ABSTRACT
Previous studies demonstrate that morphosyntactic plural markers and the structure of numeral systems have individually strong predictive power with regard to the usage of sortal classifiers in languages. We use these two factors as explanatory variables to train the computational classifier of random forests and evaluate the accuracy of their predictive power when selecting the existence/absence of sortal classifiers as response variable. Our results show that these two factors result in an excellent discrimination performance of random forests, even when taking into account sortal classifiers as an areal feature. However, the correlation between morphosyntactic plural markers and multiplicative bases is weaker than the correlation between sortal classifiers and plural markers plus multiplicative bases. We are thus able to provide novel insights with regard to probabilistic universals on sortal classifiers, and suggest an innovative cross-disciplinary approach to test the effect of implicational universals with computational methods.
1. Introduction
How languages classify nouns of the lexicon is a subject relevant to various fields such as linguistics, psychology, neuroscience, sociology, among others (Aikhenvald, Citation2016; Grinevald, Citation2015; Kemmerer, Citation2017). While languages in Europe, Africa, and the Americas commonly categorize nouns according to grammatical gender (e.g. masculine/feminine in French), languages concentrated in Asia use a system of sortal classifiers based on shape and other inherent features of the referents (Corbett, Citation2013; Gil, Citation2013). A sortal classifier is defined as a word (or morpheme) that is required within the context of enumeration (Aikhenvald, Citation2000; Seifart, Citation2010). For instance, Mandarin Chinese is considered a typical sortal classifier language due to the fact that sortal classifiers are generally obligatory in enumeration context and that the language contains an inventory of more than 100 sortal classifiers (Her & Lai, Citation2012). As shown in (1), sortal classifiers are required in quantification and may highlight the long shape or the animacy of the referents (among other features).Footnote1
(1) Example of sortal classifiers in Mandarin Chinese (Sino-Tibetan)
a. zhuo shang you san tiao shengzi
table on have three clf-long rope
‘There are three ropes on the table.’
b. jia li you liang zhi gou
home inside have two clf-animal dog
‘There are two dogs in the house.’
Several theoretical approaches have been proposed to explain the distribution of sortal classifiers within languages of the world (Borer, Citation2005; Chierchia, Citation1998; Greenberg, Citation1990a). Two hypotheses are obtained from recent studies that approach sortal classifiers from a mathematical perspective (Her, Citation2017; Her & Lai, Citation2012; Tang, Citation2017). Under such a view, sortal classifiers are considered to form a multiplicative structure with the numerals and bear the exact mathematical value of one, along with the semantic feature used to highlight the following referent. For instance, the sortal classifier tiao (clf-long) in (1) functions as a multiplicand with the value of one and forms a multiplicative structure with the numeral three, c.f., san tiao shengzi (three clf-long rope) = three times one rope = three ropes. Following this mathematical approach, the first proposed hypothesis states that the existence of sortal classifiers necessarily implies that the language has a multiplicative numeral system (Her, Tang, & Li, Citationin press). In other words, sortal classifiers require the concept of multiplication to form a multiplicative structure; sortal classifiers can thus appear in a language only if multiplicative structure is already present. However, this relation is unidirectional, as the existence of multiplicative numerals does not automatically imply that a language has sortal classifiers. The second hypothesis suggests that morphosyntactic plural markers (e.g. – s in English) should be in complementary-like distribution with sortal classifiers since the two elements represent the same formal underlying category (Tang, Her, & Chen, Citationin press). This functional account unifies plural markers and sortal classifiers as multiplicand that bears the value of one and syntactically marks the countability of nouns. It is thus unlikely to have both plural markers and sortal classifiers in the same language, and if this does occur, the two grammatical elements are then expected to be in complementary distribution in the noun phrase.
Both hypotheses have been proposed as probabilistic universals and evaluated quantitatively (Her et al., Citation2018; Tang et al., Citation2018), but these two hypotheses have not been combined to assess their interaction. The aim of this paper is to investigate the correlation between the existence of multiplicative units in the numeral system of a language and its use of plural markers and/or sortal classifiers. Moreover, while previous studies only assess the interaction of variables via the method of simple conditional inference tree, we further develop the methodology and apply the computational classifier of random forests to evaluate the individual and interactive weight of plural markers and multiplicative numerals as explanatory variables. We aim to provide additional insight with regard to the theoretical account of sortal classifiers in languages of the world. We will demonstrate the use of computational methods within the field of language universals, as the use of the classifier of random forest may be extended to other types of probabilistic universals by not only assessing the interaction of the explanatory variables but also measuring their individual importance. In particular, we show that random forests are able to take into consideration areal and genealogical effects of language that are generally undermining conventional statistical analysis in the field of linguistics.
The structure of this paper is as follows: in Section 2, we highlight the main theoretical priors and definitions for the two probabilistic universals. In Section 3 we explain the content of the data and briefly introduce the concept of random forests. Section 4 displays the results of our analysis. Section 5 summarizes our findings whereas Section 6 concludes the paper.
