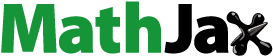
ABSTRACT
A digital transformation is currently taking place in society, where people and things are connected to each other and the Internet. The number of connected devices is projected to be 28 Billion in 2025, and our expectations on digitalization set new requirements of mobile communication technology. To handle the increased amount of connected devices and data generated, the next generation of mobile communication technology is under deployment: 5G-technology.
The manufacturing industry follows the digital transformation, aiming for digitalized manufacturing with competitive and sustainable production systems. 5G-technology meets the connectivity requirements in digitalized manufacturing, with low latency, high data rates, and high reliability. Despite these technological benefits, the question remains: Why should the manufacturing industry invest in 5G-technology?
This study aims to determine the impact of 5G-technology on manufacturing performance; based on a mixed-methods approach including a modified TOPSIS method to ensure robustness of the results. The results show that 5 G-technology will mainly impact productivity, maintenance performance, and flexibility. By linking 5G-technology to the performance of the manufacturing system, instead of focusing on network performance, the benefits of using 5G-technology in manufacturing become clear, and can thus facilitate investment and deployment of 5G-technology in the manufacturing industry.
1. Introduction
Innovations and advancements in technology are changing our society to become more digitalized. The terms ‘Internet of Things’ (IoT) and ‘Internet of Everything’ (IoE) (Miraz et al. Citation2015), where people and things are connected to the Internet, are realized as the digitalized society is developed. In this digitalized society are smartphones used to control households, it is possible to meet and socialize over the Internet, and there are expectations on autonomous cars and a possibility to control a factory from home. As more and more devices are being connected, this development constantly demands higher data rates. The number of IoT devices available is projected to be 28 billion in 2025 (Lueth Citation2020), which sets new requirements on mobile communication technology. The next generation of mobile communication technology, 5G-technology, is expected to meet these requirements, and thus amplify our digitalized society.
The manufacturing industry is not an exception in our digitalized society. Innovation and new technologies are reshaping the manufacturing industry as well as towards digitalized manufacturing. Digitalized manufacturing, for example, Industry 4.0, refers to production systems that rely on computer science and advanced manufacturing technology (Xu, Xu, and Li Citation2018). The systems are anticipated to act decentralized and autonomous (Hermann, Pentek, and Otto Citation2016), setting requirements on a reliable, fast, and safe network (Cheng et al. Citation2018; Rao and Prasad Citation2018). 5G-technology has the characteristics to solve the technological challenges of communication in digitalized manufacturing (Palattella Citation2016; Rao and Prasad Citation2018; Li, Da Xu, and Zhao Citation2018). However, it is not yet clear how this technology will benefit the manufacturing industry. Experts in mobile communication are using technological characteristics and network performance to explain the benefits of 5 G-technology (Peuster Citation2019; Morgado et al. Citation2018) while manufacturing experts are interested in the performance of the manufacturing plant. The mismatch in perspectives and domain knowledge is making it unclear how 5G-technology will contribute to performance within manufacturing, and thus why the manufacturing industry should invest in 5G-technology. There is a need to determine how the implementation of 5G-technology can benefit manufacturing performance. Hence, this study aims to answer the research question:
RQ: Which key characteristics of 5 G-technology have the highest significant impact on manufacturing performance?
To explore the opportunities of using 5G-technology in manufacturing, an initiative was taken by global manufacturing companies, a global telecommunication company, and a university to form a research project called 5G Enabled Manufacturing (5GEM). The shop floor at the two manufacturing case companies has been supplied with a Long-Term Evolution (LTE) network with 5G-technologies, aiming to demonstrate the usage of 5G-technology. Eight industrial demonstrators were developed during the 5 GEM project to demonstrate and investigate 5G-technology and its application opportunities more specifically. The eight industrial demonstrators were developed to serve real industrial needs and to be scalable solutions. Numerous previous publications have presented and explained the results from the project to serve the understanding of how 5G can support manufacturing, including (Åkerman et al. Citation2018b, Lundgren et al. Citation2017; Bärring, Johansson, and Stahre Citation2020,; Bärring et al. Citation2021). This study used the eight industrial demonstrators from the 5 GEM project to determine the impact of 5G-technology on performance indicators in industrial applications. This paper is structured as follows: first, a theoretical background is presented, followed by a description of the methodology. Next, the results are presented including a description of each demonstrator, the key characteristics of 5G-technology, and their impact on industrial performance indicators. Finally, the study is summarized in the discussion and conclusion sections, including contributions, limitations of the study, and proposed future research.
2. Theoretical background
Innovations and advancements in technology have started to reshape the manufacturing industry towards digitalized manufacturing. Digitalized manufacturing (also known as the German initiative Industry 4.0) relies on computer science and advanced manufacturing technology (Da Silva et al. Citation2020; Xu, Xu, and Li Citation2018) anticipated to radically change the manufacturing industry and increase performance (Hermann, Pentek, and Otto Citation2016; Dalenogare et al. Citation2018). Digitalized manufacturing is characterized by interconnected systems (machines, sensors, equipment, and people are connected to each other), transparency of information, and decentralized decisions (Hermann, Pentek, and Otto Citation2016) enabled by technologies such as big data, cyber-physical systems (CPS), IoT and cloud computing (Lu Citation2017). This includes digital integration across the hierarchical levels (i.e. from sensors level to corporate planning levels) to ensure data communicability among the manufacturing resources. It includes uninterrupted data flow among humans and machines, aiming to improve decision-making (Parhi, Joshi, and Akarte Citation2021). The role of operators in such a production system is to be a flexible problem solver supported by technology (Hermann, Pentek, and Otto Citation2016). The information flow between equipment and people generates a lot of data, which requires high data rates (Cheng et al. Citation2018; Rao and Prasad Citation2018) and low latency are crucial for actions in time-critical control loops (Rao and Prasad Citation2018). Relatively high data rate and low latency can be delivered by wired communication technology (e.g. Ethernet). However, wireless communication technology will play an important role in Industry 4.0 (Hermann, Pentek, and Otto Citation2016) as scalability and flexibility/mobility are needed for most of the new applications (Rao and Prasad Citation2018). The new applications in the manufacturing industry set new requirements for communication technology.
2.1. Connectivity and definition of 5G
5G will support large throughput of data over wireless communications technology, i.e. broadband services provided over-the-air instead of through cable connections, which is one of the main differences with 5G compared to previous generations of telecommunication. The 5G-technology will deliver 1000 times higher data volumes, 100 times higher data rates, 10 times lower energy consumption, and 5 times lower end-to-end latency compared to earlier generations, and at least 99.999% service reliability (Agyapong et al. Citation2014; Rao and Prasad Citation2018). These characteristics will be needed for several IoT applications (Palattella et al. Citation2016; Rao and Prasad Citation2018; Li, Da Xu, and Zhao Citation2018). To phase these requirements from 2020 and beyond, 5G has been equipped with a portfolio of access and connectivity solutions. The already existing technologies will be incorporated in the 5 G solution complemented by new radio access concepts. The new concepts include Massive Multiple-Input, Multiple-Output, Ultra-Dense Networks, Moving Networks, Device-to-Device, Ultra-Reliable, and Massive Machine Communications (Osseiran et al. Citation2014). Thus, 5G has the potential to help the manufacturing industry in upgrading to digitalization (Cheng et al. Citation2018; Rao and Prasad Citation2018; Li, Da Xu, and Zhao Citation2018). Five scenarios where 5G will be a decisive technology have been identified in the project METIS (Osseiran et al. Citation2014):
Amazingly fast: the connection should be experienced instantaneously without any notable delays for a user.
