ABSTRACT
Studies of Alzheimer’s disease are based on model mice that have been altered by transgenesis and other techniques to elicit pathogenesis. However, changes in the gut microbiota were recently suggested to diminish cognitive function in patients, as well as in model mice. Accordingly, we have created model mice of the human gut microbiota by transplanting germ-free C57BL/6N mice with fecal samples from a healthy volunteer and from an affected patient. These humanized mice were stably colonized and reproduced the bacterial diversity in donors. Remarkably, performance on Object Location Test and Object Recognition Test was significantly reduced in the latter than in the former at 55 weeks of age, suggesting that gut microbiota transplanted from an affected patient affects mouse behavior. In addition, metabolites related to the nervous system, including γ-aminobutyrate, taurine, and valine, were significantly less abundant in the feces of mice transplanted with microbiota from the affected patient.
Graphical Abstract
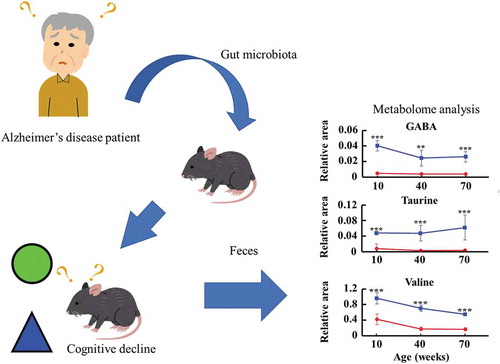
Transplantation of fecal microbiota from patients with Alzheimer’s disease alters fecal metabolome and behavior in recipient mice.
The microbiota in the human gut provides healthful benefits and plays a critical role in maintaining health. Conversely, changes in the microbiota, i.e., dysbiosis, were recently linked to various diseases such as type 2 diabetes [Citation1], inflammatory bowel disease [Citation2], obesity [Citation3,Citation4], allergy [Citation5], and neurologic disorders [Citation6]. Accordingly, germ-free animals were developed to directly examine not only the germ-free state but also gnotobiotic states [Citation7]. Importantly, germ-free mice can be “humanized” by transplanting human fecal microbiota, enabling direct observation of changes in microbiota composition and metabolism, as well as interactions between host and microbiota [Citation8]. Indeed, genetically modified and germ-free model mice enable experiments that cannot be performed in humans, and thus are considered indispensable tools in biology and medicine [Citation9–Citation11]. Nevertheless, species differences between mice and humans should be considered in interpreting results. For example, 85% of microbial genera found in mice are not observed in humans, although the same bacterial phyla are present [Citation12]. On the other hand, comparison of humanized gnotobiotic mice with various human microbiota datasets indicated similarities in major metabolic features, implying that individual specific features are reconstituted in model mice [Citation13].
The diversity of gut microbiota is now well-known to change with age and to correlate with host health. Indeed, high microbial diversity is traditionally associated with better health, although a growing body of research does not seem to support this relationship. For example, newer studies show that major active depressive disorder and autism are more strongly related to microbiota composition than to its diversity [Citation14,Citation15].
Recently, the microbiota was linked to Alzheimer’s disease [Citation16], a progressive degenerative disorder of the central nervous system that impairs cognition, presumably via a microbiota-gut-brain axis, which appears to be essential to health. For example, probiotic supplementation improves cognitive behavior in model mice of the disease [Citation17,Citation18]. Moreover, functional studies on the effects of bacteria in patients with Alzheimer’s disease are beginning [Citation19,Citation20]. Changes in intestinal microorganisms were also reported to affect the behavior of the host and progeny [Citation21,Citation22]. In this light, humanized gnotobiotic mice were prepared in this study using fecal samples from a patient with Alzheimer’s disease and were compared, in terms of microbiota content and behavior, to mice transplanted with microbiota from a healthy volunteer.
Materials and methods
Collection of fecal samples
The study was reviewed by the Ethics Committee at Okayama University Graduate School of Medicine, Dentistry, and Pharmaceutical Sciences and at Okayama University Hospital (approval number: KEN1612-031). Those patients who were judged by a doctor as having severe Alzheimer’s disease were included in the study. Fecal samples were collected from patients with Alzheimer’s disease, as well as from age-matched healthy volunteers, stored at 4°C under anaerobic conditions using AnaeroPack (Mitsubishi Gas Chemical Company, Japan), and transported to the laboratory within two days. In the laboratory, samples were suspended 2:1 in phosphate-buffered saline (Life Technologies, Japan) containing 40% glycerol (Nacalai Tesque, Japan), immediately frozen in liquid nitrogen, and stored at – 80°C until analysis. Fecal samples were also collected from gnotobiotic mice every five weeks, and transported to the laboratory as soon as possible and under anaerobic conditions using Anaeropack. In the laboratory, samples were suspended in 200 µL of 40% glycerol, immediately frozen in liquid nitrogen, and stored at – 80°C until use. Fecal samples for metabolite analysis were also collected from gnotobiotic mice every five weeks, immediately frozen in liquid nitrogen, and stored at – 80°C until use.
