ABSTRACT
The freely available European Parliament Proceedings Parallel Corpus, or Europarl, is one of the largest multilingual corpora available to date. Surprisingly, bibliometric analyses show that it has hardly been used in translation studies. Its low impact in translation studies may partly be attributed to the fact that the Europarl corpus is distributed in a format that largely disregards the needs of translation research. In order to make the wealth of linguistic data from Europarl easily and readily available to the translation studies community, the toolkit ‘EuroparlExtract’ has been developed. With the toolkit, comparable and parallel corpora tailored to the requirements of translation research can be extracted from Europarl on demand. Both the toolkit and the extracted corpora are distributed under open licenses. The free availability is to avoid the duplication of effort in corpus-based translation studies and to ensure the sustainability of data reuse. Thus, EuroparlExtract is a contribution to satisfy the growing demand for translation-oriented corpora.
1. Introduction
Recent years have witnessed a growing influence of mono- and multilingual corpora not only in translation practice but also in theoretical and applied translation studies (TS). While even among advocates of this trajectory there is no consensus on whether corpus-based translation research is a well-established and independent paradigm in TS (most recently, e.g. Fantinuoli & Zanettin, Citation2015, p. 1; Hu, Citation2016, pp. v, 8, 26) or only a useful research method that still lacks robustness (e.g. Li, Citation2017, p. 132), in terms of research output the impact of linguistic corpora is clearly noticeable and a trend that is likely to continue (Zanettin, Saldanha, & Harding, Citation2015, p. 180). Therefore, it comes as no surprise that there is demand for more translation-oriented corpora, both large, varied ones (i.a. Mikhailov & Cooper, Citation2016, pp. 1–2; Zanettin, Citation2013, p. 30) and small but tightly controlled ones (Malamatidou, Citation2018; Zanettin, Citation2013, p. 31). What is surprising, though, is that one of the largest multilingual parallel corpora available to date, the European Parliament Proceedings Parallel Corpus (Koehn, Citation2005), or Europarl, has received relatively little attention in TS, as evidenced by the exploratory bibliometric analyses in section 2 of the present paper. After all, corpora in as many languages as possible and not restricted to literary texts only are needed to increase the generalisability of findings in corpus-based TS (Hu, Citation2016, pp. 227–232; Olohan, Citation2004, p. 190–191), thus reducing language-pair and genre bias of theoretical claims about translation. In view of its free availability, size, linguistic diversity, data authenticity, sentence-aligned architecture as well as homogeneity in terms of register, text type and subject domain, Europarl is a prime candidate for translation-oriented corpus inquiries. In order to promote and facilitate its use in corpus-based TS, this paper presents EuroparlExtract, a corpus processing toolkit for the extraction of bilingual parallel subcorpora, as well as mono- and bilingual comparableFootnote1 subcorpora, from the Europarl corpus. The toolkit aims to maximise the utility of Europarl, which, in its original release, is of limited value for translation research due to the inability to compile comparable subcorpora and so-called ‘directional’ subcorpora (Cartoni & Meyer, Citation2012, p. 2133), i.e. parallel corpora where the source and target languages are clearly identified for each language pair. In other words, EuroparlExtract addresses the disregard for translation directionality in the original Europarl corpus, as well as its inclusion in larger multi-corpus collections and query tools such as the Open Source Parallel Corpus (Tiedemann, Citation2012) or Sketch Engine (Kilgarriff et al., Citation2014). Europarl’s disregard for directionality manifests in bilingual subcorpora that not only mix up translation directions without making the actual source and target languages explicit, but also contain text pairs in which both the purported source and target text are, in fact, translations from another language (see section 3.1).
EuroparlExtract is driven by the aim of making the wealth of linguistic data contained in the Europarl corpus easily available to researchers and students in the field of TS and contrastive linguistics, even if they lack programming skills. It is therefore conceived as an addition to Europarl that aims to lower the barrier to corpus-based TS. Potential fields of application may include, for instance, translation teaching, but also research into the distinctive characteristics of translated texts (translation universals, translationese, language-specific or text-specific shining-through effects, etc.), contrastive issues (cross-linguistic correspondence and variation, directionality effects, etc.), translation quality or domain-specific phenomena in political, economic and legal texts (terminology, phraseology, etc.).
2. Motivation
Being one of the largest multilingual parallel corpora, the freely available Europarl corpus is used extensively in natural language processing (NLP, including computational linguistics and human language technology), most prominently in the field of machine translation. By contrast, the corpus has been far less influential in TS, despite the rise of data-driven research methods. Islam and Mehler (Citation2012, p. 2506) even claimed that Europarl has not been used at all in TS; this claim is somewhat mitigated by the following bibliometric analyses.
While mentions of Europarl in authoritative reference works on TS (e.g. Kenny, Citation2009, p. 61) and in dedicated introductions to corpus-based TS (e.g. Hu, Citation2016; Mikhailov & Cooper, Citation2016; Zanettin, Citation2012) show that the corpus is known among translation scholars, only a very limited number of studies using Europarl data has been conducted so far. Thus, in the Translation Studies Bibliography (Gambier & van Doorslaer, n.d.) there are only 18 publications that make reference to Europarl in the title or abstract. Similarly, a query in the Bibliography of Interpreting and Translation (BITRA) (Franco Aixelá, Citation2001-Citation2017) returns 15 publications mentioning Europarl. According to the impact summary provided by BITRA, Europarl has been cited by only nine publications. Taken together, the consulted bibliographies suggest, irrespective of their inherently incomplete coverage (Grbić, Citation2013, p. 21), that Europarl has had a noticeable yet relatively limited impact in TS.