2. Hypothesis: Classifiers as Multiplicands
The connection between sortal classifiers and multiplication originates from an observation on word order. In an enumerative construction composed of numeral, classifier, and noun; cross-linguistically the noun is never attested to intervene between the numeral and the classifier (Aikhenvald, Citation2000, p.104–105; Greenberg, Citation1990b, p.185; Peyraube, Citation1998; Wu, Feng, & Huang, Citation2006; Her, Citation2017). As an example, constructions such as [NUM CLF N] or [N NUM CLF] are commonly found in languages such as Mandarin Chinese and Thai but no languages show the [CLF N NUM] or [NUM N CLF] patterns. This distribution has been tentatively explained by considering the relation between numerals and sortal classifiers as a multiplicative structure that cannot be interrupted by the noun, i.e. [NUM CLF] = [NUM × 1]. Under such approach, sortal classifiers carry the necessarily fixed numerical value of 1, along with an inherent semantic feature of the referent (Au Yeung, Citation2005, Citation2007; Her, Citation2012a; Yi, Citation2009, Citation2011). For instance, in Mandarin Chinese, san zhi gou (three clf-animal dog) ‘three dogs’, the quantity of the referents is 3 × 1, and the sortal classifier zhi highlights the animacy of the referents.Footnote2
Probabilistic universals have been derived from this multiplicative approach. For instance, if a language has sortal classifiers, the same language is very likely to have multiplicative bases (Comrie, Citation2006, Citation2013) in its numeral system since both structures require the concept of multiplication (Greenberg, Citation1990a, p.292; Her, Citation2017, p.298).Footnote3 Moreover, the word order of sortal classifiers and multiplicative bases is very likely to be aligned (Her, Citation2017; Her et al., Citation2018). Taking the Chinese numeral system for example, wu-bai san-shi (five-hundred three-ten) ‘530ʹ has the internal relation of [(5x100)+(3x10)]. Numeral bases ‘100ʹ and ‘10ʹ function as multiplicands and follow the multiplier numbers ‘5ʹ and ‘3ʹ. Sortal classifiers likewise function as multiplicands and follow the numerals in Mandarin Chinese, e.g. san zhi gou (three clf-animal dog) ‘three dogs’. While the word order correlation is not directly relevant to the current study, the first part of the probabilistic universal which predicts that a language with sortal classifiers also has multiplicative bases in its numerals is further analyzed in the following section.
Studies on sortal classifiers also note the largely complementary distribution of sortal classifiers and plural markers, which is commonly referred to as the Greenberg-Sanches-Slobin generalization (Greenberg, Citation1990b; Sanches & Slobin, Citation1973). It initially states that if a language uses sortal classifiers in its basic structure of quantitative expressions, then the noun is normally not marked for number in the same structure (Greenberg, Citation1990b, p.177). For instance in (1), Mandarin Chinese uses sortal classifiers in quantitative expressions, the nouns following the numeral and the classifier are therefore generally not marked by plural, c.f., shengzi ‘rope’ and gou ‘dog’ (Some possible occurrences of plural markers in quantitative expressions in Mandarin Chinese are discussed in the following paragraph). This generalization involves complementary distribution but not collective exhaustivity (Fromkin, Rodman, & Hyams, Citation2011), i.e. sortal classifiers and plural markers tend not to co-occur, however, it does not imply that either one of the two is always found in languages of the world. By way of illustration, a classifier language commonly lacks plural marking, but languages without plural marking do not necessarily have classifiers (Doetjes, Citation2012, p.2566). Moreover, the generalization does not forbid the co-occurrence of sortal classifiers and plural markers in the same language; nevertheless, it does predict that if both the structures are allowed in the same language, they are not likely to co-occur in the same nominal phrase (T’sou, Citation1976, p.1216).
It has thus been claimed that classifiers and plural markers belong to the same syntactic category, and their complementary distribution have been investigated, qualitatively and quantitatively, in languages of the world (Borer, Citation2005; Her, Citation2012b; Jenks, Citation2017). Several languages (e.g. Hungarian, Mandarin Chinese, Persian, among others) are found attested with both sortal classifiers and plural markers, but they are generally considered as not real-exceptions due to the optional nature of sortal classifiers and/or plural markers in the targeted languages (Bisang, Citation2012; Csirmaz & Dekany, Citation2010; Doetjes, Citation2012; Gerner, Citation2006; Ghomeshi, Citation2003). Yet, the fact that the co-occurrence of sortal classifiers and plural markers can even be found in typical classifier languages such as Mandarin Chinese raises questions about the validity of the generalization (Kim & Melchin, Citation2018; Zhang, Citation2013). For instance in Chinese, the plural marker -men is occasionally found in quantitative expressions, c.f., san wei laoshi (three CLF-HUMAN teacher) and san wei laoshi men (three CLF-HUMAN teacher pl) ‘three teachers’.Footnote4
Recent studies suggest that the theoretical definition of sortal classifiers and plural markers is the main explanation to these apparent counter-examples (Tang et al., Citation2018). On the one hand, sortal classifiers should be differentiated from other types of classifiers such as noun classifiers and verbal classifiers (Aikhenvald, Citation2000; Dixon, Citation1986; Grinevald, Citation2015). On the other hand, only morphosyntactic plural markers (Kibort & Corbett, Citation2008) should be counted in the generalization, i.e. morphosemantic nominal plural markers such as collective or associative plurals (Rijkhoff, Citation2000; Vogel & Comrie, Citation2000) should be excluded. Morphosyntactic plural markers involve grammatical agreement with other elements of the clause while morphosemantic plural markers do not. For instance in French, plural is marked on nouns, articles, adjectives, and verbs, c.f., le bureau est petit (the.MASC.SG office be.MASC.SG small.MASC.SG) ‘the office is small’ and les bureaux sont petits (the.MASC.PL office.pl be.MASC.PL small.MASC.PL) ‘the offices are small’. As for Mandarin, the plural marker -men is in fact a collective marker that highlights the homogeneity of the group instead of the additive plurality as in French (Lo, Citation2015). By way of illustration, the use of -men does not trigger plural marking in other elements of the clause, c.f., laoshi xiang chuqu (teacher want go out) ‘the teacher wants to go out’ and laoshi-men xiang chuqu (teacher-pl want go out) ‘the teachers want to go out’. Under this definition, languages such as Mandarin Chinese do not represent an exception to the generalization as they only possess morphosemantic plural markers.
