ABSTRACT
The potential of modified spring barley crops with improved nitrogen (N) use efficiency to reduce nitrogen (N) leaching and to increase soil organic carbon (SOC) storage was assessed at the regional scale. This was done using simulation model applications designed for reporting according to the Helcom (Helsinki Commission) and Kyoto protocols. Using model simulations based on modified crops N dynamics and SOC were assessed for three agro-ecological regions (latitudes ranging 55°20′–60°40′ N) in Sweden over a 20-year period. The modified N use properties of spring barley were implemented in the SOILNDB model (simulating soil C, N, water and heat, and plant N dynamics) by changing the parameters for root N uptake efficiency and plant N demand within a range given by previous model applications to different crops. A doubling of the daily N uptake efficiency and increased N demand (by ca 30%) reduced N leaching by 24%–31%, increased plant N content by 9%–12%, depending on region. The effects of the modified crop on SOC was simulated with the ICBM model, resulting in an increased SOC content (0–25 cm depth) by 57–79 kg C ha−1 y−1. The results suggest that a modified crop might reduce N leaching from spring barley area, in a range similar to the targets of relevant environmental protection directives, a result which held more in the northern than southern regions. The simulated SOC increase on a hectare basis was highest for the central region and least for the most northern region. For the total agricultural area the share of spring barley area was small and more crops would need to be modified to reach the emission reduction targets.
Introduction
A strong motivation for the development of genetically modified (GM) crops is that they can significantly reduce the environmental impact of agriculture on a large scale. However, observations of such large-scale effects can be obtained only many years after the GM crops have been introduced into the market, and thus their promises are based on predictions. How can such predictions (hypotheses) be based on scientifically tested methodologies? In this study we apply the methodologies that are used to assess the effects of the current crops on nitrogen (N) leaching and soil organic carbon (SOC) at the regional and national scales (e.g. HELCOM Citation2011; Blombäck et al. Citation2011a, Citation2011b; the Kyoto protocol, SNV Citation2015). The simulation models used (SOILNDB, Johnsson et al. Citation2002; ICBM, Andrén et al. Citation2004) are based on ecophysiology processes and have been tested in scientifically evaluated field experiments (e.g. Johnsson et al. Citation1987; Eckersten & Jansson Citation1991; Andrén & Kätterer Citation1997; Jansson et al. Citation1999). Because of the computational nature of the problem (i.e. extrapolation in space and time) the models have only been tested against a few of all the growing conditions that calculations are made for. But by introducing the proposed changes of GM crops into the current national calculation systems, we can create hypothetical assessments of what the impact of GM crops might be and identify observations needed to test these hypotheses. These types of analyzes can make a contribution to the development of GM crops that significantly reduce the environmental impact of agriculture, by evaluating how modification of specific crop traits might impact ecosystem C and N dynamics under variable growing conditions in the field.
High nutrient supply rates in intensive cropping systems are a prerequisite for high yields with high protein content. However, they also increase the risk for N losses (e.g. N leaching to the Baltic Sea; Blombäck et al. Citation2013). Genetically improved crop varieties might be key components in the development of more resource efficient high-yielding cropping systems that utilize the applied nutrients more efficiently. Increased allocation within the plants towards denser and deeper root system or overexpression of N transporting proteins in roots are both possible breeding targets for improving N uptake efficiency (McAllister et al. Citation2012; Skovby Rasmussen et al. Citation2015). Since enhanced crop yields also lead to the production of more crop residues and roots, these new traits would also increase C input to the soil and result in higher soil carbon sequestration (Kätterer et al. Citation2012).
Introducing genetically modified cereal traits with enhanced nitrogen uptake capacity may also reduce both concentrations of inorganic nitrogen in soil and the amounts of N fertilizers required for obtaining a certain grain yield. This in turn may reduce the risk of N leaching, N2O emissions and the amount of energy used to manufacture fertilizers (Tidåker et al. Citation2016).
The results from field studies with spring barley are promising (Good et al. Citation2007). For wheat, Foulkes et al. (Citation2009) proposed that Alanine aminotransferase would influence both N uptake efficiency (grain yield/N availability) and N utilization efficiency (grain yield/plant N). For spring barley, it has been hypothesized (Beatty et al. Citation2013) that an overexpression of alanine aminotransferase in the roots, and its subsequent transportation to shoots, leads to an increased demand for nitrogen. This hypothesis has been supported by observations of increased grain yield at the whole plant or crop level, as mentioned above.
To evaluate the potential of these types of breeding efforts on scales larger than those covered by controlled experiments, there is a need to assess the significance of the benefits at regional and national scales. To do this, previously tested process based models can be used by mimicking the modifications of the crops in terms of model parameters representing eco-physiological processes. Dynamic modeling frameworks (e.g. DSSAT, Jones et al. Citation2003; APSIM, Keating et al. Citation2003) provide such measures of crop productivity and environmental impacts. For Swedish conditions the SOIL/SOILN models (Eckersten & Jansson Citation1991; Jansson & Halldin Citation1980; Johnsson et al. Citation1987) and their successors (SOILNDB, Johnsson et al. Citation2002 and ICBM, Andrén et al. Citation2004) have frequently been used to simulate crop and soil C, N, water and heat conditions, and provide metrics for evaluating productivity (biomass yield), nutrient use, changes in SOC stock and N leaching. The SOIL/SOILN models were originally developed and tested for field scale application and are based on similar principal eco-physiological processes as in many other crop-soil models (e.g. Hay & Porter Citation2006).
As a part of a Swedish national research program (MistraBIOTECH; SLU Citation2016) with a mission to study the potential biological and economic impacts of higher yielding crop types, the overall objective of our study was to explore the possible effects of modified spring barley crops that are more efficient in utilizing soil N compared to present varieties.
By introducing changes into the SOILNDB and ICBM model applications in the form of scenarios, we assess the effects that modified crop properties might have on the protocols used in national and regional strategic decisions. The evaluations go beyond most ‘ideotype’ studies (e.g. Montesino-San Martin et al. Citation2014; Rötter et al. Citation2015) since they take into account spatial variations in field conditions within the region by weighting 1-D simulations for different soil types, climates and management practices on an aerial basis. The same tools are frequently used to support strategic policy decisions, thus considering regional natural conditions and management practices.
Material and methods
Model description
The effects of the modified crop on plant N and N leaching are simulated with the SOILNDB model, whereas the effects on SOC are simulated separately with the ICBM model, each model using their respective regional database frameworks. These two models have both been developed from the basic model concept of the SOIL/SOILN models, which consists of two spatially one dimensional models. The SOIL model (Jansson & Halldin Citation1980) simulates water and heat dynamics of a one dimensional multilayered soil profile, which in turn provides daily inputs to the SOILN simulations of carbon (C) and nitrogen (N) dynamics in the corresponding soil profile and plant N uptake (the SOILN model original version has crop biomass as input; Johnsson et al. Citation1987).