As a complementary perspective to the query in manually curated TS bibliographies, a citation analysis based on Google Scholar data provides further evidence for the limited use of Europarl in corpus-based TS. At the time of writing (April 2018), Google Scholar lists 2,350 citations to Koehn’s (Citation2005) publication of the Europarl corpus. In order to estimate how many of these citations can be attributed to the scholarly field of TS, the bibliographic information of the publications citing Koehn’s paper was retrieved with the free bibliometric software Publish or Perish (Harzing, Citation2016). Google Scholar was given preference over alternative data sources supported by the software (Scopus, Web of Science, Crossref, Microsoft Academic), because it is the most appropriate source for citation comparisons across disciplines (Harzing, Citation2011, p. 237) and because it has by far the highest coverage in the humanities (Harzing & Alakangas, Citation2017), which reflects the other sources’ limited coverage of non-journal publications (Harzing & Alakangas, Citation2017). One disadvantage of Google Scholar is that it limits the results of any query to the 1,000 top-cited items (Harzing, Citation2011, 173). Thus, the retrieved sample of works citing Europarl is potentially biased against TS publications and towards high-impact NLP publications. Upon retrieval of bibliographic data, all citing works from the sample were manually classified into scholarly fields according to the editorial information provided online (e.g. in the aims and scope section of journal websites). The classification scheme was determined inductively by the author of this paper, taking into account the main scope of the respective journals, volumes or book series. Conference proceedings were classified according to the main conference topic, and theses according to authors’ affiliations. Ambiguous cases, such as interdisciplinary and multi-thematic publications, were resolved by choosing the subject of publications as specified by publishers’ subject catalogues. In short, it was not the topic of the citing works that was decisive for classification, but the addressed research community. For example, papers on computer-assisted translation would be assigned to TS if published in Perspectives – Studies in Translation Theory and Practice and to NLP if published in the proceedings of the Annual Meeting of the Association for Computational Linguistics. This procedure yielded seven scholarly fields to which Europarl citations can be assigned:
NLP, including human language technology, computational linguistics and artificial intelligence
TS
contrastive linguistics
terminology and lexicography
corpus linguistics
other linguistic fields, e.g. theoretical linguistics, general linguistics, language teaching, English linguistics, psycholinguistics, etc.
other academic fields, e.g. psychology, political science, human–computer interaction, library science, digital humanities, general science, etc.
The fully classified sample of citing works, including the rules for coding the works into categories and explanations for borderline cases, is provided as supplementary material. As shown in , the vast majority (92.1%) of the 1,000 analysed works were published in NLP publications. TS accounts only for 1.9% of the citations; in TS is grouped with contrastive linguistics, corpus linguistics and terminology/lexicography (3.8% of all citations), because on the one hand it is known that translation scholars do not publish exclusively in dedicated TS journals or series (Grbić, Citation2013, p. 21) and on the other hand it is hard to draw clear-cut boundaries between TS and neighbouring disciplines – note that, for instance, TS and contrastive linguistics can be regarded as two cooperative fields with increasing mutual cross-fertilization (Vandepitte & De Sutter, Citation2013).
Figure 1. Percentage of citations of Europarl according to field of study. Total number of citations = 1,000.
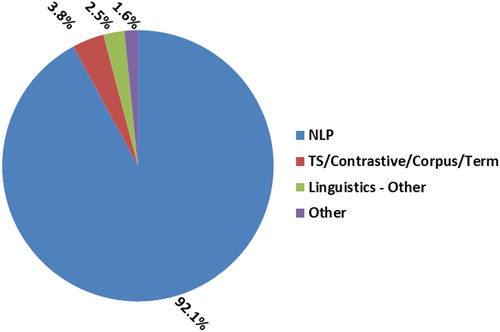
Even though the sample of Europarl citations is skewed and the classification of numerous interdisciplinary publications into distinct scholarly fields is a gross simplification, the bibliographic analyses provide quantitative evidence that the Europarl corpus has played only a minor role in corpus-based TS and related fields, whereas in NLP it is one of the most influential resources of recent years.
But why is it that Europarl is infrequently used in TS? One possible explanation is that the corpus contains several types of recurring and frequent errors (Graën, Batinic, & Volk, Citation2014). Unlike NLP, where sheer corpus size may compensate for noise in the data, corpus-based TS (and corpus linguistics in general) usually prioritises data quality over data quantity. Another explanation is that Europarl has been explicitly designed and developed for statistical machine translation. Owing to that, the directionality of language pairs is not taken into account in the corpus, because the standard practice in the training of machine translation systems is to use all available bilingual data for a given language pair, irrespective of which of the two languages is the actual source language (Kurokawa, Goutte, & Isabelle, Citation2009, p. 85). It goes without saying that the disregard for translation directions is a serious shortcoming from the perspective of TS. However, information about the original language of texts actually is contained in Europarl source files in the form of metadata tags, but is ignored in the procedure employed by Koehn (Citation2005) to generate bilingual parallel subcorpora for individual language pairs. Consequently, the information about translation direction is implicitly given in Europarl, but making it explicit is not entirely straightforward due to the architecture of the Europarl distribution (see section 3.1) and recurring metadata mark-up errors identified in Europarl by Graën et al. (Citation2014). Thus, the extraction of Europarl subcorpora that do account for directionality requires corpus pre-processing and programming skills. In order to avoid the duplication of effort in corpus-based TS, the EuroparlExtract toolkit aims to provide the research community with a freely and readily available Europarl distribution that includes the two fundamental types of translational corpora, viz. comparable and directional parallel corpora, thus meeting the requirements of translation and contrastive research.
