Abstract
In the years 2002–2005, special trials concerning the level of infection of pea varieties by downy mildew were performed in Poland. In these trials, the large number of varieties were tested in many locations (environments), separately on reach and light soils. Obtained trial data are unique because of the large scale of the performed investigations and also for the fact that all the observations were made by the same observer. In a paper, two methods of statistical analysis of such (ordered) data are compared.
Several models have been proposed for the statistical interpretation of ordinal data. One of the most popular is the cumulative-type fixed logistic model. In the present work, using two field pea data sets, we considered whether adding random effects to the simple logistic model can improve inference. It was investigated whether there is any difference between the decisions concerning varieties resulting from the simple logistic model and the proposed mixed logistic model. The two models were also compared in terms of goodness of fit. According to two applied goodness-of-fit statistics, the mixed model performed better in all the cases. Statistical analysis (what is important for practical agriculture) enabled identification of the most resistant and the most susceptible variety from the analyzed set of cultivars.
Introduction
In cultivar testing and plant breeding experiments, certain traits, such as frost resistance, lodging, breaking of straw or susceptibility to diseases, are assessed on an ordinal scale. The disease resistance of cultivars is an issue considered by many authors. Ajala et al. (Citation2003) have studied infection by downy mildew on maize populations, while Spetsov et al. (Citation2013) – resistance to powdery mildew and leaf rust in wheat lines and Nashaat et al. (Citation2004) – infection by downy mildew on brassica juncea. In most cases, the resistance is assessed in an ordinal scale. The cereal diseases caused by downy mildew is an often considered problem.
Downy mildew is one of the fungal diseases often appearing in pea fields in Poland. It is caused by a pathogen named Peronospora viciae (Berk.) Casp. f. sp. pisi Sydow. In case of early infection, it can considerably influence the yielding level. It is important especially when the pathogen infection is severe. High humidity and low temperatures are favorable for the pathogen appearing in the pre- and blossoming period. This type of studies, concerning the area of Poland in general, has not been carried out yet. Susceptibility of the varieties before and after registration status has not been known either. Therefore, this issue is worth recognizing.
To analyze this kind of data, several methods have been proposed in the literature (see, e.g. Simko & Piepho Citation2011, or Tutz Citation2012, and the references therein). One of the most popular models is the cumulative-type model, proposed and extensively studied by McCullagh (Citation1980). Bakinowska et al. (Citation2012) used a simple logistic model to analyze the susceptibility to downy mildew of some varieties of field pea in a series of cultivar registration trials. The same model was used by Bakinowska and Kala (Citation2007) for comparison of varieties of seed pea with respect to lodging. A generalized linear mixed model with a single variance component was applied by Kristensen (Citation2011) to analyze ordinal data from distinctness, uniformity and stability (DUS) trials.
The main aim of this work was to compare the performance of two logistic modes often used for ordered data: a fixed logistic model and a cumulative link mixed model with several variance components. The practical purpose was aimed at indication of the most resistant varieties. The data concerning the level of infection of Polish varieties tested in numerous locations form the basis of all considerations.
Materials and methods
Two data sets were used to compare the models, including the results of series of field trials with field pea conducted on two different soil types. All measurements were taken by the same phytopathologist.
Dataset 1 consisted of cultivar field trials carried out from 2002 to 2005 in Bobrowniki, Cicibor, Kaweczyn, Lubinicko, Marianowo, Maslowice and Wyczechy in a light soil. The trials were conducted in a randomized complete block design with five replicates. For each plot, one measurement of downy mildew was taken at the blossoming stage. The number of cultivars evaluated varied from year to year. During the 4 years of the study, 17 varieties were tested at 7 different sites (experimental stations) belonging to the Research Center for Cultivar Testing (see Bakinowska et al. Citation2012). In these trials, only varieties originary from Poland were evaluated.
Susceptibility to downy mildew was one of the observed characteristics. In each plot, the disease intensity was assessed on a disease severity scale from 0 to 5, where a score of 0 denotes the desired situation (no disease). It means that there were five measurements for each variety within each trial. In all the years, the highly susceptible cultivar ‘Hubal’ was used as the control.