16S rrna sequencing and analysis
Bacterial DNA was isolated and purified from human and mouse feces by enzymatic lysis, as previously described, with minor modifications [Citation23,Citation24]. Briefly, bacterial pellets were incubated for 1 h at 37°C with 15 mg/mL lysozyme (Sigma-Aldrich) in 10 mM Tris-HCl and 1 mM EDTA, and then for 30 min at 37°C with 2,000 units/mL achromopeptidase (Wako Pure Chemical Industries). Samples were then treated for 1 h at 55°C with 1% (w/v) sodium dodecyl sulfate and 1 mg/mL proteinase K (Merck, Japan), and extracted with phenol/chloroform/isoamyl alcohol (Life Technologies Japan). DNA was precipitated with isopropanol, pelleted for 15 min at 4°C and 3,300 × g, rinsed with 75% ethanol, dried, and dissolved in 10 mM Tris-HCl and 1 mM EDTA. Subsequently, DNA samples were digested for 30 min at 37°C with 1 mg/mL RNase A (Wako Pure Chemical Industries) and precipitated by adding equal volumes of 26% polyethylene glycol 6,000 and 1.6 M NaCl. DNA was pelleted at 15,000 × g and 4°C, washed with 7% ethanol, and dissolved in 10 mM Tris-HCl.
Microbial 16S rDNA profiles were collected by MiSeq sequencing (Illumina) of V3–V4 fragments, strictly following the Illumina 16S Metagenomic Sequencing Library Preparation Guide. Library preparation and sequencing were performed at Okayama University Hospital Biobank (Okadai Biobank) and at Fasmac (Atsugi City, Kanagawa, Japan). Datasets were analyzed in Quantitative Insights Into Microbial Ecology (QIIME) 1.9.1 using the GreenGenes 13.08 database.
Animal experiments
Animal protocols were approved by the Committee for Animal Experiments at Okayama University Advanced Science Research Center (approval number: OKU-2017 289). Briefly, germ-free male C57BL/6N mice (n = 7 per group) were purchased at 3 weeks of age from CLEA Japan (Shizuoka, Japan), and housed in sterilized isolators at 22 ± 2°C and 55 ± 15% humidity, and with a 12 h light/12 h dark cycle. Radiation-sterilized standard laboratory rodent chow (CL-2, CLEA Japan) and autoclave-sterilized water were provided ad libitum.
Generation of humanized gnotobiotic mice
Frozen human feces were thawed by diluting in an equal volume of degassed phosphate buffer under anaerobic conditions. Fibers and human-derived cells were removed by filtration on a FALCON Cell Strainer (REF352360), and 0.15 mL of non-settling material was administered into germ-free recipient mice (4 weeks of age).
Behavior test
Behavior was assessed by Object Location Test (OLT) and Novel Object Recognition Test (ORT), as adapted from Benice et al. [Citation25], Goodman et al. [Citation26], and Kikusui et al. [Citation27]”. Novel Object Recognition Test was originally designed by Ennanceur and Delacour in 1988 [Citation28], and is widely used in rodents that respond to novelty. This test included an exploration phase, during which a mouse was placed for 5 min in an open field with two identical objects. The total time spent exploring the two objects was measured. The mouse was then returned to its home cage for 15 min. In the subsequent test phase, the mouse was placed in the same open field but after one of the two objects was moved to a new location. The time spent exploring the two objects was measured over 5 min as well. After a second 15-min interval in the home cage, the mouse was placed again in the same open field after one of the two objects was replaced with a different object but kept in the same location. The mouse was allowed to explore for another 5 min, during which the time spent exploring each object was measured. Tests were recorded on a video camera for subsequent analysis.
Metabolite extraction
Metabolites were extracted at Human Metabolome Technologies (Tsuruoka, Yamagata, Japan). To extract ionic metabolites, approximately 25 mg of fecal sample was dissolved 1:9 (w/v) in sterile pure water (Nacalai Tesque, Japan), homogenized using a vortex mixer, and centrifuged at 2,300 × g and 4°C for 5 min. After centrifugation, 80 µL of the supernatant was mixed with 20 µL of internal standard (H3304-1002, Human Metabolome Technologies), depleted of macromolecules by filtration for 120 min at 9,100 × g and 4°C through a Millipore 5,000-Da centrifugal filter (UltrafreeMC-PLHCC, Human Metabolome Technologies), and analyzed by capillary electrophoresis and time-of-flight mass spectrometry. To extract non-ionic metabolites, approximately 25 mg of fecal sample was dissolved 1:20 w/v in methanol containing internal standard (H3304-1002, Human Metabolome Technologies), homogenized, and centrifuged as described. After centrifugation, 200 µL of the supernatant was transferred to a glass vial, evaporated under nitrogen gas, reconstituted with 200 µL of 50% isopropanol (v/v), and analyzed by liquid chromatography and time-of-flight mass spectrometry.