Plant N uptake in the SOIL/SOILN models is the lowest value of either the N available for root uptake or the plant N demand. N availability is equal to the fraction (UPMA; d−1) of the soil mineral N content that the roots can take up per day, while N demand is proportional to statistical crop yields averaged over several years, and increased by a constant fraction (Pot-N) to allow for between year variations. A fraction of the plant N is returned to soil as litter (a fixed C/N ratio is used). The soil is divided into layers depending on soil profile. The combination of water flows among layers and mineral nitrate-N concentrations in the soil water determines N leaching and denitrification. The soil carbon content is determined by the net balance between C input from plant litter, and the C output due to microbial decomposition as influenced by soil temperature and water conditions. The soil organic matter is divided into two pools of different quality represented by specific decomposition rates, one fast and one slow pool, respectively.
The SOILNDB model is a version of the field based SOIL/SOILN-models adapted to simulate N leaching on a regional scale as part of the NLeCCS framework (Nutrient Leaching Coefficient Calculation System; Johnsson et al. Citation2002; ). The main output is coefficients for N leaching from fields (kg N ha−1 y−1) for each crop and soil type combination, for the production areas concerned. The model has been extensively used to assess N leaching coefficients for all Swedish agricultural production areas (Kyllmar et al. Citation2005; Johnsson et al. Citation2008), to provide inputs to catchment models simulating N loads in the river outlets to the sea (cf. Blombäck et al. Citation2013). The NLeCCS framework includes calculations using SOILNDB, a crop sequence and management generator, time series of meteorological data, and agricultural statistics of crops and area distribution, standard yields, normal fertilization rates and crop management practices. The simulations are made for a large number of years using the meteorological time series repeatedly to provide N leaching coefficients that are normalized with respect to year to year variation in weather conditions and crop production.
Figure 1. Schematic description of the SOILNDB model system (Kyllmar et al. Citation2005).
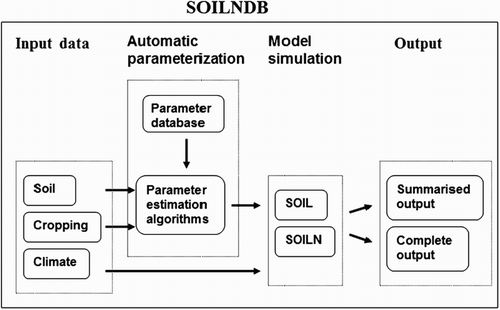
The ICBM model is also based on the SOIL/SOILN field models described above, but has been simplified to be suitable for making projections of soil C dynamics at field, regional or national scale (Andrén & Kätterer Citation1997; Kätterer & Andrén Citation2001). The ICBM model takes C input to the soil as input data (estimated from average crop yields), and while subsequently using the same principle equations for decomposition of organic matter as the SOILN model, it is structurally simplified to one single topsoil layer. This simplification reduces the number of parameters needed to five (). In ICBM, the soil temperature and water conditions are simulated based on inputs for daily standard weather station data (air temperature and total precipitation), specific soil properties and crop characteristics (Fortin et al. Citation2011). ICBM is currently used for national reporting on greenhouse gas emissions from mineral agricultural soils in Sweden (Andrén et al. Citation2004, Citation2008), and has also been used for other regional assessments of changes in soil C stocks in, e.g. the USA (Lokupitiya et al. Citation2010) and Norway (Borgen et al. Citation2012).
Figure 2. ICBM model showing soil carbon pools, fluxes and parameters. Y and O denote ‘young’ and ‘old’ pools, respectively (i: annual input; kY and kO: decomposition rate constants for Y and O, respectively; h: humification ratio; re: external rate multiplier). (Andrén & Kätterer Citation1997).
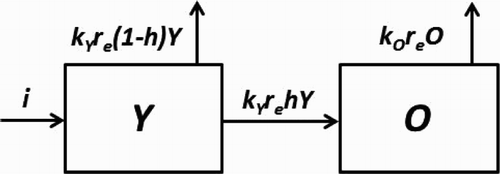
Model application scenarios
The two latest national assessments (Blombäck et al. Citation2011b and SNV Citation2015) provide the outputs for two baseline scenarios. In addition the two models are here used to evaluate five modified spring barley crop scenarios for three agro-ecological regions in Sweden (). These regions are combinations of a set of production regions used to perform the national assessments. In the two baseline scenarios N leaching, total plant N and soil C storage were assessed under current conditions for the selected regions, using daily weather and official statistics on management and yields as input. Twenty year periods were simulated for both baselines, but the N scenarios (1985–2004) period started a few years earlier than the SOC scenarios (1990–2009). Management and spatial and temporal arrangements of barley fields within the regions in the modified crop scenarios were the same as in the baseline scenarios. Two sections below describe the N scenarios (baseline and modified crop, respectively), and the following two sections the corresponding SOC scenarios.
Figure 3. Regional areas of the scenario studies in Sweden. Areas enclosed by solid, dashed and dotted lines are regions South, Central and North, respectively, including areas within latitudes from 55°20′ to 60°40′N (ca 580 km), and longitudes from 12°00′ to 18°40′E. Stars are network climate stations used. (Background map is taken from Johnsson et al. Citation2008; figures refer to the 18 Swedish production regions (P18) used in the national assessments; see text.) Region North consist of sub-regions NorthWest (5b) and NorthEast (6). The open circle in sub-region NorthEast is the Uppsala site (see Discussion).
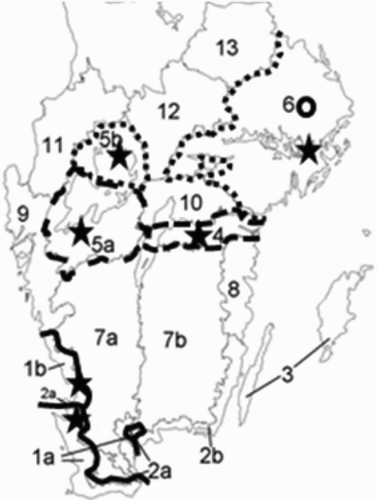
Site and data description
The spring barley area was a smaller share of the total agricultural area in region Central, than in the other two regions. The spring barley ‘standard-yields’ (corrected for between year variations and trends; SCB Citation2012) in regions South and Central were about 30% and 10% higher than in region North, respectively. Also, the regional averaged mineral fertilization rate was higher in region South. However, considering that the areas fertilized with mineral and organic N differed among regions the average total fertilization amounts per hectare were similar for regions South and Central (106, 108 and 97 kg N ha−1 for region South, Central and North, respectively; ).
Table 1. Baseline N scenarios; inputs to simulations. Yield and fertilization refer to annual values for spring barley. (calculated from Blombäck et al. Citation2011b).