As a summary, two factors have been proposed to predict the distribution of sortal classifiers in language: the absence/occurrence of multiplicative bases and morphosyntactic plural markers. While both hypotheses have been investigated theoretically and empirically by previous studies, they have not been assessed together, even though they share a common theoretical basis. This paper thus attempts to fill this gap by taking both factors into account and evaluating their predictive power with regard to the distribution of sortal classifiers. The merge of the two probabilistic universals would result in the following statements:
If a language has sortal classifiers, multiplicative bases are expected in its numeral system. Therefore, if a language does not have multiplicative bases, it is not expected to have sortal classifiers.
If a language has morphosyntactic plural markers, multiplicative bases are expected in its numeral system. Therefore, if a language does not have multiplicative bases, it is not expected to have morphosyntactic plural markers.
Sortal classifiers and morphosyntactic plural markers are not expected within the same nominal structure in a language.
Since sortal classifiers and morphosyntactic plural markers belong to the same category of multiplicand, both entail that a language has multiplicative bases. This relation is only unidirectional, the presence of multiplicative bases therefore does not imply that a language necessarily has sortal classifiers and/or morphosyntactic plural markers. Finally, sortal classifiers and morphosyntactic plural markers tend not to co-occur in the same language; if they do, they are expected to not appear within the same nominal structure.
3. Methodology
In this section, we present the dataset used in our experiment and list the main features encoded and providing language examples. We then introduce the concept of conditional inference tree and random forests and also explain the methods of evaluating their output.
3.1. Materials
To ensure comparable and reproducible results, we apply the same dataset used in the two previous studies on multiplicative bases and morphosyntactic plural markers (Her et al., Citation2018; Tang et al., Citation2018). The dataset comprises of a sample of 400 languages weighted according to geographical and genealogical factors. For instance, since the Austronesian family accounts for 17.14% (1262/7363) of languages in the world (Lewis, Simons, & Fennig, Citation2009), the same ratio is applied in the dataset (19.00%, 76/400). Likewise for geographical factors: Since the Pacific region accounts for 18.74% (1380/7363) of the languages worldwide, a similar ratio is found in the dataset (18.50%, 74/400). This dataset is not an absolute representative of all 7363 languages of the world, but it is estimated to be sufficient for macro-analyzes. A visual representation of the 400 languages is shown in .
Each language in the dataset is annotated in terms of the features listed in . The features may be divided in the two main categories of grammatical information and metadata. Grammatical information relates to whether the language has morphosyntactic plural markers, multiplicative bases, and sortal classifiers. Metadata refers to the precise location of the language, along with its continent and genus affiliation. The last two features are included to assess the potential areal and genealogical effect on the distribution of sortal classifiers. Both genus and locations are extracted from the World Atlas of Language Structures, whereas the information of continent is based on Ethnologue. One major difference with previous studies is that we replaced the categorical variables of continent and genus by dummy variables. The main motivation for such change is that information tends to be lost when a large amount of levels are considered as one variable. For instance, there are in total 234 genera attested for the 400 languages of the dataset; considering them as one variable is costly in terms of computational resource and would fail to capture the importance of every individual level within the categorical variable. For instance, the categorical variable continent is represented by five variables instead of one, c.f., continent_Africa, continent_Americas, continent_Asia, continent_Europe, and continent_Pacific. Mandarin Chinese is located in Asia and thus has the value of 1 for continent_Asia and 0 for the four other dummy variables related to continent.
Table 1. Features encoded in the 400 languages of the dataset.
As a general example, French is annotated as yes for morphosyntactic plural, yes for multiplicative bases, and no for sortal classifiers. Examples of morphosyntactic plural have been given in Section 2. As for multiplicative bases, they are equally present in French, e.g. in deux cents (two hundred) ‘two hundred’ the multiplicand is represented by cent ‘hundred’; while sortal classifiers are not found in French. With regard to the metadata, French is affiliated to the Romance genus and pinpointed in continent_Europe geographically. The annotation of grammatical information is limited in the sense that it is restriction-type features. By way of illustration, the productivity of sortal classifiers is not distinguished cross-linguistically; thus, Chinese with obligatory sortal classifiers has the same value as Hungarian with optional sortal classifiers. Likewise in terms of inventory size and frequency across spoken and written data. Gradient data would probably provide additional insight to the subject (Corbett & Fedden, Citation2016; Grinevald, Citation2000) but for the current purpose of investigating the general distribution of grammatical features, this coding is considered sufficient.
3.2. Random Forests
The algorithm of random forests generates two main outputs: Conditional inference recursive partitioning trees and conditional permutation variable importance. Conditional inference tree is a method of regression and classification based on binary recursive partitioning (Breiman, Friedman, Stone, & Olshen, Citation1984), which is widely used in data mining and machine learning (Chen & Ishwaran, Citation2012, p.324) and has recently being applied in the field of linguistics (Levshina, Citation2015; Tagliamonte & Baayen, Citation2012). As a general method, these data are recursively partitioned in a binary pattern to form homogeneous groups. During this process, the model uses a bootstrap sample of the original data and randomly selects a subset of variables for each split instead of using all variables, so that the variance of the output is maintained as low as possible. The algorithm stops the partitioning process when no variables may split the data with statistical significance. The output can then be used to assess the interaction of the variables within the data. Based on the generated trees, the algorithm can then depict the relative importance of the predictors via conditional permutation-based variable importance, i.e. it allows us to rank the individual importance of variables. This ranking is obtained via random permutation in the out-of-bag data of the tree, from which the estimate of prediction error is calculated. The importance of variable is thus the average difference between the estimate and the out-of-bag error without permutation. The larger the importance of a variable, the more predictive it is. As a summary, inference trees would show how the variables interact with each other and their statistical significance within the data, whereas the importance of variable would display the relative ranking of the variables in terms of influencing power.
