. R. Beckman, C. Morgan, E. A. Daly, and M. Litz. 2013. Multivariate models of adult Pacific salmon returns. Plos One 8 (1):e54134.
- Ciannelli, L., M. Hunsicker, A. Beaudreau, K. Bailey, L. B. Crowder, C. Finley, C. Webb, J. Reynolds, K. Sagmiller, J. M. Anderies, D. Hawthorne, J. Parrish, S. Heppell, F. Conway, and P. Chigbu. 2014. Transdisciplinary graduate education in marine resource science and management. ICES Journal of Marine Science 71 (5):1047–1051.
- Crozier, L., and R. W. Zabel. 2006. Climate impacts at multiple scales: Evidence for differential population responses in juvenile Chinook salmon. Journal of Animal Ecology 75 (5):1100–1109.
- Dambacher, J. M., D. J. Gaughan, M. J. Rochet, P. A. Rossignol, and V. M. Trenkel. 2009. Qualitative modelling and indicators of exploited ecosystems. Fish and Fisheries 10 (3):305–322.
- Dambacher, J. M., H. W. Li, and P. A. Rossignol. 2002. Relevance of community structure in assessing indeterminacy of ecological predictions. Ecology 83 (5):1372–1385.
- Dambacher, J. M., P. C. Rothlisberg, and N. R. Loneragan. 2015. Qualitative mathematical models to support ecosystem-based management of Australia's Northern Prawn Fishery. Ecological Applications 25 (1):278–298.
- Elith, J., J. R. Leathwick, and T. Hastie. 2008. A working guide to boosted regression trees. Journal of Animal Ecology 77 (4):802–813.
- Field, J., R. Francis, and A. Strom. 2001. Toward a fisheries ecosystem plan for the Northern California Current. CalCOFI Reports 42:74–87.
- Fletcher, P. J., C. R. Kelble, W. K. Nuttle, and G. A. Kiker. 2014. Using the integrated ecosystem assessment framework to build consensus and transfer information to managers. Ecological Indicators 44:11–25.
- Gaichas, S. K., K. Y. Aydin, and R. C. Francis. 2010. Using food web model results to inform stock assessment estimates of mortality and production for ecosystem-based fisheries management. Canadian Journal of Fisheries and Aquatic Sciences 67 (9):1490–1506.
- Greene, C. M., D. W. Jensen, G. R. Pess, and E. A. Steel. 2005. Effects of environmental conditions during stream, estuary, and ocean residency on Chinook salmon return rates in the Skagit River, Washington. Transactions of the American Fisheries Society 134 (6):1562–1581.
- Griffiths, S. P., R. J. Olson, and G. M. Watters. 2013. Complex wasp-waist regulation of pelagic ecosystems in the Pacific Ocean. Reviews in Fish Biology and Fisheries 23 (4):459–475.
- Harvey, C., N. Garfield, E. Hazen, and G. Williams, eds. 2014. The California Current integrated ecosystem assessment: Phase III report. U.S. Department of Commerce, NOAA. http://www.noaa.gov/iea/CCIEA-Report/index.
- Harvey, C., and P. Kareiva. 2005. Community context and the influence of non-indigenous species on juvenile salmon survival in a Columbia River reservoir. Biological Invasions 7 (4):651–663.
- Kaplan, I. C., C. J. Brown, E. A. Fulton, I. A. Gray, J. C. Field, and A. D. M. Smith. 2013a. Impacts of depleting forage species in the California Current. Environmental Conservation 40 (4):380–393.
- Kaplan, I. C., I. A. Gray, and P. S. Levin. 2013b. Cumulative impacts of fisheries in the California Current. Fish and Fisheries 14:515–527.
- Kaplan, I. C., and J. Leonard. 2012. From krill to convenience stores: Forecasting the economic and ecological effects of fisheries management on the US West Coast. Marine Policy 36:947–954.
- Keefer, M. L., R. J. Stansell, S. C. Tackley, W. T. Nagy, K. M. Gibbons, C. A. Peery, and C. C. Caudill. 2012. Use of radiotelemetry and direct observations to evaluate sea lion predation on adult Pacific salmonids at Bonneville Dam. Transactions of the American Fisheries Society 141 (5):1236–1251.
- Kershner, J., J. F. Samhouri, C. A. James, and P. S. Levin. 2011. Selecting indicator portfolios for marine species and food webs: A Puget Sound case study. Plos One 6 (10):e25248.
- Larson, S., D. M. De Freitas, and C. C. Hicks. 2013. Sense of place as a determinant of people's attitudes towards the environment: Implications for natural resources management and planning in the Great Barrier Reef, Australia. Journal of Environmental Management 117:226–234.
- Levin, P. S., M. J. Fogarty, S. A. Murawski, and D. Fluharty. 2009. Integrated ecosystem assessments: Developing the scientific basis for ecosystem-based management of the ocean. Plos Biology 7 (1):23–28.
- Levin, P. S., and F. B. Schwing, eds. 2011. Technical background for an integrated ecosystem assessment of the California Current: Groundfish, salmon, green sturgeon, and ecosystem health. US Department of Commerce.
