Abstract
Efficient non-chemical weed control like flame weeding often requires repeated treatments. In weed control experiments the effect of each treatment may be estimated by removing and weighing the remaining weed biomass after the treatment, but the method influences the weed plants ability to regrow, and therefore it may influence the long-term effect of repeated treatments. Visual assessment of weed cover or image analysis do not affect the remaining parts of the weed plants after treatment, but the methods may have other disadvantages. In order to evaluate and compare three methods we measured changes in vegetation cover of perennial ryegrass after flaming by (1) a simple image analysis programme counting green pixels, (2) visual assessment of images and (3) by taking biomass samples. Plants were flame treated with eight different dosages (0, 20, 30, 35, 45, 60, 90 and 180 kg propane ha−1) and with various treatment frequency (2, 4, 6, 8 and 10 yearly treatments). Image analysis and visual assessment of images were easy methods to measure vegetation cover. The experiments showed that increasing dosages and frequent treatments resulted in increasing reduction of plant weight and vegetation cover. However, there were significant differences in the estimated effective doses (e.g., ED50 and ED90 values) depending on assessment method and treatment frequency. One reason could be that image analysis and visual assessment did not affect the remaining weed parts after treatment and therefore gave a more realistic picture of the long-term effect of repeated treatments. Image analysis was most useful for assessing the effect of repeated treatments when weed cover was relatively low (below 40%) and when plots contained relatively much withered plant material. However, when weed cover is close to 100%, dry weight measurements reflected the effect of the treatment better.
Introduction
In many countries the risk of pollution of the environment has led to restrictions on pesticide use, and herbicides have been replaced by other means like flame weeding or mechanical weed control, e.g., in urban areas (Bond & Grundy, Citation2001; Kristoffersen et al., Citation2008a). These methods control a wide range of annual weed species, but plant species with protected meristems such as grass weeds and some perennial weed species pose a special problem because of their ability to regrow after treatment (Ascard, Citation1994, Citation1995; Andreasen et al., Citation2002). Therefore repeated treatments are necessary.
A literature study on non-chemical weed control on hard surfaces (Rask & Kristoffersen, Citation2007) revealed a need of proper definition of efficiency of the weed control methods. Different methods to measure the effect of non-chemical treatments may lead to different results (Hansson, Citation2002). Often dry weight of surviving plants is measured (e.g., Andreasen et al., Citation2002; Rask & Andreasen, Citation2007). It may give a useful and qualified estimate of the effect of a treatment, but may not be appropriate for measuring regrowth, because the plots, or part of them, will be destroyed by the harvest. Additionally, it may be very labour-intensive. Dead or withered plant parts needs to be removed before weighing and this will also increase the work load considerably. It may also be difficult to decide when a plant is dead. The plant may still have some green parts or cover, living shoots or buds even though most of the plant seems to be withered away (Rask et al., Citation2011). If the sample size is small, it may be possible to count the number of surviving plants.
Regrowth can also be estimated by visual assessment (Kristoffersen et al., Citation2008b; Melander et al., Citation2009) or by image analysis. However, visual rating of percentage weed cover is a subjective assessment method and may only be accepted when data are partly supported by an objective measurement method (e.g., Weed Technology, Editors Note, Citation2011). Several attempts have been done to use image analysis in weed science. Andreasen et al. (Citation1997) suggested a method to estimate weed densities by using image analysis and Gerhards et al. (Citation2002) used image analysis to identify weed seedlings. Hansson (Citation2002) used image analysis to assess the weed control effect of a hot water treatment on white mustard (Sinapis alba L.). Data were used to describe the dose–response relationship. In one experiment it was easy to analyse data with image analysis since wilted plant parts had a colour that separated them from the soil. This encouraged us to use the method in our experiments.
Our objective of this study was to compare three methods to measure the effect of repeated flame weeding of a common grass species (Lolium perenne L.). We used different dosages (0–180 kg propane ha−1) and treatment frequency (2–10 yearly treatments) and compared the result obtained from biomass samples, image analysis of green cover and visual assessments of green cover on images. Our hypotheses were that image analysis and visual assessment would be easier and a more precise method to estimate the effect of flame treatment over time than biomass measurements because image acquisition can be done rapidly and do not affect the remaining weed parts after treatment and therefore do not affect the weeds ability to regrow in the same way as if the biomass is removed. On the other hand, we also expected that use of images may be inappropriate if there is a low effect of the flaming and the weed biomass is large because of tall plants and/or a high leaf area index.