The main advantage of random forests is the use of permutation when retrieving p-values. The labels of data points are reshuffled randomly and the statistical test is applied for each shuffled data. The result is statistically significant if the proportion of the permutations providing a test statistic greater than or equal to the one observed in the original data is smaller than the significance level. This methodology can handle data with small quantity of observations and large number of possibly correlated variables, which usually represents a difficulty for conventional statistical tests (Tagliamonte & Baayen, Citation2012). Moreover, recursive partitioning can bypass several distributional assumptions and handle more easily the presence of outliers (Levshina, Citation2015, p.292).
The output of random forests can be evaluated by three methods: The index of concordance C, the Rand index, and the f-score. The index of concordance C is a generalization of the area under the receiver-operating characteristic curve (Harrell, Citation2001). It quantifies how the model discriminates the values of the response variable. The C-index ranges between 0 and 1, a value equals 0.5 shows a by-chance classification performance, whereas a value above 0.7 represents acceptable performance and above 0.8 indicates a good performance. The Rand index commonly generates similar output with the C-index and refers to the overall predictive accuracy of the model and is calculated by dividing the total number of correctly retrieved tokens by the total number of retrieved tokens (Rand, Citation1971). Then, the detailed performances are investigated category-internally to assess if one of the value of the response variable represented more difficulties for the classifiers, e.g. were classifier languages easier to identify than non-classifier languages. The two values of precision and recall are thus generated. Precision evaluates how many tokens are correct among all the output of the classifier, whereas recall quantifies how many tokens are correctly retrieved among all the expected correct output. The two measures assess different facets of the output, and are then combined into the f-score, which is equal to the harmonic mean of the precision and recall, i.e. 2(recall × precision)/(recall+ precision) (Ting, Citation2010). Finally, since the quantity of classifier and non-classifier languages is unbalanced within the dataset, we use the rules of majority-label prediction (Zero rule) as a benchmark of accuracy. To be more precise, since more non-classifier languages than classifier languages are attested in the dataset (69.75%, 279/400), the computational classifier could reach a prediction precision of 69.75% just by labelling all the 400 languages as non-classifier languages. We thus expect that the use of morphosyntactic plural markers and multiplicative bases as explanatory variables should at least exceed the accuracy of 69.75%.
4. Results
The calculation of the current Section is realized via the packages rms, randomForest, randomForestExplainer, and party (Harrell, Citation2015; Hothorn, Hornik, & Zeileis, Citation2006; Liaw & Wiener, Citation2002; Paluszynska, Citation2017) from R (R-Core-Team, Citation2018). First, in order to clarify the complex interaction of the predictors evaluated by the random forests, we tested the statistical model of conditional inference tree with sortal classifiers as response variable and the parameters of numeral bases plus morphosyntactic plurals as explanatory variables. Then, we added the geographical and genealogical factors as explanatory variables to investigate their interactive and individual effect on the prediction of sortal classifiers in language. Finally, we extracted the importance of each variable from the random forests.
displays the conditional inference tree obtained via Monte Carlo simulations when only including morphosyntactic plurals and multiplicative bases as explanatory variables. The variables that are statistically significant are listed in the upper nodes, which are able to divide the data into several buckets (Node two, four, and five). The buckets are coloured according to the ratio of classifier languages. For instance, Node 4 does not contain classifier languages and is thus in gray, whereas Node 5 contains approximately 60% of classifier languages coloured in black. The Figure shows that if a language does not have morphosyntactic plural (Node 1 to Node 3) and does have multiplicative bases (Node 3 to Node 5), it is statistically highly significant (p 0.001) that it is going to have classifiers. In other cases, it is unlikely to have classifiers (e.g. if the language has morphosyntactic plural, or if the language does not have morphosyntactic plural but does not have base).
Figure 2. Conditional inference tree with sortal classifiers as response variable and plural markers along with multiplicative bases as explanatory variables.
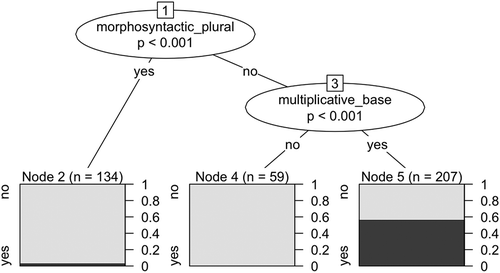
The C-statistic of the current model is 0.82, which infers excellent discrimination, as the model can explain nearly 80% of the data. Likewise, the Rand index equals 76.5 and shows higher accuracy than the Zero rule (69.75%). Yet, we also need to scrutinize the classification performance in terms of precision and recall. As shown in , the recall of non-classifier language (67.7%) is much lower than the recall of classifier languages (96.7%), whereas the precision of classifier languages (56.5%) is much lower than the precision of non-classifier languages (97.9%). This shows that the model tended to over-predict languages as having sortal classifiers and be too conservative in predicting languages as non-classifier languages. This process thus resulted in a wide quantity of languages being predicted as classifier languages while they were not (c.f., low precision and high recall of ‘with classifiers’) and very few languages being interpreted erroneously as non-classifier languages (c.f., high precision but low recall of ‘no classifiers’). As a summary, the model was able to reach an overall good performance based on only two explanatory variables; however, the analysis of precision and recall shows that the model tends to inflate the amount of classifier languages.
Table 2. Precision and recall with plural markers and multiplicative bases as explanatory variables.