Dataset 2 consisted of 27 trials performed from 2002 to 2005 in rich soil fields. Seven trials were conducted in 2002, 2003 and 2005 (not necessarily at the same sites each year). In 2004, there were only six trials. The number of cultivars evaluated varied from year to year: 27 varieties in 2002, 24 varieties in 2003 and 2004, and 18 varieties in 2005. As in dataset 1, all trials were conducted using a randomized complete block design with five replicates. From 2002 to 2004, cultivars were compared with the reference variety ‘Zekon’ and with ‘W45’ in 2005. Disease severity was assessed as described above.
Fixed logistic model
Pea cultivar registration trials in Poland include resistance to downy mildew, which is assessed on an ordinal scale. Usually, it is assumed that the observed data follow the multinomial distribution, which is determined by probabilities , where
is the probability that the jth variety (j = 1, … , b) belongs to the ith category (i = 1, … , a) with respect to the test trait (disease severity). It is obvious that for a given variety,
. Various methods have been proposed in the literature to model the ordinal responses (McCullagh & Nelder Citation1989). The most popular is the cumulative-type model, which has been extensively studied by McCullagh (Citation1980). This model can be written as (see, e.g. Miller et al. Citation1993; Halekoh et al. Citation2006; Bakinowska & Kala Citation2007 and the references therein):
(1) where
is the ith cumulative probability corresponding to units of the jth variety,
is the cutpoint of the ith category and
is the effect of the jth variety. When analyzing real data,
are replaced by
, where
denote observed frequencies.
The analysis has the aim of estimating the unknown probabilities and cumulative probabilities in model (1) based on the experimental data. The estimates of unknown probabilities in model (1) were obtained by the maximum likelihood method. The main difficulty is solving non-linear maximum likelihood equations. The solution can be obtained using iterative methods (see, e.g. McCullagh & Nelder Citation1989, p. 42; McCulloch & Searle Citation2001, p. 105) under the restriction that .
To test the hypothesis(2) against
Wald's test statistic was applied, which, under the null hypothesis, has an approximate
distribution (see, e.g. McCulloch & Searle Citation2001, p. 24).
Cumulative link mixed model
Statistical analysis of the considered type of data may also be performed using a mixed model approach. Taking into account the localities (sites) from which the data come, now the probability is the probability that the jth variety (j = 1, … , b) belongs to the ith category (i = 1, … , a) at the kth site (k = 1, … , c), and
for a given variety j at the kth site. Various methods have been proposed to model such responses (see, e.g. Simko & Piepho Citation2011; Tutz Citation2012). The cumulative link mixed model can be written as
(3) where
denotes the ith cumulative probability corresponding to the jth variety at the kth site,
is the cutpoint of the ith category and
is the effect of the jth variety. Cutpoint and variety effects are assumed to be fixed. The site effect and variety × site effect are assumed to be random, and are denoted by
and
, respectively. It is assumed that
and
.
As in the fixed logistic model, the main purpose of the analysis is to estimate unknown values of the cumulative probabilities in the cumulative link mixed model, using the data. The maximum likelihood method with Laplace approximation, under the restriction , was used for estimation of the unknown parameters of the cumulative link mixed model.
To test hypothesis (2), the t-test statistic was used:where
is the estimated effect of the jth variety and
is the estimated standard error of
. Under the null hypothesis, the test statistic t has a t-Student distribution (see, e.g. McCulloch & Searle Citation2001, p. 24, p. 105 and Allison Citation2001, p. 205).
Data were analyzed separately for each dataset and year. All calculations were performed in SAS (Statistical Analysis System, version 9.3) using PROC LOGISTIC GLM (for the fixed logistic model) and PROC GLIMMIX (for the cumulative link mixed model). The graphical presentation of the results was obtained using the R packages STATS (R Core Team version 3.0.1) and LATTICE (Sarkar Citation2008).
Results
The estimated values of variance components for both data sets are summarized in . In the case of both considered data sets, the variance component for sites was always larger than the variance component for variety × site interaction.