3. Related work
3.1. The original Europarl corpus
The Europarl corpus was obtained by automatically harvesting the proceedings of the European Parliament from its website.Footnote2 The proceedings contain the edited and revised transcripts of all speeches delivered in plenary debates by the members of the European Parliament in their native languages, as well as the translations of the transcripts into all other official languages of the European Union (EU) (Bernardini, Ferraresi, & Miličević, Citation2016, pp. 68–69). In the Europarl corpus, the transcripts and translations for each day of debate are stored in one monolingual plain text file per language. The files (187,720 in total) also contain metadata tags indicating the identity of speakers and in which language statements were originally uttered. Thanks to the speaker identifiers, it is possible to match statements that correspond to each other across languages. shows examples of corresponding statements from three Europarl files; the original language of transcribed statements and the source language of translated transcripts are indicated as ISO 639-1 two-letter codes in the LANGUAGE attribute of the SPEAKER tags: Example 1 is a speech originally given in Spanish and subsequently translated into German and English, respectively, whereas in Example 2 all three language versions are translations from an original speech in French.
Table 1. Samples of the German, English and Spanish monolingual Europarl files ep-11-05-09-018.txt (= proceedings of chapter 18 from May 9, 2011). Information about speaker and original language in metadata tags.
Apart from the monolingual source files, Europarl provides a set of pre-processing tools for the extraction of sentence-aligned bilingual parallel corpora. However, a major drawback is that the resulting corpora do not account for directionality. Take the English–German corpus, for instance, where no distinction between translations from English into German and the opposite direction is made and where large portions of text are not translations of each other but of other languages, such as the German and English samples in originally uttered in Spanish and French, respectively.
The latest version of Europarl (v7),Footnote3 on which this paper is based, covers the period from 1996 to 2012. It includes 21 languages, which corresponds to the 23 official EU languages at the time of release, excluding Maltese and Gaelic. According to the Europarl website, which lacks figures for Bulgarian and Greek, the corpus totals around 600 million words across all languages, with amounts of monolingual data ranging from 7 million (Polish) to almost 55 million (Spanish) words. With the provided pre-processing tools, up to 210 non-directional bilingual parallel corpora can be generated.
3.2. Adaptations of the Europarl corpus
A number of researchers have adapted the Europarl corpus for specific purposes in order to further enhance its usefulness and to compensate for some of its drawbacks. Graën et al. (Citation2014) have discovered and quantified various types of systematic errors that partly stem from the data-crawling and -processing procedures applied by Koehn (Citation2005) and partly from imperfections in the European Parliament website. The most frequent error type is incorrectly coded metadata, e.g. information about speakers or the original language of speakers’ statements. As a matter of fact, Graën et al. (Citation2014, p. 225) estimated that half of all language identifiers erroneously appear within text segments instead of metadata mark-up tags. This is especially important with regard to the exploitation of Europarl in TS, because language identifiers, even improperly coded ones, are fundamental to the extraction of directional and comparable corpora, as outlined in section 4.1. Based on the error analysis, Graën et al. (Citation2014) corrected several error types and additionally enriched the corpus. Their cleaned and structurally enriched version of the Europarl corpus is stored in XML format and made freely available as the Corrected & Structured Europarl Corpus (CoStEP),Footnote4 mainly for the purpose of NLP tasks, such as part-of-speech tagging or parsing, and linguistic research in general.
Motivated by the observation that many existing multilingual and parallel corpora, including Europarl, are not suitable for TS, Islam and Mehler (Citation2012) addressed the disregard for directionality in Europarl (see section 3.1) by providing a customised version of Europarl. Contrary to CoStEP, they did not aim to clean and enrich Europarl, but to extract directional parallel data from it. The resulting corpusFootnote5 is encoded in the Text Encoding Initiative (TEI) format and comprises 414 sentence-aligned language pairs.
Along the same line, Cartoni and Meyer (Citation2012) extracted directional corpora from Europarl, too. In addition, they extracted comparable corpora that can be used to contrast (a) texts originally produced in a particular language with texts translated into that language (e.g. translated English vs non-translated English), and (b) translated texts in a particular language from different source languages (e.g. English translated from French vs English translated from German, and so on). The resulting Europarl-direct corpusFootnote6 comprises 14 directional subcorpora for seven language pairs provided in plain text format. An important aspect of their work is that they addressed the problem of incomplete metadata about the actual source language of speeches (see also the paragraph on related work carried out by Graën et al., Citation2014) by extending available language identifiers to speeches that lack these identifiers. Since metadata about texts’ source languages is essential for the compilation of directional and comparable corpora, this metadata normalisation procedure increases the recall of text data during subcorpus extraction.
The three papers presented above share the same general idea of restructuring and redistributing Europarl data in customised formats. This is mainly achieved by normalising inconsistent or incomplete and exploiting untapped (meta-)data from the original Europarl distribution. EuroparlExtract, which will be explained in more detail in the following section, is similar to these three papers in that it has the same aims and partly takes similar approaches to data extraction. However, there are some important differences that provide added value: From the strategic point of view, EuroparlExtract maximises the recall of text data for all subcorpora, and thus their size, in order to get the most out of Europarl; and from a technical point of view it gives users more flexibility to customise extracted subcorpora in terms of language pair selection and output formats.
