ABSTRACT
To reveal the regulatory mechanisms underlying the productivity of long-term continuous cropping of processing tomato, a multi-year study was carried out to understand the effects of long-term continuous cropping on the community structures of the root zone microbes. Soil samples collected from continuous cropping of processing tomato after 3, 5 and 7 years were used for this study. Results showed that soil microbial biomass C (SMBC), N (SMBN) and microbial quotient (qMB) significantly decreased with longer cropping. After seven years of continuous cropping, the SMBC and SMBN contents, and qMB respectively significantly decreased by 52.3%, 78.8% and 48.2% (p < 0.01). In contrast, soil microbial biomass P (SMBP) increased during the first three years of continuous cropping, peaking to up to 1.6 times higher than that of the control, which also declined in the succeeding years. Continuous cropping significantly increased the PLFA of fungi, whereas the opposite trend was observed for bacteria, total PLFAs biomass, and the ratios of bacteria and fungi. The PLFA of actinomycetes have no change throughout. This suggests that fungi were better adapted to nutrient poor conditions than bacteria. Microbial diversity indices reached the highest after three years of continuous cropping, with the lowest observed after seven years. This indicated that microbial community diversity and uniformity decreased with increasing number of planting years. Furthermore, our results revealed that the microbial community structure in processing tomato soils changed with extended cropping, resulting to a decline in soil microbial biomass, which may be both a cause and a reflection of the poor soil quality.
Introduction
Problems in succession cropping usually take place in croplands that have been cultivating the same crop for so long, passively impacting both crop yield and quality (Nayyar et al. Citation2009; Gentry et al. Citation2013). Several crops abide to the yield amercement caused by degradation in soil quality or sickness, such as corn (Zea mays L.) (Gentry et al. Citation2013), cotton (Gossypium hirsutum L.) (Zhang et al. Citation2013), pea (Pisum sativum L.) (Lupwayi et al. Citation2012) and cucumber (Cucumis sativus L.) (Zhou et al. Citation2012).
Miscellaneous factors have been viewed as to stimulate soil sickness, including corruption of soil physico-chemical properties (Xiao et al. Citation2013), accumulation of autotoxins (Jiang et al. Citation2015), weakening of soil enzymatic activities (Xiong et al. Citation2015), and an amplitude of the soil-borne pathogens (Huang et al. Citation2013). Recently, studies have primarily speculated that the change in soil microflora would also result to problems in continuous cropping associated with long-term plantations (Gentry et al. Citation2013; Zhang et al. Citation2013; Xiong et al. Citation2015). However, the underlying mechanisms causing soil sickness are complex, and have been associated with numerous agricultural practices, soil characteristics and other limitations.
Processing tomato (Lycopersicon esculentum Mill.), a variety of industry grade tomato, is now grown throughout the region of Xinjiang, China. Its industrial production and continuous cropping are common, and as a result, problems with mono-cropping have now been augmented by its sustainable and high yields. Soil microbial biomass, community structure, and diversity have all played essential roles in the evolution of the soil’s quality (Zhou et al. Citation2014). However, much less research has focused on the analysis of barriers to the continuous cropping of processing tomato, specifically looking at the effects of soil microbial biomass, microbial quotient (qMB) (Rutigliano et al. Citation2014), soil microbial communities and the link between these variables and their effects on soil productivity. Previous studies on cucumber (Cucumis sativus L.) (Zhou et al. Citation2014), cotton (Gossypium hirsutum L.) (Zhang et al. Citation2013) and similar cash-crops have all reported that soil microbial carbon (SMBC), soil microbial biomass nitrogen (SMBN), soil microbial phosphorus (SMBP) and qMB are negatively influenced by continuous cropping. Thus, investigations on soil microbial biomass, qMB and microbial community structure under continuous cropping of processing tomato will greatly contribute to the further understanding of problems of continuous cropping.
At present, many methods are primarily used to study soil microbial community diversity, such as dilution-plate method (Li et al. Citation2014), denaturing gel gradient electrophoresis (PCR-DGGE) (Zhang et al. Citation2013), and high-throughput sequencing (Xiong et al. Citation2015), but all have some limitations. First, many soil microbes are nonculturable, while culturable microorganisms only account for 1%–10% of soil microbiota (Mccarthy and Murray Citation1996). Second, the commonly used PCR-DGGE method fails to separate out large fragments (>500 bp), and a single band in the spectrum does not necessarily represent a single bacterial strain (Myers et al. Citation1985; Ferris and Ward Citation1997). Lastly, high-throughput sequencing technology was very costly before 2015, making it challenging for our team to process multiple samples. Alternatively, analysis of phospholipid fatty acids (PLFAs) to investigate soil microbial community has been proven to be faster, cheaper, and more reliable to adequately detect sudden changes in soil microflora (Frostegård et al. Citation1993; Ge et al. Citation2017). PLFAs have been used as biomarkers for microbial groups (Frostegård et al. Citation1993) and reflect the general composition of soil microbial communities (Ge et al. Citation2017). Additionally, diverse PLFAs biomarkers can effectively discern different major microbial groups such as fungi and bacteria (Hao et al. Citation2008), and have been efficiently used to probe microbial community composition and biomass (Mahmoudi et al. Citation2015; Zhang et al. Citation2015).
In this study, we used PLFAs characterization to conduct a quantitative analysis of the microbial community structure in soils where processing tomato are grown and have been under continuous cropping for 3, 5, and 7 years in Xinjiang, China. We then measured changes in the content of SMBC, SMBN, SMBP and qMB as the continuous cropping years were extended to reveal the variations in patterns of soil microbial populations during the long-term growth of processing tomatoes. Specifically, the objectives of the our study were (i) to confirm the types of PLFAs biomarkers that could distinguish changes in the community after long-term continuous cropping; (ii) to look through the transformation occurring in the soil microflora along with continuous cropping years; (iii) to probe into the underlying correlation between soil physio-chemical properties and soil microbial biomass, and (iv) to provide a better comprehension of the influences of soil microbial communities on the growth of processing tomatoes under continuous cropping system.