For each study region, nine major crop types and ten mineral soil textural classes were defined (; organic soils were excluded in the N scenarios but not in the SOC scenarios, see Discussion). In region South more than two-thirds of the area is sandy loam or loamy sand with the rest primarily loam, whereas in region Central about 50% is sandy loam or loam and the rest soils with clay. In region North soil types with clay are dominant covering two-thirds of the area. All crop types were assumed to be equally distributed among soil types within the regions. Other inputs from official statistics were catch crop area and timing of management practices including soil cultivation, application of fertilizers and duration of fallows (Andrén et al. Citation2008; Blombäck et al. Citation2011b). The daily weather records for mean air temperature, relative air humidity and wind speed, and daily sums of precipitation and global radiation, were taken from two representative network stations per region (Barkåkra and Halmstad, Linköping/Malmslätt and Såtenäs, and Karlstad airport and Stockholm, for region South, Central and North, respectively; SMHI, www.smhi.se). In the N scenarios the precipitation data were corrected to adjust the simulated water discharge from a field to fit the average runoff on a catchment level as simulated by hydrological models from SMHI (Swedish Meteorological and Hydrological Institute; see further Brandt et al. Citation2008). The regional averaged run off was 357, 290 and 213 mm y−1 in region South, Central and North, respectively. Average air temperature was 8, 7 and 7°C, respectively. Average length of vegetation period during 1961–1990 was 210 days or more, 190–200 days and 180–190 days for the three regions, respectively, and the ten-year average has increased by 1–2 weeks from 1990 to 2010 (SMHI Citation2017).
Table 2. Percentage (%) area of soil types in each region (recalculated from Blombäck et al. Citation2011b; organic soil types were not included in the assessments).
For the SOC-scenarios, the agricultural area and yield records for each crop type and region, as well as statistics on manure application rates (Andrén et al. Citation2004) were taken from the same sources as for the N scenarios. However, due to the different aggregation levels and simulation periods these records were slightly different compared with the N scenarios (see Discussion). The total agricultural area was 332, 523 and 612 103 ha, spring barley area proportion 21%, 9% and 18%, and average yield 5261, 4542 and 4195 kg ha−1 y−1 for regions South, Central and North, respectively. Due to the same reasons, and that precipitation data were not adjusted to runoff data, also the climatic driving variables differed slightly compared with the N scenarios although taken from the same network of meteorological stations (Andrén et al. Citation2008).
Baseline N scenarios
The baseline N scenarios (1985–2004) representing agricultural conditions of 2011 were taken from the national assessments by Blombäck et al. (Citation2011b), following a similar structure as used in assessments for 2005 by Johnsson et al. (Citation2008). The parameterization of soil and crop components were the same as used by Johnsson et al. (Citation2002; Citation2008), except that plant N demand parameters were adapted to fit simulated N yield concentrations to the standard biomass yields of 2011 (SCB Citation2012). The N assessments of six sub-regions in the main agricultural areas, out of the 18 production sub-regions of Sweden (P18; Blombäck et al. Citation2011a, Citation2011b), were grouped into three larger regions (South, Central and North, respectively; ) to be comparable with the regions of the SOC scenarios. For each of the six sub-regions, separate simulations were made for each type of soil () using data from one climate station per sub-region (), resulting in soil type specific estimates of N leaching and plant N per hectare. Averages for these six sub-regions were then calculated by weighting these outputs in accordance with the areal fractions of each soil type. Thereafter, an average hectare value for each of the three larger study regions was calculated by averaging the hectare values (e.g. kg N ha−1 y−1 of N leaching) of the six sub-regions, weighted by their spring barley areas.
In the baseline, region South had clearly the highest plant N uptake (16–19 kg N ha−1 y−1 higher than the other two regions), N leaching was also highest from region South, more than 15 and 30 kg N ha−1 y−1 higher than in region Central and North, respectively. These differences were related to N mineralization simulated to be 138, 123 and 105 kg N ha−1 y−1, and N deposition estimated to be 12, 7 and 6 kg N ha−1 y−1 in regions South, Central and North, respectively. Outputs of baseline scenarios are presented in below for comparison with modified crop scenarios.
Modified crop N scenarios
There were no quantitative seasonal field estimates available for the UPMA model parameter (i.e. the fraction of soil mineral N that could be taken up by roots per day) that could be interpreted for their use in the model. Therefore UPMA was set to cover the range of estimates available from model applications to field experiments on other crops with quite different UPMA values (cereals e.g. Blombäck et al. Citation2003; leys e.g. Eckersten et al. Citation2007; spruce forest Eckersten & Beier Citation1998). For the modified crop N scenarios UPMA was either increased by 50% or doubled. This was combined with either Pot-N (potential N demand) being increased to 40%, or using the baseline value of 10% (). The Pot-N parameter is not directly comparable with corresponding parameters of crop growth models, but a value of 40% might correspond to an increase of radiation use efficiency by about 30%, which in turn corresponds relatively well to the changes used to mimic the effects of a doubling of atmospheric carbon dioxide concentration in experiments and climate change impact assessment studies (e.g. Downing et al. Citation2000; Eckersten et al. Citation2001). For computational technical reasons the changes of UPMA and Pot-N were applied to all crops in the crop sequence, not only spring barley (see Discussion).
Table 3. Modified crop N scenarios. Baseline scenarios were taken from the national assessments by Blombäck et al. (Citation2011b).
In the model an increased Pot-N would lead to greater N uptake when there is enough soil mineral N in the root zone; this in turn causes the soil water nitrate concentration to decrease, resulting in reduced N leaching. The increased plant N uptake would eventually reduce the soil mineral N content to levels below which N uptake becomes limited by the root N uptake efficiency. In this case, the increased N demand would have no effect, and instead increased N uptake efficiency (UPMA) would be needed to promote plant N uptake.
Baseline SOC scenarios
The baseline SOC scenarios (1990–2009) were taken from the Swedish National Reporting System (SNV Citation2015), as described in Andrén et al. (Citation2004, Citation2008). The SOC stock changes were calculated for the topsoil (0–25 cm) layer of mineral agricultural soils. The national SOC reporting system aggregates the 18 regions (PO18) used in the corresponding N leaching reporting system into 8 regions (PO8). Three of these regions, the same as for the N scenarios (), were selected to represent the baseline SOC scenarios (in the special national scenarios all eight regions were used). The model output was an average annual change in SOC stocks (Mg C ha−1 y−1) for each selected PO8 region, due to the introduction of a modified crop. The climate-management parameter (re: ) was first calculated for each weather station, and thereafter averaged for the region concerned (Andrén et al. Citation2008).
ICBM has been calibrated using data from a Swedish long-term (35 years; 1956–1991) field experiment in Uppsala, the Ultuna frame trial (Andrén & Kätterer Citation1997). The SOC pools (in ) used in the simulations were initialized based on a database from the Swedish national soil monitoring program with averaged observed values of 70.8, 81.8 and 80.9 Mg C ha−1 for regions South, Central and North, respectively (Andrén et al. Citation2008; Poeplau et al. Citation2015).