Great service in a crowd: mobile broadband will be available even in more crowded situations with a reasonable service.
Best experience following you: a high level of service should be delivered to the user also while traveling or being on the way.
Super-real time and reliable connections: for applications (for example mission-critical control) 5G will provide a reliable network with low latency in the communication.
Ubiquitous things communicating: more connected devices should be handled in an effective manner where requirements can vary to a large extent. This can imply requirements for scalability and flexibility in the network.
2.2. Applications of 5 G-technology in manufacturing
Billions of sensors, machine-controlled robots, and autonomous logistics which can operate remotely in real-time are anticipated to contribute to productivity improvements in the manufacturing industry (Rao and Prasad Citation2018). Thanks to the development of 5G-technology, these smart systems have become more powerful for ubiquitous connectivity on the shop floor (Turner et al. Citation2019). Therefore, 5G-technology will bring faster and more reliable communication between machines, sensors, and computing systems, resulting in a more flexible and productive manufacturing system (Rao and Prasad Citation2018). Digital Catapult (Citation2019) reports a productivity improvement of 2%. Much research has been conducted to describe possible scenarios where 5G-technology plays an important role, and some examples are summarized in with an explanation of the requirements of 5G technology to fulfill this.
Table 1. Summary of the application area and the involved requirements of 5 G-technology
5G-technology brings characteristics that have the potential to make the technology applicable to a broader area of use cases () than was previously possible for mobile networks. Use cases where time-critical aspects or connecting ubiquitous things are of interest can be supported by 5 G-technology. Still, there are some barriers to implementing 5 G-technology involving the costs involved and expected return on investment (ROI), lack of competence in the new technology, and where to start, but also concerns about IT security (Digital Catapult Citation2019). Another aspect that makes it more difficult for a successful implementation of new technology, is that developers and business leaders tend to focus on the potential of technology that can collect and analyze big volumes of data rather than on the expected outcome of applying the technology (Patel, Shangkuan, and Thomas Citation2017). Therefore, in order to identify the right problems to address in an organization, which can be supported by more and better data enabled by the technology, the domain expert stays important. This person can with the deep knowledge and expertise in an area identify where the biggest opportunities and challenges are (McAfee and Brynjolfsson Citation2012). To design and build a data value chain in manufacturing (incorporating Big Data applications) knowledge needs to be developed about the digital technologies in combination with the already existing expertise of the manufacturing processes (Åkerman et al. Citation2018a). Based on these mentioned considerations that need to be taken into account, 5G-technology will only be fully realized with its full potential if the value gained by the technology can exceed the deployment costs of the network at the same time as the Quality of Service (QoS) requirements by the end-user can be met (Idachaba Citation2016).
2.3. Performance measures and indicators
When it comes to the performance of 5 G-technology, most research focuses on setting requirements of the network, or discuss its architecture, and neglect the practical implementations (Peuster et al. Citation2019). Requirements on performance are general about peak data rate, energy efficiency, mobility, mobile interruption time, connection density (i.e. the number of connected devices), area traffic capacity, etc. (Morgado et al. Citation2018). In contrast, performance in the manufacturing industry is related to the ability to implement a chosen strategy (Neely, Gregory, and Platts Citation1995). Performance measurement usually consists of several performance indicators (PIs) (Kang et al. Citation2016), for example, the number of products produced, production cost per product, mean time between failure (MTBF), and cycle time. Performance is measured in many industrial companies to support operations and make sure that actions are taken to support the strategies and objectives of an organization (Lynch and Cross Citation1991). Consequentially, 5G-technology’s impact on performance in the manufacturing industry should thus be associated with how 5G-technology can contribute to the objectives of the organization. There is a need to investigate the outcome of the technology (Patel, Shangkuan, and Thomas Citation2017) and PIs have the potential to be used for evaluation on how technology can contribute to performance in manufacturing.
3. Methodology
This study is based on a mixed-methods approach (Creswell Citation2014), combining quantitative and qualitative data to study the projected impact on 5G-technology in the manufacturing industry. Eight demonstrators (demos) from the research project 5GEM, aiming to demonstrate different applications of 5G-technology in the manufacturing industry, were included in the study. The eight demonstrators are about: 1) planning and design of the radio network, 2) design of network and cloud architecture for the 5G-pilot-network, 3) connectivity for stationary equipment, 4) connectivity to mobile equipment and people, 5) large-scale deployment of 5G-technology at one manufacturing plant, 6) analytics of data collected via the 5G-pilot-network, 7) manufacturing control tower, and 8) operators use of 5G-technology. The demonstrators are explained more in detail in chapter 4, Results and Analysis. An overview of the method is given in .
Figure 1. The figure gives an overview of the methodology, including data collection and data analysis of quantitative and qualitative data.

Firstly, a literature selection was conducted to collect characteristics of 5G-technology and industrial PIs for the creation of an evaluation matrix. Secondly, interviews with four experts were conducted to collect data on the projected effects of 5G-technology and its impact on the manufacturing industry. Next, the data were analyzed for each of the demos. Lastly, the data were analyzed, including all demos. The following sections describe the data collection and data analysis in detail.
3.1. Data collection
Data collection could be divided into two parts: (1) collection of characteristics for 5G-technology and industrial PIs from literature to create an evaluation matrix and (2) evaluation of 5G-technology and impact in industrial PIs with the help of the evaluation matrix. An overview of the data collection is given in .
Figure 2. The figure describes the data collection procedure. Firstly, data from the literature was used to create an evaluation matrix. Secondly, the evaluation matrix was then used during an interview setting to collect quantitative data. Qualitative data were also collected during the interview by posting open questions.
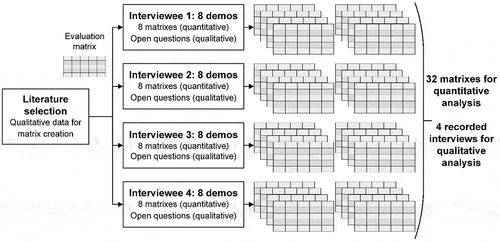
Firstly, a literature selection was conducted to get a gross list of PIs. The PIs were divided into five categories: productivity, quality, maintenance performance, flexibility, and sustainability. Three PIs from each category were selected to be used in the evaluation matrix, based on the goal of the 5GEM project and the authors’ experience of PIs in industry. Literature was also used to identify relevant characteristics of 5G-technology to use in the evaluation matrix. These 5G-characteristics were cross-checked with 5G-experts in the 5GEM project. The aim of the literature search and selection was not to make a proper review of the literature but to find relevant 5G-characteristics and PIs for the evaluation. This resulted in a matrix with eleven characteristics of 5G-technology on one side and fifteen industrial PIs on the other side. The evaluation matrix is presented in Appendix A.
Secondly, the evaluation of 5G-technology and its impact on industrial PIs were based on interviews and a modified TOPSIS method. There are no explicit rules in the literature for a requisite number of experts from which you collect the response in TOPSIS methodology. Instead, the respondents’ knowledge and professional expertise about the subject matter is of importance. Thus, purposive sampling (Palys Citation2008) was used to select interviewees, as it allows the selection of interviewees based on their knowledge and experiences about the subject under investigation. For determining the impact of 5G-technology on the industrial demos from the 5GEM-project, three criteria were used to select interviewees: (1) domain knowledge about manufacturing, (2) knowledge about 5G-technology, and (3) familiarity with the eight demos included in the study. Four interviewees were selected. presents information about the interviewees.