Metabolite analysis
Ionic and non-ionic metabolites were analyzed in Dual Scan (Human Metabolome Technologies), as described previously [Citation29,Citation30]. Briefly, ionic metabolites were separated on an Agilent CE capillary electrophoresis system equipped with an Agilent 6210 time-of-flight mass spectrometer, an Agilent 1100 isocratic HPLC pump, an Agilent G1603A CE-MS adapter kit, and an Agilent G1607A CE-ESI-MS sprayer kit (Agilent Technologies, Waldbronn, Germany). Instruments were controlled via Agilent G2201AA ChemStation version B.03.01 for CE (Agilent Technologies), and samples were eluted through a fused silica capillary (50 μm internal diameter × 80 cm total length). Commercial electrophoresis buffers (H3301-1001 and H3302-1021, Human Metabolome Technologies) were used as electrolytes for cation and anion analyses, respectively. Spectra were collected over m/z 50–1,000 [Citation29]. On the other hand, non-ionic metabolites were analyzed on an Agilent 1200 series RRLC system SL equipped with an Agilent 6230 time-of-flight mass spectrometer (Agilent Technologies) and fitted with an ODS column (2 × 50 mm, 2 μm) [Citation30]. Instruments were controlled via Agilent G2201AA ChemStation version B.03.01 for CE (Agilent Technologies).
Peaks were extracted by automatic integration in MasterHands (Keio University, Tsuruoka, Yamagata, Japan) to obtain m/z, peak area, and migration time for capillary electrophoresis and retention time for liquid chromatography [Citation31]. Peaks corresponding to isotopomers, adduct ions, and other product ions of known metabolites were excluded, and the remaining peaks were annotated against the Human Metabolome Technologies database based on m/z value, migration time, and retention time. Annotated peaks were then normalized to internal standards and sample amounts to obtain relative levels of each metabolite. Hierarchical clustering and principal components analysis were performed in PeakStat and Sample Stat (Human Metabolome Technologies), respectively. Detected metabolites were mapped to metabolic pathways using VANTED [Citation32].
Statistical analysis
Data were compared by Student’s t-test and Mann-Whitney U-test, and outliers were identified by Smirnov-Grubbs test. For deterioration of cognitive function over time, one-way analysis of variance (ANOVA test) followed by the Dunnett test was used.Relative peak areas of individual metabolites were compared by Welch’s t-test. p < 0.05 was considered significant in all cases. Statistical tests were performed in EZR version 1.37 (Saitama Medical Center, Jichi Medical University) [Citation33], a graphical user interface for R version 2.13.0 (The R Foundation for Statistical Computing).
Results
Selection of fecal samples for constructing a model mouse of alzheimer’s disease
The fecal microbiota of 14 healthy volunteers (female 71%, mean age: 81.6 ± 2.7 years) and 13 affected patients (female 69%, mean age: 84.8 ± 5.4 years) were compared to identify two samples, the microbiota of which were clearly far from each other by PCoA and representative of healthy or affected volunteers (). The samples selected in this manner were obtained from a 76-year-old healthy female donor and from an 82-year-old male donor with Alzheimer’s disease. Both samples were diluted in phosphate-buffered saline, filtered, and orally inoculated into germ-free mice. Colonization was investigated by analysis of fecal bacteria two weeks after inoculation. The data show that recipient mice were stably colonized, and reproduced much of the bacterial diversity in the donor microbiota. In particular, 97.5% and 88.5% of genera detected in healthy and affected donors were also detected in recipient mice, as assessed by sequencing of V3-V4 fragments in 16S rRNA. This result indicates that mice were successfully humanized, with engraftment rate higher than that previously achieved [Citation12,Citation34]. We note that humanized model mice have been used in many studies [Citation35], and that germ-free mice transplanted with human microbiota are deemed important research resources in immunology, bacteriology, and medicine. The 16S rRNA profiles of mice transplanted with microbiotas from healthy and affected donors were deposited in DDBJ/EBI/NCBI under accession numbers SAMD00154374 and SAMD00154375, respectively.
Figure 1. Comparison of microbiota in healthy volunteers (HC) and patients with Alzheimer’s disease (AD).
(a) Principal coordinate analysis of microbiotas from 14 healthy volunteers (blue) and 13 patients (red). Fecal samples AD35 and HC49 were selected for transplantation. (b) Principal coordinate analysis clearly separates healthy and affected volunteers based on UniFrac distance. Data are mean ± SEM. ***, p < 0.001 by Mann-Whitney U test.