4. Subcorpus extraction method
4.1. Source language identification and statement matching
This section describes how the EuroparlExtract toolkit uses metadata about the original language of Europarl speeches in order to extract comparable and directional subcorpora. The guiding principle behind the method is to gather as much relevant data as possible for each subcorpus, i.e. to maximise precision and recall of the extraction procedure. The reason for this decision is that Europarl contains data even for small languages and less common language pairs (e.g. Hungarian–Latvian) that are otherwise under-resourced in terms of corpus availability. Since data for these languages and language pairs is comparatively scarce in Europarl, it makes sense to maximise the amount of extracted data.
The necessary precondition for subcorpus extraction is the identification of the original language of statements in monolingual Europarl files. However, a major problem of Europarl is that a large number of statements have no metadata about the original language attached. Thus, in Europarl v7 there are 2,831,433 speaker identifiers across all 21 languages, but only 1,181,701 (41.7%) of them have non-empty language tags. Fortunately, for many statements without a proper language tag the language information can be found in corresponding statements in another language file. For instance, in Example 1 of information about English being the original language is missing for the English and Spanish versions but is available in the German translation. The first step of the source language identification is thus to collect the language information from all speaker identifiers in all Europarl files in order to extend the available language identifiers to speaker identifiers without proper language tags. To this end, corresponding statements need to be matched across Europarl language files according to speaker identity and date of debate. This idea has been implemented by Cartoni and Meyer (Citation2012), too. Although this procedure largely increases the number of language-tagged statements, it does not fully exploit source language information available in Europarl: Frequently, language information is not indicated in the SPEAKER metadata tags, but erroneously appears in parentheses within the speeches (see also Graën et al., Citation2014, 223). These parenthesised language tags may either occur in addition to one or more proper language identifier for corresponding statements (see Example 2 of ), or be the sole language information if none of the language files contains a proper language identifier in the SPEAKER tag (Example 3 of ). Contrary to Cartoni and Meyer (Citation2012),Footnote7 Europarl-Extract capitalises on parenthesised language tags as well, which helps to further increase the number of statements with clearly identified source languages. An evaluation of this procedure is provided in section 5.2.
Table 2. Examples of inconsistent and incorrectly encoded source language identifiers in Europarl source files.
One difficulty in exploiting parenthesised tags is that for some Slavic languages the string ‘(ES)’ in speeches does not necessarily represent the language code for Spanish, but may also be part of titles of regulations and directives, as in the Czech example nařízení (ES) č. 562/2006 ‘Regulation (EC) No 562/2006’. Regular expressions were used to filter out such exceptions, thus avoiding incorrect source language identification.
The second step of the procedure consists in finding corresponding statements across languages by matching speakers’ names, rather than speaker IDs only. This step is necessary because the IDs are not perfectly matched across Europarl source files, which means that statements with the same ID number are not necessarily multilingual correspondences of each other but may have been uttered by different people. Matching speaker names is not entirely straightforward because of frequent misspellings and formatting errors, inconsistent naming conventions, multiple speaker names, the parallel use of the Latin, Greek and Cyrillic alphabets, as well as multilingual variants of the denomination of the title ‘President’ (see ). Consequently, simple string matching would not yield satisfactory results and would considerably decrease the recall of corresponding statements. Therefore, EuroparlExtract internally normalises speaker names (lowercasing; removal of punctuation, blanks and diacritics; normalisation of ligatures; Latinisation of Cyrillic and Greek characters; unification of multilingual president titles) and subsequently compares them using a simple edit-distance-based heuristic. Eventually, only statements that match each other across languages according to both ID and normalised speaker name are identified as corresponding statements in order to avoid noise in the data.
Table 3. Examples of inconsistencies in speaker names that require normalisation prior to statement matching.
The third and final step of source language identification is to resolve ambiguous source language information, i.e. cases of corresponding statements for which the first step of the procedure identified contradictory language tags across Europarl files, by majority voting: Each statement is assigned the language code whose count accounts for more than 60% of all language codes found for that statement in the proper and parenthesised language tags. The result of the source language identification and statement matching procedure is an internal representation of the metadata associated with all Europarl statements. The representation can be exported in comma-separated values (CSV) format and serves as the basis for subsequent subcorpus extraction.
4.2. Generation of output
4.2.1. Command line interface
Building upon the internal representation of Europarl statements described above, all statements relevant to particular subcorpora of choice are extracted from Europarl source files and stored as individual files in dedicated folders that indicate the translational status and language direction of texts. EuroparlExtract has a command line interface that allows users to specify corpus type (parallel or comparable), language combinations and output formats. In this way, subcorpora tailored to users’ specific needs can be extracted without any programming. EuroparlExtract is implemented in Python (version 3) and is freely availableFootnote8 under an open source license.
As far as parallel corpora are concerned, data can be extracted either for selected language combinations individually or for all supported language directions in one go. The following output formats can be specified: sentence-aligned Translation Memory eXchange (TMX) files, sentence-aligned plain text files and non-aligned plain text files. Contrary to parallel corpora, comparable corpora consist of individual monolingual files in the chosen language(s) rather than of (aligned) bilingual bitexts. Comparable corpora can be extracted either for selected languages or for all supported languages at once.
