Abstract
A possible cost-effective real-time patch spraying implementation against seed-propagated broad-leaved weeds in cereals is a camera mounted in front of the tractor taking images at feasible distances in the direction of travel, on-board image analysis software and entire boom switched on and off. To assess this implementation, manual weed counts (0.25 m2 quadrats) in a 1.5 m×2 m grid, were used to simulate camera outputs. Each quadrat was classified into ‘spray’ and ‘not spray’ decisions based on a threshold model, and the resulting map defined the ‘ground truth’. Subsequently, ‘on/off’ spraying at larger control areas where sizes were given by the boom width and image distance, and spraying decision controlled by weed status at the single quadrat simulating the camera's view, were simulated. These coarser maps were compared with ‘ground truth’, to estimate mapping error (area above threshold not sprayed), spraying error (area below threshold sprayed), total error (sum of mapping and spraying error) and the herbicide reduction. Three levels of the threshold model were tested. Results were used to fit models that predict errors from boom width and image distance. Size of control area did not on average affect the magnitude of the simulated herbicide reductions, but the bigger the control area the higher the risk that the simulated herbicide reduction deviate from the reduction in ‘ground truth’. Mean simulated herbicide reductions were 42–59%, depending on threshold level. Only minor differences due to threshold level were seen for mean mapping and spraying errors at given spraying resolutions. Using original threshold level and image distance 2 m, predicted total errors for boom widths 2 m, 6 m, 20 m and 40 m would be 6%, 10%, 16% and 17%, respectively. Results indicate that control area should not exceed about 10 m2 if acceptable total error is maximum 10%.
Introduction
The spatial distribution of weeds within agricultural fields is generally heterogeneous, with the weeds occurring in patches (e.g. Wallinga et al., Citation1998; Dieleman & Mortensen, Citation1999; Cousens & Croft, Citation2000). Therefore, site-specific weed control or herbicide patch spraying represents a potential for reduced herbicide consumption (Johnson et al., Citation1995), increased net return (Timmermann et al., Citation2003) and lowered risk of soil and water pollution.
There are two main approaches for implementing site-specific weed control. In the real-time approach weed monitoring and spraying are carried out during one operation, and in the map-based approach in subsequent operations. While reflectance based devices with real-time specifications are available (e.g. Weedseeker, Ntech Industries, Inc.), they are of limited use unless the (green) weed is detected against a bare soil background (Scotford & Miller, Citation2005). For row crops the use of image analysis technology for real-time implementations has proved promising (Steward & Tian, Citation1999; Tian, Citation2002; Onyango & Marchant, Citation2003). Cereals, however, are grown in narrow rows (in northern Europe separated by only 12–15 cm) that frequently cause the leaves from cereals and weeds to overlap in the images. Together with the generally high diversity of weed species and leaf shapes, the development of robust image analysis algorithms for real-time patch spraying within cereals has progressed slowly. However, this task is continually challenged (e.g. Gerhards et al., Citation1993; Andreasen et al., Citation1997; Pérez et al., Citation2000; Sökefeld et al., Citation2000; Manh et al., Citation2001; Gerhards & Christensen, Citation2003; S⊘gaard, Citation2005), and it is expected that feasible algorithms will be available. Owing to demands on image quality, it seems unrealistic to apply images of the same size and area as a sprayer's swath, and questions related to image sampling and spraying resolution will inevitably arise when implementing the real time one-camera system. At what distances in the direction of travel should images be taken? Is the width of booms feasible? What is the recommended width of boom sections if sprayings errors are to be limited to an acceptable level, e.g. 10%?
Since an increasing resolution has an important associated cost (e.g. Barroso et al., Citation2004), we explored the magnitude of discrepancy in spraying decisions between high resolutions of mapping and spraying on the one hand (‘ground truth’) versus decreasing mapping and spraying resolutions on the other. Suitability of this envisaged implementation in terms of magnitude of mapping errors (areas above threshold not sprayed) and spraying errors (areas below threshold incorrectly sprayed) is poorly understood. The method currently presented has only marginally been studied for weeds in cereals and, to the best of our knowledge, never at this high resolution (2 m×1.5 m, i.e. more than 3000 samples of size 0.25 m2 ha−1, corresponding to about 7.5% of total area mapped). Working on a much coarser scale, Nordbo et al. (Citation1994) showed that mean erroneously treated (‘spray’, ‘not spray’) area increased when reducing the monitoring effect from 25 samples ha−1 down to 1.3 samples ha−1. By distance transform analysis of scanned weed maps, Carroll and Holden (Citation2005) showed for one selected patch spraying technology (pressure controlled, ‘on/off’ and three individual 5-m boom segments on a 15-m boom) that errors due to ‘control distance perpendicular to direction of travel’, ‘forward speed’ and ‘control time’, varied between cereal fields due to the field-specific (grass) weed patchiness (number, shape, and size of patches). Berge et al. (Citation2007), using a coarser grid than currently, showed that mean total spraying errors increased linearly by increasing boom width (i.e. decreasing spraying resolution).
From studies on the map-based approach, it is known that reliability of interpolated weed maps is largely influenced by the mapping resolution, particularly the distance between the quadrats (Rew & Cousens, Citation2001; Cousens et al., Citation2002; Dille et al., Citation2002), simply because a small subsample of a surveyed area (often <2%) might miss important characteristics (Backes et al., Citation2005). It has also been illustrated that reliability of discrete samples depends on the actual weed patch size and the biological features (‘scattered’ or ‘clustered’) of the species (Backes & Plümer, Citation2003; Backes et al., Citation2005). Obviously, the current envisaged real-time implementation would be influenced by the mapping resolution (here: distance between images in the travel direction) and the spraying resolution (here: the size of control area). Hence, research is needed to assess the consequences of the envisaged application before it can be implemented.