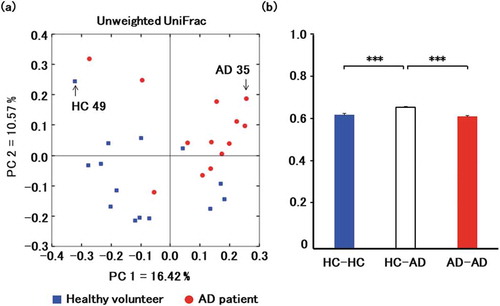
Time-dependent changes in transplanted microbiota
16S rRNA was extracted from fecal samples at age 6, 40, 55, and 70 weeks to investigate long-term microbial colonization. Sequencing generated approximately 4.9 million bases, and reads were clustered into OTUs at 97% similarity. Firmicutes (57.1 ± 5.8% and 58.6 ± 2.6% in mice transplanted with microbiota from healthy and affected donors) and Bacteroidetes (31.6 ± 3.1% and 28.9 ± 1.8%) were the dominant phyla in all mice. In contrast, Verrucomicrobia (9.3 ± 2.0%) was less dense in mice transplanted with microbiota from the healthy donor, as were Verrucomicrobia (10.0 ± 1.4%) and Proteobacteria (1.3 ± 0.4%) in mice transplanted with microbiota from the affected donor (). Principal coordinate analysis of weighted UniFrac scores clustered mice based on only the donor microbiota, confirming that the compositions of transplanted microbial communities are stable over time ()). Based on the Shannon Diversity Index, microbial diversity was lower in the former than in the latter, but stable over time ()).
Figure 2. Changes in the gut microbiota of a model mouse of Alzheimer’s disease. Time-dependent changes in bacterial phyla of Firmicutes (a), Bacteroidetes (b), Verrucomicrobia (c), and Proteobacteria (d) in mice transplanted with microbiota from a healthy volunteer (blue) and from a patient with Alzheimer’s disease (red).
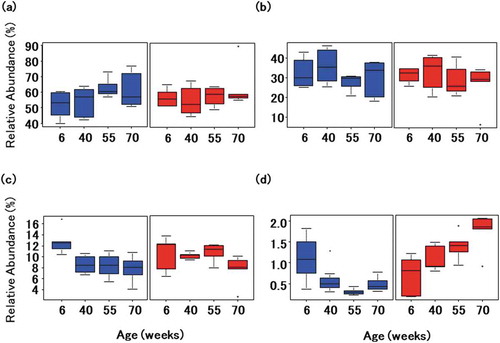
Figure 3. Gut microbial composition over time in mice transplanted with microbiotas from healthy and affected donors.
(a) Principal coordinate analysis of weighted UniFrac distances. Data points are individual mice colored by donor microbiota and age in weeks. (b) Shannon index by age and donor microbiota.
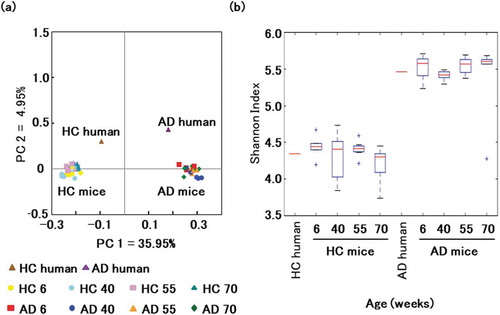
Behavioral characteristics
Behavior was assessed by Object Location Test and Novel Object Recognition Test once every five weeks. In brief, mice were placed for 5 min in an acrylic plastic cage with an open field (182 × 260 × 128 mm) and with two objects. The time spent exploring familiar or novel objects, as a percentage of the total exploration time allotted, was calculated. At a young age (10–25 weeks of age), there was no difference among all mice in the time spent exploring a novel object, implying that these mice distinguish between familiar and unfamiliar objects. However, time spent exploring the unfamiliar object in both object location and object recognition tests was lower at 55 weeks of age in mice transplanted with microbiota from affected patients, implying that these mice cannot distinguish between familiar and unfamiliar objects and have experienced loss of cognitive function (). This difference persisted to 70 weeks of age. On the contrary, significant deterioration of cognitive function was observed through both the Object Location Test (70 and 75 weeks of age vs. 10 weeks of age) and Novel Object Recognition Test (70 weeks of age vs. 10 weeks of age) in mice transplanted with microbiota from affected patients ().
Figure 4. Novel object recognition test.
(a) Design of object location test (OLT) and object recognition test (ORT). (b) Ratio of time spent exploring a familiar object in a new location to time spent exploring a familiar object in an old location. Blue, mice transplanted with microbiota from a healthy donor. * and ** indicate comparison between groups and significant differences were observed at 55 and 70 weeks of age; red, mice transplanted with microbiota from a patient with Alzheimer’s disease. Red bar, Mice transplanted with microbiota from a patient with Alzheimer’s disease had significantly altered cognitive function at 70 and 75 weeks of age compared with that at 10 weeks of age. (c) Ratio of time spent exploring a novel object to time spent exploring a familiar object. Blue, mice transplanted with microbiota from a healthy donor. * and ** indicate comparison between groups and significant differences were observed at 30, 55, 60, 65 and 70 weeks of age; red, mice transplanted with microbiota from a patient with Alzheimer’s disease. Red bar, Mice transplanted with microbiota from a patient with Alzheimer’s disease had significantly altered cognitive function at 70 weeks of age compared with that at 10 weeks of age. Data are mean ± SEM. *, p < 0.05; **, p < 0.01.