4.2.2. Sentence alignment
EuroparlExtract uses Tan and Bond’s (Citation2014) implementation of the Gale–Church algorithm (Gale & Church, Citation1993) for parallel sentence alignment. To ensure the quality of the extracted data, statements with diverging numbers of paragraphs between source and target text are omitted in sentence alignment, which is consistent with the method applied by Koehn (Citation2005, p. 81). Since the Gale–Church algorithm tends to produce incorrect alignments when portions of source and target texts differ strongly in terms of sentence segmentation (which may be the case if translators split one very long source sentence into many shorter target sentences), the sentence alignment procedure of EuroparlExtract omits all paragraphs that have more than twice the number of sentences of their respective counterpart in either source or target language. Although this restrictive measure reduces the quantity of sentence-aligned text in the extracted corpora, it minimises incorrect alignments and thus increases data quality, which is of paramount importance for research purposes. A further drawback of the alignment algorithm is that it occasionally produces empty alignments, i.e. sentences without matched counterparts. Given that such empty alignments result from alignment errors, a post-processing procedure merges unmatched segments at the beginning or end of paragraphs with adjacent segments to improve the alignment quality. All remaining cases of incorrectly produced empty alignments are discarded.
Note that no data are discarded if the non-aligned output format is chosen for parallel corpus extraction. This format may be the better choice, for instance, if the maximum possible amount of parallel data is to be extracted or if the research focus is on segmentation differences between source and target texts.
5. Results of extraction
5.1. Estimation of statement matching accuracy
As outlined in section 4.1, EuroparlExtract aims to maximise recall and the precision of the extraction. The most error-prone part of the method is the identification of corresponding statements across languages based on normalised speaker names, which determines whether statements with identical IDs but divergent speaker names can be attributed to the same speaker irrespective of spelling variants (see ), or if they have been uttered by different speakers and should therefore be discarded rather than treated as corresponding statements. Across all Europarl language files there are 66,743 statements with identical speaker IDs but ambiguous speaker names, of which the EuroparlExtract heuristic disambiguated 54,978 as matching and discarded 11,765 as non-matching statements. Since there is no gold standard available to determine the accuracy of the matching heuristic, a manual evaluation based on the exported metadata representation file (see section 4.1) has been carried out. From the 54,978 disambiguated statements, a random sample of 3,000 statements (= 5.5%) was drawn from the CSV file. Out of these manually examined statements, only four were erroneously classified as matching statements by the matching heuristic, although in reality they were uttered by different speakers. Hence, there were only four false positives, which equals an error rate of 0.13%. Assuming this error rate for the whole set of statements with ambiguous speaker names, there are only 73 false positives in the entire corpus, which is a satisfactory result in view of the simplicity of the heuristic and overall size of the corpus. False negatives, i.e. statements discarded by the heuristic despite being corresponding statements, have not been evaluated, because from the perspective of data quality precision is more of a concern than recall.
5.2. Description of extracted corpora
quantifies the output of the method described in section 4.1. Most importantly, the exploitation of parenthesised language identifiers in addition to proper language identifiers largely increases the number of statements that qualify for extraction, i.e. statements with language tags and unambiguously matched speaker identity: from 129,204 to 167,302 (+ 29.5%). Ultimately, 78.1% of all distinct Europarl statements meet the requirements for extraction.
Table 4. Number of Europarl statements yielded by source language identification and speaker matching procedure.
From the entire Europarl corpus, EuroparlExtract is able to extract 420 directional parallel subcorpora, i.e. all language combinations contained in Europarl. With regard to comparable corpora, two corpus sections can be distinguished for each language: one containing only texts originally produced in a given language, and one containing only texts translated into that language; the latter can be further subdivided according to source languages. In the translated section of comparable corpora, only the target side of each language combination is extracted, while information about the source language is used as metadata. Non-translated data can be extracted for 21 languages and translated data for 462 language combinations. Total sizes of the extracted corpora are shown in . Interestingly, the difference in size between the aligned and non-aligned parallel corpus is only 4.6%, although due to data quality reasons the alignment procedure discards speeches and paragraphs unequally segmented across languages (see section 4.2). This indicates the overall quality of structural mark-up in Europarl on the one hand, and that Europarl translations tend to adhere to source text segmentation on the other.
Table 5. Total sizes of extracted subcorpora in tokens. For parallel corpora, only target language tokens are counted.
Due to the large number of language combinations in the extracted corpora, no breakdown of corpus size per language combination is presented here.Footnote9 Instead, network visualisations of text quantity per language direction, generated with the Gephi software package (Bastian, Heymann, & Jacomy, Citation2009), are shown in and .
Figure 2. Text quantity in parallel subcorpus by languages. Node size and colour proportional to number of tokens translated into given language; weight and colour of directed edges proportional to number of tokens translated from given language.