Carbon inputs to soil were calculated from yield using allometric functions developed from detailed investigations of carbon allocation for agricultural crops (Andrén et al. Citation2004). The proportion of above-ground harvest residues left on the field was lowest in region South (0.53, 0.76 and 0.77 for regions South, Central and North, respectively). This, together with region-specific organic fertilizers, provided C inputs to soil being one of the main driving variables of the ICBM simulations.
The soil climate-management parameter (re), which summarizes the external influences on soil biological activity and modifies decomposition rates and acts as a multiplier in the first-order decay rates of the two SOC pools (main equations are given in ; Fortin et al. Citation2011; Bolinder et al. Citation2013), was estimated on a daily basis. Differences in simulated soil temperature and moisture conditions among regions resulted in re being about 25% higher in region South than in the other regions (Andrén et al. Citation2008).
Modified crop SOC scenarios
SOC changes due to the introduction of the modified spring barley were simulated as for the baseline scenario, except that at the end of each year the litter transferred into the soil (i; ) the following year was increased. These increases were taken the same as the relative change of crop total biomass at harvest () simulated by corresponding scenarios for a site in region North (Uppsala site, ; Eckersten et al. manuscript Citation2017; see Discussion). This was expected to mimic the effects of introducing the modified spring barley crop as monoculture cultivations year after year. The increased annual C input to soil would increase the SOC content, but how much depends on decomposition losses. Since yields were higher in region South than in North, C inputs in absolute values would increase most in the South region. However, this increase would be counteracted by a lower fraction of crop residues left in the field at harvest, and a higher decomposition rate due to a higher microbial activity (re). The litter increase was introduced only to the area cultivated with spring barley.
Results
Modified crop N scenarios
Regional N leaching responded (in relative terms) most to the modified crop in region North and least in region South, due to differences in management, soil types and/or climatic conditions, in turn influencing N demand. The reduction of N leaching ranged from 13% to 20% due to increased Pot-N (the y-values deviation from 1.00 for x = 1.00 in ), whereas the reduction due to increased N uptake efficiency (UPMA) alone was lower (10%) and did not differ among regions (parallel curves in ). The Plant N response to increased N demand also differed among regions, but in relative terms much less than N leaching, and the response was lowest for region North and highest for region Central. The relative response of plant N to increased N uptake efficiency (UPMA) was low (upper curves in ).
Figure 4. Relative changes compared with baseline scenario (y-axis) of simulated annual plant N content (y-values > 1) and N leaching (y-values < 1) per hectare for a modified crop with Pot-N = +40% and different relative changes of UPMA (x-axis). The x-axis is the relative change of UPMA; x = 1 corresponds to UPMA = 0.08 d−1 and Pot-N = +40%. Solid (◊), dashed (O) and dotted (X) lines are region South, Central and North, respectively.
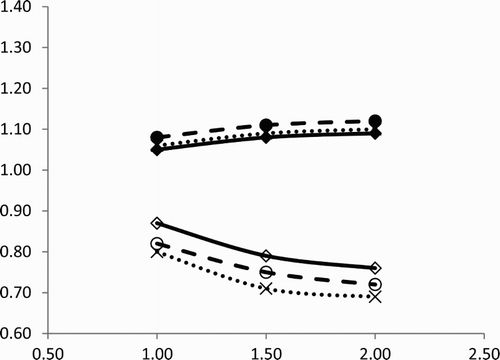
In absolute terms increased N demand (alone) increased plant N least in regions North (4.7 kg N ha−1) and South (4.9 kg N ha−1), and most in region Central (6.6 kg N ha−1). The absolute reduction of N leaching was highest in regions South and Central (6.0 and 5.6 kg N ha−1, respectively) and significantly less in region North (3.2 kg N ha−1). For the most modified crop (including both UPMA and N demand increase) the plant N increased most in region Central (8.8, 9.8 and 7.9 kg N ha−1 for regions South, Central and North, respectively), and N leaching decreased most in region South (11.1, 8.6 and 4.9 kg N ha−1, respectively; ).
The regional soil N balances were slightly changed (2 kg N ha−1 y−1 or less) by introducing the modified crop. In region South the soil N balance became less negative, in region Central it did not change and in region North it became negative. The difference between regions decreased from 27 to 23 kg N ha−1 y−1 ().
The reason of N leaching being highest in region South compared with the other regions, was related to the higher runoff from this region. Then the modified crop with improved utilization of the available soil N (which reduced soil mineral N concentrations) reduced N leaching most efficiently in region South. The plant N increase of the most modified crop being highest in region Central was due to the increase of N demand (Pot-N value). This can be explained by the differences in soil N balance indicating higher N availability in region Central than in region South. The reason why the plant N increase in region Central was higher than in region North (despite the higher N balance of that region) was due to the more favorable climate and higher potential (only weather limited) yields of region Central.
Modified crop SOC scenarios
The simulated increase in SOC stocks was linearly related to the increase in the C input factor. The increase of SOC showed a similar difference among regions as the differences in plant N, being highest for region Central, and least for region North (). In region Central the input of C was high due to a large fraction of crop residues being left in the field (i was higher; ), and a lower decomposition rate (re was lower due to climatic conditions; ), compared with region South. The reason for the higher SOC increase compared with region North was the higher yields in region Central (; i was higher in ). The responses were mostly due to changes in UPMA (), explained by the plant biomass changes (taken from the site scenarios of a relatively light soil) being sensitive to UPMA changes ().
Figure 5. Simulated mean (1990–2009) annual soil organic carbon (SOC) increase compared with baseline scenario (y-axis; kg C ha−1 y−1) for a modified crop with increased N demand and different relative changes of UPMA (x-axis). The x-axis is the relative change of UPMA; x = 1 corresponds to no increase of UPMA but increased N demand. Solid (◊), dashed (O) and dotted (X) lines are region South, Central and North, respectively (values from ). Long dashed (Δ) line is the national average value (of 8 regions). All changes refer to hectare of spring barley, except for the lowest line which is the national average per hectare of total agricultural area.
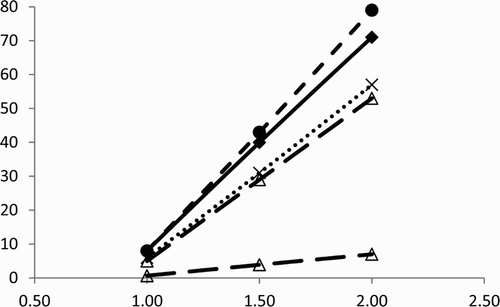
Table 4. Modified crop SOC scenarios. Baseline scenarios were taken from the Swedish National Reporting System 1990 to 2009, and changes of (i) values from Eckersten et al. (manuscript Citation2017).