Table 2. Information about the interviewees including branch, their working title/role, and years of experience
The four interviewees estimated (independently of each other) how each 5G-characteristic will impact each PI respectively, by filling the matrix. Numbers between 1 and 5 were used for the impact; 1 represents a low impact and 5 represents a high impact. This was done for each of the eight demonstrators, resulting in thirty-two quantitative matrixes to analyze. The interviews ended with more open-ended questions about the value of 5G-technology in manufacturing in general, and for the eight demos in particular, to complement the quantitative data with qualitative data. Qualitative data collection methods allow an in-depth understanding of a phenomenon (Creswell Citation2014). Therefore, the qualitative, semi-structured interviews were suitable for collecting the interviewees’ understanding of the potential values and use of 5G-technology in the manufacturing industry.
3.2. Data analysis
3.2.1. Analysis of quantitative data
Analysis of the quantitative data in the evaluation matrixes was done by modifying the Technique for Order Preference by Similarity to an Ideal Solution (TOPSIS) method introduced by Hwang and Yoon (Citation1981), to ensure the robustness of the results. TOPSIS is an effective method for solving various multi-attribute decision-making (MADM) problems, with the underlying principle of selecting an alternative closest to the positive ideal solution and farthest from the negative ideal solution (Priya and Malhotra Citation2020; Shih, Shyur, and Lee Citation2007).
The classical TOPSIS was used as a basis in this study to utilize the advantages of a TOPSIS method; a systematic evaluation based on a scientific and robust method. However, the method was modified in this study to not use criteria weight, but equally evaluate the different 5G-characteristics and their impact on PI, based on four experts’ estimations. The benefit of such a method is that it includes a robust aggregation procedure to convert four expert’s estimation to one objective estimation and enables to determine the interrelationship between 5G-characteristics and PI. An overview of the modified TOPSIS method is given in .
In this method, an impact index was calculated for each 5G-characteristic across all PIs using the five main steps in in an iterative way. The notations used in these steps to construct the method are explained in . The structure of the evaluation matrix is given in Equation (1)
Table 3. Notations used to construct the modified TOPSIS method
where denotes the 5G characteristics;
represents PIs. The PIs were categorized into five main categories: productivity, quality, maintenance performance, flexibility, and sustainability. These PI categories consist of PIs for certain values of j and they were defined as sets (i.e.
,
,
,
, and
) given in . Moreover,
indicates the impact evaluation of the 5G-characteristics
with respect to PIs
by interviewee
(K = 4) for demo
(D = 8).
Step 1 in : The evaluation matrixes obtained from the interviewees were aggregated by applying the geometric mean method (GMM). GMM reduces the bias effect of extreme values (very low or very high) (Aull-Hyde, Erdogan, and Duke Citation2006) and was therefore selected to obtain the aggregated matrix for each demo.
The aggregated matrix is denoted in EquationEquation (2)
(2)
(2) and this matrix
is computed by EquationEquation (3)
(3)
(3) where
is the element of
. In other words, four matrixes (one from each of the experts) were aggregated into one matrix for each of the demos.
Step 2 in : The ideal lowest impact value (LIV) and the ideal highest impact value (HIV) were determined based on the used scale ranging from low to high for the impact evaluation. In this context, LIV and HIV for each demo are given in
Step 3 in : Distance to the ideal lowest impact value (DLIV) and distance to the ideal highest impact value (DHIV) were determined based on each PI category per demo (to be used for individual impact index). This was done by using the Euclidean distance metric (minimization of the square root of the sum of squared distances); commonly used in the classical TOPSIS method to calculate distances (Olsson Citation2004; Taşabat Citation2019). In this context, the distance between the aggregated value of the 5G-characteristics to the
for each PI category per demo is denoted
and calculated by the formula given in EquationEquation (6)
(6)
(6) . Consequently, five
were obtained for each 5G-characteristics corresponding to each PI category per demo.
Similarly, the distance between the aggregated value of the 5G-characteristics to the
for each PI category per demo is denoted
and calculated by the formula given in EquationEquation (7)
(7)
(7) and five
were obtained for each 5G characteristics corresponding to each PI category per demo.
Step 4 in : This step consists of the calculation of the individual impact index that was calculated for each 5G-characteristics based on each PI category per demo by using the formula given in EquationEquation (8)(8)
(8) . The formula is adapted from the study proposed by Deng, Yeh, and Willis (Citation2000).
where and
are the computed values in Step 3. It should be noted that five individual impact indexes
are obtained for each 5G-characteristic corresponding to each PI category per demo. The
value can be in the range
. The larger the index value, the more impact of the 5G-characteristic on each PI category. In other words, if
then
(corresponding 5G-characteristic) has the highest impact on the PI category; if
then
(corresponding 5G-characteristic) has the lowest impact on the PI category.
Step 5 in : 5G-characteristics were ranked through the descending order of and
values computed in Step 4.1 and 4.2, respectively. Afterward, the ranking results demonstrate the most important 5G-characteristics for each PI category per demo.
The same method for aggregated analysis was also carried out for all demos. In this analysis, the same Steps 1–5 explained above were applied to calculate global and overall impact indexes, respectively, with certain adjustments. For instance, in Step 3, the calculations were performed across all demos instead of per demo. Therefore, EquationEquations (6(6)
(6) –Equation7
(7)
(7) ) (see Step 3) were slightly adjusted to calculate DLIV and DHIV, and new EquationEquations (9
(9)
(9) –Equation10
(10)
(10) ) were formulated as below:
where and
equals 1 and 5, respectively. Consequently, five
and
were obtained for each 5 G-characteristics corresponding to each PI category across all demos.
Similarly, to calculate DLIV and DHIV across all PI categories, new EquationEquations (11(11)
(11) –Equation12
(12)
(12) ) were formulated as below:
Consequently, two overall distance values such as and
were obtained for each 5G-characteristics across all PI categories and all demos.
As summarized for Step 3, the calculations were also performed across all demos instead of per demo in Step 4. Therefore, EquationEquation (13)(13)
(13) was formulated to calculate the global impact index and as below:
where and
are the computed values in EquationEquations (10
(10)
(10) –Equation11
(11)
(11) ). It should be noted that five global impact indexes
were obtained for each 5G-characteristic corresponding to each PI category. On the other hand, a new EquationEquation (14)
(14)
(14) was formulated to calculate the overall impact index for each 5 G-characteristics across all PI categories and all demos.
where and
are the computed values in EquationEquations (11
(11)
(11) –Equation12
(12)
(12) ).
Finally, another overall impact index across all demos and all 5G-characteristics was also calculated to determine the most affected PI categories by using the following equation
where and
are the computed values for five PI categories in EquationEquations (9
(9)
(9) –Equation10
(10)
(10) ).
In Step 5, 5G-characteristics were ranked through the descending order of and
values computed in EquationEquations (13
(13)
(13) –Equation14
(14)
(14) ), respectively. Afterward, the ranking results demonstrate the top three 5G-characteristics for each PI category and across all PI categories across all demos. Additionally, PI categories were also ranked through the descending order of
values computed in EquationEquation (15)
(15)
(15) to determine the most affected PI categories.