The practical use of weed damage threshold models (e.g. Gerowitt & Heitefuss, Citation1990; Black & Dyson, Citation1993) has been limited because cost-effective methods to assess the weed populations have been unavailable (Wilkerson et al., Citation2002). In the near future, image analysis technology will hopefully represent this cost-effective method. In the present paper, we applied a threshold model proposed for spring cereals in Norway (Fykse, Citation1991), in a site-specific manner. Since threshold values probably vary between cereal species and varieties (Christensen, Citation1994), threshold values were also modified by ±25%.
The main objective of the present study was to assess the accuracy of using one envisaged sample image (0.25 m2) captured in the direction of travel to decide the spraying decision of control areas (about 4–600 m2) whose size were given by the boom width and the distance between the images, an approach intended for ‘on/off’ patch spraying against newly emerged broad-leaved weeds in cereals when appropriate, robust image analysis algorithms become available. Other aims were to determine whether the results depended on the actual level of the applied threshold model, and to estimate herbicide reductions through the simulated mapping and spraying resolutions.
Material and methods
Nineteen trials randomly located within 19 different spring cereal fields in south-east Norway, were surveyed in either 2001 or 2002. Because these trials were also used for other purposes, they had four replications arranged in a block design with 3 m between the blocks. For the present study, the weed seedlings were manually counted by species within quadrats (0.25 m2) at the intersections of a 1.5 m×2 m grid within each block just prior to the normal time for post-emergence herbicide application, i.e. the crop at Zadoks 13–14 (Zadoks et al., Citation1974). Each trial included 192 quadrats and covered approximately 630 m2 ().
Figure 1. Experimental design with an example of spraying decisions in ‘ground truth’: open (□) and filled squares (▪) are quadrats (0.25 m2) with weed densities below and above the threshold, respectively. Rectangles (- - -) delimit sprayed patches by a 14.5 m wide boom travelling in direction 1 and images taken every 1.5 m (3 m) along the line indicated by the arrow on the left. Further details in text (Material and methods).
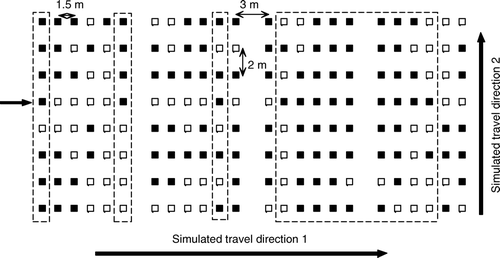
Treating (‘spray’/‘not spray’) each quadrat (and its nearest zone) individually represented the finest spraying resolution possible by the current dataset, and was defined as ‘ground truth’. A damage threshold model developed for seed-propagated broad-leaved weeds in spring cereals at Zadoks 13–14 of the crop (Fykse, Citation1991) was applied site-specifically (). If the density of all weed species exceeded 175 plants m−2, or the threshold density of either Galeopsis spp. L., Chenopodium album L., Brassica rapa ssp. oleifera (DC.) Metzger, Stellaria media (L.) Vill. or Galium aparine L. was passed, the decision was ‘spray’ (). Furthermore, if the sum of two or more of these five species exceeded the threshold density of the species having the highest threshold value, the decision was also ‘spray’. The presence of G. aparine often leads to lodging, and the threshold value (1 plant m−2) expresses the problems connected to harvest and drying, and not its yield-depressing effect. As an attempt to apply the weed densities from the original threshold model with more confidence, the original threshold levels were also increased and reduced by 25% (). The original threshold model (Fykse, Citation1991) also included relative crop/weed ground coverage, but as this parameter was not recorded in the current fields, it was omitted in this study.
Table I. Definition and weed threshold densities (plants m−2) of threshold model, after Fykse (1991). Decision is ‘spray’ if criterion xx is met.
‘On’/‘off’ patch spraying was simulated in control areas whose sizes were defined by the working width of the envisaged sprayer and the distance between images in the direction of travel, hereafter termed ‘image distance’ (). Quadrats selected to represent the camera observations were randomized between the two middle-most ‘camera positions’, and randomized for each working line. To counteract possible random effects of camera position, 2–5 simulations (replicates) were run for each combination of boom width, image distance and threshold level per trial. In total, 7524 simulations were run (396 simulations per trial). The width of the sprayer in metres was defined as the distance between the outer edges of the margin quadrats delimiting the boom width. Two directions of travel were simulated (). Seven different boom widths between 2.0 m and 39.5 m, and eight different image distances between 1.5 m and 19.5 m, were simulated. These combinations corresponded to control areas being from 3.75 m2 to 632 m2.