Materials and methods
Study site
The experiment was carried out at an experimental field (85°59′50″N′44°18′58″E) for cropping of processing tomatoes in the Agricultural College, Shihezi University in Xinjiang. The experiment site was located at an altitude of 437–450 m. The average sun exposure all year round was from 2721–2818 h, while the frost-free period was 168–171 days, and the average annual rainfall at the site was 208 mm with an evaporation capacity of about 1660 mm. The average annual temperature was 7–8° C. The site experienced a typical continental climate, with sufficient sunshine and low rainfall, a large temperature difference between day and night, and was suitable for irrigated agriculture. The soil type was calcaric fluvisol with a sandy loam soil texture.
Experimental design
In April 2007, an open area of land that had no-tillage for more than 3 years was selected for the study, at 12 m long and 5 m wide and a total land area of 60 m2. This open area was then divided into 3 plots of 20 m2 as 3 replicates. Processing tomato, variety Ligeer 87-5, was used as the model species and planted at a density of 48,000 individual plants hm−2. Cultivation with drip irrigation under mulch was used with two lines under each mulch, direct sowing, wide narrow row of 80 + 40 cm, and with row spacing 35 cm. The plants were irrigated every seven or eight days and reached a total of 10 times per growth duration. The total irrigation was about 5,250 m3 hm−2, with a total of nitrogen (N) 207 kg hm−2, phosphorus (P2O5) 84.86 kg hm−2, potassium (K2O) 56.18·kg hm−2 used for the entire duration of the experiment.
We also tested the effect of time or duration of cropping on the microbial communities by investigating the changes after certain years. Specifically, soil samples were processed after continuous cropping of 1, 2, 3, 4, 5, 6 and 7 years, and was conducted from October 2007 to 2014, respectively. Soil samples that were collected within a year after planting was treated as the control group (CK). Root-zone samples were collected to investigate the changes in soil physiochemical properties and soil microbial biomass, from CK (2007) and from continuous cropping after 3 years (2010), 5 years (2012), and 7 years (2014).
Collection and pretreatment of experimental soils
After harvesting the processing tomatoes in early October of 2007, 2010, 2012 and 2014, surface soil samples from 0–20 cm deep were collected at the root zone using a 2 cm-inner diameter soil auger. Plant residues, stones and other debris were then removed from the samples. One part of the soil was immediately sifted through a 1 mm sift after being collected and stored in the refrigerator at 4°C to prevent further decay, and were subsequently used to determine the SMBC, SMBN and SMBP contents. Another part was naturally dried, and separated first by 100 mesh nylon sift and then by 20 mesh nylon sift, and finally sealed in bags to measure the physical and chemical properties of the soil (Guan Citation1986), results of which are listed in .
Table 1. Basic the physical and chemical properties of the soil at the experimental site (0–20 cm depth).
We selected 15 mature processing tomato plants with uniform growth in each plot from where we took soil samples under them in 2007, 2010, 2012 and 2014 using the methods described in the previous section and carried out the pretreatment. After mixing them thoroughly, samples were taken to the laboratory in sterile plastic bags before being sifted with 2 mm earth sieve and stored in the refrigerator at –80°C for analysis of PLFAs content.
Testing items and methods
Measurement of soil microbial biomass and calculation of qMB
SMBC and SMBN were measured using the chloroform sterilizaition-K2SO4 extraction method and SMBP using the soil fumigation extraction method (Brookes et al. Citation1982; Jenkinson Citation1988). The formulas are as follows.
To calculate microbial C:(1) To calculate microbial N:
(2) Where EC is the difference value between the soil organic carbon measured by fumigation and non-fumigation methods and KEC is the conversion coefficient with a value of 0.38; EN is the difference value between the soil total nitrogen measured by fumigation and non-fumigation methods and KEN is the conversion coefficient with a value of 0.45.
To calculate microbial P:(3) Where EP is the difference value between the phosphorus content measured by soil fumigation extraction method and soil non-fumigation extraction method and KEN is the conversion coefficient with a value of 0.45.
To calculate soil microbial entropy (qMB) (Anderson and Domsch Citation1978):(4) Where qMB is the soil microbial carbon entropy, SMBC is the soil microbial biomass carbon (g kg−1) and SOC is the soil organic carbon (g kg−1).
(5)
Where 1.724 represents the average conversion coefficient to translate SOC into SOM (Guan Citation1986).
Measurement of soil PLFA
Soil PLFA was measured using the Bligh-Dyer method. The interior label was esterified C19:0, measured by Agilent GC-MS (7890A-5975C). The GC-MS analytical conditions are as follows: chromatographic column is a quartz capillary of hp5-MS (30 m × 0.25 mm × 0.25 μm), carrier gas He (0.9 ml·min−1), injection port temperature 250°C, split ratio 10:1, ion source temperature 230°C and mass spectrometry full scan range 30–600 m z−1. The temperature of the column oven was 70°C at the start, and remained so for 5 min; then it reached 190°C at the rate of 20°C min−1 and was held there for 3 min. After that it reached 200°C at the rate of 5°C min−1and was held there for a further 3 min; finally it reached 280°C at the rate of 10°C min−1 and remained there for 5 min. The identification and quantitative analysis of fatty acids referred to peak area and interior label curve method by BAME (Bacterial Acid MethylEsters) Mix and Supelcoe 37 Component FAME Mix (Sigma Company), respectively, with PLFA content expressed in nmol g−1.
The characterization of specific microorganisms is marked by specific biomarkers. Partial specific biomarkers for fatty acids are summarized according to the literature as listed in .
Table 2. PLFA characterizing microbes.
Analysis of microbial community diversity indices
The microbial community diversity of soil under continuous cropping for processing tomato was analyzed using the Shannon-Wiener diversity index, Brillouin diversity index, Simpson dominance index and Pielou evenness index (Garland and Mills Citation1991). The indices are:(6)
(7)
(8)
(9)
Where S is the total number of types of fatty acids in the community, ni is the number of ith fatty acid as in Pi = ni/N, and N is the total number of fatty acids in the experiment.
Statistical analyses
One-way analysis of variance (ANOVA) and Duncan’s multiple range tests (p < 0.05) were performed in SPSS 19.0 and Excel 2010 to test for differences in soil microbial biomass, soil physicochemical properties, and soil microbial PLFAs, among the different continuous monoculture processing tomato. Correlations between all measurable variables were estimated using Pearson’s r with p < 0.05 significance threshold also in SPSS 19.0. The PLFA data were also plotted using principal component analysis (PCA) to inspect patterns of species associations among each experimental field plot using SPSS 16.0 for Windows.