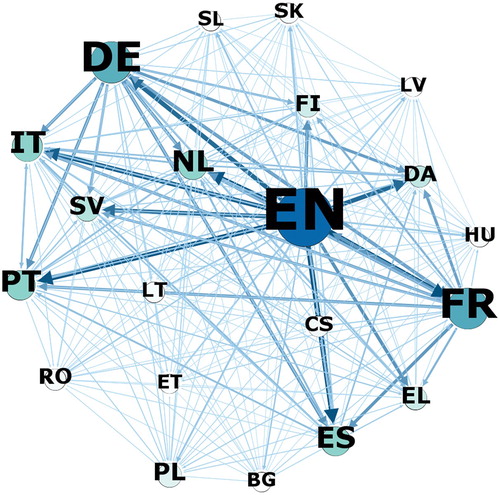
As expected, the languages of countries that joined the EU at later enlargement rounds are less represented in the corpus, while English, French and German are most prominent. The two ‘outliers’ located at the margins of stand for Gaelic and Maltese, for which no text data is available in Europarl. However, a total of 1,270,963 and 920,258 tokens, respectively, translated from these two languages into the remaining 21 EU languages can be found in the translated section of the comparable subcorpus.
The two subcorpora accumulate a huge amount of data in hundreds of language directions; in the highly compressed .tar.gz format, the entire parallel and comparable corpus occupies 2.6 GB and 1.5 GB of disk space, respectively. Both of them are freely and readily available for download.Footnote10 Given that such large files and data quantities may be impractical to handle on the one hand, and that users are not necessarily interested in all the data on the other, users may prefer to extract data directly from the Europarl source release on demand according to their own requirements via the command line interface of Europarl Extract. This brings the additional advantage of having the possibility to customise the data in terms of output formats. EuroparlExtract comes with detailed step-by-step instructions, as well as all required pre- and post-processing tools, two of which – the sentence splitter and tokeniser – are based on third-party open-source software (Agerri, Bermudez, & Rigau, Citation2014; Koehn, Citation2005). Currently, EuroparlExtract is optimised for Linux; support for other operating systems is planned in the future.
5.3. Comparison with related work
The main differences that distinguish EuroparlExtract from the related work reviewed in section 3.2 can be summarised as follows. First, Islam and Mehler (Citation2012) did not extract comparable corpora, and their parallel corpora covered only 414, as compared to 420, language directions. In terms of size, their corpus totals approximately 86 million tokens, while EuroparlExtract is able to extract almost 480 million tokens of sentence-aligned data. Although the authors did not detail their extraction method, it appears that they did not capitalise on parenthesised language tags to maximise recall, which may explain the large difference in size. Apart from that, the only metadata available in their corpus is information about language directions, which means that it is impossible to assign portions of texts in a given language direction to particular speeches, speakers or dates. Thus, valuable information is not carried over from Europarl to the extracted data.
Second, with the aim of cleaning and restructuring Europarl, CoStEP (Graën et al., Citation2014) has different yet partly overlapping aims. Notable methodological similarities between EuroparlExtract and CoStEP are the use of parenthesised language tags to maximally exploit available source language information, and the cross-linguistic detection of corresponding statements based on speaker names. The main difference is that CoStEP does not provide readily extracted comparable or parallel subcorpora, but requires using the XPath query language to identify relevant portions of data.
Finally, Europarl-direct is the work most similar to EuroparlExtract, especially in terms of the main target group and their data needs. However, Cartoni and Meyer (Citation2012) did not exploit parenthesised language tags, because they did not aim to maximise the quantity of extracted data. A quantitative comparison with EuroparlExtract data is not sensible because their extraction was based on an older and smaller version of Europarl (v6) and because their extracted corpora only cover the period from 1996 to 1999 for five language pairs. A further difference is that with EuroparlExtract users can compile and customise subcorpora on their own, whereas Europarl-direct offers additional language pairs only upon request.
6. Conclusion
Recently, advanced multivariate quantitative data analysis (e.g. De Sutter, Lefer, & Delaere, Citation2017; Ji, Oakes, Defeng, & Hareide, Citation2017; Mellinger & Hanson, Citation2017; Oakes & Ji, Citation2012) has been gaining momentum and thus promoting methodological innovation in (corpus-based) TS. Similarly, more comprehensive frameworks for the combination of different corpus types, as well as qualitative and quantitative methods, have emerged (Malamatidou, Citation2018). One of the keys to the fruitful application of state-of-the-art research methods is the availability of relevant, real-world translation data for a wide range of languages and text varieties. While building new corpora is one solution to satisfy the demand for translation corpora, the re-use and enrichment of existing resources is an efficient and less costly alternative. Despite a number of disadvantages, the highly multilingual Europarl corpus contains large quantities of relevant non-literary data for translation and contrastive research. Nevertheless, its impact on TS and contrastive linguistics has been merely moderate. The EuroparlExtract toolkit aims to facilitate the use of this resource by providing programming-free and open access to Europarl in a form that makes better use of translation-specific metadata. Depending on the underlying research questions, part or all of the extracted data may be used either as the sole or as complementary sources for corpus inquiry. Methodologically, the extracted subcorpora may be employed in research designs that pursue increased rigour by means of advanced statistics and/or by combining large-scale quantitative analyses with in-depth qualitative analyses of smaller corpora (Zanettin, Citation2013, p. 31); for example, within the framework of corpus triangulation (Malamatidou, Citation2018). Exploratory analyses of a qualitative nature could benefit from Europarl as well, because, due to its size, it may capture less frequent domain-specific phenomena. Therefore, the use of EuroparlExtract is a priori not restricted to particular research designs.