Because the total spring barley area was larger in region North than in region Central, the modified crop increased regional SOC most for region North, although the increase per hectare spring barley area was lowest. For instance, for the most modified crop, the total SOC increase for regions North and Central was 6213 and 3581 Mg C y−1, respectively, whereas the corresponding average increase per hectare spring barley was 56.5 and 79.4 kg C ha−1 y−1, respectively. For region South both these increases were in between (4893 Mg C y−1 for the region and 70.7 kg C ha−1 y−1 for the average field, respectively).
The average SOC increase per hectare for all Swedish agricultural land cropped with a modified spring barley crop was estimated (as an average of all eight P08 regions) to be 5, 24, 29, 45 and 53 kg C ha−1 y−1 for the modified crop scenarios #C1N, # C2N, # C3N, # C4N and # C5N, respectively (), which were slightly lower SOC increases than for region North due to the influence of the P08 regions located in the north of the region denoted North in our study. On a national level the average proportion of spring barley area of the total agricultural area was 12.9%. Thus, when expressed per hectare of Swedish total agricultural land the effect of the modified crop was much less ().
Discussion
The relative reductions of N leaching due to the modified crop (ranging 7%–31%; ; ) covered the range of targets set up by the EU Water Framework Directive. In a study assessing possible reduction of N losses to the Baltic sea from a catchment located in region North, the target reduction was set at 22%, which was achieved in that study by altering the cropping systems (Blombäck et al. Citation2013). In another study, for region South, the maximum reduction was assessed to be 21%, when implementing all reasonable management operations known to reduce N leaching (Larsson et al. Citation2005). Both these levels of reductions were achieved in our study by increasing the potential N uptake (Pot-N; from 10% to 40%) without changing N uptake efficiency (UPMA), in regions Central and North. In region South, UPMA also needed to be increased (by 50%) to meet the targets. A change of UPMA alone was in no region, enough to reduce N leaching by more than half than required to meet the environmental targets.
Table 5. The effect of baseline and modified barley crop properties on harvested N, N leaching, and soil C change.
The inter-annual variation of SOC due to the modified crop (ca ± 200 kg C ha−1 y−1 for the baseline scenario; data not shown) was higher than the average effect of the modified crop (up to 80 kg C ha−1 y−1; ; ). This SOC sequestration rate is lower than that of other management options under Nordic conditions, such as the introduction of cover crops (Poeplau et al. Citation2015) or leys in arable rotations (Kätterer et al. Citation2012), but comparable with the climate change mitigation effect due to returning instead of removing straw from field (Poeplau et al. Citation2015; Poeplau et al. Citation2016), which is not negligible.
Uncertainties
A number of factors caused uncertainties to our estimates of the modified crop effects. Firstly, in the SOC scenarios the modified crop was represented by its relative increase of plant biomass (i; ) as predicted by a crop growth model for the Uppsala site in region North (). If instead these relative increases would have been taken to be the same as the relative increases of plant N as predicted by the regional N scenarios () then the increase in plant C input would have been 2% and 3% (for the UPMA-scenarios C2n and C4n, respectively), instead of 14% and 26% as used in this study. Under these conditions, changes in SOC would have decreased, from 26 to 47 kg C ha−1 y−1 () to 7 and 9 kg C ha−1 y−1, in the two scenarios respectively (Tidåker et al. Citation2016). This illustrates that the assessments of plant growth, and the scale at which it is assessed, are important uncertainty factors in predicting SOC changes.
Second, the soil type distribution used for the N and SOC scenarios differed slightly. For instance, for the N scenarios in region North 26% of the spring barley area was on silt loam or lighter soils and 73% on clay loam or heavier soils (). The corresponding fractions for the SOC scenarios were 29% and 69%, respectively (data not shown). To evaluate the potential influence of differences in soil distribution on the simulated effects of the modified crops, we compared the two sub-regions of region North () having quite different soil types, i.e. 78% of the light soils in NorthWest and 81% of the heavy soils in NorthEast. This resulted in regional N leaching of the most modified crop to decrease by 30% in NorthWest and 42% in NorthEast, respectively (data not shown). This indicates that the effect of the differences in soil distribution between the N and SOC scenarios possibly had a minor effect on the estimated modified crop effects (for regions South and Central the fraction of light soils were 96% and 56% in the N scenarios, and 87% and 54% in the SOC scenarios, respectively).
Third, in the N scenarios the changes of UPMA and Pot-N were applied to all crops in the crop sequence (a requirement needed in order to run the SOILNDB model). This action is expected to have influenced the modified barley crop scenarios by underestimating soil mineral N levels at sowing due to enhanced N uptake by the pre-crop. Underestimation of the soil mineral N in turn might have led to underestimation of the reducing effect on N leaching compared to the results if only the spring barley would have been modified. However, a minor evaluation of the possible impact of these underestimations on the results indicated that this effect was small (data not shown).
Fourth, the omission of plant growth assessments might also influence the interpretation of the regional N scenarios. In site scenarios performed for similarly modified crops using a crop growth model (Eckersten et al. manuscript Citation2017) the UPMA change increased the leaf N concentration during the main vegetative growth period, thereby stimulating growth. However, later in the season plant N uptake was increasingly limited due to fertilizer N supply to the soil mineral N pool not being changed, which caused seasonal accumulated plant N uptake to increase only slightly compared with the increase of accumulated growth. Hence, increased UPMA, in the beginning of the season, increased N demand in the growth model. This dynamic response of plant growth to modified N supply was not simulated in the regional N scenarios, and might be one of the reasons for the relatively low response to increased UPMA (N leaching decreased by 10% in region North; ), compared with a scenario using a dynamic crop growth model (for the Uppsala site located in region North N leaching decreased by 30%; Eckersten et al. manuscript Citation2017). However, when both UPMA and Pot-N were increased the relative reductions in N leaching were similar for the regional scenarios () and the site-specific application using the growth model. Another reason of the lower response to UPMA in the regional scenarios, compared with the site scenarios, might be the low proportion of light soils with low fertility in the region (), whereas the soil type of the site was a sandy loam.
Lastly, application of soil and crop models at regional scale requires (theoretically) a large number of simulations using field specific inputs on weather, soil type and management data. In studies with several integrated crop and soil models, including the CoupModel (successor of the SOIL/SOILN models; Jansson Citation2012) simulation results using aggregated inputs of successively larger spatial scales for a region in Germany showed a strong influence of spatial heterogeneity of soil types, especially on N leaching and SOC (cf. Coucheney et al. manuscript Citation2017), whereas spatial aggregation of weather input caused less errors (cf. Zhao et al. Citation2015; Hoffmann et al. Citation2015). These results support our approach of aggregation using ten different soil types, but only two climate stations per region. The variation in weather between years though, can largely influence the effects of the modified crop, especially on SOC, as shown for the Uppsala site by Eckersten et al. (manuscript Citation2017). This variation was considered in our study by making simulations for a twenty year period. However, the inputs for the SOC scenarios were taken from plant growth scenarios at a single site (the Uppsala site in region North) representing a cold climate and a soil with low N availability in comparison with the range covered by the studied regions. For region North with a large fraction of N rich soils () this might have overrated the sensitivity to the UPMA parameter. In contrast, the Uppsala sandy loam was more representative for region South (), whereas the Uppsala climate was cold in comparison with that region, which might have decreased plant N demand and thereby also the sensitivity to UPMA. For region Central the conditions were in between since the Uppsala site might have underestimated both the soil N availability and the plant N demand of that region. In summary this means that the values and line in might be too high respectively too steep for region North, too low respectively too flat for region South, and possibly more adequate for region Central.