3.2.2. Analysis of qualitative data
Analysis of the qualitative data from the interviews was done to compare to the results of the quantitative analysis for verification purposes. The interviews were audio-recorded, and a selection of quotes was transcribed. The quotes were transcribed if they were related to the impact and effects of 5G in manufacturing, challenges in using the technology, challenges in the deployment of the technology, or expectations on 5G-technology.
4. Results and analysis
This chapter will firstly present the evaluation matrix from the initial literature review and interviews, used for the evaluation during the interviews. Secondly, the analysis of each demo will be presented, including a description of the demo and the top three 5G-characteristics. For each of those characteristics, the impact on each PI category is presented in terms of the individual impact index. The impact index should be interpreted as follows: ‘1’ indicates high impact, and ‘0’ indicates no/low impact. The step-wise calculation for individual impact index for Demo 1 is given in Appendix B. Thirdly, the aggregated analysis of all demos presenting the top three most important 5G-characteristics for each of the PI-category (global impact index) and the top three most important 5G-characteristics for all PI categories aggregated, as well as the impact of 5G-technology on each of the PI category (overall impact index). Fourth and finally, results from the analysis of the qualitative data from the open questions in the interviews are presented to complement findings from the quantitative analysis.
4.1. The evaluation matrix
The evaluation matrix consists of 15 performance indicators (PIs) (columns) and 11 5G-characteristics (rows). The 15 PIs are grouped into five PI categories: productivity, quality, maintenance performance, flexibility, and sustainability. Still, 15 columns and 11 rows give 165 values to enter in the matrix (see Appendix A).
4.2. Description and evaluation of demo 1
To meet the demand of the future factory in terms of connectivity, to enable communication between equipment and personnel, the reliance on a radio network that can ensure good coverage and overall network performance is crucial. The planning and design of the radio network are therefore of high importance. An offline method for radio network planning (Demo 1) was developed to ensure high network performance without testing it in the real environment (which causes production disturbance). The offline method relies on 3D laser scanning to get a point cloud and further a 3D-CAD geometry of the environment. Ray-tracing is then applied in the 3D-CAD geometry used to calculate wave propagation in the geometric model, to get an idea of how the radio network performs in the environment. The results were validated by comparing the simulated radio network to manual measurements of the installed network.
Based on quantitative data from the evaluation matrixes used in TOPSIS analysis, the top three 5G-characteristics for Demo 1 are: additional data sources, secured spectrum, and wide network coverage. The top three 5G-characteristics and their individual impact index for each of the PI categories are shown in .
Figure 4. The figure describes the individual impact index of additional data sources, secured spectrum and, wide network coverage on productivity, quality, maintenance performance, flexibility, and sustainability respectively in Demo 1.
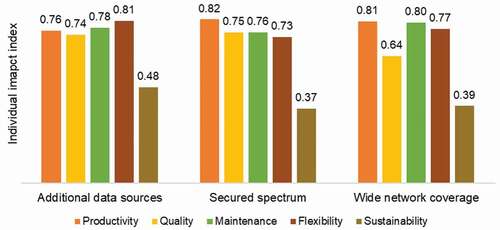
The height of the bar represents the individual impact index. For example, additional data sources will impact flexibility in first hand in Demo 1, with the individual impact index calculated to 0.81. Additional data sources will also impact maintenance performance, productivity, and quality (individual impact indexes 0.78, 0.76, and 0.75 respectively). In contrast, sustainability will be less affected (impact index 0.48).
4.3. Description and Evaluation of Demo 2
Demo 2 is a design of network and cloud architecture for the 5G network at the case company. It consists of hardware and software enabling mobile connectivity, data storage, distributed computing, and capabilities for data analytics. The demo aimed to demonstrate the installation and deployment of 5G-technology, as well as cloud services.
Based on quantitative data from the evaluation matrixes used in TOPSIS analysis, the top three 5G-characteristics in Demo 2 are: additional data sources, data traffic policies with prioritized devices, and centrally controlled device connectivity. The top three 5G-characteristics and their individual impact index for each of the PI categories are shown in .
Figure 5. The figure describes the individual impact index of additional data sources, data traffic policies – prioritized devices, and centrally controlled device connectivity on productivity, quality, maintenance performance, flexibility, and sustainability respectively in Demo 2.
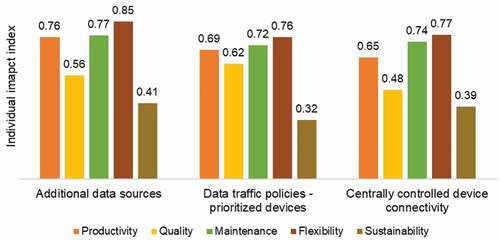
The height of the bar represents the individual impact index. For example, additional data sources will impact flexibility in first hand (individual impact index 0.85), but also productivity and maintenance performance (individual impact index 0.77 and 0.76). Quality and sustainability will be less affected (individual impact index 0.56 and 0.41).
4.4. Description and Evaluation of Demo 3
Demo 3 is a connected grinding machine, aiming to demonstrate how stationary equipment can be connected to a centralized cloud through 5G-technology. Connected equipment aims to reduce disturbances (i.e. unplanned stops) and quality issues. Data was collected from the grinding machine from the internal control system and externally mounted sensors. One objective in the project was to develop machine-specific algorithms for machine health assessment, decision support, and root cause analysis to reduce production disturbances such as stop time and quality issues.
Based on quantitative data from the evaluation matrixes used in TOPSIS analysis, the top three 5G-characteristics in demo 3 are: low latency, deterministic latency, and additional data sources. The top three 5G-characteristics and their individual impact index for each of the PI categories are shown in .
Figure 6. The figure describes the individual impact index of low latency, deterministic latency, and additional data sources on productivity, quality, maintenance performance, flexibility, and sustainability respectively in Demo 3.
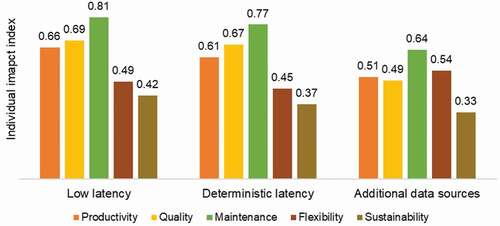
The height of the bar represents the individual impact index. For example, low latency will have a major impact on maintenance performance (individual impact index 0.81). In contrast, flexibility and sustainability (impact index 0.49 and 042) will be less affected by low latency in Demo 3.
4.5. Description and Evaluation of Demo 4
Demo 4 aims to demonstrate the connectivity of mobile equipment and people. A tool called MOST (Mobile Operator Support Tool), used at one of the case companies, was connected to the pilot-5 G-network and the cloud. MOST aims to support personnel on the factory floor by providing information, decision support, documentation, follow-up of machine parameters, etc. in a mobile, interactive application.
Based on quantitative data from the evaluation matrixes used in TOPSIS analysis, the top three 5G-characteristics in Demo 4 are: wide network coverage, additional data sources, and scalability. The top three 5G-characteristics and their individual impact index for each of the PI categories are shown in .
Figure 7. The figure describes the individual impact index of wide network coverage, additional data sources, and scalability on productivity, quality, maintenance performance, flexibility, and sustainability respectively in Demo 4.
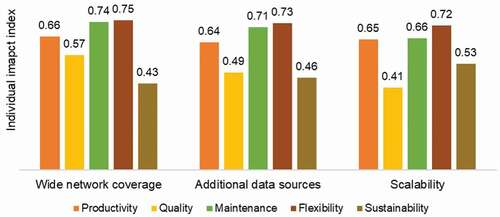
The height of the bar represents the individual impact index. In Demo 4, wide network coverage will have a relatively large impact on flexibility and maintenance performance (individual impact index calculated to 0.75 and 0.74). In contrast, wide network coverage will have a rather low impact on sustainability (individual impact index 0.43).