As a second step, we include the geographical and genealogical factors as explanatory variables. Moreover, we further investigate the individual and interactive importance of each variable. displays the conditional inference tree generated via Monte Carlo simulations when adding geographical and genealogical factors in the analysis (the continent and genus features in ). We see a strong geographical effect as the continent factor is located at the top of the root. In other words, the model can identify the majority of the classifier languages just by selecting languages located in Asia. For languages found in Asia, the interaction observed in still holds as languages with morphosyntactic plurals tend not to have sortal classifiers (p 0.001). However, for languages not affiliated to the Asia region, the effect of genus seems to be stronger than the effect of morphosyntactic plurals. Most classifier languages outside of Asia are mostly found in the Oceanic genus (i.e. the Austronesian language family), the conditional inference tree thus displays that this feature is by itself sufficient to identify classifier languages outside of Asia with high precision. Finally, the variable of multiplicative bases is not shown in the tree, which means that it is not considered to have predictive powers as strong as the variables included in the current tree.
Figure 3. Conditional inference tree with sortal classifiers as response variable and morphosyntactic plural markers, multiplicative bases, continent, and genus as explanatory variables.
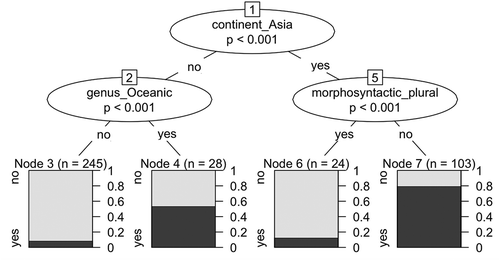
The C-statistic of the random forests rises to 85.4, and its Rand index elevates to 85.5, showing an improvement in the predictive power of the model when geographical and genealogical factors are included. As for precision and recall, shows a major improvement in the recall of non-classifier languages (67.7 to 87.7) and the precision towards identifying classifier languages (56.5 to 74.1), which were the main limitations of the first model. While the recall of classifier languages and the precision of non-classifier languages slightly decrease, the overall performance (f-score) is improved for both classifier and non-classifier languages.
Table 3. Precision and recall when adding geographical and genealogical factors as explanatory variables.
Finally, we also extract the individual importance of variables by sorting the relative importance of the predictors via conditional permutation-based variable importance. In other words, the analysis by conditional inference tree in showed the most relevant variables when considering the interaction of all the variables. However, we still need to investigate the individual importance of each variable, i.e. a variable could have a strong effect but not be shown on the conditional inference tree due to a slight difference of predictive power with the listed variables or a weakened effect when interacting with other variables. The predictors include the features listed in , i.e. morphosyntactic_plural, multiplicative_base, continent, and genus. shows the frequency of minimal depth for each variable across all the trees generated by the random forests and its mean. The minimal depth refers to how far is the node with the variable from the root node. A small value indicates that the variable is frequently represented as the root node (or a top node in the tree) and is thus more important. We only list here the ten variables with the smallest mean minimal depth. Morphosyntactic_plural is by far the most important variable, followed by multiplicative_base and continent_Asia. Some predictivity is detectable for other geographical and genealogical factors, but their minimal depth is relatively bigger than the top three variables. We may therefore infer that even though multiplicative bases are not showing on the conditional inference tree of , the variable still plays a significant role in the distribution of sortal classifiers in languages of the world, whereas the areal effect of ‘classifiers in Asia’ is once more observed.
This observation is equally attested in different measures. By way of illustration, shows the importance of variables sorted according to their effect on the accuracy and purity of nodes. The mean decrease of accuracy refers to how worse the model performs without each variable; a high decrease thus indicates that the variable has a strong predictive power. The mean decrease of the Gini coefficient shows how each variable contributes to the homogeneity of the nodes and the end of the tree, i.e. can this variable contribute to clearly separated buckets. Again, a high decrease of Gini coefficient when removing a variable indicates that this variable has a strong predictive power and therefore a high importance. In both measures, the variables morphosyntactic_plural, multiplicative_base, and continent_Asia are consistently at the top, which further supports our observations in . Moreover, all measures also show that the variable of morphosyntactic plurals is stronger in terms of predictive power than the variable of multiplicative bases.
Figure 5. Importance of the variables with sortal classifiers as response variable and morphosyntactic plural markers, multiplicative bases, continent, and genus as explanatory variables.
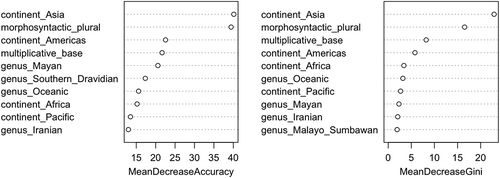
Finally, an overview of the importance of variables is displayed in . The x-axis represents the mean minimal depth of each variable, the y-axis points out the frequency that a variable is used to split the root node, and the size of the bubbles indicates the total number of nodes that use the variable for splitting. The top ten important variables are labelled and highlighted in blue. The three variables being used the most as root nodes and being included the most frequently across all the generated trees are still morphosyntactic_plural, multiplicative_base, and continent_Asia.
As a summary, the variables of morphosyntactic plurals and multiplicative bases can predict the occurrence/absence of sortal classifiers in language with high precision. Among these two variables, morphosyntactic plurals show stronger predictive power than multiplicative bases. Adding geographical and genealogical factors as variables improves the performance of the model and demonstrates that sortal classifiers are subject to a strong areal affect as most classifier languages are found in Asia, whereas the genealogical effect is of a minor nature.