Table 1. Estimates of variance component parameters in the cumulative link mixed model for both data sets.
To compare the performance of models (1) and (3), two commonly used goodness-of-fit statistics, –2 log L and AIC (Akaike information criterion), were used.
The values of these statistics are given in (smaller values are better). For both data sets, the values of these two goodness-of-fit statistics were lower for the mixed model. The largest improvement in model performance was obtained for the second data set (rich soil) in the years 2003–2004, while the smallest was observed for the second data set in 2005.
Table 2. Values of goodness-of-fit measures for the fixed logistic model and the cumulative link mixed model.
For both data sets, the analyses provided estimates of unknown parameters ( and ). In the year 2002 for the first data set the most resistant was variety 3-Kos, while in all the years from 2003 to 2005 the best was variety 11-Sok (see ). In the case of the second data set (rich soil, see ), the variety 1-Ade had the highest susceptibility to downy mildew (estimated effect equal to −2.764), while the most resistant was the variety 11-Jav (estimated effect 1.875) in the year 2002. Both varieties differ significantly (at α = 0.01) from the reference variety 47-Zek. In the year 2003, the most resistant was variety 34-S97, whereas in years 2004 and 2005 the best was variety 39-Ter.
Table 3. Estimated parameters and their significance in both models for the light soil data set.
Table 4. Estimated parameters and their significance in both models for the rich soil data set.
For the light soil data set, the values of cumulative probabilities (given that there are no random effects; and ) were calculated, and these are plotted in .
Figure 1. Cumulative probabilities for the light soil data set (bright bars – fixed logistic model, black bars – the cumulative link mixed model, symbols y:v in the heads of columns denote last digit of year and variety numbers, respectively).
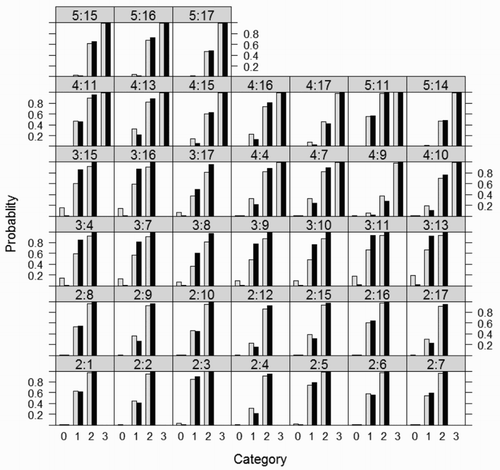
Using the cumulative probabilities, for each variety, the probabilities of obtaining a given score were calculated (). In most cases, the cumulative probability of receiving a score not larger than zero is higher for the fixed logistic model (bright bars) than for the cumulative link mixed model (black bars) (). A similar behavior can be observed in , where for all varieties the probability of obtaining the score 0 is higher for the fixed model than for the mixed model. No such regularity can be observed for scores of 3 and 4 on the right side of the scale. For some varieties, the bright bars are higher, while for others the dark bars are higher.
Figure 2. Probabilities for the light soil data set (bright bars – fixed logistic model, black bars – the cumulative link mixed model, symbols y:v in the heads of columns denote last digit of year and variety numbers, respectively).
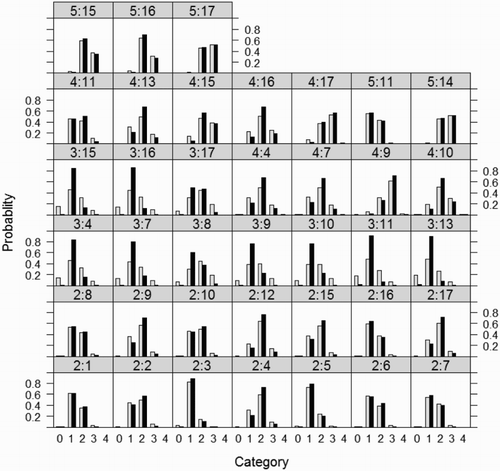
As regards the probability of particular scores (see ) as per the mixed model, the medium scores are more probable, which means that extreme scores are less probable. This phenomenon also influences the values of the cumulative probabilities (see ). As very similar figures were obtained for reach soil data, these are not included. The model used (fixed or mixed) can also potentially influence decisions concerning the choice of pea cultivars.