4.6. Description and Evaluation of Demo 5
Demo 5 aims at demonstrating a large-scale deployment of 5G-technology, including all production cells at the case company: around 20 production cells and 100 devices. Possibilities to connect the factory local area networks (LAN) to other LANs, such as facility LAN, office LAN, etc. are explored in this demo, including access rules and policies.
Based on quantitative data from the evaluation matrixes used in TOPSIS analysis, the top three 5G-characteristics in Demo 5 are: low latency, high bandwidth, and high data rate. The top three 5G-characteristics and their individual impact index for each of the PI categories are shown in .
Figure 8. The figure describes the individual impact index of low latency, high bandwidth, and high data rate on productivity, quality, maintenance performance, flexibility, and sustainability respectively in Demo 5.
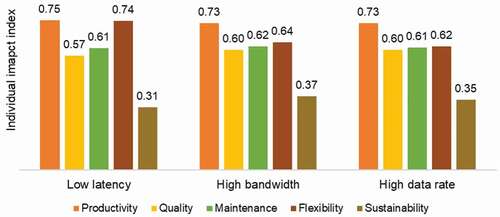
The height of the bar represents the individual impact index. For example, low latency will have the highest impact on productivity and flexibility (impact index 0.75 and 0.74), and the lowest impact on sustainability (impact index 0.31).
4.7. Description and Evaluation of Demo 6
Demo 6 is an analytics demonstrator, aiming to provide remote analyses based on data collected from both wired and wirelessly connected sensors via the pilot-5 G-network. The demo demonstrates the deployment of machine intelligence to increase awareness of machine conditions and improve maintenance planning, aiming to enhance manufacturing performance as well as financial performance.
Based on quantitative data from the evaluation matrixes used in TOPSIS analysis, the top three 5G-characteristics in Demo 6 are: additional data sources, low latency, and deterministic latency. The top three 5G-characteristics and their individual impact index for each of the PI categories are shown in .
Figure 9. The figure describes the individual impact index of additional data sources, low latency, and deterministic latency on productivity, quality, maintenance performance, flexibility, and sustainability respectively in Demo 6.
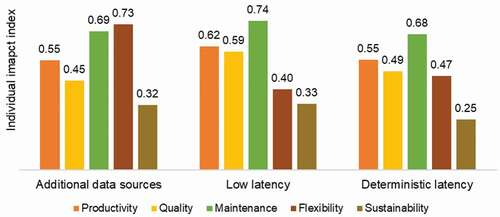
The height of the bar represents the individual impact index. For example, flexibility is the PI category that will be most affected by additional data sources in Demo 6. The impact index was calculated to 0.73. Sustainability will be less affected by additional data sources (impact index 0.32).
4.8. Description and Evaluation of Demo 7
A manufacturing control tower is demonstrated in Demo 7: a virtual control room enabled by 5G-technology. The manufacturing control tower has three essential capabilities: visibility (real-time visibility of data, dashboards, to visualize the status of the factory, etc.), analytics (for example artificial intelligence (AI) for rapid decision), and execution (disseminating information and action plans, monitoring execution compliance). Sensors and cameras will collect real-time data to provide visualizations (2D as well as 3D) of the complete factory/cell, positions of components, materials, and humans.
Based on quantitative data from the evaluation matrixes used in TOPSIS analysis, the top three 5G-characteristics in Demo 7 are: low latency, additional data sources, and high bandwidth. The top three 5G-characteristics and their individual impact index for each of the PI categories are shown in .
Figure 10. The figure describes the individual impact index of low latency, additional data sources, and high bandwidth on productivity, quality, maintenance performance, flexibility, and sustainability respectively in Demo 7.
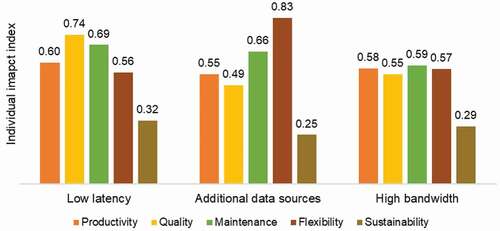
The height of the bar represents the individual impact index. As an example of Demo 7, low latency will have a relatively high impact on quality (0.74). The impact index on sustainability was calculated to 0.31, indicating a rather low impact.
4.9. Description and Evaluation of Demo 8
Demo 8 focuses on interaction with operators using 5G-technology for designing skill-based and skill-developing workplaces. 5G-technology is used to enable data collection, cloud computing, analysis of data, and presentation of data and information, based on the individual skills of each of the operators. The main objective of Demo 8 is to facilitate data and information presentation in a mobile and wireless device in an efficient way.
Based on quantitative data from the evaluation matrixes used in TOPSIS analysis, the top three 5G-characteristics in Demo 8 are: wide network coverage, low latency, and scalability. The top three 5G-characteristics and their individual impact index for each of the PI categories are shown in .
Figure 11. The figure describes the individual impact index of wide network coverage, low latency, and scalability on productivity, quality, maintenance performance, flexibility, and sustainability respectively in Demo 8.
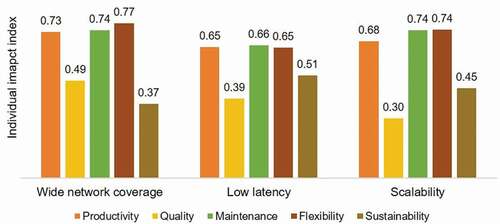
The height of the bar represents the individual impact index. For example in Demo 8, wide network coverage will have a high impact on flexibility, maintenance performance, and productivity (impact index 0.77, 0.74, and 0.73 respectively). Quality and sustainability will be less affected, with an impact index calculated to 0.49 and 0.37.
4.10. Aggregated analysis of all demos
As a final step in the quantitative data analysis, a global impact index was calculated based on aggregated values for all of the demos. A global impact index was calculated to identify the top three 5G-characteristics for each of the PI categories across all eight demos by using EquationEquation (13)(13)
(13) . For the impact on productivity, additional data sources, high data volume, and low latency will be most important. From a quality aspect, the top three 5G-characteristics are low latency, additional data sources, and deterministic latency. Maintenance performance will be most affected by low latency, additional data sources, and high data volume. The top three 5G-characteristics from a flexibility perspective are: additional data sources, scalability, and wide network coverage. At last, the top three 5G-characteristics from a sustainability point of view are low latency, scalability, and additional data source. The top three 5G-characteristics and the global impact index per PI category are summarized in .
Table 4. Top three 5G-characteristics and global impact index (all demos) per PI category
Further, an overall impact index was calculated to identify the top three 5G-characteristics based on all PI categories and all eight demos by using EquationEquation (14)(14)
(14) . This resulted in overall top three 5G-characteristics, namely additional data sources, low latency, and scalability. The overall impact index was calculated to 0.58, 0.57, and 0.53 respectively, i.e. relatively low compared to individual impact index (evaluation of each demo, for example, Demo 3, where impact index of low latency on maintenance performance was 0.81). This indicates that the application determines which characteristics are most important. A summary of the overall impact index for the top three 5 G-characteristics based on all demos is shown in .