5. Discussion
The main research goal is to investigate the three hypotheses obtained by combining the implicational universals proposed by previous studies. The first hypothesis states that if a language has sortal classifiers, multiplicative bases are expected in its numeral system, whereas languages without multiplicative bases are not expected to have sortal classifiers. The second hypothesis states the same parameter for morphosyntactic plural markers due to their shared syntactic nature with sortal classifiers. The third hypothesis then claims the complementary-like distribution between morphosyntactic plural markers and sortal classifiers. Our results in Section 4 directly support the first and third hypotheses. As shown in the conditional inference tree in and further displayed in the detailed numbers in , most classifier languages (96.7%,117/121) are with multiplicative bases and without morphosyntactic plurals.
Table 4. Distribution of grammatical features within the 121 classifier languages of the dataset.
With regard to the second hypothesis, our results in and did not show multiplicative base as a node relevant to the distribution of morphosyntactic plurals, which infers that multiplicative bases are less relevant for languages with morphosyntactic plurals. Such speculation is first supported by the analysis of non-classifier languages in . We observe that even though only 7.2% (20/279) of the languages show counter examples to the second hypothesis (i.e. languages with plural markers but without multiplicative bases), the majority of the languages (53.4%, 149/279) are without morphosyntactic plural markers and spread across languages with and without multiplicative bases. Even though this does not represent a counter example to the second hypothesis, we expect that the correlation between morphosyntactic plural markers and multiplicative bases is weaker than the correlation between sortal classifiers and multiplicative bases.
Table 5. Distribution of grammatical features within the 279 non-classifier languages of the dataset.
This is further shown by running the conditional inference tree and random forests with morphosyntactic plural markers as response variable (). The effect of sortal classifiers and multiplicative bases as explanatory variables is statistically significant (p 0.001); however, the C-statistic (77.1) and Rand-index (71.5) of the model only reach the threshold of good discrimination. We also observe that the variable of multiplicative bases does not have a strong predictive power, as the ratio of languages with morphosyntactic plural markers in Node 3 and Node 4 is not very different (), i.e. even though the variable has a significant effect in terms of probability, the effect size is small. Moreover, the variable of multiplicative bases is not statistically significant when geographical and genealogical factors are taken into account (). On the other hand, we observe a strong areal affect as within non-classifier languages, languages located in Asia are more likely to have plural markers, followed by the Americas and the Pacific.
The ranking of variables according to their mean decrease of accuracy () and mean decrease of Gini coefficient () equally demonstrates that while the variable of sortal classifiers consistently plays a prominent role (ranked first and fourth, respectively) in the prediction of morphosyntactic plural markers, the variable of multiplicative bases only have a minor effect as it is only ranked 12th and 18th in both measures. Likewise in terms of mean minimal depth: the mean minimal depth of sortal classifiers is the smallest among all variables with 8.93, whereas the mean minimal depth of multiplicative bases is 21.61.
Figure 8. Importance of the variables with morphosyntactic plural markers as response variable and sortal classifiers, multiplicative bases, continent, and genus as explanatory variables.
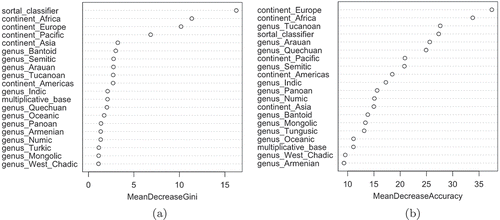
However, we should point out that even though the statistical analyzes indicate that the correlation between morphosyntactic plurals and multiplicative bases is not as strong as theoretically expected, it may be worthwhile to further investigate the 20 languages in the current dataset that violate this correlation, most of which are minority languages in South America and some in Africa, to make sure that their indigenous numeral systems genuinely lack of multiplicative bases and thus that the absence of multiplicative bases is not due to language contact and the borrowing of multiplicative bases from the dominant language in contact, e.g. Spanish in the South American context. Should some of these languages have indigenous multiplicative bases historically, a revised dataset might validate this correlation after all.
To sum up, the output of random forests supports the first and the third hypothesis, and can be summarized as follow: if a language has sortal classifiers, it tends to have multiplicative bases and not to have morphosyntactic plural markers. However, the second hypothesis assumes the unification of sortal classifiers and morphosyntactic plural markers and suggests that if a language has morphosyntactic plural markers, it tends to have multiplicative bases. This hypothesis is not fully supported by our results, as the variable of multiplicative bases has very low predictive power with regard to the absence/existence of morphosyntactic plural markers in language when taking into account geographical and genealogical effects. Finally, our results provide novel data and insight to the distribution of sortal classifiers in languages of the world; however, such results may relate to more than one linguistic theory that can explain the correlation patterns identified in this study. As an example, it applies equally to the Greenberg-Sanches-Slobin generalization or the count-mass hypothesis chierchia_plurality_1998. Further features are thus required to investigate the individual predictive power of each theory.
6. Conclusion
In this study, we demonstrate how computational methods can be applied to linguistic hypotheses. Specifically, the model of random forests is able to reveal the interaction pattern of linguistic variables along with their individual importance under various measures. Such a methodology allows a multifaceted approach of linguistic theories and provides a ranking of variables in terms of importance rather than an arbitrary clear-cut division. Our results are partially consistent with existing linguistic hypotheses as multiplicative bases and morphosyntactic plural markers have a strong predictive power with regard to the absence/occurrence of sortal classifiers in a language, even when taking into account geographical and genealogical effects. However, the results from the statistical analysis indicate that the correlation between morphosyntactic plurals and multiplicative bases is not as strong as theoretically expected, and we suggest that further studies can look into the 20 languages that violate this correlation to make sure that the absence of numeral bases in each of these languages is genuine.
Acknowledgments
We thank the two anonymous reviewers for their constructive comments, which led to significant improvements of the paper. All remaining errors are our own.
Disclosure statement
No potential conflict of interest was reported by the authors.