Materials and methods
Experimental design
Field experiments were carried out from May to October 2008. All experiments were conducted at Taastrup, Denmark (55°40′10N; 12°18′32E). After ploughing and harrowing, perennial ryegrass (L. perenne cv. Maurice) was sown in September 2007 on an area of 2600 m2 with a density of 25 kg seeds ha−1.
In April 2008, the area was divided into three block replicates. Each block was subdivided into 37 randomly distributed treatment areas (length 6 m, width 0.75 m) corresponding to 5 treatment frequency, 7 dosages () and 2 untreated areas. Within each treatment area three plots with dense and homogeneous vegetation (no bare soil) were selected before the experiment was set up. The plots were marked for repeated image registration (0.65×0.43 m). At the end of the experimental period, biomass samples were collected from these plots.
Table I. Treatment plan from May to October 2008.
Application details
Treatments were carried out with a HOAF thermHIT® 75M gas burner (HOAF Infrared Technology) mounted on a Kersten self-driving machine. The working width of the flamer was 0.75 m and mean gas consumption was calculated to 5.25 kg gas h−1. Different propane doses were achieved by modifying the operating speed. Time spent on each treatment was recorded and used to calculate the applied dose. The ground speed of the flame weeder was 0 (untreated control areas), 0.4, 0.6, 0.7, 0.9, 1.2, 1.8 or 3.6 km h−1, giving propane doses of 0, 20, 30, 35, 45, 60, 90 and 180 kg ha−1.
Biomass sampling procedure
Above ground biomass samples were collected in October 2008, one day after the images were captured. The samples were collected with a 0.1×0.4 m frame within the plots that were marked for repeated image analysis. A total of nine samples per treatment (3 samples per treatment area×3 blocks) were collected. In untreated areas a total of 18 samples were collected (3 samples×2 untreated areas in each block×3 blocks). The total number of biomass samples was 333 samples [(35 treated areas+2 untreated areas)×3 samples×3 blocks]. The samples were collected two weeks after the last treatment to assess the effect of the treatments on the regrowth. All leaves were cut 1 cm above the soil surface, dried for 36 h at 80°C and weighted. Withered leaves were not removed and therefore included in the total dry weights.
Image analysis
Images were captured every second week from April to October () when the weather condition allowed it. Data presented here are from October 2008, i.e., two weeks after the last treatments had been carried out. All images were taken with a Canon EOS 400D (ISO speed and shutter speed manually adjusted). Image colour was RGB (Red, Green, Blue) with 8 bits per colour component. To avoid camera shaking and to get images of the same size, a camera mounting was used. Camera position was vertical and the camera lens was placed 1 m above ground. To obtain diffuse light on sunny days the camera mounting was covered with nylon material on four sides. A frame (0.65 m×0.43 m=0.28 m2) was placed inside the tent. The objectives of the image analysis were to:
1. | Obtain a binary image where green leaves could be segmented from soil surface, withered grass and dark shadows. | ||||
2. | Remove the frame by combining the thresholded binary image with a mask before the final counting of green pixels. | ||||
3. | Make a general macro in ImageJ that could be used on all images. |
The analysis of the images was carried out by the open-source software ImageJ (http://rsbweb.nih.gov/ij/) with the plugin ‘Threshold colour’, and the colour image was represented as a HSB (Hue, Saturation, Brightness) stack. Thresholds for each parameter were set on basis of trying different thresholds on 50 random sampled images. The same threshold was used on all images, as the aim was to be able to run the macro on all images automatically.
Visual assessment
Visual assessment was done on the images that were used for image analysis. Percentage green cover within the frame was assessed on 333 randomly ordered images. Percentage bare soil was recorded as well.