Table 5. The top three 5 G-characteristics, based on all demos
Lastly, an overall impact index for each of the PI categories was calculated based on all 5 G-characteristics and all demos by using EquationEquation (15)(15)
(15) . 5G will have the most impact on maintenance performance, productivity, and flexibility. The impact index of 5G on quality and sustainability shows that these PI categories will be less affected. shows a summary of the overall impact index of 5G on the PI categories, based on all demos.
Table 6. Overall impact index of 5 G on each PI category, based on all demos
4.11. Analysis of qualitative data from open questions
The interviewees experienced it a bit challenging to estimate the impact of 5G-technology using the evaluation matrix. The demonstrator, the specific PI, and the specific 5G-characteristic needed to be kept in mind while evaluating the impact. Still, Interviewee 3 said: ‘You need to connect it to the demonstrator. Otherwise, it will be too generalized’. In other words, what you should use 5G-technology for, and what kind of effects to expect, depends on the application. Interviewee 3 continues: ‘You need to look at different cases [i.e. different applications/demos]. They will impact differently’. This was also highlighted by Interviewee 1: ‘What’s the connection between “returned goods” and “factory radio design”? [i.e. a quality PI related to Demo 1]’. The same evaluation matrix was used for all demos (i.e. same 5G-characteristics and PIs), which made it clear that not all PIs are directly related to each of the demos.
Whether you need 5G-technology or not for certain applications were mentioned during the interviews. 5G-technology offers high bandwidth and low latency, which also can be delivered through Ethernet. However, it is extremely costly to change installations including cables. As Interviewee 3 said: ‘It is extremely costly to change today. Install cables and network. And the flexibility to add data points. We can do it today, but it is time-consuming and costs a lot of money. That is the difference’. The flexibility aspect was also highlighted by interviewee 1: ‘We have high requirements on flexibility: both up and down in production volume, but also to move equipment. Of course, we will be more flexible without wiring’. Even though 5G-technology is not the only wireless communication technology, ‘we have a lot of problems with Wi-Fi today […]. It is not reliable, we cannot use that for critical applications’ (Interviewee 1). In other words, 5G-technology will increase flexibility, without compromising on reliability. However, some practical issues need to be solved before deployment of the technology in full scale ‘[…] but I am sure we will solve them’ (Interviewee 2).
The interest in maintenance has accelerated in the digitalization context and was mentioned by some of the interviewees. For example: ‘It is a lot of focus on maintenance […] that is great!’ (Interviewee 3). It was mentioned that maintenance departments generally get responsible for equipment that they have not had the opportunity to impact on the specification during purchase. The expectation of digitalization is to provide data and information about machine health conditions, to lower downtime of equipment, which in turn will have an impact on productivity.
The benefits of 5G-technology have mostly been discussed in technological aspects. Similarly, IT departments at manufacturing plants usually have a technological focus on PIs; for example, how long time it takes to solve the IT problem. Interviewee 3 told about one of their customers, where they have introduced new PIs for the IT department: ‘a strong PI now is related to production output’. In this factory, they have started to link the IT department to productivity, as the goal of the factory (and thus also the IT department) is to produce goods.
The awareness of benefits in using 5G-technology, and other new technology as well, is increasing. Interviewee 1: ‘The important aspect, for us, is not the demonstrator itself, but the process of increasing the awareness regarding 5G-technology’. Still, there are some challenges in the investment of new technology, mostly related to lacking knowledge; ‘they do not know what to buy’ (Interviewee 4). But when they get over it, most people understand the benefit of digitalization. Interviewee 3: ‘Some management levels have realized that there are more than technological benefits with digitalization and 5G-technology’. To summarize, the interviewees project 5G-technology to mainly impact the PI categories flexibility, productivity, and maintenance performance.
5. Discussion
The new applications characterizing digitalized manufacturing are introducing new requirements on communication technology. Experts in mobile communication technology are using technological characteristics and network performance to explain the benefits of 5G-technology in, for example, manufacturing (Peuster et al. Citation2019; Morgado et al. Citation2018). However, industry practitioners are rather interested in how 5G-technology can impact the performance of the manufacturing plant than technological characteristics. This study aims to determine the impact of 5G-technology on manufacturing performance by answering the research question: Which key characteristics of 5G-technology have the highest significant impact on manufacturing performance? Additional data sources, low latency, and scalability are projected to have a significant impact on productivity, flexibility, and maintenance performance. The results make it clearer how 5G-technology is projected to impact the performance of the manufacturing system, and thus why the manufacturing industry should invest in 5G-technology. Thereby, this paper makes a range of contributions.
Firstly, 5G-technology is linked to manufacturing performance. From a theoretical point of view, the focus shifts from network performance to manufacturing performance. The practical implication is that it becomes clearer for industry practitioners why they should invest in 5G-technology and how this technology can be applied. The results from this study show that productivity, flexibility, and maintenance performance will gain the most from 5G-technology. These aspects (especially flexibility) have also been acknowledged in the literature; to benefit from 5G-technology and enable digitalized manufacturing (Palattella et al. Citation2016; Rao and Prasad Citation2018; Li, Da Xu, and Zhao Citation2018; Digital; Catapult Citation2019).
The characteristics of 5G-technology have not been evaluated concerning manufacturing performance indicators before; leading to the second contribution. This study highlights the top three characteristics of 5G-technology: additional data sources, low latency, and scalability. 5G-technology will make it easier to add data points from where to collect data. For example, it becomes easier to upgrade an old machine by adding sensors to collect and analyze data from equipment that is not equipped with the latest technology. Similar results have previously been reported. Reliability, low latency, scalability, high data rates, high device density, security, and mobility/flexibility are all important characteristics for several IoT applications (Palattella et al. Citation2016; Rao and Prasad Citation2018; Li, Da Xu, and Zhao Citation2018). However, this study is linking such characteristics to manufacturing performance.
Thirdly, this study makes it clear that the benefit of 5G-technology differs between applications; the top three 5G-characteristics varied between the eight demonstrators. For example, low latency tends to be more important in applications focusing on connectivity to production cells, machines, and other devices to enable monitoring, controlling, and analysis of data in real-time. This was seen in demo 3, 5, 6, 7, and 8. Applications including data and information for human operators are dependent on wide network coverage, seen in demo 4 and 8. The human operators are supposed to move around in the production system and connectivity is needed everywhere to ensure that they can access the data and information needed to operate the machine. It is thus necessary to consider the application area when evaluating how to use 5G and for what purpose. This is confirmed from both the quantitative and the qualitative analysis. From the quantitative analysis, the value of the individual impact indexes was higher compared to the global impact indexes. For example, the individual impact index of low latency on maintenance performance was calculated to 0.81 in demo 3. The global impact index of low latency on maintenance performance was calculated to 0.69. This means that low latency is considered more important for maintenance performance in demo 3, compared to the importance of low latency for maintenance performance across all demos. This was simplified by one of the interviewees: ‘You need to look at different cases [i.e. different demos]. They will impact differently’.
Fourthly and lastly, this study contributes with a method that can be used for evaluating the potential values that can be gained from a digital technology applied in a manufacturing process: the modified TOPSIS method. There is an urge and need for new ways of evaluating digital technologies to identify the values they can bring to the right application. Regarding the ability of managers to decide which new technology to invest in, one of our interviewees said: ‘they do not know what to buy’. This comment suggests that managers are still lacking the knowledge and tools they need to evaluate new technology. The domain expert in a manufacturing area is crucial for identifying where the biggest opportunities and challenges are for implementing digital technologies (McAfee and Brynjolfsson Citation2012). The modified TOPSIS makes it possible to analyze the relationship between the characteristics of 5G-technology and performance indicators, with implications that the benefits of 5G-technology can be disseminated to a wider range of industrial companies.