A computer program, written in Matlab (CitationMathworks Inc.), generated the following output data: number of quadrats sprayed in ‘ground truth’, but unsprayed by coarser resolution (U), number of quadrats not sprayed in ‘ground truth’, but sprayed by coarser resolution (S), number of quadrats below threshold in ‘ground truth’ (B) and number of quadrats unsprayed by coarser resolution (R). Five response variables were defined and calculated per simulation:
Results
Characteristics of the weed populations
Mean total weed density (across all trials) was 151 weeds m−2, standard deviation 161 weeds m−2 and coefficient of variation 107% (, last column). Mean total weed density per trial varied from 4 to 377 plants m−2. Total weed densities varied substantially also within the trials, as coefficients of variation were high, ranging from 26% to 117%. Among the threshold model species, C. album and S. media were very common, each missing in one trial only (). Their mean densities per trial varied from less than 1 to about 190 plants m−2. Galeopsis spp. were absent in three trials, and varied from less than 1 to 22 plants m−2 where present. B. rapa and G. aparine were very rare, and their mean densities per trial were generally low, i.e. less than 1 plant m−2, except in trials No. 18 and 15, respectively. Across trials, mean density of Galeopsis spp. was 3 plants m−2, of C. album 20 plants, of B. rapa 1 plant, of S. media 35 plants and of G. aparine 0.3 plants m−2 (, last column).
Table II. Summary statistics of total weed density per trial and as mean of all trials (last column).
Table III. Mean total weed density and mean density (weeds m−2) of the five criteria species in the threshold model (cf. ), per trial and as mean of all trials (last column). Rows denoted by letters (a,b,c) are proportions of quadrats (%) above the actual criterion in ‘ground truth’ when threshold values were either reduced by 25% (a), original (b) or increased by 25% (c). Empty cells = species not present.
Mapping, spraying and total errors
At each threshold level, MAE, SPE and TOE increased by boom width (p<0.0001) and by image distance (p<0.0001). For a given combination of boom width and image distance, mean MAE and SPE (across all trials) were nearly equal and about half the mean TOE value (results not shown). The smallest mean error values (irrespective of threshold level) – 3% (MAE and SPE) and 6% (TOE) – occurred at the two finest spraying resolutions: boom width 2.0 m combined with image distance 2.0 m, and boom width 2.5 m combined with image distance 1.5 m (, TOE values). The highest mean errors, at the original threshold level – 9% (MAE), 10% (SPE) and 19% (TOE) – occurred at boom width 14.5 m combined with image distance 19.5 m, and boom width 18.5 m combined with image distance 16.0 m (, TOE values).
Figure 2. Mean total error (TOE) with 95% confidence interval bars of mean versus boom width and image distance, original threshold level. Black bold lines: connected means of equal boom width.
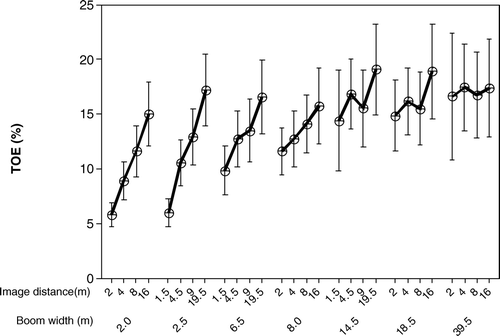
The increase in error by increasing image distance was most pronounced at the narrower booms. For MAE and TOE there were statistically significant effects of image distance, at each threshold level, for boom widths 2.0 m, 2.5 m and 6.5 m. At boom width 6.5 m, image distance 1.5 m gave lower MAE (p≤0.0316) and TOE (p≤0.0110) than image distance 19.5 m. SPE was significantly affected by image distance at boom width 8.0 m with the increased threshold level and at boom width 2.5 m with the reduced and original levels. Although the mean error values for a given spraying resolution (across trials) were relatively limited, the error values realized during one single application could deviate much from the mean and be much higher, particularly for the wider booms (results not shown).
The mean error for a given spraying resolution per trial differed greatly, and seemed to depend on the proportion of quadrats with weed infestation below the threshold (WBT) per trial ().
Figure 3. Total error (TOE) per trial at three threshold levels and two spraying resolutions: (A) boom width 2 m and image distance 2 m, and (B) boom width 14.5 m and image distance 4.5 m, versus proportion of quadrats with weed infestation below the threshold (WBT) per trial at the original threshold level. Figures above bars are trial numbers.
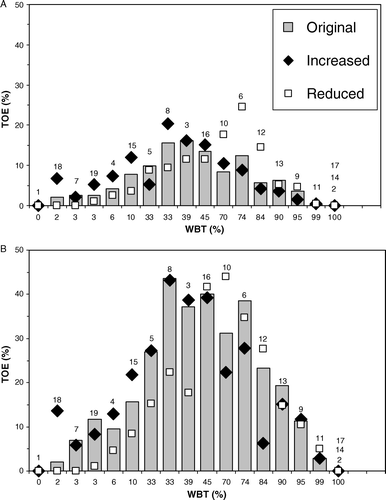
The simulation results were used to estimate regression models that could predict errors from threshold levels and spraying resolutions. Non-linear models were found to fit best:
Figure 4. Predicted total error (TOE) as a function of boom width and image distance from non-linear regression model (see equation Equation6) with parameter coefficients for original threshold ().
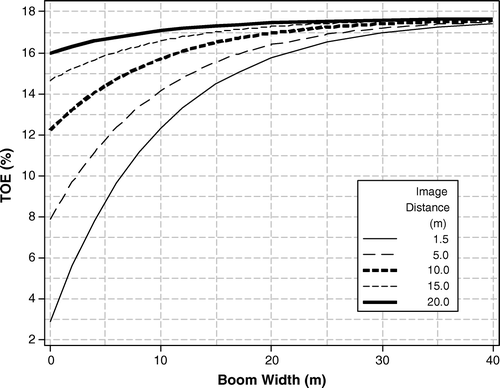
Table IV. Regression model parameter estimates for prediction of mean mapping error (MAE), spraying error (SPE) and total error (TOE) from boom width and image distance. SE = standard error, R 2=coefficient of determination, MSE = mean square error. See equation Equation6 for model.