Discussion
Several methods were proposed for analyzing ordinal data. Mila et al. (Citation2004) applied a fixed logistic model to develop explanatory models of Sclerotinia stem rot prevalence in four states of the northern-central part of the United States. Hampel and Hartmann (Citation2011) studied multi-location frost resistance data from several years using a fixed logistic model, where cultivar and environmental effects were treated as fixed factors. Such an approach is often questioned, because the conclusions drawn from such analysis are valid for the experimental sites only, and cannot be extrapolated to the regions represented by those sites. For this reason, it was suggested that the environmental effects should be treated as random factors (Yates & Cochran Citation1938). In the present work, using two field pea data sets, we investigated whether adding random effects to the fixed logistic model can improve goodness of fit and inference about cultivar susceptibility to downy mildew, and subsequently influence the selection of pea varieties. In all cases, the mixed model was better according to two goodness-of-fit criteria ().
Based on the results, it can be concluded that adding random effects to the fixed logistic model had a positive influence in model performance in both data sets and resulted in a better fit (according to the two applied goodness-of-fit statistics). The largest improvement was obtained in 2003–2004 in dataset 2. Comparing the values of variance components for site effect and variety × site interaction (), it can be concluded that the mixed model is particularly appropriate when the ratio between the variance component for sites and variance component for variety × site interaction is large.
Because the restriction was imposed, the estimated effects for other (non-standard) varieties can be interpreted as a comparison with the standard variety. The estimates of the parameters of interest are quite similar as per these two models ( and ). Comparing the significance of the estimated variety effects, the decisions derived from the two models evaluated are similar for both data sets in most cases. However, there are also varieties for which the significance of their effects differs between the two models. An example is the variety ‘Pom’ in 2002 for the light soil data set (see ). This variety was significantly more susceptible than the standard (α = 0.05) according to the fixed logistic model, whereas according to the cumulative link mixed model, the same comparison was found to be not significant. Certain varieties were slightly more resistant than the reference variety in the fixed logistic model (), while in the cumulative link mixed model the same cultivars were significantly more resistant or significantly more susceptible to downy mildew (e.g. ‘Boh’ in 2004). Such behavior can be partly explained by the relationship of the site and variety × site interactions. The behavior of tested varieties (probabilities of receiving of particular scores) has been clearly illustrated using and .
In multinomial data, as for other distributions for count data, there is often a problem with overdispersion. This can be due to correlated observations or unobserved heterogeneity in the data. In data sets considered in this study, interplot inferences among treatments were observed, i.e. the more susceptible varieties obtained higher scores when they had a more resistant variety as a left neighbor. A similar fact was also observed by Chakraborty and Smith (Citation1995). The authors suggested including neighbor effects in the model. It would be interesting to check whether the use of a cycling design (John Citation1987) could improve the goodness of fit and possibly improve the inferences concerning tested varieties. We plan to explore this problem in our future work.
Another way to overcome the overdispersion problem is to use an approach similar to that proposed by Piepho (Citation1999). However, this cannot be done in a straightforward way, because the available software (SAS System) does not include such solutions for multinomial data (at least for now). From a practical point of view, one may use the approach proposed by Chen and Kuo (Citation2001), namely to fit the multinomial logit mixed model by the Poisson log-linear mixed model. However, further research is needed to solve this problem.
Other methods of handling multinomial data are described in the literature (see, e.g. Bathke et al. Citation2010; Simko & Piepho Citation2011). For this reason, it is interesting to compare the methods of modeling ordinal responses described in Simko and Piepho (Citation2010) with the generalized linear mixed models and the nonparametric approach (see Bathke et al. Citation2011). This problem will be explored in future work.
Disclosure statement
No potential conflict of interest was reported by the authors.
References
- Allison PD. 2001. Logistic regression using the SAS system: Theory and application. New York, USA: SAS Institute Inc. and Wiley.