The authors would like to acknowledge some limitations of the study that can give insights to future research areas. Firstly, for the modified TOPSIS method (Hwang and Yoon Citation1981), GMM was used for aggregating the impact of the evaluation matrixes composed of the answers from the interviews. Afterward, the Euclidean distance metric was used to calculate the distances to the ideal lowest and highest impact value, from where individual, global and overall impact indexes were obtained. The study did not include any other aggregation methods for comparison. For future research, the authors suggest testing other aggregation methods (such as harmonic mean operator) and other distance metrics (for example Manhattan distance) (Shih, Shyur, and Lee Citation2007) for comparative analysis.
Secondly, 5G-technology is not yet fully implemented in the manufacturing industry, making it impossible to measure its actual impact on performance. Instead, the results from this study are based on estimations from four manufacturing experts possessing relevant IT knowledge to perform this evaluation. This study aimed to contribute to a more specific description of the impact on 5G-technology in different applications (i.e. the demos); rather than making a broad empirical study about 5G-technology in manufacturing. Three criteria were used to select interviewees: (1) domain knowledge about manufacturing, (2) knowledge about 5G-technology, and (3) familiarity with the eight demos in the 5GEM-project. Four interviewees were selected. A modified TOPSIS method was used to ensure the robustness of the results, without requiring any specific number of respondents. However, for future research, the authors suggest having a broader range of respondents, aiming to generalize the use of 5G-technology in the manufacturing industry. The authors also propose research studies where 5G-technology’s impact on industrial performance indicators are measured and followed up in industrial applications.
6. Conclusions
This study aimed at determining the impact of 5G-technology on manufacturing performance, to facilitate investment and deployment of 5G-technology in the manufacturing industry. The results show that 5G-technology in manufacturing will impact the most on productivity, maintenance performance, and flexibility. A slightly lower impact is seen for the quality and sustainability aspects. Low latency, the ability to add new data sources, and scalability are the most important technical characteristics of 5G-technology. Nevertheless, the importance of specific 5G-characteristics is dependent on the application where 5G-technology is used. Similarly, the impact of 5G-technology differs between applications. Still, it is possible to conclude that 5G-technology will mainly impact productivity, maintenance performance, and flexibility. The results of this study are based on a modified TOPSIS method; an advantage in terms of computational efficiency in projecting the impact of 5G in a structured mathematical form which ensures the robustness of the results.
5G-technology meets the network requirements for many applications in digitalized manufacturing. By describing the impact of 5G-technology in terms of industrial PIs, rather than the performance of the network, it becomes clear how 5G-technology will impact the performance of the manufacturing plant. Knowing the impact on manufacturing performance can facilitate investment and deployment of 5G-technology in the manufacturing industry. Thus, industrial companies can continue the development of digitalized manufacturing with the support of 5G-technology.
Acknowledgments
The authors would like to thank all individuals who contributed to the project 5GEMII, a research project financed by VINNOVA under Grant number 2018-02820. We especially thank the case companies for commitment and collaboration. This work has been performed within the Production Area of Advance at Chalmers. The support is greatly appreciated.
Disclosure statement
No potential conflict of interest was reported by the author(s).
Additional information
Funding
References
- Agyapong, P. K., M. Iwamura, D. Staehle, W. Kiess, and A. Benjebbour. 2014. “Design Considerations for a 5G Network Architecture.” IEEE Communications Magazine 52 (11): 65–75. doi:https://doi.org/10.1109/MCOM.2014.6957145.
- Åkerman, M., C. Lundgren, M. Bärring, V. Berggren, M. Folkesson, V. Berggren, U. Engström, and M. Friis. 2018a. “Challenges Building A Data Value Chain to Enable Data-Driven Decisions: A Predictive Maintenance Case in 5G-Enabled Manufacturing.” Procedia Manufacturing 17: 411–418. doi:https://doi.org/10.1016/j.promfg.2018.10.064.
- Åkerman, M., J. Stahre, U. Engström, O. Angelsmark, D. McGillivray, T. Holmberg, M. Bärring, C. Lundgren, M. Friis, and Å. Fast-Berglund. 2018b. “Technical Interoperability for Machine Connectivity on the Shop Floor.” Technologies 6 (3): 3. doi:https://doi.org/10.3390/technologies6030057.
- Aull-Hyde, R., S. Erdogan, and J. M. Duke. 2006. “An experiment on the consistency of aggregated comparison matrices in AHP„. European journal of operational research, 171(1): 290–295
- Bärring, M., B. Johansson, and J. Stahre. 2020. “Digital Technologies Enabling Data of Production Systems for Decision Support.” Smart and Sustainable Manufacturing Systems 4 (2): 62–79. doi:https://doi.org/10.1520/SSMS20190034.
- Bärring, M., O. Iupikov, A. A. Glazunov, M. Ivashina, J. Berglund, B. Johansson, J. Stahre, F. Harrysson, U. Engström, and M. Friis. 2021. “Factory Radio Design of a 5g Network in Offline Mode.” IEEE Access 9: 23095–23109. doi:https://doi.org/10.1109/ACCESS.2021.3055941.
- Catapult, D. 2019. “Made in 5G - 5G for the UK Manufacturing Sector.” Accessed 9 June 2020 from https://assets.ctfassets.net/nubxhjiwc091/2gtsKJ9qmYPUxMovbIQ3zb/dc7950443e985d1802486470dc73f00f/DC_113_5G_Manufacturing_Report_Digital.pdf
- Cheng, J., W. Chen, F. Tao, and C. L. Lin. 2018. “Industrial IoT in 5G Environment Towards Smart Manufacturing.” Journal of Industrial Information Integration 10: 10–19. doi:https://doi.org/10.1016/j.jii.2018.04.001.
- Creswell, J. W. 2014. Research Designs: Qualitative, Quantitative, and Mixed Methods Approaches. Callifornia: Sage.
- Da Silva, V. L., J. L. Kovaleski, R. N. Pagani, J. D. M. Silva, and A. Corsi. 2020. “Implementation of Industry 4.0 Concept in Companies: Empirical Evidences.” International Journal of Computer Integrated Manufacturing 33 (4): 325–342. doi:https://doi.org/10.1080/0951192X.2019.1699258.
- Dalenogare, L. S., G. B. Benitez, N. F. Ayala, and A. G. Frank. 2018. “The Expected Contribution of Industry 4.0 Technologies for Industrial Performance.” International Journal of Production Economics 204: 383–394. doi:https://doi.org/10.1016/j.ijpe.2018.08.019.
- Deng, H., C. H. Yeh, and R. J. Willis. 2000. “Inter-company Comparison Using Modified TOPSIS with Objective Weights.” Computers & Operations Research 27 (10): 963–973. doi:https://doi.org/10.1016/S0305-0548(99)00069-6.
- Fettweis, G. P. 2014. “The Tactile Internet: Applications and Challenges.” IEEE Vehicular Technology Magazine 9 (1): 64–70. doi:https://doi.org/10.1109/MVT.2013.2295069.
- Hermann, M., T. Pentek, and B. Otto. 2016. “Design Principles for Industrie 4.0 Scenarios.” Proceedings of 2016 49th Hawaii International Conference on Systems Science, January 5–8,Maui, Hawaii. doi:https://doi.org/10.1109/HICSS.2016.488.Koloa, Maui, Hawaii.