Herbicide reductions through patch spraying
Among criteria A–F of the threshold model, total weed density (criterion A) was on average the most important criterion for the potential herbicide reductions (PHR), followed by S. media, C. album, G. aparine, Galeopsis spp. and B. rapa (, last column). Applying all threshold criteria (‘Full model’, cf. ), PHR per trial ranged from 0 to 100% for all threshold levels (results not shown).
Threshold level had a main effect on simulated herbicide reductions (SHR), with each level being different from the others (p<0.0001). Neither boom width nor image distance (p≥0.116) were main effects on the SHR (). Hence, mean SHR (across all trials and spraying resolutions) at the three threshold levels were the same as the mean PHR values per threshold level – 42%, 52% and 59% at the reduced, original and increased threshold levels, respectively. The SHR per trial varied considerably, and was clearly dependent on the PHR per trial, but the lower the spraying resolution the more deviated the relationship between SHR and PHR from linearity ().
Figure 5. Mean simulated herbicide reduction (SHR) versus potential herbicide reduction (PHR) per trial at two selected spraying resolutions: • = boom width 2.0 m and image distance 2.0 m, ○ = boom width 39.5 m and image distance 16.0 m. Original threshold level. Data point labels are trial ID numbers.
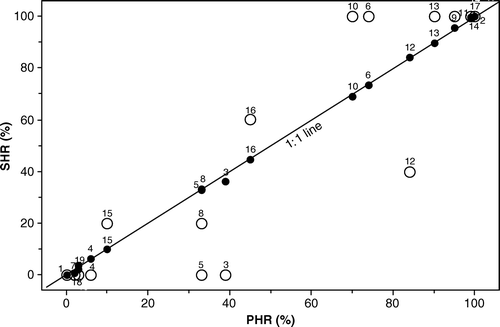
If all individual figures of SHR per spraying resolution per trial (and threshold level) were plotted, the range of the SHR values typically increased by increasing boom width and by increasing image distance (results not shown). In six trials all individual SHR values were either 0% (trial No. 1: all threshold levels; trial No. 7 and 18: reduced threshold level) or 100% (trial No. 2, 14 and 17: all threshold levels) irrespective of spraying resolution.
Influence of the threshold level
The mean errors (across trials) for the different spraying resolutions were statistically significant only for MAE, with errors at the reduced level smaller than those of the increased level (p=0.0248), but the actual differences were small: 0–5% difference between the 25% modified threshold levels. In the prediction models, significant but small differences due to threshold level occurred between the three C 0 coefficient values for MAE, SPE and TOE (all p values ≤ 0.0286). For TOE, C 0 estimates were 16%, 18% and 19% for the reduced, original and increased threshold levels, respectively ().
The influence of threshold levels on errors varied significantly between individual trials (), from no influence to e.g. about 20% difference in TOE between the reduced and the increased threshold levels at boom width 14.5 m and image distance 4.5 m (B). It seemed as if trials with either relatively low (<10%) or high (>90%) WBT values (proportion of quadrats with weed infestation below the threshold) at the original threshold were least affected by modified threshold level, particularly at the coarser resolutions (B). A notable exception was trial No. 16, particularly at the coarser spraying resolution (cf. B). This was possibly linked to a very flat frequency distribution of total weed density in this trial (results not shown). Trial No. 18 had comparable high errors at the increased threshold level, probably due to a relatively strong B. rapa infestation (cf. ).
In the four trials where the threshold level did not influence the errors, neither PHR nor SHR were affected by changed threshold level. As with the errors, trials with either relatively low (<10%) or high (>90%) WBT values at the original threshold level, seemed to be least affected by modified threshold level for both PHR (results not shown) and SHR ().
Discussion
Mapping, spraying and total errors
When the distance between image samples and size of control area increased, the deviations from spraying decisions in ‘ground truth’, i.e. the spraying and mapping errors, increased. Although conducted with different datasets and approaches, earlier research has shown that errors based on discrepancies between ‘ground truth’ and coarser scales, increased with increasing ‘sample point distance’ (Nordbo et al., Citation1994), ‘control distances’ (Carroll & Holden, Citation2005) and ‘boom widths’ (Berge et al., Citation2007) and with decreasing ‘resolution’ (Barroso et al., Citation2004). A new and interesting phenomenon seen in this study was that the sum of mapping and spraying errors was not increasing boundlessly, but was restricted to below 20% (cf. and ), which we think is reasonably low, indicating relatively small risks for the envisaged one-camera system.