Results
Soil microbial biomass
The ratio of soil microbial biomass and corresponding soil total nutrients reflects the conversion efficiency of nutrients to biomass as well as nutrient loss (Sparling Citation1997). The content of SMBC, SMBN and qMB significantly declined as the years of continuous cropping of processing tomato were extended, while the ratio of SMBC/SMBN showed an increasing trend (). Compared with CK, after continuous cropping of 7 years, SMBC, SMBN and qMB decreased by 52.3%, 78.8% and 48.2% (P < 0.01), respectively. Soils after 7 years continuous cropping had SMBC/SMBN that was 1.67 times higher than that of the CK (P < 0.01). Soil microbial biomass P (SMBP) also increased during the first three years of continuous cropping, peaking up to 1.6 times higher than the control and then decreasing drastically (P < 0.01).
Table 3. Effect of continuous cropping years of processing tomato on microbial C, N, P and qMB, microbial C/N in the surface soil (0–20 cm depth).
Soil microbial community structure
PLFA markers on soil microorganisms at mature stage
During the mature stage of the processing tomatoes, the soils from different years of continuous cropping were rich in PLFA markers. A total of 13 PLFAs were detected in all soil samples (). Among these, 11 PLFA markers were bacterial in origin with 5 G+ bacteria, namely, i14:0, i15:0, i16:0, a17:0, and i17:0, and 3 G− bacteria including 16:1ω11c; 16:1ω7 and 16:1ω9c. The rest were from general bacteria, such as 15:0, 16:0 and 20:0. Additionally, there was 1 actinomycete (10Me18:0) and 1 fungal PLFA (18:1ω9c).
Figure 1. Bar graphs showing the different PLFA biomarkers concentrations (nmol g−1) detected in the different soils exposed to different number of years of continuous processing. Error bars represent standard deviation, SD (n = 3).
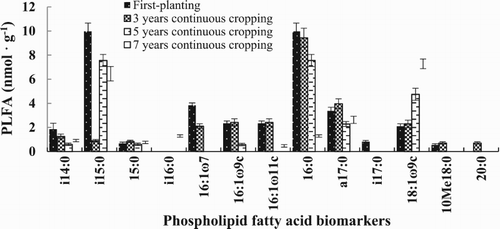
As shown in , during the maturation stage of the processing tomato, PLFAs differed significantly among the soils with different years of continuous cropping. The observed PLFAs varied strongly with some soils containing only one while others to as much as 4 types associated with different microorganisms, suggesting that continuous cropping of processing tomato could have changed the composition of the soil microbial community. For example, in CK, the predominant PLFA markers were i15:0 (one of the 5 G+ bacterial biomarkers) and 16:0 (one of 3 general bacterial biomarkers) while in soil under continuous cropping of 3 years and of 5 years, it was 16:0. In the soil under continuous cropping of 7 years, 18:1ω9c which is the only fungal biomarker dominated the community followed by i15:0. Meanwhile, the general bacteria (16:0), G+ bacteria (i15:0) and fungus (18:1ω9c) consistently dominated the soil samples regardless of the number of years of cropping.
Different PLFA contents of soil microbial groups
suggests significant difference in the effects of processing tomato continuous cropping on the different microbial groups in the soil as seen from the variations in the PLFAs. Specifically, bacterial PLFAs decreased significantly as the cropping was extended, whereas, fungal PLFAs increased dramatically. Fungal PLFAs under continuous cropping of 3, 5 and 7 years increased by 8.7%, 55.8% and 60.2%, respectively, compared with that of CK. Notably, actinomycetic PLFAs first increased drastically followed by a sudden decrease. Overall, the total PLFAs of different microbial groups in processing tomato soils displayed a declining trend as the years of continuous cropping extended (). Specifically, the total soil microorganism PLFAs under continuous cropping of 3, 5 and 7 years decreased by 28.5%, 33.5% and 50.3%, respectively, compared with that of CK. Thus, the effects of years of continuous cropping on the total PLFAs in the soil of the processing tomato varied significantly.
Table 4. Effect of the different continuous cropping years of processing tomato on PLFA contents of microbial groups in the surface soil (0–20 cm depth).
Using the definition by Frostegård et al. (Citation1993), we calculated for the PLFAs and the pairwised ratios of gram positive and negative bacteria, actinomycetes and fungi. The results are given in , and suggest that under different years of continuous cropping, soil microbial groups were mainly bacteria, actinomycetes and fungi, and no protozoa were detected. These microbial PLFAs in the studied soils, when ranked from high to low, were bacteria > fungi > actinomycetes. Bacterial PLFAs accounted for around 69%–93% of total microbial PLFAs; fungi 5%–28%; actinomycetes 2%–26%.
The ratio of PLFAs contents among major soil microorganisms
Results also showed that as the years of continuous cropping were extended, patterns in the gram positive and negative bacterial PLFAs in the rhizosphere of the matured tomatoes exhibited opposite trends (). Specifically, G+ PLFAs first decreased followed by an increase, while the G− PLFAs showed the opposite. shows how the ratio of gram positive and gram negative bacteria (G+/G−) PLFA content changed during the maturation stage of processing tomato, characterized first by a decrease and then an increase (). Under continuous cropping of 3 years, G+/ G− reached its minimum value of 2.53, which was 63% lower than that of CK. After 7 years, it reached its maximum value of 22.38, 3.3 times higher than that of the CK.
Figure 2. Summary of the ratios of Gram-positive to Gram-negative bacteria, and the bacterial to fungal biomass. Different lowercase letters indicate significant differences among different continuous cropping years at P < 0.05. Error bars represent SD (n = 3).