- Hwang, C. L., and K. Yoon. 1981. Multiple Attribute Decision Making: Methods and Applications. New York: Springer-Verlag.
- Idachaba, F. E. 2016. “5G Networks: Open Network Architecture and Densification Strategies for beyond 1000x Network Capacity Increase.” Proceedings of 2016 Future Technologies Conference (FTC): 1265–1269, San Francisco, United States. DOI: https://doi.org/10.1109/FTC.2016.7821762.
- Kang, H. S., J. Y. Lee, S. Choi, H. Kim, J. H. Park, J. Y. Son, B. H. Kim, and S. Do Noh. 2016. “Smart Manufacturing: Past Research, Present Findings, and Future Directions.” International Journal of Precision Engineering and Manufacturing-Green Technology 3 (1): 111–128. doi:https://doi.org/10.1007/s40684-016-0015-5.
- Li, S., L. Da Xu, and S. Zhao. 2018. “5G Internet of Things: A Survey.” Journal of Industrial Information Integration 10: 1–9. doi:https://doi.org/10.1016/j.jii.2018.01.005.
- Lu, Y. 2017. “Industry 4.0: A Survey on Technologies, Applications and Open Research Issues.” Journal of Industrial Information Integration 6: 1–10. doi:https://doi.org/10.1016/j.jii.2017.04.005.
- Lueth, K. L. 2020. “IoT 2019 in Review: The 10 Most Relevant IoT Developments of the Year.” Accessed June 30 from: https://iotanalytics.com/iot-2019-in-review/
- Lundgren, C., A. Skoogh, B. Johansson, J. Stahre, and M. Friis. 2017. “The Value of 5g Connectivity for Maintenance in Manufacturing Industry.” 2017 Winter Simulation Conference (WSC).Las Vegas, United States
- Lynch, R. L., and K. F. Cross. 1991. Measure Up!: The Essential Guide to Measuring Business Performance. London: Mandarin.
- McAfee, A., and E. Brynjolfsson. 2012. “Big Data: The Management Revolution.” Harvard Business Review 90 (10): 60–68.
- Miraz, M. H., M. Ali, P. S. Excell, and R. Picking. 2015. “A Review on Internet of Things (Iot), Internet of Everything (Ioe) and Internet of Nano Things (Iont).” Proceedings of Internet Technologies and Applications (ITA): 219–224. (ITA), Wrexham, U.K., September.Wrexham, United Kingdom.
- Morgado, A., K. M. S. Huq, S. Mumtaz, and J. Rodriguez. 2018. “A Survey of 5G Technologies: Regulatory, Standardization and Industrial Perspectives.” Digital Communications and Networks 4 (2): 87–97. doi:https://doi.org/10.1016/j.dcan.2017.09.010.
- Neely, A., M. Gregory, and K. Platts. 1995. “Performance Measurement System Design: A Literature Review and Research Agenda.” International Journal of Operations & Production Management 15 (4): 80–116. doi:https://doi.org/10.1108/01443579510083622.
- Olson, D. L. 2004. “Comparison of Weights in TOPSIS Models.” Mathematical and Computer Modelling 40 (7–8): 721–727. doi:https://doi.org/10.1016/j.mcm.2004.10.003.
- Osseiran, A., F. Boccardi, V. Braun, K. Kusume, P. Marsch, M. Maternia, O. Queseth, M. Schellmann, H. Schotten, and H. Taoka. 2014. “Scenarios for 5G Mobile and Wireless Communications: The Vision of the METIS Project.” IEEE Communications Magazine 52 (5): 26–35. doi:https://doi.org/10.1109/MCOM.2014.6815890.
- Palattella, M. R., M. Dohler, A. Grieco, G. Rizzo, J. Torsner, T. Engel, and L. Ladid. 2016. “Internet of Things in the 5G Era: Enablers, Architecture, and Business Models.” IEEE Journal on Selected Areas in Communications 34 (3): 510–527. doi:https://doi.org/10.1109/JSAC.2016.2525418.
- Palys, T. 2008. “Purposive Sampling.” In The Sage Encyclopedia of Qualitative Research Methods, edited by L. M. Given, 697–698. Vol. 2. Los Angeles: Sage.
- Parhi, S., K. Joshi, and M. Akarte. 2021. “Smart Manufacturing: A Framework for Managing Performance.” International Journal of Computer Integrated Manufacturing 34 (3): 227–256. doi:https://doi.org/10.1080/0951192X.2020.1858506.
- Patel, M., J. Shangkuan, and C. Thomas. 2017. “What’s New with the Internet of Things?” McKinsey&Company. Accessed June 25 from https://www.mckinsey.com/industries/semiconductors/our-insights/whats-new-with-the-internet-of-things
- Peuster, M., S. Schneider, D. Behnke, M. Müller, P. B. Bök, and H. Karl. 2019. “Prototyping and Demonstrating 5G Verticals: The Smart Manufacturing Case.” In 2019 IEEE Conference on Network Softwarization (NetSoft): 236–238. IEEE.Paris, France.
- Priya, B., and J. Malhotra. 2020. “5GAuNetS: An Autonomous 5G Network Selection Framework for Industry 4.0.” Soft Computing 24 (13): 9507–9523. doi:https://doi.org/10.1007/s00500-019-04460-y.
- Rao, S. K., and R. Prasad. 2018. “Impact of 5G Technologies on Industry 4.0.” Wireless Personal Communications 100 (1): 145–159. doi:https://doi.org/10.1007/s11277-018-5615-7.
- Shih, H. S., H. J. Shyur, and E. S. Lee. 2007. “An Extension of TOPSIS for Group Decision Making.” Mathematical and Computer Modelling 45 (7–8): 801–813. doi:https://doi.org/10.1016/j.mcm.2006.03.023.
- Taşabat Erpolat, S. 2019. “A Novel Multicriteria Decision-Making Method Based on Distance, Similarity, and Correlation: DSC TOPSIS.” Mathematical Problems in Engineering. doi:https://doi.org/10.1155/2019/9125754.
- Turner, C., C. Emmanouilidis, T. Tomiyama, A. Tiwari, and R. Roy. 2019. “Intelligent Decision Support for Maintenance: An Overview and Future Trends.” International Journal of Computer Integrated Manufacturing 32 (10): 936–959. doi:https://doi.org/10.1080/0951192X.2019.1667033.
- Xu, L. D., E. L. Xu, and L. Li. 2018. “Industry 4.0: State of the Art and Future Trends.” International Journal of Production Research 56 (8): 2941–2962. doi:https://doi.org/10.1080/00207543.2018.1444806.
Appendix A – Evaluation matrix
Appendix B – Step-wise calculation results of the modified TOPSIS method: An example for Demo 1
Step 1: The aggregated matrix for Demo 1 according to EquationEquations (2(2)
(2) –Equation3
(3)
(3) ).
Table
Step 2: The LIV and HIV according to EquationEquations (4(4)
(4) –Equation5
(5)
(5) ).
Step 3: The calculated DLIV and DHIV for each PI category (set) according to EquationEquations (6(6)
(6) –Equation7
(7)
(7) ).
Table
Step 4: The calculated individual impact index for each PI category (set) according to EquationEquation (8)(8)
(8) .
Table
Step 5: The ranking of 5 G-characteristics for each PI category (set).
Table