Statistical analysis
Statistical analyses were performed using the open-source program R, version 2.11.1 (R Development Core Team, Citation2010) and the software package drc (Ritz & Streibig, Citation2005). The effect of flaming can be described by dose–response curves similar to those described by Streibig et al. (Citation1993) for herbicides:
where y is the response variable (biomass or green cover in relation to untreated plots (%) in this example) and x is the energy dose in kg gas ha−1. D is the upper limit of the curve (which is 100 when the relative response is measured) and C is the lower limit. The parameter e is equivalent to ED50, which is the dose to achieve a 50% reduction in dry weight or percentage weed cover. Parameter b describes the slope of the curve around e (inflexion point). At first the above four parameter model was chosen but it was not possible to fit the curves with this model. Lack of data points around the lower limit for some of the curves resulted in one case of negative C values. Knezevic et al. (Citation2007) recommended the use of the three parameter log logistic model in such a case. Moreover, this model was found to be more closely related to data, and it is expected that the response will go towards 0 with increasing dose:
The package drc in R provides functions to compute EDy values. EDy is defined as the dose that yields a response which is (100–y)% of the maximum response D (a reduction of y%). The values are derived from the regression model utilising the delta method (Knezevic et al., Citation2007). Estimates of ED50, ED80 and ED90 and their standard errors for all curves were calculated.
The appropriateness of the model was tested by a graphical check of the data. A residual plot showed a weak tendency towards increasing variation with increasing values, i.e., the variance was not constant. It was not possible to use Box–Cox transformation (Streibig et al., Citation1993) as many values in the data-set were zero or close to the lower limit.
Results and discussion
The effect of dose and treatment frequency
All assessment methods showed a relation between dose and treatment frequency on the reduction of plant weight or decrease in vegetation cover (). The higher dose the better weed control and the more treatments per year the better effect. The differences between assessment methods highly depended on the number of treatments as well as the dose. The main reason was that it was not possible to record three dimensional differences when vegetation was tall. When the dose was very low or the time interval between treatments was long, differences in treatment effect could only be measured by weighing plant material. Therefore, the response of the weeds after two treatments was not possible to measure with image analysis (B) or visual assessment (C) as these treatment areas were completely covered with vegetation. After four treatments there were relatively high amounts of withered grass in comparison with green leaves. Assessment by image analysis or visual assessment showed higher effect of four treatments in comparison with the biomass samples where withered leaves were included (A).
Figure 1. Response of Lolium perenne as a function of propane dose (kg ha−1) and number of flamings per year. (A) Response was measured as plant dry weight, (B) percentage green cover estimated by image analysis and (C) percentage green cover estimated by visual assessment on images. Each bar corresponds to the mean of nine replicates (three per block, 18 replicates for control plants (six per block).
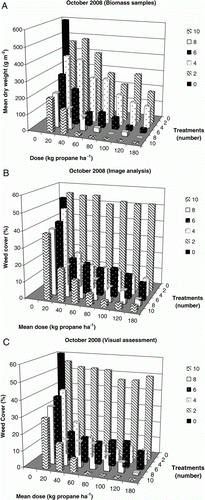
Sometimes the image analysis programme could not discriminate completely between yellowish soil, yellowish grass and green pixels. At very high doses and short treatment intervals, some pixels were counted as green even though there were no green leaves assessed by visual assessment. Therefore weed cover was not registered to be zero in any samples, when image analysis was used.
It is important, when choosing image analysis as assessment method, that the colours of the leaves can be segmented easily from the colours of the soil and withered leaves. In our experiment, perennial ryegrass had very light green leaves, almost yellowish, and it was difficult to discriminate between the colour of the soil and withered plant parts. However, it may be possible with another more advanced image analysis programme (e.g., Thomas et al., Citation1988; Hansson, Citation2002; Rasmussen et al., Citation2007). We tried to use a digital image analyzer programme developed by Rasmussen et al. (Citation2007) named ‘Imaging crop response analyzer’. It seemed promising when it was tried on the first images from a pilot experiment in 2007 with mixed grass species. However, it turned out to be inappropriate in our experiment in 2008, because the images were not bright enough or the algorithm was not appropriate for the leaf colour of perennial ryegrass.