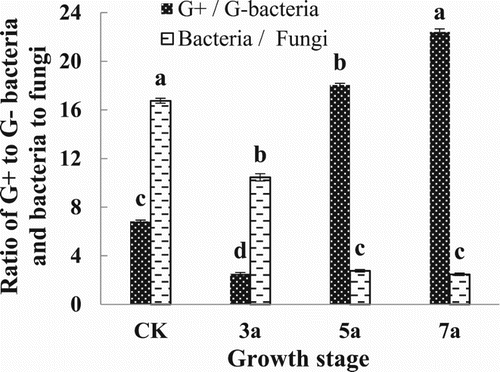
The PLFAs ratio of bacteria/fungi is an important indicator that represents the buffer capacity of the soil ecosystem (Bossio et al. Citation2005). In this study, continuous cropping of processing tomato led to a significant decrease in the bacteria/fungi PLFAs ratio (). During the maturation stage however, no significant difference in the ratio was observed between those from continuous cropping after 5 and 7 years, which both decreased by 92.7% and 93.5%, respectively, compared with that of CK. Thus, this could indicate that continuous cropping of processing tomato could have led to the weakening of the buffering capacity of the soil ecosystem to cope with continuous cropping stress.
PCA analysis of soil microbial PLFAs
shows the results of the PCA analysis, where the first principal component (PC1) was composed of soil samples from CK, those from after 3 and 5 years of continuous cropping, which explained 71.5% of the variation of the clustering. PC2 on the other hand, contributed 22.1% of the variation and was associated with the soil samples collected after 7 years continuous cropping. Thus, PC1 and PC2 separated the PLFA of the soil microbial community under continuous cropping. Further analysis revealed that the projection points of CK, continuous cropping of 3, 5 and of 7 years were dispersed in the PCA biplot, showing that there was a small difference between the effects of continuous cropping on the composition of the soil microbial community during the maturation stage of processing tomato. Thus, continuous cropping of processing tomato dramatically changed the structure of the soil microbial community.
Figure 3. PCA showing variations in soil microbial PLFAs atlas with different continuous cropping years of processing tomatoes. PC 1 explains 71.473% of the variation, PC 2 another 22.055%.
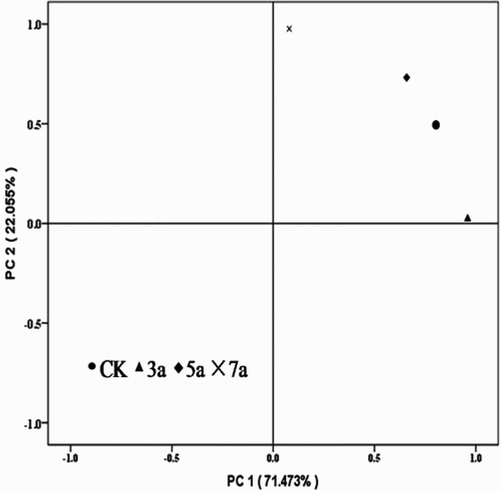
Varimax rotation (orthogonal) was used to further simplify the factor matrix structure, which further separated the load values of the different variables and better explained the relationships between the variables and principal factors (). The variable with the largest load value was i14:0 at 0.972, followed by those with load values >0.900 including i15:0, 16:1ω11c, a17:0, 18:1ω9c. Those with load values from 0.80 to 0.89 included 16:0, 16:1ω7, 16:1ω9c, i17:0. These 9 variables were best correlated and contributing the most to PC1, making PC1 their representative factor. For PC2, the variable with the largest load value was 10Me18:0 (0.968), followed by 20:0 (0.908), i16:0 and 15:0 (load value >0.820). Thus, these 4 variables were used as the representative factors of PC2. The PLFAs i14:0, i15:0 and a17:0 marked G+ bacteria, while 18:1ω9c was a feature marker of fungi PLFAs, and 10Me18:0 was specific for actinomycetes. In summary, G+ soil bacteria were influenced the most by processing tomato continuous cropping, followed by fungi and then by actinomycetes.
Figure 4. PCA showing loading values for individual PLFAs in soil with different continuous cropping years of processing tomatoes. PC 1 explains 71.473% of the variation, PC 2 another 22.055%. a-i14:0, y-i15:0, f-16:0, l-a17:0, o-i17:0, g-16:1ω7, h-16:1ω9c, i-16:1ω11c, and m-18:1ω9c were belong to PC 1, while j-i16:0, d-15:0, n-10Me18:0, p-20:0 were belong to PC 2. Other PLFAs biomarkers were c-a14:0, b-14:0, k-17:0.
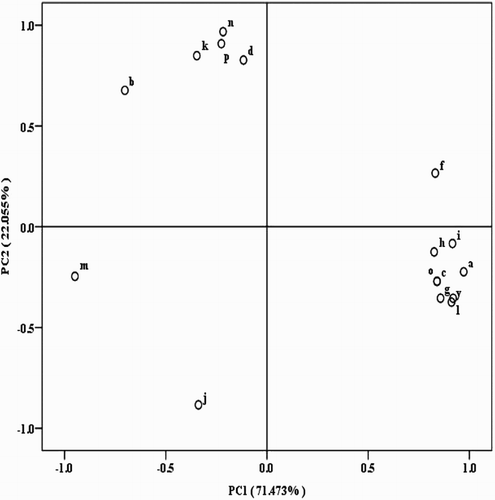
Soil microbial community diversities
Diversity indices are considered good evaluators of microbial community diversity in different soils (Klose et al. Citation2006). shows that the different indices (i.e. Shannon-Wiener, Brillouin and Simpson indices) first increased followed by a decrease as continuous cropping got extended. Soil microorganisms in particular displayed highest indices after continuous cropping of 3 years, and the least after 7 years. This suggests that continuous cropping can at first increase the number microorganism species and community richness. After 3 years, however, microbial biodiversity started to decline, as well as the total number of microorganisms. The Pielou evenness index for example, which represents the relative density of biological species in the community where higher evenness indicates higher the relative density (Bossio et al. Citation2005), reached its peak value under continuous cropping of 3 years and its minimum value at 7 years (). This observation was similar to the other indices and with no significant differences among different treatments.
Table 5. Effect of the different continuous cropping years of processing tomato on diversity indices of microbial community at matutity.
Correlation between soil physicochemical properties and soil microbial biomass
Correlation analysis () showed no significant correlation between pH and soil microbial biomass; significant positive correlation among soil nutrient, soil microbial biomass and qMB, while there was a highly significant negative correlation between the soil nutrient and SMBC/SMBN.
Table 6. Relationships between soil physicochemical properties and soil microbial ecological characteristics.