Additional information
Funding
Notes
1. Sortal classifiers are semantically and syntactically different from mensural classifiers (e.g. san shuang xiezi (three mens-pair shoe) ‘three pairs of shoes’ in Mandarin Chinese) and measure terms (e.g. three bottles of wine in English). This paper is concerned with sortal classifiers shown in (1). For further discussion on the differentiation of these three categories, refer to Aikhenvald (Citation2000), Her (Citation2012a), and Kilarski (Citation2014).
2. As a disclaimer, mensural classifiers such as san ping shui (three mens-bottle water) ‘three bottles of water’ in Mandarin Chinese are different from sortal classifiers, even though they are two subtypes of the same syntactic category known as ‘numeral classifiers’. This paper only discusses sortal classifiers. For further references on this distinction, please refer to Aikhenvald (Citation2000) and Her (Citation2012a).
3. This implication is only unidirectional, i.e. languages with sortal classifiers tend to have multiplicative bases but clearly not all languages with multiplicative bases have sortal classifiers.
4. While the grammaticality of such examples is still subject to debate in Sinitic studies, it still represents a potential counter-example to the generalization (Lo, Citation2015).
References
- Aikhenvald, A. Y. (2000). Classifiers: A typology of noun categorization devices. Oxford: Oxford University Press.
- Aikhenvald, A. Y. (2016). Gender, shape, and sociality: How humans are special in Manambu. International Journal of Language and Culture, 3(1), 68–89.
- Au Yeung, W. H. B. (2005). An interface program for parameterization of classifiers in Chinese. PhD dissertation, Hong Kong University of Science and Technology, Hong Kong.
- Au Yeung, W. H. B. (2007). Multiplication basis of emergence of classifiers. Language and Linguistics, 8(4), 835–861.
- Bisang, W. (2012). Numeral classifiers with plural marking: A challenge to Greenberg. In D. Xu (Ed.), Plurality and classifiers across languages of China (pp. pages 23–42). Berlin: De Gruyter Mouton.
- Borer, H. (2005). Structuring Sense, part I. Oxford: Oxford University Press.
- Breiman, L., Friedman, J., Stone, C. J., & Olshen, R. (1984). Classification and regression trees. Taylor & Francis, New York: Chapman & Hall.
- Chen, X., & Ishwaran, H. (2012). Random forests for genomic data analysis. Genomics, 99(6), 323–329.
- Chierchia, G. (1998). Plurality of mass nouns and the notion of semantic parameter. In S. Rothstein (Ed.), Events and grammar (pp. 53–104). Dordrecht: Kluwer.
- Comrie, B. (2006). Numbers, language, and culture. Jyvaskyla. Paper presented at the 16th Jyväskylä Summer School, July 24–August 11, 2006. Jyväskylä: University of Jyväskylä.
- Comrie, B. (2013). Numeral bases. In M. S. Dryer & M. Haspelmath (Eds.), The world atlas of language structures online. Leipzig: Max Planck Institute for Evolutionary Anthropology. Retrieved from https://wals.info/chapter/131
- Corbett, G. G. (2013). Number of Genders. In M. S. Dryer & M. Haspelmath (Eds.), The world atlas of language structures online. Leipzig: Max Planck Institute for Evolutionary Anthropology. Retrieved from https://wals.info/chapter/30
- Corbett, G. G., & Fedden, S. (2016). Canonical gender. Journal of Linguistics, 52(3), 495–531.
- Csirmaz, A., & Dekany, E. (2010). Hungarian classifiers. Rome. Paper presented at the Conference on Word classes: Nature, typology, computational representation, March 24–26, 2010. Rome: Roma Tre University.
- Dixon, R. M. W. (1986). Noun class and noun classification. In C. Craig (Ed.), Noun classes and categorization (pp. pages 105–112). Amsterdam: John Benjamins.
- Doetjes, J. (2012). Count/mass distinctions across languages. In C. Maienborn, K. V. Heusinger, & P. Portner (Eds.), Semantics: An international handbook of natural language meaning, part III (pp. 2559–2580). Berlin: Mouton de Gruyter.
- Fromkin, V., Rodman, R., & Hyams, N. (2011). An introduction to language. Boston: Wadsworth, Cengage Learning.
- Gerner, M. (2006). Noun classifiers in Kam and Chinese Kam-Tai languages: Their morphosyntax, semantics and history. Journal of Chinese Linguistics, 34(2), 237–305.
- Ghomeshi, J. (2003). Plural marking, indefiniteness, and the noun phrase. Studia Linguistica, 57(2), 47–74.
- Gil, D. (2013). Numeral classifiers. In M. S. Dryer & M. Haspelmath (Eds.), The world atlas of language structures online. Leipzig: Max Planck Institute for Evolutionary Anthropology. Retrieved from https://wals.info/chapter/55
- Greenberg, J. H. (1990a). Generalizations about numeral systems. In K. Denning & S. Kemmer Eds. On language: Selected writings of Jeseph H. Greenberg. Stanford: Stanford University Press. 271–309.[Originally published 1978 in Universals of Human Language, ed by Joseph H. Greenberg, Charles A. Fergson, & Edith A. Moravcsik, Vol 3, 249–295. Stanford; Stanford University Press.]
- Greenberg, J. H. (1990b). Numeral classifiers and substantival number: Problems in the genesis of a linguistic type. In K. Denning & S. Kemmer Eds. On language: Selected writings of Joseph H. Greenberg Stanford: Stanford University Press. 166–193.[First published 1972 in Working Papers on Language Universals 9. 1-39. Stanford, CA: Department of Linguistics, Stanford University.].
- Grinevald, C. (2000). A morphosyntactic typology of classifiers. In G. Senft (Ed.), Systems of nominal classification (pp. 50–92). Cambridge: Cambridge University Press.