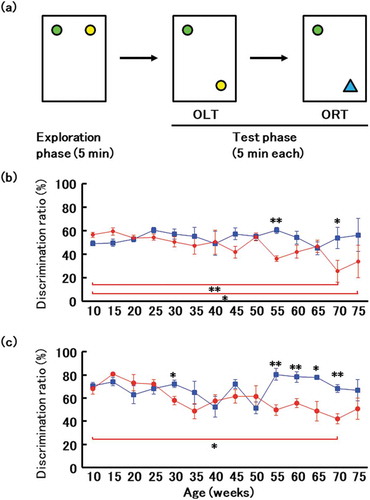
Analysis of fecal metabolomes
Metabolites were surveyed in feces collected at 10, 40, and 70 weeks of age. However, feces collected at 10 weeks from a mouse transplanted with microbiota from a healthy donor was identified by Smirnov-Grubbs test as an outlier, and was excluded from analysis. A total of 322 metabolites (218 cations and 104 anions) associated with the gut microbiota were identified by capillary electrophoresis and mass spectrometry from all mice. Another 195 metabolites (98 positives and 97 negatives) were identified by liquid chromatography and mass spectrometry. Principal component analysis, a typical tool to classify and visualize high dimensional data, indicated that the donor microbiota was a significant determinant of fecal metabolomes (). By comparing 30 metabolites that were significantly altered between mice transplanted with microbiotas from healthy and affected donors, we found that GABA, taurine, tryptophan, tyrosine, and valine were more abundant in the former. Conversely, propionic acid was less abundant ().
Figure 5. Comparison of metabolites in mouse feces.
Principal component analysis of microbial metabolites in mice transplanted with microbiotas from a healthy volunteer (blue) and from a patient with Alzheimer’s disease (red).
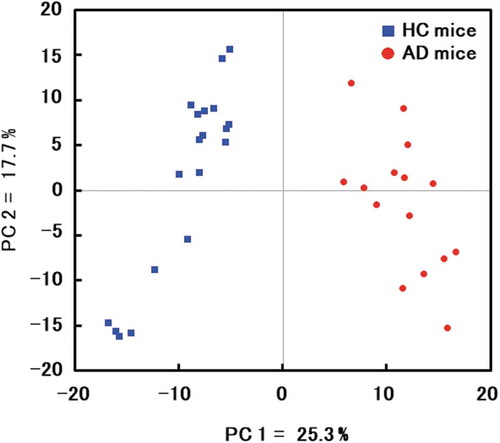
Figure 6. Changes in fecal metabolites of recipient mice.
Fecal metabolomes of GABA (a), taurine (b), valine (c), tryptophan (d), tyrosine (e), and propionic acid (f) were surveyed at age 10, 40, and 70 weeks in mice transplanted with microbiotas from a healthy volunteer (blue) and a patient with Alzheimer’s disease (red). Data are mean ± SD. *, p < 0.05; **, p < 0.01; ***, p < 0.001 by t test.
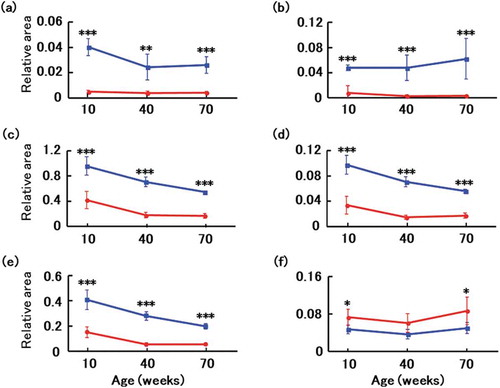
Discussion
Alzheimer’s disease is a neurodegenerative disorder characterized by progressive cognitive decline, for which therapies have not been established. Recently, various model mice were constructed to investigate potential therapeutic methods [Citation36]. However, most such models are established by gene modification, and some validation is necessary to demonstrate onset of disease. On the other hand, Alzheimer’s disease has been linked to the gut microbiota [Citation37], although the underlying mechanisms are not fully understood. Hence, we constructed humanized gnotobiotic mice with gut microbiota from an affected patient, with a view to investigate pathogenesis and possible treatments. These mice reproduced the characteristics of the donor microbiota, generating gut microbial communities that are stable over time. Strikingly, microbial diversity was higher in mice transplanted with microbiota from an affected donor than in mice transplanted with microbiota from a healthy donor. In contrast, the gut microbiota of affected patients was previously reported to have low diversity, although results have been inconsistent among studies [Citation37]. On the other hand, the APP/PSS1 model mouse of Alzheimer’s disease is similar to those we have established in terms of microbial diversity [Citation38]. In this study, the selected samples were from healthy female and male donors with Alzheimer’s disease but the diversity of the microbiota does not vary by gender [Citation39,Citation40]. In addition, Bacteroides have been reported to be associated with Japanese dementia [Citation41]; a similar trend was observed in this study (data not shown).