The major strength of EuroparlExtract is that it focuses on maximising the amount of extracted data by combining various sources of metadata from Europarl. At the same time, measures that decrease data quantity, but in exchange increase data quality, are taken. In this way, relevant research data, including under-resourced language pairs, is made more accessible to corpus-based TS. Customisation options and the support of various output formats are included to ensure the usability of the toolkit for users in the field of TS. Regarding the limitations of EuroparlExtract, an observation by Cartoni and Meyer (Citation2012, p. 2134) needs to be mentioned: In Europarl there is no reliable information as to which texts have been translated directly from one language to another and which are the result of indirect translations via pivot languages. But even so, the coexistence of direct and indirect translations in Europarl may open new lines of research, because to date the vast majority of research into indirect translation has dealt with literary translation, largely neglecting non-literary texts (Pięta, Citation2017, p. 200). A further limitation of EuroparlExtract is that the implemented third-party tool for sentence alignment struggles with unequally segmented source-target pairs, which is why some interesting and relevant text data have to be discarded for quality reasons. An improvement of the alignment procedure is left for future work.
In sum, EuroparlExtract is concerned with the sustainability of corpus exploitation. It is hoped that the re-use of Europarl will ease the demand for new translation-oriented multilingual corpora and thus help spur data-driven translation research.
Supplemental Material
Download Comma-Separated Values File (239.6 KB)Supplemental Material
Download Comma-Separated Values File (1.8 KB)Acknowledgments
The author thanks the two anonymous reviewers for their thoughtful comments and suggestions for improvement of the manuscript.
Disclosure statement
No potential conflict of interest was reported by the author.
Data availability statement
The data extracted with the tool presented in this article are available in Zenodo at https://zenodo.org/record/1066474#.WnnEM3wiHcs (DOI: 10.5281/zenodo.1066473) and https://zenodo.org/record/1066472#.WnnEYXwiHcs (DOI: 10.5281/zenodo.106647). These data were derived from the European Parliament Proceedings Parallel Corpus (www.statmt.org/europarl). The data supporting the bibliometric analyses presented in this article are available within the supplementary materials.
Notes on contributor
Michael Ustaszewski is assistant professor in the Department of Translation Studies at the University of Innsbruck, Austria. His research interests include corpus-based translation studies, translation technology and third language acquisition. He is principal investigator of the corpus-building project TransBank – A Meta-Corpus for Translation Research.
ORCID
Michael Ustaszewski http://orcid.org/0000-0002-2000-5920
Additional information
Funding
Notes
1 The terminology adopted in this paper follows the corpus typology suggested by Olohan (Citation2004, pp. 24–44) and Zanettin (Citation2012, pp. 10–11).
3 Freely available from www.statmt.org/europarl, where previous versions of the corpus and the version history can be found as well.
4 Freely available from http://pub.cl.uzh.ch/purl/costep.
5 Freely available from www.texttechnologylab.org/applications/corpora/customized-europarl-corpus under the CC BY-SA 4.0 DE license.
6 Available upon registration from www.idiap.ch/dataset/europarl-direct under a free research-only license.
7 I would like to thank Thomas Meyer for clarifying the details of their extraction procedure in personal communication via email.
8 The source code and documentation can be found at github.com/mustaszewski/europarl-extract.
9 Detailed figures for all subcorpora and all language combinations can be found in the documentation of EuroparlExtract at https://github.com/mustaszewski/europarl-extract.
10 See https://zenodo.org/record/1066474#.WnnE43wiHcs for the parallel and https://zenodo.org/record/1066472#.WnnE8XwiHcs for the comparable subcorpus.
References
- Agerri, R., Bermudez, J., & Rigau, G. (2014). IXA pipeline: Efficient and ready to use multilingual NLP tools. In N. Calzolari, K. Choukri, T. Declerck, H. Loftsson, B. Maegaard, J. Mariani, … S. Piperidis (Eds.), Proceedings of the 9th international conference on language resources and evaluation (pp. 3823–3828). Reykjavik: European Languages Resources Association.
- Bastian, M., Heymann, S., & Jacomy, M. (2009). Gephi: An open source software for exploring and manipulating networks. In E. Adar, M. Hurst, T. Finin, N. Glance, N. Nicolov, & B. Tseng (Eds.), Proceedings of the 3rd international AAAI conference on weblogs and social media (pp. 361–362). San Jose: The AAAI Press.
- Bernardini, S., Ferraresi, A., & Miličević, M. (2016). From EPIC to EPTIC – exploring simplification in interpreting and translation from an intermodal perspective. Target, 28(1), 61–86. doi: 10.1075/target.28.1.03ber
- Cartoni, B., & Meyer, T. (2012). Extracting directional and comparable corpora from a multilingual corpus for translation studies. In N. Calzolari, K. Choukri, T. Declerck, M. Uğur Doğan, B. Maegaard, J. Mariani, … S. Piperidis (Eds.), Proceedings of the 8th international conference on language resources and evaluation (pp. 2132–2137). Istanbul: European Languages Resources Association.
- De Sutter, G., Lefer, M.-A., & Delaere, I. (Eds.). (2017). Empirical translation studies. New methodological and theoretical traditions. Berlin: de Gruyter.
- Fantinuoli, C., & Zanettin, F. (2015). Creating and using multilingual corpora in translation studies. In C. Fantinuoli, & F. Zanettin (Eds.), New directions in corpus-based translation studies (pp. 1–11). Berlin: Language Science Press.
- Franco Aixelá, J. (2001-2017). BITRA (Bibliography of interpreting and translation) [Open-access database]. Retrieved from dti.ua.es/en/bitra/introduction.html, Doi:10.14198/bitra
- Gale, W. A., & Church, K. W. (1993). A program for aligning sentences in bilingual corpora. Computational Linguistics, 19(1), 75–102.