Concluding remarks and future work
The results suggest that a spring barley crop with increased N demand might reduce the N leaching on a hectare basis in a range that could meet targets set by BSAP (Baltic Sea action plan; SNV Citation2009) and HELCOM (Citation2011). The relative reduction by the most modified crop was highest in region North and least in region South () partly explained by the differences in soil N balance among the regions (). In contrast, the reduction of N leaching in absolute values (kg N ha−1 y−1) was highest in region South. This was due to the high N leaching () caused by the high runoff from region South, despite the negative soil N balance of spring barley areas of this region (). The relative increase of plant N was highest in region Central () due to the higher soil N balance than in region South and more favorable weather (higher potential yields) than in region North.
Table 6. Soil N balances of the regional scenarios (kg N ha−1 y−1).
A crop with an increased efficiency for taking up soil mineral N would also make a stronger sink for atmospheric carbon dioxide. On a hectare basis this would be most important in region Central and least in region North, whereas on a regional basis the largest sink would occur in region North because of its larger area with spring barley. However, the contribution of the most modified barley to C sequestration in Swedish agricultural soils was small (), indicating that corresponding modifications would also be needed for the other crops to have a significant impact at the national scale.
The marginal effects of increased N uptake efficiency (UPMA parameter) became smaller the more it was increased, both for N leaching and plant N, and similar for all regions (). In contrast, the corresponding marginal effects on SOC content remained unchanged (). This was due to the fact that in the site scenarios which provided the litter input to the SOC scenarios (), the plant growth increased linearly with the UPMA change (in contrast to the plant N), possibly related to the low N availability in the sandy loam of the site.
Concerning calculation uncertainties, using growth estimates from a single site as input to the SOC scenarios might have overestimated the effects in region North and underestimated the effects in region South. For effects on N leaching, the uncertainties in using databases for soil type distributions were assumed to be negligible, and applying parameter changes to all crops in the crop sequence might have caused a small underestimation of the sole spring barley effect. Finally, the omission of the growth assessments might have had a minor influence on the effects on N leaching for the most modified crop (i.e. both UPMA and Pot-N were changed), whereas the relative importance of these two parameters might had been quite different using a growth model for assessing the plant N dynamics. Hence, of all these factors, probably the way crop growth is estimated needs most attention in future work. To improve the link between the model concept and the crop physiological processes, being targets of breeding, the regional N scenarios would need to include a dynamic crop growth model. For the regional SOC scenarios the growth model, providing the estimates on C input to soil, would need to be applied to more sites to cover the main climate-soil combinations of the region concerned.
For evaluating how breeding achievements might be implemented successfully in model scenarios field experiments with the modified crops are needed. For instance, the assumption that changes of the UPMA and Pot-N parameters do not interact with other process parameters of the model, need to be tested. The observations of plant biomass and N content and soil mineral N etc. should then be made on a regular basis throughout the year to provide calibration targets for the model simulations when adjusting the UPMA and Pot-N parameters. These experiments should be made for variable environmental and nutritional conditions. Because of the complicated relation between model complexities and the statistical constraints to the evaluation of model predictability, there are several model concepts which might show a similar range of predictability. Therefore, to assess the uncertainty of the performance of a crop modified by breeding under variable environmental conditions, the field experiments with the modified crop need to be combined with applications and tests of several different models (cf. Rötter et al. Citation2012; Tao et al. Citation2017) under several different cultivation conditions.
Disclosure statement
No potential conflict of interest was reported by the authors.
Notes on contributors
Henrik Eckersten is a professor in cropping systems at the Swedish University of Agricultural Sciences. His main research is on modelling biomass, carbon, nitrogen, water, heat and trace element dynamics in plant and soil, e.g. for assessing impacts of climate.
Martin Bolinder is a research scientist at the Swedish University of Agricultural Sciences. His main research is to assess the influence of agricultural practices on changes in soil organic carbon and nitrogen stocks, soil quality and other environmental indicators for agricultural sustainability.
Holger Johnsson is an associate professor in water quality management at the Swedish University of Agricultural Sciences. Currently, he is mainly working with the assessment of nutrient losses from agricultural land to the water environment including the development and evaluation of model tools applicable for such calculations.
Thomas Kätterer is a professor in ecosystem ecology at the Swedish University of Agricultural Sciences. His research focus is on carbon cycling in terrestrial ecosystems and how this is affected by land use and management.
Kristina Mårtensson is a research engineer at the Swedish University of Agricultural Sciences. Currently, she is mainly working with the assessment of nutrient losses from agricultural land to the water environment including the development and evaluation of model tools applicable for such calculations.
Dennis Collentine is a visiting associate professor at the Swedish University of Agricultural Sciences. He is an economist working primarily on multi-disciplinary research with a focus on agri-environmental policy analysis.
Pernilla Tidåker is an agronomist and researcher at RISE - Research Institutes of Sweden. Her work focuses on environmental assessment of food and crop production systems using e.g. life cycle assessment.
Håkan Marstorp is an agronomist and associate professor in soil science at the Swedish University of Agricultural Sciences. His current research interests are mainly about productivity and sustainability of farming systems especially in relation to soil aspects both in temperate and tropical environments.
Additional information
Funding
References
- Andrén O, Kätterer T. 1997. ICBM – the introductory carbon balance model for exploration of soil carbon balances. Ecol Appl. 7:1226–1236. doi: 10.1890/1051-0761(1997)007[1226:ITICBM]2.0.CO;2
- Andrén O, Kätterer T, Karlssson T. 2004. ICBM regional model for estimations of dynamics of agricultural soil carbon pools. Nutr Cycling Agroecosyst. 70:231–239. doi: 10.1023/B:FRES.0000048471.59164.ff
- Andrén O, Kätterer T, Karlsson T, Eriksson J. 2008. Soil C balances in Swedish agricultural soils 1990–2004, with preliminary projections. Nutr Cycling Agroecosyst. 81:129–144. doi: 10.1007/s10705-008-9177-z
- Beatty PH, Carroll RT, Shrawat AK, Guevara D, Good AG. 2013. Physiological analysis of nitrogen-efficient rice overexpressing alanine aminotransferase under different N regimes. Botany-Botanique. 91(12):866–883. doi: 10.1139/cjb-2013-0171
- Blombäck K, Duus Børgesen C, Eckersten H, Giełczewski M, Piniewski M, Sundin S, Tattari S, Väisänen S, editors. 2013. Productive agriculture adapted to reduced nutrient losses in future climate - Model and stakeholder based scenarios of Baltic Sea catchments. Available from: http://www.balticcompass.org/PDF/Reports/WP5-Productive-agriculture-adapted-to-reducednutrient-losses-in-future-climate.pdf
- Blombäck K, Eckersten H, Lewan E, Aronsson H. 2003. Simulations of soil carbon and nitrogen dynamics during seven years in a catch crop experiment. Agr Syst. 76:95–114. doi: 10.1016/S0308-521X(02)00030-6
- Blombäck K, Johnsson H, Lindsjö A, Mårtensson K, Persson K, Schmieder F. 2011a. Läckage av näringsämnen från svensk åkermark för år 2009 beräknat med PLC5-metodik - Beräkningar av normalläckage av kväve och fosfor för 2009. SMED Rapport Nr 57 2011.