Although generally associated with aging [Citation42], Proteobacteria diminished in mice transplanted with microbiota from the healthy donor, but accumulated with age in mice transplanted with feces from the patient with Alzheimer’s disease. This phenomenon was previously reported in other model mice of the disease [Citation38]. We hypothesize that microbial composition affects the metabolic activity of the gut microbiota and the host, and thereby affects the metabolites produced, including bile acids, amino acids, and short-chain fatty acids. Ultimately, these metabolites may modulate host behavior over time, as observed in mice [Citation43]. Metabolites altered in mice transplanted with microbiota from an affected donor include GABA, taurine, and valine, which are involved in central nervous system function. In addition, differences in the concentrations of other amino acids and short-chain fatty acids, such as tryptophan, tyrosine, and propionic acid, respectively, were observed. GABA is known to be produced by Lactobacillus, Bifidobacterium, and Bacteroides [Citation44,Citation45]. It has been suggested that these bacteria cause GABA accumulation in feces and may influence mood and behavior [Citation46]. While taurine is also produced by gut microbiota [Citation47], it is thought that its absorption into the body may have also been increased upon transplantation with microbiota from an affected donor, as it was accumulated in the feces of mice transplanted with microbiota from a healthy volunteer. Thus, taurine is a potential oral therapy for cognitive impairment that may limit damage from Aβ plaques [Citation22,Citation48]. In addition, as valine is known to be produced by Lactobacillus and Corynebacterium [Citation49,Citation50], it has been suggested that accumulation of valine in feces by these bacteria is associated with cognitive decline [Citation51]. On the contrary, tryptophan which has been reported to be increased by Bifidobacterium [Citation52], is a precursor of serotonin, a neurotransmitter. Accordingly, loss of tryptophan, mostly due to direct metabolism of dietary tryptophan by gut microorganisms [Citation53,Citation54], was reported to diminish serotonin and elicit cognitive decline. Similarly, dietary tyrosine has been reported to be affected by enteric bacteria [Citation55], and it has been suggested that these bacteria affect tyrosine accumulation in feces. Oral administration of tyrosine was reported to improve memory and cognitive function [Citation56]. Finally, propionic acid is known to be produced by Lactobacillus and Propionibacterium [Citation46,Citation57], and it has been suggested that these bacteria affect propionic acid accumulation in feces. The propionic acid was found to be significantly more abundant in mice transplanted with microbiota from the affected patient, in line with previous reports linking propionic acid to cognitive deficits [Citation58].
Although the difference between these metabolites has been observed from 10 weeks of age, we thought that it will take a long time to affect the behavior after the metabolites produced by the gut microbes are absorbed.
In this study, no significant deterioration of cognitive function was observed until 75 weeks of age in mice transplanted with microbiota from healthy volunteer, as shown in ,c), whereas significant deterioration of cognitive function was observed through both the Object Location Test (70 and 75 weeks of age vs. 10 weeks of age) and Novel Object Recognition Test (70 weeks of age vs. 10 weeks of age) in mice transplanted with microbiota from affected patients. It was suggested that gut microbiota transplanted from an affected patient affects mouse behavior. Therefore, the mice transplanted with microbiota from affected patients compared to the mice transplanted with microbiota from healthy volunteer observed significant cognitive decline in the Object Location Test (55 and 75 weeks of age) and Novel Object Recognition Test (30, 55, 60, 65 and 70 weeks of age).
In summary, we found that oral administration of microbiota from a patient with Alzheimer’s disease triggers cognitive decline in recipient mice. The data also show that differences in gut metabolites as a result of differences in the composition of intestinal microorganisms elicit assorted behaviors, reinforcing the idea of a gut-brain axis. Therefore, this model mouse may prove useful in investigating the causes of Alzheimer’s disease and in developing novel therapeutic methods.
Acknowledgments
We thank Okayama University Hospital Biobank (Okadai Biobank) for Illumina sequencing.
Disclosure statement
No potential conflict of interest was reported by the authors.
Additional information
Funding
References
- Qin J, Li Y, Cai Z, et al. A metagenome-wide association study of gut microbiota in type 2 diabetes. Nature. 2012;490:55–60.
- Manichanh C, Borruel N, Casellas F, et al. The gut microbiota in IBD. Nat Rev Gastroenterol Hepatol. 2012;9:599–608.
- Ley RE, Turnbaugh PJ, Klein S, et al. Microbial ecology: human gut microbes associated with obesity. Nature. 2006;444:1022–1023.
- Le Chatelier E, Nielsen T, Qin J, et al. Richness of human gut microbiome correlates with metabolic markers. Nature. 2013;500:541–546.
- Pascal M, Perez-Gordo M, Caballero T, et al. microbiome and allergic diseases. Front Immunol. 2018;9:1584.
- Umbrello G, Esposito S. Microbiota and neurologic diseases: potential effects of probiotics. J Transl Med. 2016;14:298.