- Ajala SO, Kling JG, Kim SK, Obajimi AO. 2003. Improvement of maize populations for resistance to downy mildew. Plant Breeding. 122:328–333. doi: 10.1046/j.1439-0523.2003.00867.x
- Bakinowska E, Kala R. 2007. An application of logistic models for comparison of varieties of seed pea with respect to lodging. Biometrical Lett. 44:143–154.
- Bakinowska E, Pilarczyk W, Osiecka A, Wiatr K. 2012. Analysis of downy mildew infection of field pea varieties using the logistic model. J Plant Protect Res. 52:264–270. doi: 10.2478/v10045-012-0038-z
- Bathke AC, Harrer SW, Wang H, Zhang K, Piepho HP. 2010. Series of randomized complete block experiments with nonnormal data. Comput Stat Data Anal. 54:1840–1857. doi: 10.1016/j.csda.2010.02.007
- Chakraborty S, Smyth GM. 1995. A stochastic model incorporating the effect of weather conditions on anthracnose development in Stylosanthes scabra. J Phytopathol. 143:495–499. doi: 10.1111/j.1439-0434.1995.tb04561.x
- Chen Z, Kuo L. 2001. A note on the estimation of the multinomial logit model with random effects. Am Stat. 55:89–95. doi: 10.1198/000313001750358545
- Halekoh U, Hojsgaard S, Yan J. 2006. The R package geepack for generalized estimating equations. J Stat Softw. 15:1–11. doi: 10.18637/jss.v015.i02
- Hampel D, Hartmann J. 2011. Testing frost resistance for cereals in the Czech Republic. Cultivar Testing Bull. 33:83–90.
- John JA. 1987. Cyclic designs. London, New York, GB, USA: Chapman and Hall.
- Kristensen K. 2011. Analyses of visually accessed data from DUS trials using a combined over years analysis for testing distinctness. Cultivar Testing Bull. 33:49–62.
- McCullagh P. 1980. Regression model for ordinal data (with discussion). J R Stat Soc B. 42:109–127.
- McCullagh P, Nelder JA. 1989. Generalized linear models. 2nd ed. London, UK: Chapman and Hall.
- McCulloch ChE, Searle SR. 2001. Generalized, linear, and mixed models. New York, USA: Wiley.
- Mila AL, Carriquiry AL, Yang XB. 2004. Modeling the prevalence of Sclerotinia stem rot of soybeans in the North Central region of the United States. Phytopathology. 94:102–110. doi: 10.1094/PHYTO.2004.94.1.102
- Miller ME, Davis CHS, Landis JR. 1993. The analysis of longitudinal polytomous data, generalized estimating equations and connections with weighted least squares. Biometrics. 49:1033–1044. doi: 10.2307/2532245
- Nashaat NI, Heran A, Awasthi RP, Kolte SJ. 2004. Differential response and genes for resistance to Peronospora parasitica (downy mildew) in Brassica juncea (mustard). Plant Breeding. 123:512–515. doi: 10.1111/j.1439-0523.2004.01037.x
- Piepho H-P. 1999. Analysing disease incidence data from designed experiments by generalized linear mixed models. Plant Pathol. 48:668–674. doi: 10.1046/j.1365-3059.1999.00383.x
- Sarkar D. 2008. Lattice, multivariate data visualization with R. New York, USA: Springer.
- Simko I, Piepho H-P. 2011. Combining phenotypic data from ordinal rating scales in multiple plant experiments. Trends Plant Sci. 16:235–237. doi: 10.1016/j.tplants.2011.02.001
- Spetsov P, Daskalova N, Plamenov D, Moraliyski T. 2013. Resistance to powdery mildew and leaf rust in wheat lines derived from a Triticum aestivum/Aegilops variabilis cross. Turkish J Field Crops. 18:128–133.
- Tutz G. 2012. Regression for categorical data. Cambridge, UK: Cambridge University Press.
- Yates F, Cochran WG. 1938. The analysis of groups of experiments. J Agric Sci. 28:556–580. doi: 10.1017/S0021859600050978