- Gambier, Y., & van Doorslaer, L. (Eds.). (n.d). Translation studies bibliography [Online database]. Retrieved from benjamins.com/online/tsb/
- Graën, J., Batinic, D., & Volk, M. (2014). Cleaning the Europarl corpus for linguistic applications. In J. Ruppenhofer, & G. Faaß (Eds.), Proceedings of the 12th edition of the KONVENS conference, Hildesheim, Germany, October 8–10, 2014 (pp. 222–227). Hildesheim: Universitätsverlag Hildesheim.
- Grbić, N. (2013). Bibliometrics. In Y. Gambier, & L. van Doorslaer (Eds.), Handbook of translation studies (Vol. 4, pp. 20–24). Amsterdam: John Benjamins.
- Harzing, A.-W. (2011). The publish or perish book. Your guide to effective and responsible citation analysis. Melbourne: Tarma Software Research.
- Harzing, A.-W. (2016). Publish or Perish (Version 6.20.6110) [Computer software]. Retrieved from www.harzing.com/pop.htm
- Harzing, A.-W., & Alakangas, S. (2017). Microsoft academic is one year old: The phoenix is ready to leave the nest. Scientometrics, 112, 1887–1894. doi: 10.1007/s11192-017-2454-3
- Hu, K. (2016). Introducing corpus-based translation studies. Berlin: Springer.
- Islam, Z., & Mehler, A. (2012). Customization of the Europarl corpus for translation studies. In N. Calzolari, K. Choukri, T. Declerck, M. Uğur Doğan, B. Maegaard, J. Mariani, … S. Piperidis (Eds.), Proceedings of the 8th international conference on language resources and evaluation (pp. 2505–2510). Istanbul: European Languages Resources Association.
- Ji, M., Oakes, M., Defeng, L., & Hareide, L. (Eds.). (2017). Corpus methodologies explained. An empirical approach to translation studies. London: Routledge.
- Kenny, D. (2009). Corpora. In G. Saldanha, & M. Baker (Eds.), Routledge encyclopedia of translation studies (2nd ed., pp. 59–62). London: Routledge.
- Kilgarriff, A., Baisa, V., Bušta, J., Jakubíček, M., Kovvář, V., Michelfeit, J., … Suchomel, V. (2014). The sketch engine: Ten years on. Lexicography, 1(1), 7–36. doi: 10.1007/s40607-014-0009-9
- Koehn, P. (2005). Europarl: A parallel corpus for statistical machine translation. In Proceedings of the 10th machine translation summit (pp. 79–86). Phuket, Thailand: Asia-Pacific Association for Machine Translation.
- Kurokawa, D., Goutte, C., & Isabelle, P. (2009). Automatic detection of translated text and its impact on machine translation. In Proceedings of the 12th machine translation summit (pp. 81–88). Ottawa: International Association for Machine Translation.
- Li, D. (2017). Translator style. A corpus-assisted approach, In M. Ji, M. Oakes, L. Defeng, & L. Hareide (Eds.), Corpus methodologies explained. An empirical approach to translation studies (pp. 103–136). London: Routledge.
- Malamatidou, S. (2018). Corpus triangulation. Combining data and methods in corpus-based translation studies. London: Routledge.
- Mellinger, C. D., & Hanson, T. A. (2017). Quantitative research methods in translation and interpreting studies. London: Routledge.
- Mikhailov, M., & Cooper, R. (2016). Corpus linguistics for translation and contrastive studies. A guide for research. London: Routledge.
- Oakes, M. P., & Ji, M. (Eds.). (2012). Quantitative methods in corpus-based translation studies. Amsterdam: John Benjamins.
- Olohan, M. (2004). Introducing corpora in translation studies. London: Routledge.
- Pięta, H. (2017). Theoretical, methodological and terminological issues in researching indirect translation: A critical annotated bibliography. Translation Studies, 10(2), 198–216. doi: 10.1080/14781700.2017.1285248
- Tan, L., & Bond, F. (2014). NTU-MC toolkit: Annotating a linguistically diverse corpus. In L. Tounsi, & R. Rak (Eds.), Proceedings of COLING 2014, the 25th international conference on computational linguistics: System demonstrations (pp. 86–89). Dublin: Association for Computational Linguistics.
- Tiedemann, J. (2012). Parallel data, tools and interfaces in OPUS. In N. Calzolari, K. Choukri, T. Declerck, M. Uğur Doğan, B. Maegaard, J. Mariani, … S. Piperidis (Eds.), Proceedings of the 8th international conference on language resources and evaluation (pp. 2214–2218). Istanbul: European Languages Resources Association.
- Vandepitte, S., & De Sutter, G. (2013). Contrastive linguistics and translation studies. In Y. Gambier, & L. van Doorslaer (Eds.), Handbook of translation studies (Vol. 4, pp. 36–41). Amsterdam: John Benjamins.
- Zanettin, F. (2012). Translation-driven corpora. Manchester: St. Jerome.
- Zanettin, F. (2013). Corpus methods for descriptive translation studies. Procedia - Social and Behavioral Sciences, 95, 20–32. doi: 10.1016/j.sbspro.2013.10.618
- Zanettin, F., Saldanha, G., & Harding, S.-A. (2015). Sketching landscapes in translation studies: A bibliographic study. Perspectives, 23(2), 161–182. doi: 10.1080/0907676X.2015.1010551