- Reyniers JA, Trexler PC, Ervin RF. Rearing germ-free albino rats. Lobund Reports. 1946;1:1–84.
- Bowey E, Adlercreutz H, Rowland I. Metabolism of isoflavones and lignans by the gut microflora: a study in germ-free and human flora associated rats. Food Chem Toxicol. 2003;41:631–636.
- Bäckhed F, Manchester JK, Semenkovich CF, et al. Mechanisms underlying the resistance to diet-induced obesity in germ-free mice. Proc Natl Acad Sci U S A. 2007;104:979–984.
- Matthews RA. Medical progress depends on animal models - doesn’t it? J R Soc Med. 2008;101:95–98.
- Bracken MB. Why animal studies are often poor predictors of human reactions to exposure. J R Soc Med. 2009;102:120–122.
- Ley RE, Bäckhed F, Turnbaugh P, et al. Obesity alters gut microbial ecology. Proc Natl Acad Sci U S A. 2005;102:11070–11075.
- Marcobal A, Kashyap PC, Nelson TA, et al. A metabolomic view of how the human gut microbiota impacts the host metabolome using humanized and gnotobiotic mice. Isme J. 2013;7:1933–1943.
- Finegold SM, Dowd SE, Gontcharova V, et al. Pyrosequencing study of fecal microflora of autistic and control children. Anaerobe. 2010;16:444–453.
- Jiang H, Ling Z, Zhang Y, et al. Altered fecal microbiota composition in patients with major depressive disorder. Brain Behav Immun. 2015;48:186–194.
- Hu X, Wang T, Jin F. Alzheimer’s disease and gut microbiota. Sci China Life Sci. 2016;59:1006–1023.
- Kobayashi Y, Sugahara H, Shimada K, et al. Therapeutic potential of Bifidobacterium breve strain A1 for preventing cognitive impairment in Alzheimer’s disease. Sci Rep. 2017;7:13510.
- Bonfili L, Cecarini V, Berardi S, et al. Microbiota modulation counteracts Alzheimer’s disease progression influencing neuronal proteolysis and gut hormones plasma levels. Sci Rep. 2017;7:2426.
- Zhan X, Stamova B, Jin L-W, et al. Gram-negative bacterial molecules associate with Alzheimer disease pathology. Neurology. 2016;87:2324–2332.
- Nguyen TTT, Fujimura Y, Mimura I, et al. Cultivable butyrate-producing bacteria of elderly Japanese diagnosed with Alzheimer’s disease. J Microbiol. 2018;56:760–771.
- Goeden N, Velasquez J, Arnold KA, et al. Maternal inflammation disrupts fetal neurodevelopment via increased placental output of serotonin to the fetal brain. J Neurosci. 2016;36:6041–6049.
- Kim S, Kim H, Yim YS, et al. Maternal gut bacteria promote neurodevelopmental abnormalities in mouse offspring. Nature. 2017;549:528–532.
- Kim S-W, Suda W, Kim S, et al. Robustness of gut microbiota of healthy adults in response to probiotic intervention revealed by high-throughput pyrosequencing. DNA Res. 2013;20:241–253.
- Morita H, Kuwahara T, Ohshima K, et al. An improved DNA isolation method for metagenomic analysis of the microbial flora of the human intestine. Microbes and Environ. 2007;22:214–222.
- Benice TS, Rizk A, Kohama S, et al. Sex-differences in age-related cognitive decline in C57BL/6J mice associated with increased brain microtubule-associated protein 2 and synaptophysin immunoreactivity. Neuroscience. 2006;137:413–423.
- Goodman T, Trouche S, Massou I, et al. Young hippocampal neurons are critical for recent and remote spatial memory in adult mice. Neuroscience. 2010;171:769–778.
- Kikusui T, Shimozawa A, Kitagawa A, et al. N-Acetylmannosamine improves object recognition and hippocampal cell proliferation in middle-aged mice. Biosci Biotechnol Biochem. 2012;76:2249–2254.
- Ennaceur A, Delacour J. A new one-trial test for neurobiological studies of memory in rats. 1: behavioral data. Behav Brain Res. 1988;31:47–59.
- Ohashi Y, Hirayama A, Ishikawa T, et al. Depiction of metabolome changes in histidine-starved Escherichia coli by CE-TOFMS. Mol BioSyst. 2008;4:135–147.
- Ooga T, Sato H, Nagashima A, et al. Metabolomic anatomy of an animal model revealing homeostatic imbalances in dyslipidaemia. Mol BioSyst. 2011;7:1217–1223.
- Sugimoto M, Wong DT, Hirayama A, et al. Capillary electrophoresis mass spectrometry-based saliva metabolomics identified oral, breast and pancreatic cancer-specific profiles. Metabolomics. 2010;6:78–95.
- Junker BH, Klukas C, Schreiber F. VANTED: A system for advanced data analysis and visualization in the context of biological networks. BMC Bioinformatics. 2006;7:109.
- Kanda Y. Investigation of the freely available easy-to-use software ‘EZR” for medical statistics.’. Bone Marrow Transplant. 2013;48:452–458.