- Blombäck K, Johnsson H, Markensten H, Mårtensson K, Orback C, Persson K, Lindsjö A. 2011b. Läckage av näringsämnen från svensk åkermark för år 2011 beräknat med PLC5-metodik - Beräkningar av normalläckage av kväve och fosfor för 2011. Rapport till Jordbruksverket (99 pages).
- Bolinder MA, Fortin JG, Anctil F, Andrén O, Kätterer T, de Jong R, Parent LE. 2013. Spatial and temporal variability of soil biological activity in the Province of Québec, Canada (45–58 °N, 1960–2009)—calculations based on climate records. Clim Change. 117:739–755. doi: 10.1007/s10584-012-0602-6
- Borgen SK, Grønlund A, Andrén O, Kätterer T, Tveito OE, Bakken L, Paustian K. 2012. CO2 emissions from cropland in Norway estimated by IPCC default and Tier 2 methods. Greenhouse Gas Meas Manage. 1:1–17. doi:10.1080/20430779.2012.672306.
- Brandt M, Ejhed H, Rapp L. 2008. Näringsbelastningen på Östersjön och Västerhavet 2006. Rapport 5815, 95pp, Naturvårdsverket, Bromma.
- Coucheney E, Eckersten H, Hoffmann H, Jansson P-E, Gaiser T, Ewert F, Lewan E. manuscript 2017. Spatial aggregation for crop modeling at regional scales: the effects of soil variability. Uppsala: Swedish University of Agricultural Sciences.
- Downing TE, Barrow EM, Brooks RJ, Butterfield RE, Carter TR, Hulme M, Olesen JE, Porter JR, Schellberg J, Semenov MA, Vinther FP, Wheeler TR, Wolf J. 2000. Quantification of uncertainty in climate change impact assessment. In: Downing TE, Harrison PA, Butterfield RE, Lonsdale KG, editors. Climate change, climatic variability and agriculture in Europe. Oxford (UK): Environm Change Unit. Univ of Oxford; p. 415–434.
- Eckersten H, Beier C. 1998. Comparison of N and C dynamics in two Norway spruce stands using a process oriented simulation model. Environ Pollut. 102:395–401. doi: 10.1016/S0269-7491(98)80059-6
- Eckersten H, Blombäck K, Kätterer T, Nyman P. 2001. Modelling C, N, water and heat dynamics in winter wheat under climate change in southern Sweden. Agric Ecosyst Environ. 86:221–235. doi: 10.1016/S0167-8809(00)00284-X
- Eckersten H, Jansson PE. 1991. Modelling water flow, nitrogen uptake and production for wheat. Fert Res. 27:313–329. doi: 10.1007/BF01051137
- Eckersten H, Marstorp H, Collentine D, Johnsson H, Tidåker P, Kätterer T. manuscript 2017. Ecosystem C and N dynamics of genetically modified spring barley with increased nitrogen uptake efficiency - a simulation case study. Uppsala: Swedish University of Agricultural Sciences.
- Eckersten H, Torssell B, Kornher A, Boström U. 2007. Modelling biomass, water and nitrogen in grass ley: Estimation of N uptake parameters. Eur J Agron. 27:89–101. doi: 10.1016/j.eja.2007.02.003
- Fortin JG, Bolinder MA, Anctil F, Kätterer T, Andrén O, Parent LE. 2011. Effects of climatic data low-pass filtering on the ICBM temperature- and moisture-based soil biological activity factors in a cool and humid temperate climate. Ecol Modell. 222:3050–3060. doi: 10.1016/j.ecolmodel.2011.06.011
- Foulkes MJ, Hawkesford MJ, Barraclough P, Holdsworth BMJ, Kerr S, Kightley S, Shewry PR. 2009. Identifying traits to improve the nitrogen economy of wheat: recent advances and future prospects. Field Crops Res. 114(3):329–342. doi: 10.1016/j.fcr.2009.09.005
- Good AG, Johnson SJ, De Pauw M, Carroll RT, Savidov N, Vidmar J, Lu Z, Taylor G, Stroeher V. 2007. Engineering nitrogen use efficiency with alanine aminotransferase. Can J Bot. 85:252–262. doi: 10.1139/B07-019
- Hay RKM, Porter JR. 2006. The physiology of crop yield. 2nd ed. Oxford (UK): Blackwell Publishing. ISBN-13: 978-14051-0859-1. p. 314.
- HELCOM. 2011. Activities 2011. Overview. Baltic Sea Environment Proceedings No. 132. Helsinki Commission; p. 50. Available from: http://www.helcom.fi/stc/files/Publications/Proceedings/BSEP132%20FINAL.pdf
- Hoffmann H, Zhao G, Van Bussel LGJ, Enders A, Specka X, Sosa C, Yeluripati J, Tao F, Constantin C, Raynal H, et al. 2015. Variability of effects of spatial climate data aggregation on regional yield simulation by crop models. Clim Res. 65:53–69. doi: 10.3354/cr01326
- Jansson PE. 2012. Transactions of the ASABE. 55(4):1337–1346. Available from: http://rymd.lwr.kth.se/Vara%20Datorprogram/CoupModel/
- Jansson PE, Halldin S. 1980. Model for annual water and energy flow in a layered soil. In: S Halldin, editor. Comparison of forest water and energy exchange models. Copenhagen: International Society for Ecological Modelling; p. 145–163.
- Jansson PE, Persson T, Kätterer T. 1999. Nitrogen processes in arable and forest soils in the Nordic countries – Field scale modelling and experiments. Nordic Councils of ministers. TemaNord 1999:560. 203 pp.
- Johnsson H, Bergström L, Jansson PE. 1987. Simulated nitrogen dynamics and losses in a layered agricultural soil. Agric Ecosyst Environ. 18:333–356. doi: 10.1016/0167-8809(87)90099-5
- Johnsson H, Larsson M, Lindsjö A, Mårtensson K, Persson K, Torstensson G. 2008. Läckage av näringsämnen från svensk åkermark - Beräkningar av normalläckage av kväve och fosfor för 1995 och 2005. Naturvårdsverket rapport nr 5823. 152 pp.
