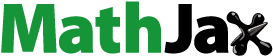
ABSTRACT
I investigate the underlying cognitive mechanisms and socio-emotional factors behind conspiracy theory (CT) beliefs through the lens of the Free-Energy Principle (FEP). The FEP framework is employed to explain the emergence of CTs in the face of cumulative uncertainties and the influence of emotions on belief formation. The FEP account I propose concludes that considering emotional factors, distrust of established authorities, and the social environment, believing in CTs is a bounded rational choice for some individuals in certain contexts. This explains why CT believers are resistant to changing their views. Applying FEP to the complex human behavior of CT belief and propagation, this paper not only provides insights into the phenomenon but also enhances the theoretical credence of FEP itself.
1. Introduction
Conspiracy theories (hereafter, CTs) are a relatively recent topic of discussion. We are a species that conspires. In the right context and with the right justification, there is also a survival advantage to suspecting that others are conspiring. That said, the forms of CTs to which we have become accustomed are often associated with irrationality, prejudice, and similar moral and epistemic vices. Notorious examples from the U.S.A include the 9/11 bombing of the Twin Towers, J. F. Kennedy’s assassination, and far-right anti-Semitic conspiracies. Against this background, the COVID-19 pandemic has contributed novel iterations, notably widespread anti-vaccination campaigns. CT beliefs concerning COVID-19 range from the belief that 5 G technology spreads the virus to the belief that transnational pharmaceutical consortia producing the vaccines are deliberately spreading the virus. New pandemic-related CTs seem to fuse seamlessly with older CTs. An example is the theory that the virus is part of a “deep state” plot to cull the global population to levels more amenable to control.
The rapid spread, global reach, amplification, and sheer variability of CTs has drawn the attention of contemporary mainstream philosophers. Philosophical questions regarding CTs include the following: Why is it so difficult to change the minds of CT believers? Does commitment to CTs reflect irrationality? And, if so, in what sense?
My goal in this paper is to answer these questions by way of the free-energy principle (FEP) (Friston et al., Citation2016; Friston, Citation2010, Citation2012). I shall also emphasize the emotional factors and the social environment involved in CT believers’ adherence to CTs. Specifically, individuals tend to believe a CT when they are distrustful of established authorities (the government, the scientific community, health authorities, etc.). This may be attributable to past experiences or emotional factors, or to a context in which the risks involved in false CT beliefs do not outweigh the social benefits involved. I conclude that for some individuals in certain social and/or emotional contexts, believing in CTs is a bounded rational choice. It is a rational choice given the relevant agent’s cognitive and environmental constraints (Simon, Citation1956, Citation2000).
I apply FEP because it is a theory that defines human agents (or organisms or self-organizing systems in general) as entities that persist in time by resisting handling uncertainties they have about the world (Clark, Citation2016). FEP makes explicit reference to the natural or social environment that agents inhabit. This makes FEP a suitable platform for explaining how CTs arise against a backdrop of cumulative uncertainties. FEP also shows how our emotional lives influence (even determine) what we believe (Hohwy, Citation2013; Pezzulo, Citation2014). It does so through its theoretical connection to predictive processing. Moreover, reference to the survival of an organism in an external environment stands in contrast to the more domain-specific Bayesian theories of cognition that FEP is often associated with. As such, an FEP account cannot simply be replaced with an amalgam of domain-specific Bayesian accounts.
There is also a different kind of benefit. Providing an FEP account of CT can benefit FEP itself. It can increase the theoretical credence of FEP. Many applications of FEP remain subpersonal explanations of simple behavior, such as oculomotor control (Friston et al., Citation2010). But, if FEP purports to be a unifying theory of how the brain works, then it must inform explanations of complex human behavior. Such behavior includes the belief in and propagation of CTs.
In section 2, I elucidate CTs and CT believers as the key (and interwoven) explananda in my account.
In section 3, I outline which specific properties of CTs and CT believers make CT beliefs problematic.
In section 4, I present an FEP account of CT. I argue under certain socio-psychological conditions, believing in a CT can be a bound-rational choice.
In section 5, I discuss implications of my account. I also put forward some possible interventions that may counteract the adoption and proliferation of CTs.