The resolution at which patch spraying is operated is likely to be a major factor in determining its cost effectiveness (Paice et al., Citation1996), and costs will increase with the use of finer resolutions (Rew & Cousens, Citation2001; Barroso et al., Citation2004). Hence, to reduce investment cost for patch spraying, sprayers already in use should be utilized (Carroll & Holden, Citation2005). In other words, boom width should in practice be assumed fixed, while image distance might be adjusted. Since present findings showed that reducing image distance is generally worthwhile, the question is how short is image distance practically feasible? If the driving speed is fixed to 7 km h−1 and the time between image capture and nozzle response is 5 s (cf. Carroll & Holden, Citation2005), minimum image distance would be approximately 10 m ((7×1000 m)/(60×60 s)×5 s = 9.72 m). Spray booms used in Norway are generally not wide, and for a 12-m boom (without segmentation), image distance of 10 m would correspond to mean TOE 16% (original threshold level). For wider booms common in European grain crop production, e.g. 20 m and 40 m, mean TOE would not be much higher – 17% and 18%, respectively. If the acceptable errors are lower than these figures, spray booms with individually controllable segments (cf. Stafford & Miller, Citation1993; Gerhards et al., Citation2002b; Carroll & Holden, Citation2005; Gerhards & Oebel, Citation2006) must be implemented, with one camera per segment, i.e. increasing the mapping and the spraying resolution. For a boom segment of only 2 m, present results suggest TOE still to be 13% (for the original threshold and image distance 10 m). Alternatively, driving speed has to be reduced, thereby rendering possible shorter image distances. From one among very few studies on real time camera-controlled ‘on/off’ spraying in cereals, response time was only 1 s (Gerhards et al., Citation2002a). If this response time was utilized, the image distance could be about 2 m ((7×1000 m)/(60×60 s)×1 s = 1.9 m), and the predicted TOE for boom widths 2 m, 12 m, 20 m and 40 m would be 6%, 14%, 16% and 17%, respectively. MAE, that probably is generally harder to accept than SPE among farmers, would be about half of these TOE values, meaning that all the above resolutions would yield MAE below 10%. Are these error rates acceptable? To recommend an optimal mapping and spraying resolution based on present results, a more comprehensive model would be required that took into account the major associated costs and gains by the different spatial resolutions, including camera, herbicide and crop prices, possible governmental subsidies for reduced pesticide input and the consequences of unsprayed weeds. This was beyond the scope of this paper, but based on present results it could be said that if acceptable TOE is restricted to e.g. 10%, the size of control area should not exceed approximately 10 m2. For a 10-m boom, that would correspond to images taken every 1 m, which might be unrealistic, unless driving speed is reduced to about 3.6 km t−1 when response time is 1 s ((3.6×1000 m)/(60×60 s)×1 s = 1 m).
Is there a relationship between the magnitude of error in a trial and this trial's proportion of area with weed infestation below the threshold (WBT)? When parabolic curves were fitted to the relationship between WBT and the trial's value of either MAE, SPE and TOE (per threshold level) at a given spraying resolution, the maximum points of the parabolas were generally about 50% WBT (results not shown, but indicated in ). How can this be explained? For a trial with WBT value of about 50%, the generally strong autocorrelation of weeds at these spatial scales (Dieleman & Mortensen, Citation1999; Cousens & Croft, Citation2000; Backes et al., Citation2005; Heijting et al., Citation2005) implies that this trial will have a relatively high proportion of quadrats with weed densities near the thresholds. Since neighbour quadrats with weed densities near the thresholds will automatically yield more quadrat-to-quadrat shifts in spraying decisions, than neighbour quadrats with weed densities far from the thresholds, and these quadrat-to-quadrat shifts generate the errors, we hypothesize that the trials with WBT near 50% will generally generate the highest errors. Berge et al. (Citation2007) showed likewise that among three fields (approximately 1–3.8 ha), the one with mean densities of threshold criteria near the threshold values, had larger errors than fields with mean weed densities far from the thresholds. In a simulation study, Barroso et al. (Citation2004) showed that their ‘absolute mapping and spraying errors’ increased with increasing infestation level of Avena sterilis Huds. per field (sized 0.92 ha). Since their maximum simulated infestation level of field was (only) 64%, that probably explains the lack of decreasing error in highly infested trials, as currently seen. Further studies are needed to decide if this is a general rule.
We are aware that the ‘ground truth’ resolution in the present investigation does not give a complete representation of the weed populations. This means that the present ‘ground truth’ is an approximation to ‘real ground truth’, and the reliability of present error computations has to be addressed. Our mapping resolution (grid distance and quadrat size) corresponded to surveying approximately 7–8% of the area, which is reasonably good (cf. Backes et al., Citation2005). In a case study of G. aparine, Backes et al. (Citation2005) showed that discrepancies between weed quadrat counts (presumably 0.4 m2) and the equidistant surrounding ‘reference area’, in terms of root mean squared error, mean average error and bias, were small for resolutions higher than 2 m×2 m. Furthermore, Cousens et al. (Citation2002) presented ‘safe’ mapping resolutions and quadrat sizes for estimation of the geostatistical ‘range’, which for weeds can be interpreted as the characteristic scale of weed patchiness (Rew & Cousens, Citation2001). As the current ‘ground truth’ resolution was well within their ‘safe’ range, and quadrat size also comparable (0.36 m2), the present mapping and spraying error estimates should be fairly good. Since G. aparine has a very low threshold density (1 plant m−2) and generally has a very ‘patchy’ distribution (Wallinga et al., Citation1998; Backes et al., Citation2005), and hence represented a critical species, some areas of the seven trials where this species was present (cf. ) could have been missed by the discrete ‘ground truth’ mapping, consequently resulting in over-estimated herbicide reductions and inaccurate error estimates. Particularly at trial No. 11 and 15, where G. aparine was relatively important and abundant (cf. ), this could have had an effect. However, on average, we believe the present error estimates were quite reliable.
It should also be emphasized that the simulated image size (0.25 m2) was probably relatively large compared to the image sizes that will be implemented with real time image analysis technology (cf. Gerhards & Oebel, Citation2006, image size 0.02 m2). By reducing the image size, a less precise spraying decision could be expected (cf. Nordbo et al., Citation1994). A solution proposed to mitigate a too high variability in camera controlled spraying decisions within short distances, and that also would increase the above mentioned lowered precision due to small image sizes, is to base the spraying decision on a moving average, e.g. of five images (Fykse, Citation2004). The number of images actually included should be adjusted to the image size and the acceptable level of mapping and spraying errors.