Correlations between soil physicochemical properties and soil microbial properties
showed that pH was negatively and significantly correlated with PLFAs of bacteria, fungi and bacteria/fungi as well as total soil PLFAs, suggesting that the increase in soil pH inhibited the vital activity of microorganisms in processing tomato soil, which has an adverse impact on the reproduction and survival of microorganisms. Significant negative correlation was observed between bacterial PLFAs and primary soil nutrients; significant positive correlation was observed between bacterial PLFAs, bacteria/fungi and soil organic matter, total nitrogen. Thus, the PLFAs of different groups in processing tomato soil could represent soil fertility, and soil nutrient content could also directly influence the quantity and species of soil microorganisms.
Table 7. Relationships between soil physicochemical properties and soil microbial PLFAs.
Correlation among soil microbial properties
We conducted correlation analysis between soil microorganism PLFAs measured by the PLFAS method and soil microbial biomass measured by chloroform fumigation (). Fungi PLFAs significant negative correlation with soil microbial biomass and qMB, while significant positive correlation with SMBC/SMBN. Highly significant positive correlation was observed among bacterial PLFAs, SMBC, SMBN and qMB. Actinomycetic PLFAs significantly positive correlation with SMBP. Therefore, measures of soil microbial carbon, nitrogen and phosphorus can be used to enhance the accuracy of estimating bacteria, fungi and total soil microbial biomass.
Table 8. Relationships between soil microbial ecological characteristics and soil microbial PLFAs.
Discussion
Soil microbial biomass
Microbial biomass can reflect soil conditions such as the available nutrients, biological activity, and energy cycles (Tischer et al. Citation2014). Studies have shown that microbial community composition and functions are heavily influenced by the changes in the intensity and history of agricultural methods used (Grayston et al. Citation2004), which in turn are also usually directly reflected in the changes in microbial biomass. More recent models associate microorganisms, as either single or multiple pools, with biogeochemical processes through the production of extracellular enzymes catalyzing the decomposition of organic matter (Allison Citation2012). Rates of enzyme production are positively correlated with the growth of microbial biomass, which is also limited by the availability of C and N. Specifically, since fungal biomass contains relatively more C than bacterial biomass, fungi then could have higher C-demand than bacteria, while bacteria are more constrained by nutrient ratios (Keiblinger et al. Citation2010). The results of this study showed that long-term continuous cropping of processing tomato dramatically lowered soil microbial biomass ( and ), consistent with previous studies looking at the effects of long-term continuous cropping in cucumber, cotton and soybean (Acosta-Martínez et al. Citation2010; Li et al. Citation2010; Zhou et al. Citation2014).
For soil biological activity, long-term intensive farming methods like plowing severely eroded the surface soils, which accelerated the mineralization of organic matter. At the same time, the removal of straw from the field when harvesting processing tomato directly reduced the formation of soil humus, leading to a decrease in the supply of carbon and nitrogen for microorganisms. This had an adverse impact on their reproduction and metabolic activity. Further, long-term continuous cropping also caused the accumulation of autotoxins secreted by the root systems, increased pH, and changed the microflora by inducing the shift to a fungi-dominated community (Li et al. Citation2010; Zhang et al. Citation2013; Zhou et al. Citation2014). All of these changes were detrimental to the growth of the microorganisms, leading to an eventual decline in the total microflora ( and ).
The ratio of soil microbial biomass over total nutrients can better reflect the efficiency of microorganisms in using carbon and nitrogen resources and could further reveal variations in the biological properties of rhizospheres under continuous cropping systems of processing tomato (Sparling Citation1997). Our results showed that indeed, processing tomato continuous cropping decreased the qMB (), which was manifested by two major changes. First, the decrease in the active organic carbon, and its slowed turnover rate had adverse impacts on the ability of soil minerals to immobilize organic matter, which led to the loss of soil carbon and nitrogen (). Second, continuous cropping led to an imbalance in the available carbon and nitrogen usable to the microorganisms, causing differences in their requirements for external energy. This would influence the value of qMB as a whole, which was reflected in the changes in soil microflora (Sparling Citation1997; Li et al. Citation2010; Zhou et al. Citation2014).
The significant changes in SMBC/SMBN indicate that the high microbial biomass was associated with the changes in soil microbial community structure (Fang et al. Citation2013). Results showed that under continuous cropping, SMBC/SMBN tended to increase with longer cropping, varying from 4.84–10.88 (). In general, a C:N ratio of approximately 10:1 indicates the dominance of fungi in the soil, and 6:1 with actinomycetes, and a C:N ratio of approximately 5:1 with the dominance of bacteria (Paul and Clark Citation1989). Thus, as continuous cropping was extended, the soil also changed from a bacteria-dominated state to a fungi-dominated community (Li et al. Citation2010; Zhou et al. Citation2014). Other studies also asserted that the variations and quantity of root exudates could also change soil microflora and destroy the equilibrium of the rhizosphere microbial community, where harmful bacteria would be favored to reproduce and beneficial bacteria would be filtered out, impeding continuous cropping (Bever et al. Citation2012; Zhou and Wu Citation2013).
Soil microbial community structure
PLFA spectogram analysis can be used to determine variations in microbial biomass in an accurate and objective manner, and even reveal the differences among microbial community structures. Our research showed that the soils under different cropping years with processing tomato contained the same kinds of PLFA, with 13 PLFA biomarkers () in total but with significant differences in their occurrences among the soils. For example, CK had more PLFA types than those with continuous cropping of 3 years, while 3 > 5 > 7 years in terms of PLFA content (). This means that continuous cropping could lead to a sharp drop in soil microbial biomass, similar to those observed in pea (Nayyar et al. Citation2009) and maize (Guo et al. Citation2011). Long-term intensive farming measures could have destabilized the soil structure and decreased the abundance and evenness of the community, inhibited metabolic activity of the microorganisms, and led to a decrease in microbial diversity. Altogether, these changes contributed to the eventual decrease in soil microbial biomass ( and ).
Furthermore, we also observed the varying effects of continuous cropping on the PLFA content of the different microbial communities in the soil (). In particular, continuous cropping led to a decrease in the PLFA content of soil bacteria and actinomycetes, and an increase in fungi. This suggests that the soil was moving from bacteria- to fungi-dominated soil after continuous cropping, which again was consistent with previous studies (Li et al. Citation2010; Zhou et al. Citation2014). Other studies have also reported that the high concentration of root exudates after continuous cropping could induce the growth of soil fungi (Berendsen et al. Citation2012; Zhou and Wu Citation2013).