Dose–response models on total dose requirement
The estimated total dose requirement per year varied between assessment method as well as number of treatments (A–D). Due to a lack of differences in weed cover between different total doses after two treatments it was not possible to fit these dose–response curves based on data from image analysis or visual assessment. After four treatments it was not possible to obtain 80% reduction in plant dry weight within the given dose range (; A). When the response was assessed by image analysis or visual assessment, weed cover was reduced by 90% with a total dose per year of 707 or 521 kg propane ha−1, respectively (; A). There was no significant difference between these two effective doses (P=0.09), neither between the slopes of these two curves; whereas the slope was significantly flatter when the effect was assessed by dry weights. The relatively high amount of withered plant parts in these samples indicates that measuring percentage green cover may be more precise in this case.
Table II. Equation parameters (model 2) and standard errors (SE) of the effect of flame treatment on perennial ryegrass after different number of treatments per year.
The results obtained by visual assessment were closer to the results obtained by dry weights and in our study visual assessment may give more precise estimations of percentage weed cover, due to the problems we encountered with colour segmentation. The biased nature of visual scores is not vital in experiments where the main objective is to compare different treatments within the same experiment. However, when results from different experiments are of interest, different people will assess the control level differently, which make comparisons problematic (Rasmussen et al., Citation1997, Citation2007).
After 6, 8 or 10 treatments (B–D) the total dose requirement to obtain 90% control of the weeds was estimated to be lower when the weed control effect was measured in dry weights in comparison with weed cover (). Actually, the total dose requirement was more than twice as high when the control effect was measured by image analysis (six or eight treatments a year). However, after 10 treatments there was no difference between ED90 whether the control effect was assessed by visual assessment or dry weights, as no weed regrowth was recorded after 10 treatments with mean doses above 80 kg propane ha−1 (A,C). Differences in total dose requirement between the assessment methods are mainly due to a lower response measured in weed cover at low doses because of overlapping vegetation. In summary, all assessment methods showed a relation between dose and treatment interval on the reduction of plant weight or decrease in vegetation cover. However, there were considerable differences between the estimated effective doses (ED90) when assessed by weights or weed cover, especially for small and large percentage of weed cover. Overlapping vegetation resulted in small changes in weed cover when treatment frequency or doses were low, whereas measuring the effect in dry weights revealed considerable differences in control effect. On the other hand, if withered grass had not been burned off at the last flame treatment, especially after four treatments, these weights may have a relatively higher impact on the results on total dry weight of the plants at low weights.
Figure 2. Dose–response curves for the total propane dose effect on relative plant dry weight or plant cover after flaming of perennial ryegrass with different number of treatments per year. (A) 4 Treatments per year, (B) 6 treatments per year, (C) 8 treatments per year and (D) 10 treatments per year. Each data point corresponds to the mean of nine samples. The upper asymptote (control plants) is based on the mean from 18 samples. Parameter estimates are given in . The total dose requirement was assessed by three different methods: Plant dry weight (DW), weed cover assessed by image analysis (IA) or visual assessment (VA).
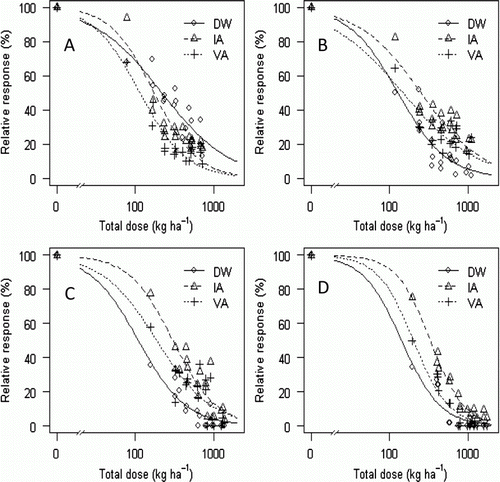
In order to get a good overview of the combination of treatment frequency and dosages some of the treatment areas developed into a dense pasture. In such situations flame weeding would not be chosen to control weeds in practice. Since image analysis was more successful when weed cover were low we recommend this methods when realistic and appropriate dose–response relationship has to be investigated for weed management programmes.
In conclusion, assessment of control effect after repeated treatments by image analysis is most useful when weed cover is low and when it is expected that the plots will contain relatively much withered plant material. However, when weed cover is close to 100%, dry weights reflect the effect of the treatment better.
References
- Andreasen , C. , Hansen , C. H. , Møller , C. and Kjær-Pedersen , N. 2002 . Regrowth of weed species after cutting . Weed Technology , 16 : 873 – 879 .