Herbicide reductions through patch spraying
Simulating the finest spraying resolution possible with the present dataset, the mean PHR (across all trials) was substantial: 42–59%, depending on threshold level. These reductions were somewhat lower than reported for three Norwegian spring fields applying the same threshold model and threshold levels (Berge et al., 2007), probably attributable to field-to-field variability in weed infestation levels. The lowest value currently reported was of the same order as reported earlier for spring barley in Europe (Christensen, Citation2006; Gerhards & Oebel, Citation2006), although variable application levels, other thresholds and resolutions were applied. The relatively high mean savings reported in the present paper were due to several trials with low infestations and to the comparatively permissive threshold densities, particularly of total weed density (cf. ).
Mapping and spraying resolution were not main effects on the simulated herbicide reduction (cf. ). Berge et al. (Citation2007), who used the same threshold concept, simulated likewise that boom width had little impact on mean herbicide reductions. In a study of Elymus repens L., only minor effects on the potential herbicide reduction were seen if resolution across a 12-m boom was changed from 2 m to 4 m (Rew et al., Citation1996). When continuous mapping resolution for grass weeds increased from 2 m to 4 m and 6 m, respectively, Rew et al. (Citation1997) showed that the area to be sprayed on average increased by 5.5% and 7.5%, respectively. The present findings mean that the potential herbicide reduction (PHR), i.e. ‘ground truth’ reduction, will be achieved in the long run whatever combination of boom width and image distance used. However, the herbicide reduction realized for one field in one year might deviate much from the PHR, as illustrated for one winter wheat field when the working width of the boom changed from 3 m to 15 m (Gerhards et al., Citation1999). Present results suggest that the lower the mapping and spraying resolution the higher the risk of great deviation between the ‘ground truth’ potential and the achieved herbicide reduction (cf. ). In this respect a high resolution should be implemented, but, as illustrated by others (e.g. Barroso et al., Citation2004), there is a cut-off level between the increased associated costs by increased resolution and the herbicide and monetary savings achieved through these increased resolutions (Rew & Cousens, Citation2001).
Figure 6. Mean simulated herbicide reductions (SHR) with 95% confidence interval bars of mean versus spraying resolution (boom width and image distance), for original threshold level (
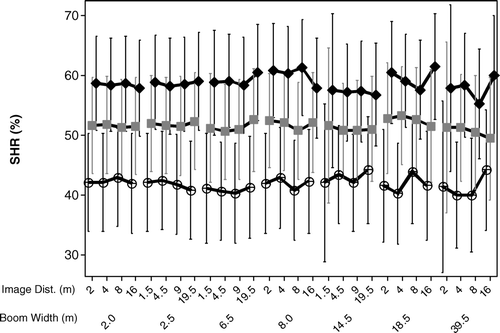
Considering the simulated herbicide reduction (SHR) at trial level, the weed infestation level per trial (results not shown) and corresponding PHR per trial were of course important (cf. ). However, the simulated herbicide reduction will also depend on how the current ‘patch morphology’ matches the implemented mapping and spraying resolutions (Johnson et al., Citation1995; Rew et al., Citation1996; Wallinga et al., Citation1998). In the present study this was illustrated by two trials with the same PHR values at the original threshold (33%). With control area being 39.5 m×16.0 m, the ‘patch morphology’ of trial No. 8 clearly coincided better with this resolution than that of trial No. 5, whereas with the smaller control area, 2 m×2 m, these trials’ ‘patchiness’ coincided equally well (cf. ). Carroll and Holden (Citation2005) suggested a method to specify the sprayer resolution based on the actual (grass) weed pattern, which could be suitable when target weed distributions were known pre-spraying. For practical real time patch spraying of young broad-leaved weeds, however, it is difficult to see how farmers could adjust equipment to the actual weed patterns.
Influence of the threshold level
For all practical purposes the tested levels of the threshold model were not influencing the magnitude of the mean spraying and mapping errors at given mapping and spraying resolutions, indicating that the tested threshold levels will be equally ‘safe’. However, when considering given resolutions for individual trials (size 632 m2), the influence of threshold level could be substantial, and seemed to depend on the actual infestation level (cf. and ). From these results we hypothesize that trials with WBT values outside the middle infestation range are least likely to be influenced by the threshold level because such trials, due to the generally strong autocorrelation in weed densities and spray decisions, have relatively small proportions of area with densities near the threshold. A change in thresholds of 25% is less likely to contribute to substantial change in these trials’ PHR values compared to trials with WBT values in the middle range. If the PHR value of a trial is not significantly affected by the threshold level, neither will the directly dependent SHR value be. If the SHR value does not differ substantially due to change in the threshold level, neither will the local spatial pattern of ‘spray’ and ‘not spray’ quadrats, and consequently not the mapping and spraying errors either. Previous results (Berge et al., 2007) have shown that the threshold level can affect the level of spraying and mapping errors also for larger trials (about 1–3.8 ha), indicating that the applied threshold level could be of importance for single seasons and relatively small fields. However, in the long run, or with increasing size of field, there are likely to be local areas with weed infestation level in the middle infestation range, where threshold level is affecting errors, and local areas with weed infestation level far below and far above threshold, e.g. at the field margins (cf. Cardina et al., Citation1997), where threshold level is not affecting errors. On average, these sub-field areas will probably counterbalance. For practical patch spraying of large fields with the one-camera system implemented, present results mean that it is the mapping and spraying resolution that controls the level of mapping and spraying errors (cf. and ), and the choice of threshold level must be founded on agronomic circumstances.