As for the ratio of bacteria and fungi PLFAs, continuous cropping significantly lowered the ratio (), indicating that the stability of the soil ecosystem gradually decreased (Bossio et al. Citation2005). It further indicates that continuous cropping could weaken the buffering capacity of soil to resist stress. In soybean continuous cropping, Li et al. (Citation2010) concluded that the changes in microbial community structure were a result of allelochemicals from root secretions. The flavones in these root allelochemicals were positively correlated with the microbial community (Guo et al. Citation2011). The processing tomato also displayed an allelopathic effect, with primary alleochemicals composed of phthalic acid, benzoic acid and cinnamic acid (Jiang et al. Citation2015). The correlation of the amounts of allelochemicals with the soil microbial community, however, is yet to be determined.
Correlations between soil microbial properties and soil physicochemical properties
In this experiment, the use of fumigation and PLFAs methods aided to thoroughly analyze the changes in the soil microbial community. Results showed strongly significant negative correlation (P < 0.01) between total fungal PLFAs and soil microbial biomass (). This was interestingly in contradiction to those reported by Zhao et al. (Citation2015). However, this disparity might be associated with the inherent differences in the soil samples and experimental treatments. Despite this, it could still be concluded that the changes in soil microbial biomass were mainly a result of the variation of the quantity of bacteria and fungi in the soil, making their variation trends significantly correlated. A highly significant positive correlation (p < 0.01) was observed between both total bacterial PLFA and total soil PLFA, and between SMBC and SMBN, and a highly significant positive correlation (R = 0.9630) between actinomycete PLFAs and SMBP (). These results were in agreement with those observed in farmland ecosystems, where the soil microbial diversity was significantly correlated with microbial biomass (Sparling Citation1997; Zhou et al. Citation2014).
There are three main soil microbial groups, namely, bacteria, fungi and actinomycetes, which together constitute majority of the soil microbial biomass. The composition of these microflora and the changes in their abundance represent biological activity. Correlation analysis suggested that highly significant negative correlation was observed between fungal PLFAs and the main soil nutrients (). A significantly positive correlation (p < 0.05) was also observed between bacterial PLFAs and total soil PLFAs, and organic matter and total nitrogen (). This suggests that organic matter and total nitrogen might be the main factors influencing the growth of soil microorganisms, thus it is possible to use total soil PLFAs, bacteria and fungi PLFAs to reflect soil carbon and nitrogen content. However, the continuous cropping in this study led to the deterioration of soil quality, decreased organic matter and total nitrogen contents (), and inhibition of the growth and reduction in the biomass of bacteria and actinomycetes, suggesting a correlation between soil microbial biomass and soil fertility (Sui et al. Citation2013; Luo et al. Citation2015). The growth of fungi on the other hand was not influenced by the deterioration of soils after continuous cropping, as seen from its higher biomass ( and ). Previous studies suggested that, compared with bacteria, fungi were more adapted to nutrient-poor conditions (Ding et al. Citation2015).
Conclusion
In conclusion, this study showed that soil microbial communities changed during a continuous cropping of processing tomato. On average, soil pH, microbial biomass C:N ratio, as well as fungal PLFAs significantly increased, while organic matter, microbial biomass, qMB, bacterial PLFAs and total PLFAs significantly declined. Available P, biomass-associated P, and actinomycetic PLFAs increased first in the third cropping and then decreased afterwards. Phospholipid fatty acid (PLFA) profiles revealed that soil microbiota and diversity were significantly affected by the continuous cropping. All these changes could ultimately result in the eventual poort growth of the processing tomato during monocropping. By implication, this suggests that growing sustainable geoponic measures to alleviate soil conditions such as pH, organic matter, microbial biomass, and even soil microbiota are strongly recommended for growing processing tomato in Xinjiang, China. However, conclusions portray is only based on a pot experiment in this study and may diversification from under other experimental conditions (such as in the field), which need to be disposed in the future.
Acknowledgements
The authors also thank Dr William J. Gale for his assistance in editing this manuscript.
Disclosure statement
No potential conflict of interest was reported by the authors.
Notes on contributors
Yalong Kang, man, 1988, Master Degree Candidate, profession:crop cultivation and farming, the main research direction is physiological-ecological characteristic.
Feng Jing, man, 1990, Master Degree Candidate, profession: crop cultivation and farming.
Wenqing Sun, man, 1990, Master degree candidate, profession: crop cultivation and farming, the main research direction is physiological-ecological characteristic.
Jianguo Liu, man, 1968, professor, The research direction is farmland ecological environment.
Guiying Jiang, woman, 1967, professor, physiological-ecological characteristic.
Additional information
Funding
References
- Acosta-Martínez V, Burow G, Zobeck TM, Allen VG. 2010. Soil microbial communities and function in alternative systems to continuous cotton. Soil Sci Soc Am J. 74:1181–1192. doi: 10.2136/sssaj2008.0065
- Allison SD. 2012. A trait-based approach for modelling microbial litter decomposition. Ecol Lett. 15:1058–1070. doi: 10.1111/j.1461-0248.2012.01807.x
- Anderson JPE, Domsch KH. 1978. A physiological method for the quantitative measurement of microbial biomass in soils. Soil Biol Biochem. 10:215–221. doi: 10.1016/0038-0717(78)90099-8
- Berendsen RL, Pieterse CM, Bakker PA. 2012. The rhizosphere microbiome and plant health. Trends Plant Sci. 17:478–486. doi: 10.1016/j.tplants.2012.04.001
- Bever JD, Platt TG, Morton ER. 2012. Microbial population and community dynamics on plant roots and their feedbacks on plant communities. Annu Rev Microbiol. 66:265–283. doi: 10.1146/annurev-micro-092611-150107
- Bossio D, Girvan MS, Verchot L, Bullimore J, Borelli T, Albrecht A, Scow K, Ball AS, Pretty J, Osborn AM. 2005. Soil microbial community response to land use change in an agricultural landscape of western Kenya. Microbial Eco. 49:50–62. doi: 10.1007/s00248-003-0209-6
- Brookes PC, Powlson DS, Jenkinson DS. 1982. Measurement of microbial biomass phosphorus in soil. Soil Bio Biochem. 14:319–329. doi: 10.1016/0038-0717(82)90001-3
- Ding XF, Wu CD, Huang J, Zhou RQ. 2015. Interphase microbial community characteristics in the fermentation cellar of Chinese Luzhou-flavor liquor determined by PLFA and DGGE profiles. Food Res Int. 72:16–24. doi: 10.1016/j.foodres.2015.03.018
- Fang SZ, Liu D, Tian Y, Deng SP, Shang XL. 2013. Tree species composition influences enzyme activities and microbial biomass in the rhizosphere: a rhizobox approach. PloS One. 8(4):e61461. doi: 10.1371/journal.pone.0061461
- Feng Y, Motta A, Reeves D, Burmester C, Van Santen E, Osborne J. 2003. Soil microbial communities under conventional-till and no-till continuous cotton systems. Soil Bio Biochem. 35:1693–1703. doi: 10.1016/j.soilbio.2003.08.016
- Ferris MJ, Ward DM. 1997. Seasonal distributions of dominant 16S rRNA-defined populations in a hot spring microbial mat examined by denaturing gradient gel electrophoresis. Appl Environ Microb. 63:1375–1381.