- Andreasen , C. , Rudemo , M. and Sevestre , S. 1997 . Assessment of weed density at an early stage by use of image processing . Weed Research , 37 : 5 – 18 .
- Ascard , J. 1994 . Dose-response models for flame weeding in relation to plant size and density . Weed Research , 34 : 377 – 385 .
- Ascard , J. 1995 . Effects of flame weeding on weed species at different developmental stages . Weed Research , 35 : 397 – 411 .
- Bond , W. and Grundy , A. 2001 . Non-chemical weed management in organic farming systems . Weed Research , 41 : 383 – 405 .
- Editors Note . 2011 . Visual ratings – weed technology's editorial policy . Weed Technology , 25 , 177 .
- Gerhards , R. , Sökefeld , M. , Timmermann , C. , Kühbauch , W. and Williams , M. 2002 . Site specific weed control in maize, sugar beet, winter wheat and winter barley . Precision Agriculture , 3 : 25 – 35 .
- Hansson , D. 2002 . Hot water weed control on hard surface areas . Doctoral dissertation, Swedish University of Agricultural Sciences, Department of Agricultural Engineering, 2002. Alnarp , Sweden . Report 323 .
- Knezevic , S. Z. , Streibig , J. C. and Ritz , C. 2007 . Utilizing R software package for dose-response studies: The concept and data analysis . Weed Technology , 21 : 840 – 848 .
- Kristoffersen , P. , Rask , A. M. , Grundy , A. , Franzen , I. , Kempenaar , C. , Raisio , J. , Schroeder , H. , Spijker , J. , Verschwele , A. and Zarina , L. 2008a . A review of pesticide policies and regulations on non-agricultural areas in seven European countries . Weed Research , 48 : 201 – 214 .
- Kristoffersen , P. , Rask , A. M. and Larsen , S. U. 2008b . Non-chemical weed control on traffic islands: A comparison of the efficacy of five weed control methods . Weed Research , 48 : 124 – 130 .
- Melander , B. , Holst , N. , Grundy , A. C. , Kempenaar , C. , Riemens , M. M. , Verschwele , A. and Hansson , D. 2009 . Weed occurrence on pavements in five North European towns . Weed Research , 49 : 516 – 525 .
- R Development Core Team . 2010 . R: A Language and Environment for Statistical Computing ( Vienna : R Foundation for Statistical Computing ). Accessed 11 August 2011, available at: http://www.R-project.org
- Rask , A. M. and Andreasen , C. 2007 . Influence of mechanical rhizome cutting, rhizome drying and burial at different developmental stages on the regrowth of Calystegia sepium . Weed Research , 47 : 84 – 93 .
- Rask , A. M. and Kristoffersen , P. 2007 . A review of non-chemical weed control on hard surfaces . Weed Research , 47 : 370 – 380 .
- Rask , A. M. , Kristoffersen , P. and Andreasen , C. 2011 . Controlling grass weeds on hard surfaces: The effect of time intervals between flame treatments . Weed Technolology , 26 : 83 – 88 .
- Rasmussen , J. , Lund , I. & Petersen , H. 1997 . Hvilken langfingerharve er bedst til korn? [Which is the best spring tine weeder in cereals?] . In A. Correll Proceedings 1997 of the 14th Danish Plant Protection Conference/Weeds ( Nyborg: Statens Planteavlsforsøg, Forskningscenter Foulum, Tjele ), pp. 203 – 213 .
- Rasmussen , J. , Nørremark , M. and Bibby , B. M. 2007 . Assessment of leaf cover and crop soil cover in weed harrowing research using digital images . Weed Research , 47 : 299 – 310 .
- Ritz , C. and Streibig , J. C. 2005 . Bioassay analysis using R. J . Statistical Software , 12 : 1 – 22 .
- Streibig , J. C. , Rudemo , M. and Jensen , J. E. 1993 . “ Dose-response curves and statistical models ” . In Herbicide Bioassays , Edited by: Streibig , J. C. and Kudsk , P. 29 – 55 . Boca Raton, FL : CRC Press .
- Thomas , D. L. , Dasilva , F. S. K. and Cromer , W. A. 1988 . Image processing technique for plant canopy cover evaluation . Transactions of the ASAE , 31 : 428 – 434 .