Figure 7. Illustration of the influence of threshold level on simulated herbicide reduction (SHR) per trial at two selected spraying resolutions: ○ = boom width 14.5 m and image distance 4.5 m. • = boom width 2 m and image distance 2 m. ‘SHR range’ is the absolute difference in SHR between the two threshold levels giving the lowest and highest SHR in the actual trial. Each trial is plotted according to its proportion of quadrats with weed infestation below the original threshold level (WBT).
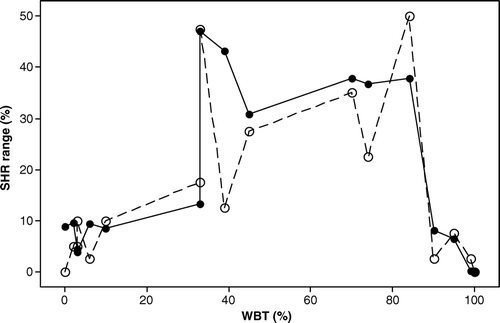
Acknowledgements
The Norwegian Institute for Agricultural and Environmental Research (BIOFORSK) is acknowledged for financial support. The authors also thank staff at BIOFORSK and The Norwegian Agricultural Extension Service for performing the fieldwork.
References
- Andreasen , C. , Rudemo , M. and Sevestre , S. 1997 . Assessment of weed density at an early stage by use of image processing . Weed Research , 37 : 5 – 18 .
- Backes , M. & Plümer , L. 2003 . On the adequacy of GIS-generated weed maps for precision farming . In K Virrantaus & H Tveite Proceedings 9th Scandinavian research conference on geographical information sciences (ScanGIS) , (pp. 261 – 268 ). Helsinki , Finland .
- Backes , M. , Schumacher , D. & Plümer , L. 2005 . The sampling problem in weed control: are currently applied sampling strategies adequate for site-specific weed control? In JV Stafford Precision Agriculture 2005. Papers presented at the 5th European conference on precision agriculture . (pp. 155 – 161 ). Uppsala , Sweden .
- Barroso , J. , Fernandez-Quintanilla , C. , Maxwell , B. D. and Rew , L. J. 2004 . Simulating the effects of weed spatial pattern and resolution of mapping and spraying on economics of site-specific management . Weed Research , 44 : 460 – 468 .
- Berge , T. W. , Fykse , H. and Aastveit , A. H. 2007 . Patch spraying of weeds in spring cereals: simulated influences of threshold level and spraying resolution on spraying errors and potential herbicide reduction . Acta Agriculturae Scandinavica, Section B, Soil and Plant Science , 57 : 212 – 221 .
- Black , I. D. and Dyson , C. B. 1993 . An economic threshold model for spraying herbicides in cereals . Weed Research , 33 : 279 – 290 .
- Cardina , J. , Johnson , G. A. and Sparrow , D. H. 1997 . The nature and consequence of weed spatial distribution . Weed Science , 45 : 364 – 373 .
- Carroll , J. P. and Holden , N. M. 2005 . A method to quantify weed distribution for relating to patch spraying systems . Transactions of the ASAE , 48 : 27 – 35 .
- Christensen , S. 1994 . Crop weed competition and herbicide performance in cereal species and varieties . Weed Research , 34 : 29 – 36 .
- Christensen , S. 2006 . Table I. Potential savings of herbicides in percentage of common practice in 59 trials with site-specific weed management in Europe . http://www.ewrs-sswm.org/homepage.htm , October 2006 .
- Cousens , R. and Croft , A. M. 2000 . Weed populations and pathogens . Weed Research , 40 : 63 – 82 .
- Cousens , R. D. , Brown , R. W. , McBratney , A. B. , Whelan , B. and Moerkerk , M. 2002 . Sampling strategy is important for producing weed maps: a case study using kriging . Weed Science , 50 : 542 – 546 .
- Dieleman , J. A. and Mortensen , D. A. 1999 . Characterizing the spatial pattern of Abutilon theophrasti seedling patches . Weed Research , 39 : 455 – 467 .
- Dille , J. A. , Milner , M. , Groeteke , J. J. , Mortensen , D. A. & Williams II , M. M. 2002 . How good is your weed map? A comparison of spatial interpolators . Weed Science , 51 , 44 – 55 .
- Fykse , H. 1991 . Damage thresholds for weeds in spring cereals . Statens fagtjeneste for landbruket-Faginfo 2 (Informasjonsm⊘te i plantevern) . (pp. 165 – 173 ). ( In Norwegian .)
- Fykse , H. 2004 . Herbicide resistance . Gr⊘nn kunnskap , 8 , 347 – 357 . ( In Norwegian .)
- Gerhards , R. , Nabout , A. , Sökefeld , M. , Kühbauch , W. & Eldin , H. A. N. 1993 . Automatic identification of 10 weed species in digital images using Fourier descriptors and shape parameters . Journal of Agronomy & Crop Science , 171 , 321 – 328 . ( In German .)
- Gerhards , R. , Sökefeld , M. , Timmermann , C. , Reichart , S. , Kühbauch , W. & Williams II , M. M. 1999 . Results of a four-year study on site-specific herbicide application . In JV Stafford Precision Agriculture1999, Part 2. Papers presented at the 2nd European conference on precision agriculture , 1999, Odense, Denmark . (pp. 689 – 697 ).