- Johnsson H, Larsson MH, Mårtenssson K, Hoffmann M. 2002. SOILNDB: a decision support tool for assessing nitrogen leaching losses from arable land. Environ Software Modell. 17:505–517. doi: 10.1016/S1364-8152(02)00013-0
- Jones JW, Hoogenboom G, Porter CH, Boote KJ, Batchelor WD, Hunt LA, Wilkens PW, Singh U, Gijsman AJ, Ritchie JT. 2003. The DSSAT cropping system model. Eur J Agron. 18:235–265. doi: 10.1016/S1161-0301(02)00107-7
- Kätterer T, Andrén O. 2001. The ICBM family of analytically solved models of soil carbon, nitrogen and microbial biomass dynamics – descriptions and application examples. Ecol Modell. 136:191–207. doi: 10.1016/S0304-3800(00)00420-8
- Kätterer T, Bolinder MA, Berglund K, Kirchmann H. 2012. Strategies for carbon sequestration in agricultural soils in northern Europe. Acta Agr Scand Sect A. 62:181–198.
- Keating BA, Carberry PS, Hammer GL, Probert ME, Robertson MJ, Holzworth D, Huth NI, Hargreaves JNG, Meinke H, Hochman Z, et al. 2003. An overview of APSIM, a model designed for farming systems simulation. Eur J Agron. 18:267–288. doi: 10.1016/S1161-0301(02)00108-9
- Kyllmar K, Mårtensson K, Johnsson H. 2005. Model-based coefficient method for calculation of N leaching from agricultural fields applied to small catchments and the effect of leaching reducing measures. J Hydrol. 304:343–354. doi: 10.1016/j.jhydrol.2004.07.038
- Larsson M, Kyllmar K, Jonasson L, Johnsson H. 2005. Estimating reduction of nitrogen leaching from arable land and the related costs. Ambio. 34:538–543. doi: 10.1579/0044-7447-34.7.538
- Lokupitiya E, Paustian K, Easter M, Williams S, Andrén O, Kätterer T. 2010. Carbon balance in US croplands during the last two decades of the twentieth century. Biogeochem. 107:207–225. doi: 10.1007/s10533-010-9546-y
- McAllister CH, Beatty PH, Good AG. 2012. Engineering nitrogen use efficient crop plants: the current status. Plant Biotechnol J. 10(9):1011–1025. doi: 10.1111/j.1467-7652.2012.00700.x
- McAllister CH, Good AG. 2015. Alanine Aminotransferase variants conferring diverse NUE phenotypes in Arabidopsis thaliana. PLoS ONE. 10(4):e0121830. doi: 10.1371/journal.pone.0121830
- Montesino-San Martin M, Olesen JE, Porter JR. 2014. A genotype, environment and management (GxExM) analysis of adaptation in winter wheat to climate change in Denmark. Agric For Meteorol. 187:1–13. doi: 10.1016/j.agrformet.2013.11.009
- Poeplau C, Aronsson H, Myrbeck Å, Kätterer T. 2015. Effect of perennial ryegrass cover crop on soil organic carbon stocks in southern Sweden. Geoderma Reg. 4:126–133. doi: 10.1016/j.geodrs.2015.01.004
- Poeplau C, Bolinder MA, Eriksson J, Lundblad M, Kätterer T. 2015. Positive trends in organic carbon storage of Swedish agricultural soils due to unexpected socio-economic drivers. Biogeosci. 12:3241–3251. doi: 10.5194/bg-12-3241-2015
- Poeplau C, Kätterer T, Bolinder MA, Börjesson G, Berti A, Lugato E. 2015. Low stabilization of aboveground crop residue carbon in sandy soils of Swedish long-term experiments. Geoderma. 237–238:246–255. doi: 10.1016/j.geoderma.2014.09.010
- Poeplau C, Reiter L, Berti A, Kätterer T. 2016. Qualitative and quantitative response of soil organic carbon to 40 years of crop residue incorporation under contrasting nitrogen fertilisation regimes. Soil Res. doi:10.1071/SR15377.
- Rötter RP, Palosuo T, Kersebaum KC, Angulo C, Bindi M, Ewert F, Ferris R, Hlavinka P, Moriondo M, Nendel C, et al. 2012. Simulation of spring barley yield in different climatic zones of Northern and Central Europe: a comparison of nine crop models. Field Crops Res. 133:23–36. doi: 10.1016/j.fcr.2012.03.016
- Rötter RP, Tao F, Höhn JG, Pal T. 2015. Use of crop simulation modelling to aid ideotype design of future cereal cultivars. J Exp Bot. doi:10.1093/jxb/erv098.
- SCB. 2012. Normskördar för skördeområden, län och riket 2011. SCB, Statistiska meddelanden, J15 SM 1201, Stockholm (Statistics Sweden; SCB). Available from: http://statistik.sjv.se.
- Skovby Rasmussen I, Bodin Dressbøll D, Thorup-Kristensen K. 2015. Winter wheat cultivars and nitrogen (N) fertilization – Effects on root growth, N uptake efficiency and N use efficiency. Eur J Agron. 68:38–49. doi: 10.1016/j.eja.2015.04.003
- SLU. 2016. MistraBioTech research project. Available from: http://www.slu.se/centrumbildningar-och-projekt/mistra-biotech/?id=46489.
- SMHI. 2017. Length of vegetation period. Available from: https://www.smhi.se/kunskapsbanken/klimat/vegetationsperiod-1.6270.
- SNV. 2009. Swedish Environmental Protection Agency. Sweden’s commitment under the Baltic Sea Action Plan. Proposal for a national action plan. Report 6318. 153 pp. Available from: http://www.naturvardsverket.se/Documents/publikationer/978-91-620-6318-4.pdf.
- SNV. 2015. Swedish Environmental Protection Agency. National Inventory Report Sweden 2015 - Greenhouse Gas Emission Inventories 1990-2013. (manuscript; ca 500 pages).
- Tao F, Rötter RP, Palosuo T, Díaz-Ambrona CGH, Mínguez MI, Semenov M, Kersebaum KC, Nendel C, Cammarano D, Hoffmann H, et al. 2017. Designing future barley ideotypes using a crop model ensemble. Eur J Agron. 82:144–162. doi: 10.1016/j.eja.2016.10.012
- Tidåker P, Bergkvist G, Bolinder M, Eckersten H, Johnsson H, Kätterer T, Weih M. 2016. Estimating the environmental footprint of barley with improved nitrogen uptake efficiency - a Swedish scenario study. Eur J Agron. 80:45–54. doi: 10.1016/j.eja.2016.06.013
- Zhao G, Hoffmann H, Van Bussel LGJ, Enders A, Specka X, Sosa C, Yeluripati J, Tao FL, Constantin J, Teixera E, et al. 2015. Effect of weather data aggregation on regional crop simulation for different crops, production conditions, and response variables. Clim Res. 65:141–157. doi: 10.3354/cr01301