- Turnbaugh PJ, Ridaura VK, Faith JJ, et al. The effect of diet on the human gut microbiome: A metagenomic analysis in humanized gnotobiotic mice. Sci Transl Med. 2009;1:6ra14.
- Atarashi K, Tanoue T, Ando M, et al. Th17 cell induction by adhesion of microbes to intestinal epithelial cells. Cell. 2015;163:367–380.
- Saito T, Matsuba Y, Mihira N, et al. Single app knock-in mouse models of Alzheimer’s disease. Nat Neurosci. 2014;17:661–663.
- Vogt NM, Kerby RL, Dill-McFarland KA, et al. Gut microbiome alterations in Alzheimer’s disease. Sci Rep. 2017;7:13537.
- Bäuerl C, Collado MC, Diaz Cuevas A, et al. Shifts in gut microbiota composition in an APP/PSS1 transgenic mouse model of Alzheimer’s disease during lifespan. Lett Appl Microbiol. 2018;66:464–471.
- Davenport ER, Mizrahi-Man O, Michelini K, et al. Seasonal variation in human gut microbiome composition. PLoS One. 2014;9:e90731.
- Odamaki T, Kato K, Sugahara H, et al. Age-related changes in gut microbiota composition from newborn to centenarian: a cross-sectional study. BMC Microbiol. 2016;16:90.
- Saji N, Niida S, Murotani K, et al. Analysis of the relationship between the gut microbiome and dementia: a cross-sectional study conducted in Japan. Sci Rep. 2019;9:1008.
- Biagi E, Nylund L, Candela M, et al. Through ageing, and beyond: gut microbiota and inflammatory status in seniors and centenarians. PLoS One. 2010;5:e10667.
- Liu W-H, Chuang H-L, Huang Y-T, et al. Alteration of behavior and monoamine levels attributable to Lactobacillus plantarum PS128 in germ-free mice. Behav Brain Res. 2016;298:202–209.
- Yunes RA, Poluektova EU, Dyachkova MS, et al. GABA production and structure of gadB/gadC genes in Lactobacillus and Bifidobacterium strains from human microbiota. Anaerobe. 2016;42:197–204.
- Strandwitz P, Kim KH, Terekhova D, et al. GABA-modulating bacteria of the human gut microbiota. Nat Microbiol. 2019;4:396–403.
- Louis P, Flint HJ. Formation of propionate and butyrate by the human colonic microbiota. Environ Microbiol. 2017;19:29–41.
- Mosier AC, Justice NB, Bowen BP, et al. Metabolites associated with adaptation of microorganisms to an acidophilic, metal-rich environment identified by stable-isotope-enabled metabolomics. MBio. 2013;4:e00484–12.
- Jang H, Lee S, Choi SL, et al. Taurine directly binds to oligomeric amyloid-β and recovers cognitive deficits in Alzheimer model mice. Adv Exp Med Biol. 2017;975(1):233–241.
- Mutaguchi Y, Kasuga K, Kojima I. Production of d-branched-Chain amino acids by lactic acid bacteria carrying homologs to isoleucine 2-epimerase of Lactobacillus buchneri. Front Microbiol. 2018;9:1540.
- Wang X, Zhang H, Quinn PJ. Production of L-valine from metabolically engineered corynebacterium glutamicum. Appl Microbiol Biotechnol. 2018;102:4319–4330.
- Toledo JB, Arnold M, Kastenmüuller G, et al. Metabolic network failures in Alzheimer’s disease: A biochemical road map. Alzheimers Dement. 2017;13:965–984.
- Desbonnet L, Garrett L, Clarke G, et al. The probiotic bifidobacteria infantis: an assessment of potential antidepressant properties in the rat. J Psychiatr Res. 2008;43:164–174.
- Wikoff WR, Anfora AT, Liu J, et al. Metabolomics analysis reveals large effects of gut microflora on mammalian blood metabolites. PNAS. 2009;106:3698–3703.
- Selkrig J, Wong P, Zhang X, et al. Metabolic tinkering by the gut microbiome: implications for brain development and function. Gut Microbes. 2014;5:369–380.
- Castillo-Rodriguez E, Fernandez-Prado R, Esteras R, et al. Impact of altered intestinal microbiota on chronic kidney disease progression. Toxins (Basel). 2018;10:7.
- Deijen JB, Wientjes CJ, Vullinghs HF, et al. Tyrosine improves cognitive performance and reduces blood pressure in cadets after one week of a combat training course. Brain Res Bull. 1999;48:203–209.
- Guan N, Du B, Li J, et al. Comparative genomics and transcriptomics analysis-guided metabolic engineering of Propionibacterium acidipropionici for improved propionic acid production. Biotechnol Bioeng. 2018;115:483–494.
- Macfabe DF. Short-chain fatty acid fermentation products of the gut microbiome: implications in autism spectrum disorders. Microb Ecol Health Dis. 2012;23:19260.