- Gerhards , R. , Sökefeld , M. , Nabout , A. , Therburg , R. D. & Kühbauch , W. 2002a . Online weed control using digital image analysis . Zeitschrift für Pflanzenkrankheiten und Pflanzenschutz (Sonderheft) , XVIII , 421 – 427 .
- Gerhards , R. , Sökefeld , M. , Timmermann , C. and Kühbauch , W. 2002b . Site-specific weed control in maize, sugar beet, winter wheat, and winter barley . Precision Agriculture , 3 : 25 – 35 .
- Gerhards , R. and Christensen , S. 2003 . Real time weed detection, decision making and patch spraying in maize, sugarbeet, winter wheat and winter barley . Weed Research , 43 : 385 – 392 .
- Gerhards , R. and Oebel , H. 2006 . Practical experiences with a system for site-specific weed control in arable crops using real time image analysis and GPS-controlled patch spraying . Weed Research , 46 : 185 – 193 .
- Gerowitt , B. and Heitefuss , R. 1990 . Weed economic thresholds in cereals in the Federal Republic of Germany . Crop Protection , 9 : 323 – 331 .
- Heijting , S. , Kruijer , W. , Stein , A. & van der Werf , W. 2005 . Species-specific spatial relations between weed patterns and soil characteristics . In Proceedings 13th European weed research society symposium , 2005, Bari, Italy , 2 pp.
- Johnson , G. A. , Mortensen , D. A. and Martin , A. R. 1995 . A simulation of herbicide use based on weed spatial distribution . Weed Research , 35 : 197 – 205 .
- Manh , A.-G. , Rabatel , G. , Assemat , L. and Aldon , M.-J. 2001 . Weed leaf segmentation by deformable templates . Journal of Agricultural Engineering Research , 80 : 139 – 146 .
- Mathworks Inc. , MATLAB, Version 7.0.1.24704 , US .
- Minitab Inc. , MINITAB Statistical Software, Release 14.20 for Windows, State College , Pennsylvania, , US .
- Nordbo , E. , Christensen , S. , Kristensen , K. and Walter , M. 1994 . Patch spraying of weeds in cereal crops . Aspects of Applied Biology – Arable farming under CAP reform , 40 : 325 – 334 .
- Onyango , C. M. and Marchant , J. A. 2003 . Segmentation of row crop plants from weeds using colour and morphology . Computers and Electronics in Agriculture , 39 : 141 – 155 .
- Paice , M. E. R. , Miller , P. C. H. and Day , W. 1996 . Control requirements for spatially selective herbicide sprayers . Computers and Electronics in Agriculture , 14 : 163 – 177 .
- Pérez , A. J. , López , F. , Benlloch , J. V. and Christensen , S. 2000 . Colour and shape analysis techniques for weed detection in cereal fields . Computers and Electronics in Agriculture , 25 : 197 – 212 .
- Rew , L. J. , Cussans , G. W. , Mugglestone , M. A. and Miller , P. C. H. 1996 . A technique for mapping the spatial distribution of Elymus repens, with estimates of the potential reduction in herbicide usage from patch spraying . Weed Research , 36 : 283 – 292 .
- Rew , L. J. , Miller , P. C. H. and Paice , M. E. R. 1997 . The importance of mapping resolution for sprayer control . Aspects of Applied Biology – Optimizing pesticide applications , 48 : 49 – 56 .
- Rew , L. J. and Cousens , R. D. 2001 . Spatial distribution of weeds in arable crops: are current sampling and analytical methods appropriate? . Weed Research , 41 : 1 – 18 .
- SAS Institute Inc. , SAS/STAT version 8.1, Cary , North Carolina, , US .
- Scotford , I. M. and Miller , P. C. H. 2005 . Applications of spectral reflectance techniques in northern European cereal production: a review . Biosystems Engineering , 90 : 235 – 250 .
- Stafford , J. V. and Miller , P. C. H. 1993 . Spatially selective application of herbicide to cereal crops . Computers and Electronics in Agriculture , 9 : 217 – 229 .
- Steward , B. L. and Tian , L. F. 1999 . Machine-vision weed density estimation for real time, outdoor lighting conditions . Transactions of the ASAE , 42 : 1897 – 1909 .
- S⊘gaard , H. T. 2005 . Weed classification by active shape models . Biosystems Engineering , 91 : 271 – 281 .
- Sökefeld , M. , Gerhards , R. & Kühbauch , W. 2000 . Site-specific weed control – from weed recording to herbicide application . Zeitschrift für Pflanzenkrankheiten und Pflanzenschutz (Sonderheft) , XVII , 227 – 232 . ( In German .)
- Tian , L. 2002 . Development of a sensor-based precision herbicide application system . Computers and Electronics in Agriculture , 36 : 133 – 149 .
- Timmermann , C. , Gerhards , R. and Kühbauch , W. 2003 . The economic impact of site-specific weed control . Precision Agriculture , 4 : 249 – 260 .
- Wallinga , J. , Groeneveld , R. M. W. and Lotz , L. A. P. 1998 . Measures that describe weed spatial patterns at different levels of resolution and their applications for patch spraying of weeds . Weed Research , 38 : 351 – 359 .
- Wilkerson , G. G. , Wiles , L. J. and Bennett , A. C. 2002 . Weed management decision models: pitfalls, perceptions, and possibilities of the economic threshold approach . Weed Science , 50 : 411 – 424 .
- Zadoks , J. C. , Chang , T. T. and Konzak , G. F. 1974 . A decimal code for the growth stages of cereals . Weed Research , 14 : 415 – 421 .