- Francisco R, Stone D, Creamer RE, Sousa JP, Morais PV. 2016. European scale analysis of phospholipid fatty acid composition of soils to establish operating ranges. Appl Soil Ecol. 97:49–60. doi: 10.1016/j.apsoil.2015.09.001
- Frostegård Å, Bååth E. 1996. The use of phospholipid fatty acid analysis to estimate bacterial and fungal biomass in soil. Biol Fert Soils. 22:59–65. doi: 10.1007/BF00384433
- Frostegård Å, Tunlid A, Bååth E. 1993. Phospholipid fatty acid composition, biomass, and activity of microbial communities from two soil types experimentally exposed to different heavy metals. Appl Environ Microb. 59:3605–3617.
- Garland JL, Mills AL. 1991. Classification and characterization of heterotrophic microbial communities on the basis of patterns of community-level sole-carbon-source utilization. Appl Environ Microb. 57:2351–2359.
- Ge T, Li B, Zhu Z, Hu Y, Yuan H, Dorodnikov M, Jones DL, Wu J, Kuzyakov Y. 2017. Rice rhizodeposition and its utilization by microbial groups depends on N fertilization. Biol Fert Soils. 53:37–48. doi: 10.1007/s00374-016-1155-z
- Gentry LF, Ruffo ML, Below FE. 2013. Identifying factors controlling the continuous corn yield penalty. Agron J. 105:295–303. doi: 10.2134/agronj2012.0246
- Grayston S, Campbell C, Bardgett R, Mawdsley J, Clegg C, Ritz K, Griffiths B, Rodwell J, Edwards S, Davies W. 2004. Assessing shifts in microbial community structure across a range of grasslands of differing management intensity using CLPP, PLFA and community DNA techniques. Appl Soil Ecol. 25:63–84. doi: 10.1016/S0929-1393(03)00098-2
- Guan SY. 1986. Soil enzyme and its research methods. Agricultural, Beijing (in Chinese).
- Guo ZY, Kong CH, Wang JG, Wang YF. 2011. Rhizosphere isoflavones (daidzein and genistein) levels and their relation to the microbial community structure of mono-cropped soybean soil in field and controlled conditions. Soil Bio Biochem. 43:2257–2264. doi: 10.1016/j.soilbio.2011.07.022
- Hao X, Liu S, Wu J, Hu R, Tong C, Su Y. 2008. Effect of long-term application of inorganic fertilizer and organic amendments on soil organic matter and microbial biomass in three subtropical paddy soils. Nutr Cycl Agroecosys. 81:17–24. doi: 10.1007/s10705-007-9145-z
- Herrmann R, Shann J. 1997. Microbial community changes during the composting of municipal solid waste. Microbial Ecol. 33:78–85. doi: 10.1007/s002489900010
- Huang LF, Song LX, Xia XJ, Mao WH, Shi K, Zhou Y-H, Yu JQ. 2013. Plant-soil feedbacks and soil sickness: from mechanisms to application in agriculture. J Chem Ecol. 39:232–242. doi: 10.1007/s10886-013-0244-9
- Jenkinson DS. 1988. Determination of microbial biomass carbon and nitrogen in soil.
- Jiang GY, Liu JG, Li YB. 2015. Allelochemicals from cotton (Gossypium hirsutum) rhizosphere soil: inhibitory effects on cotton seedlings. Allelopathy J. 35:153–162.
- Joergensen RG, Potthoff M. 2005. Microbial reaction in activity, biomass, and community structure after long-term continuous mixing of a grassland soil. Soil Bio Biochem. 37:1249–1258. doi: 10.1016/j.soilbio.2004.11.021
- Keiblinger KM, Hall EK, Wanek W, Szukics U, Hämmerle I, Ellersdorfer G, Böck S, Strauss J, Sterflinger K, Richter A. 2010. The effect of resource quantity and resource stoichiometry on microbial carbon-use-efficiency. FEMS Microbiol Ecol. 73:430–440.