- Grinevald, C. (2015). Linguistics of classifiers. In J. D. Wright (Ed.), International Encyclopedia of the Social & Behavioral Sciences (pp. pages 811–818). Oxford: Elsevier.
- Harrell, F. (2001). Regression modeling strategies. New York: Springer.
- Harrell, F. (2015). Regression modeling strategies: With applications to linear models, logistic and ordinal regression, and survival analysis. Dordrecht: Springer.
- Her, O.-S. (2012a). Distinguishing classifiers and measure words: A mathematical perspective and implications. Lingua, 122(14), 1668–1691.
- Her, O.-S. (2012b). Structure of classifiers and measure words: A lexical functional account. Language and Linguistics, 13, 1211–1251.
- Her, O.-S. (2017). Deriving classifier word order typology, or Greenberg’s Universal 20a and Universal 20. Linguistics, 55(2), 265–303.
- Her, O.-S., & Lai, W.-J. (2012). Classifiers: The many ways to profile one, a case study of Taiwan Mandarin. International Journal of Computer Processing of Oriental Languages, 24(1), 79–94.
- Her, O.-S., Tang, M., & Li, B.-T. (in press). Word order of numeral classifiers and numeral bases: Harmonization by multiplication. Language Typology and Universals.
- Hothorn, T., Hornik, K., & Zeileis, A. (2006). Unbiased recursive partitioning: A conditional inference framework. Journal of Computational and Graphical Statistics, 15(3), 651–674.
- Jenks, P. (2017). Numeral classifiers compete with number marking: Evidence from Dafing. Austin. Paper presented at the Linguistic Society of America 2017 Annual Meeting, January 5–8, 2017. Austin: JW Marriott Austin.
- Kemmerer, D. (2017). Some issues involving the relevance of nominal classification systems to cognitive neuroscience: Response to commentators. Language, Cognition and Neuroscience, 32(4), 447–456.
- Kibort, A., & Corbett, G. G. (2008). Number: Grammatical features. Guildford: University of Surrey.
- Kilarski, M. (2014). The Place of Classifiers in the History of Linguistics. Historiographia Linguistica, 41(1), 33–79.
- Kim, K., & Melchin, P. B. (2018). On the complementary distribution of plurals and classifiers in East Asian classifier languages. Language and Linguistics Compass, 12(4), 1–22.
- Levshina, N. (2015). How to do linguistics with R: Data exploration and statistical analysis. Amsterdam: John Benjamins.
- Lewis, P., Simons, G. F., & Fennig, C. D. (2009). Ethnologue: Languages of the world. Dallas: SIL International.
- Liaw, A., & Wiener, M. (2002). Classification and regression by randomForest. R News, 2(3), 18–22.
- Lo, Y. C. (2015). Plural marker -men and numeral classifiers: Convergence and divergence. Master’s thesis, National Chengchi University, Taipei.
- Paluszynska, A. (2017). Structure mining and knowledge extraction from random forest with applications to the cancer genome atlas project. Master’s thesis, University of Warsaw, Warsaw.
- Peyraube, A. (1998). On the history of classifiers in Archaic and Medieval Chinese. In B. K. T’sou (Ed.), Studia linguistica serica (pp. pages 131–145). Hong Kong: City University of Hong Kong.
- Rand, W. M. (1971). Objective Criteria for the Evaluation of Clustering Methods. Journal of the American Statistical Association, 66(336), 846–850.
- R-Core-Team. (2018). R: A language and environment for statistical computing. Vienna: R Foundation for Statistical Computing.
- Rijkhoff, J. (2000). When can a language have adjectives? An implicational universal. In P. M. Vogel & B. Comrie (Eds.), Approaches to the typology of word classes (pp. 217–257). Berlin: De Gruyter Mouton.
- Sanches, M., & Slobin, L. (1973). Numeral classifiers and plural marking: An implicational universal. Working Papers in Language Universals, 11, 1–22.
- Seifart, F. (2010). Nominal classification. Language and Linguistics Compass, 4(8), 719–736.
- T’sou, B. K. (1976). The structure of nominal classifier systems. Oceanic Linguistics Special Publications, 13, 1215–1247.
- Tagliamonte, S. A., & Baayen, H. (2012). Models, forests, and trees of York English: Was/were variation as a case study for statistical practice. Language Variation and Change, 24, 135–178.
- Tang, M. (2017). Explaining the acquisition order of classifiers and measure words via their mathematical complexity. Journal of Child Language Acquisition and Development, 5(1), 31–52.
- Tang, M., Her, O.-S., & Chen, Y.-R. (in press). Insights on the greenberg-sanches-slobin generalization: Quantitative typological data on classifiers and plural markers. Folia Linguistica.
- Ting, K. M. (2010). Precision and Recall. In C. Sammut & G. I. Webb (Eds.), Encyclopedia of machine learning (pp. 781). Boston, MA: Springer US.
- Vogel, P. M., & Comrie, B. (editors). (2000). Approaches to the typology of word classes. Number 23 in Empirical approaches to language typology. New York: Mouton de Gruyter, Berlin.
- Wu, F., Feng, S., & Huang, C. T. J. (2006). Hanyu shu+lianhg+ming geshi de laiyuan [On the origin of the construction of numeral+classifier+noun in Chinese]. Zhongguo Yuwen [Studies of the Chinese Language], 5, 387–400.
- Yi, B. U. (2009). Chinese classifiers and count nouns. Journal of Cognitive Science, 10, 209–225.
- Yi, B. U. (2011). What is a numeral classifier? Philosophical Analysis, 23, 195–258.
- Zhang, N. N. (2013). Classifier structures in Mandarin Chinese. Berlin: Mouton de Gruyter.