- Levin, P. S., B. K. Wells, and M. B. Sheer, eds. 2013. California Current Integrated Ecosystem Assessment: Phase II Report. U.S. Department of Commerce, NOAA, Seattle, WA. Available at http://www.noaa.gov/iea/CCIEA-Report/pdf/index.html
- Levins, R. 1974. The qualitative analysis of partially specified systems. Annals of the New York Academy of Sciences 231:123–138.
- Levins, R. 1998. Qualitative mathematics for understanding, prediction and intervention in complex ecosystems. In Ecosystem health, eds. D. Rapport, R. Costanza, P. Epstein, C. Gaudet, and R. Levins, 178–204. Malden, MA: Blackwell Science.
- Link, J. S., T. F. Ihde, C. J. Harvey, S. K. Gaichas, J. C. Field, J. K. T. Brodziak, H. M. Townsend, and R. M. Peterman. 2012. Dealing with uncertainty in ecosystem models: The paradox of use for living marine resource management. Progress in Oceanography 102:102–114.
- McClure, M. M., E. E. Holmes, B. L. Sanderson, and C. E. Jordan. 2003. A large-scale, multispecies status, assessment: Anadromous salmonids in the Columbia River Basin. Ecological Applications 13 (4):964–989.
- Melbourne-Thomas, J., S. Wotherspoon, B. Raymond, and A. Constable. 2012. Comprehensive evaluation of model uncertainty in qualitative network analyses. Ecological Monographs 82 (4):505–519.
- Metcalf, S. J., J. M. Dambacher, P. Rogers, N. Loneragan, and D. J. Gaughan. 2014. Identifying key dynamics and ideal governance structures for successful ecological management. Environmental Science and Policy 37:34–49.
- Moore, M., B. A. Berejikian, and E. P. Tezak. 2013. A floating bridge disrupts seaward migration and increases mortality of steelhead smolts in Hood Canal, Washington State. Plos One 8 (9):e73427.
- Norse, E. A. 2010. Ecosystem-based spatial planning and management of marine fisheries: Why and how? Bulletin of Marine Science 86 (2):179–195.
- Orians, G., M. Dethier, C. Hirschman, A. Kohn, D. Patten, and T. Young. 2012. Sound indicators: A review for the Puget Sound partnership. Olympia, WA: Washington State Academy of Sciences.
- Paulsen, C. M., and T. R. Fisher. 2001. Statistical relationship between parr-to-smolt survival of snake river spring-summer Chinook salmon and indices of land use. Transactions of the American Fisheries Society 130 (3):347–358.
- Peterson, W. T., J. L. Fisher, J. O. Peterson, C. A. Morgan, B. J. Burke, and K. L. Fresh. 2014. Applied fisheries oceanography ecosystem indicators of ocean condition inform fisheries management in the California Current. Oceanography 27 (4):80–89.
- Poe, M. R., J. Donatuto, and T. Satterfield. 2016. Sense of place: Human wellbeing considerations for ecological restoration in Puget Sound. Coastal Management, 44 (5).
- Puccia, C. J., and R. Levins. 1985. Qualitative modeling of complex systems : An introduction to loop analysis and time averaging. Cambridge, MA: Harvard University Press.
- R Core Team. 2013. R: A language and environment for statistical computing. Vienna, Austria: R Foundation for Statistical Computing. http://www.R-project.org/.
- Raymond, B., J. McInnes, J. M. Dambacher, S. Way, and D. M. Bergstrom. 2011. Qualitative modelling of invasive species eradication on subantarctic Macquarie Island. Journal of Applied Ecology 48 (1):181–191.
- Reum, J. C. P., B. E. Ferriss, P. S. McDonald, D. M. Farrell, C. J. Harvey, T. Klinger, and P. S. Levin. 2015. Evaluating community impacts of ocean acidification using qualitative network models. Marine Ecology Progress Series 536:11–24.
- Samhouri, J. F., A. J. Haupt, P. S. Levin, J. S. Link, and R. Shuford. 2014. Lessons learned from developing integrated ecosystem assessments to inform marine ecosystem-based management in the USA. ICES Journal of Marine Science 71 (5):1205–1215.
- Samhouri, J. F., and P. S. Levin. 2012. Linking land- and sea-based activities to risk in coastal ecosystems. Biological Conservation 145 (1):118–129.
- Schaller, H. A., C. E. Petrosky, and O. P. Langness. 1999. Contrasting patterns of productivity and survival rates for stream-type chinook salmon (Oncorhynchus tshawytscha) populations of the Snake and Columbia rivers. Canadian Journal of Fisheries and Aquatic Sciences 56 (6):1031–1045.
- Smith, M. D., E. A. Fulton, R. W. Day, L. J. Shannon, and Y. J. Shin. 2015. Ecosystem modelling in the southern Benguela: Comparisons of Atlantis, Ecopath with Ecosim, and OSMOSE under fishing scenarios. African Journal of Marine Science 37 (1):65–78.
- Ware, D. M., and R. E. Thomson. 2005. Bottom-up ecosystem trophic dynamics determine fish production in the Northeast Pacific. Science 308 (5726):1280–1284.
- Wright, B. E., S. D. Riemer, R. F. Brown, A. M. Ougzin, and K. A. Bucklin. 2007. Assessment of harbor seal predation on adult salmonids in a Pacific Northwest estuary. Ecological Applications 17 (2):338–351.