- Klose S, Acostamartinez V, Ajwa HA. 2006. Microbial community composition and enzyme activities in a sandy loam soil after fumigation with methyl bromide or alternative biocides. Soil Bio Biochem. 38:1243–1254. doi: 10.1016/j.soilbio.2005.09.025
- Li CG, Li XM, Kong WD, Ying W, Wang JG. 2010. Effect of monoculture soybean on soil microbial community in the Northeast China. Plant Soil. 330:423–433. doi: 10.1007/s11104-009-0216-6
- Li JG, Ren GD, Jia ZJ, Dong YH. 2014. Composition and activity of rhizosphere microbial communities associated with healthy and diseased greenhouse tomatoes. Plant Soil. 380:337–347. doi: 10.1007/s11104-014-2097-6
- Liang C, Jesus EDC, Duncan DS, Jackson RD, Tiedje JM, Balser TC. 2012. Soil microbial communities under model biofuel cropping systems in southern Wisconsin, USA: impact of crop species and soil properties. Appl Soil Ecol. 54:24–31. doi: 10.1016/j.apsoil.2011.11.015
- Luo P, Han X, Wang Y, Han M, Shi H, Liu N. 2015. Influence of long-term fertilization on soil microbial biomass, dehydrogenase activity, and bacterial and fungal community structure in a brown soil of northeast China. Ann Microbiol. 65:533–542. doi: 10.1007/s13213-014-0889-9
- Lupwayi NZ, Lafond GP, May WE, Holzapfel CB, Lemke RL. 2012. Intensification of field pea production: impact on soil microbiology. Agron J. 104:1189–1196. doi: 10.2134/agronj2012.0046
- Mahmoudi N, Robeson MS, Castro HF, Fortney JL, Techtmann SM, Joyner DC, Paradis CJ, Pfiffner SM, Hazen TC. 2015. Microbial community composition and diversity in Caspian Sea sediments. FEMS Microbiol Ecol. 91:1–11. doi: 10.1093/femsec/fiu013
- Mccarthy CM, Murray L. 1996. Viability and metabolic features of bacteria indigenous to a contaminated deep aquifer. Microbial Ecol. 32:305–321. doi: 10.1007/BF00183065
- Myers RM, Fischer SG, Lerman LS, Maniatis T. 1985. Nearly all single base substitutions in DNA fragments joined to a GC-clamp can be detected by denaturing gradient gel electrophoresis. Nucleic Acids Res. 13:3131–3145. doi: 10.1093/nar/13.9.3131
- Nayyar A, Hamel C, Lafond G, Gossen BD, Hanson K, Germida J. 2009. Soil microbial quality associated with yield reduction in continuous-pea. Appl Soil Ecol. 43:115–121. doi: 10.1016/j.apsoil.2009.06.008
- Paul EA, Clark FE. 1989. Soil microbiology and biochemistry. Sandiego, CA: Academic Press.
- Rutigliano FA, Romano M, Marzaioli R, Baglivo I, Baronti S, Miglietta F, Castaldi S. 2014. Effect of biochar addition on soil microbial community in a wheat crop. Eur J Soil Biol. 60:9–15. doi: 10.1016/j.ejsobi.2013.10.007
- Sparling GP. 1997. Soil microbial biomass, activity and nutrient cycling as indicators of soil health. In: Pankhurst CE, Doube BM, Gupta VVSR, editors. Biological indicators of soil health. Wallingford: CAB International; p. 97–119.
- Sui Y, Jiao X, Liu X, Zhang X, Ding G. 2013. Response of soil microbial biomass and enzyme activity to soil fertilization in an eroded farmland of Chinese mollisols. Commun Soil Sci Plan. 44:2809–2819. doi: 10.1080/00103624.2013.811525
- Tischer A, Potthast K, Hamer U. 2014. Land-use and soil depth affect resource and microbial stoichiometry in a tropical mountain rainforest region of southern Ecuador. Oecologia. 175:375–393. doi: 10.1007/s00442-014-2894-x
- White DC, Davis WM, Nickels JS, King JD, Bobbie RJ. 1978. Determination of the sedimentary microbial biomass by extractible lipid phosphate. Oecologia. 40:51–62. doi: 10.1007/BF00388810
- Xiao X, Cheng Z, Meng H, Liu L, Li H, Dong Y. 2013. Intercropping of green garlic (Allium sativum L.) induces nutrient concentration changes in the soil and plants in continuously cropped cucumber (Cucumis sativus L.) in a plastic tunnel. Plos One. 8: e62173. doi: 10.1371/journal.pone.0062173
- Xiong W, Li Z, Liu H, Xue C, Zhang R, Wu H, Li R, Shen Q. 2015. The effect of long-term continuous cropping of black pepper on soil bacterial communities as determined by 454 pyrosequencing. Plos One. 10: e0136946. doi: 10.1371/journal.pone.0136946
- Zelles L. 1999. Fatty acid patterns of phospholipids and lipopolysaccharides in the characterisation of microbial communities in soil: a review. Biol Fert Soils. 29:111–129. doi: 10.1007/s003740050533
- Zelles L, Bai QY, Rackwitz R, Chadwick D, Beese F. 1995. Determination of phospholipid- and lipopolysaccharide-derived fatty acids as an estimate of microbial biomass and community structures in soils. Biol Fert Soils. 19:115–123. doi: 10.1007/BF00336146
- Zhang Q, Zhou W, Liang G, Sun J, Wang X, He P. 2015. Distribution of soil nutrients, extracellular enzyme activities and microbial communities across particle-size fractions in a long-term fertilizer experiment. Appl Soil Ecol. 94:59–71. doi: 10.1016/j.apsoil.2015.05.005
- Zhang W, Long X, Huo X, Chen Y, Lou K. 2013. 16S rRNA-based PCR-DGGE analysis of actinomycete communities in fields with continuous cotton cropping in Xinjiang, China. Microbial Ecol. 66:385–393. doi: 10.1007/s00248-012-0160-5
- Zhao J, Zeng Z, He X, Chen H, Wang K. 2015. Effects of monoculture and mixed culture of grass and legume forage species on soil microbial community structure under different levels of nitrogen fertilization. Eur J Soil Biol. 68:61–68. doi: 10.1016/j.ejsobi.2015.03.008
- Zhou X, Gao D, Liu J, Qiao P, Zhou X, Lu H, Wu X, Liu D, Jin X, Wu F. 2014. Changes in rhizosphere soil microbial communities in a continuously monocropped cucumber (Cucumis sativus L.) system. Eur J Soil Biol. 60:1–8. doi: 10.1016/j.ejsobi.2013.10.005
- Zhou X, Wu F. 2013. Artificially applied vanillic acid changed soil microbial communities in the rhizosphere of cucumber (Cucumis sativus L.). Can J Soil Sci. 93:13–21. doi: 10.4141/cjss2012-039
- Zhou X, Yu G, Wu F. 2012. Soil phenolics in a continuously mono-cropped cucumber (Cucumis sativus L.) system and their effects on cucumber seedling growth and soil microbial communities. Eur J Soil Biol. 63:332–340. doi: 10.1111/j.1365-2389.2012.01442.x
- Zogg GP, Zak DR, Ringelberg DB, White DC, MacDonald NW, Pregitzer KS. 1997. Compositional and functional shifts in microbial communities due to soil warming. Soil Sci Soc Am J. 61:475–481. doi: 10.2136/sssaj1997.03615995006100020015x