2. Explananda: CTs and their believers
In this section, I first define CTs and then discuss the nature of CTs. This is to secure the extension of the concepts in question. An analysis along these lines is required because not all CTs are problematic. Take the hypothetical example of a CT about the assassination of Julius Caesar. Let us say that the CT is endorsed by a qualified scholar specializing in Ancient Roman history. Such a CT would probably not be considered problematic, even if it is unsubstantiated by further evidence. This is because there must have been some conspiracy to assassinate Julius Caesar, and the scholar has the skillset to conduct the relevant inquiry.
Most characterizations of CTs include negative epistemological evaluation. Vermeule and Sunstein (Citation2009, p. 204), for instance, state that CTs are (1) false, (2) harmful, and (3) unjustified. I find (1) and (2) particularly problematic.
Regarding the falsehood criterion, CTs could turn out to be true. The problem with CTs stems from the way in which the relevant belief is formed, and not from the falsity of the belief per se. It is possible for a CT believer to contingently adopt a true belief by sheer randomness of chance.
Sunstein and Vermeule’s characterizations of harmfulness is too vague to serve as a discriminating criterion in a definition of CTs. Does “harmful” mean harmful to society? Does it mean harmful only to CT believers or only to non-CT believers? It is not clear.
Regarding justification, most theorists find the problem with CTs in their epistemological aspect. It therefore seems plausible that CTs are unjustified.
Neil Levy (Citation2007) provides a plausible definition, one that focuses on the social aspects of CTs (aspects that I also emphasize). For Levy, CTs are explanations of events that (1) refer to plots (viz. conspiracies) and (2) are not supported by the “right kind” of epistemic authority. However, it may be impossible to specify the right kind of epistemic authority without begging the question. I therefore propose a neutral definition:
(CTs neutral definition) CTs are explanations that (1) refer to plots and (2) are not supported by established epistemic authorities.
Levy’s definition and my neutral definition are likely to be coextensional in reality. The “right kind” of epistemic authority will most probably comprise experts recognized by scientific communities, government committees, and the like (in other words, established authorities). Nevertheless, my proposed modification carries less normative import, which will become important shortly.
Following Coady (Citation2003), I would also like to introduce the notion of official stories. Official stories stand in contrast to CTs.
(Official Story) An official story is an explanation of events supported by established epistemic authorities.
3. Important characteristics of CTs and CT believers
Which properties of CTs and CT believers make CT beliefs problematic? My goal in this section is to answer this question.
CTs are sometimes said to be self-sealing (Cassam, Citation2019; Vermeule & Sunstein, Citation2009). A self-sealing theory is a theory that is (or becomes) insulated from evidence and immune to refutation. CT beliefs are incorrigible in the sense that CT believers are not persuaded by rigorous empirical evidence or rational persuasion. These are undoubtedly key properties of CTs (dialectic persuasion is particularly tricky). However, the label that CTS are self-sealing or incorrigible is descriptive rather than explanatory. For an explanation that fully captures it, we need to take a closer look at what exactly self-sealing or incorrigibility is.
I contend that the following is applicable here: (1) the epistemic and agential nature of the things CTs are about and (2) the psychological features of CT believers. To begin with, what seems outstanding about CTs is that their contents are by-and-large insulated from the ordinary world we live in. Following Lisa Bortolotti’s (Citation2009) taxonomy of rationality, CTs exhibit characteristics of procedural insulation. “Procedural insulation” means that a CT’s impact on someone’s general belief system is relatively limited. For example, it does not affect beliefs to do with ordinary actions (including everyday social interactions with other members of society). Apart from activities directly related to CT beliefs, there are limited consequences in non-conspiratorial contexts.
To illustrate, think of a CT to the effect that COVID-19 is part of a plot orchestrated by the deep state to cull the human population. Someone who subscribes to this CT is not necessarily going to believe that a family member is an impostor or that the local pharmacist is an agent for the deep state. Mostly, the scope of the belief in the CT will be limited to belligerent pub debates, social media furors, and the like. This relative inconsequentiality regarding actions related to CTs diverges from clinical delusions, which are not self-contained in the same way. In sum, there is often little risk involved in holding unsupported CT beliefs.
In fact, believing in a CT can be socially beneficial. CTs can facilitate fellowship between individuals and groups subscribing to similar beliefs. Thanks to the internet, fellowship is not geographically circumscribed. CT believers can find “allies” on social media. Online activist communities include anti-vaccination groups such as Stand Up X, Stop New Normal, and Save Our Rights UK.
The above suggests that CT beliefs may be an extreme form of socially adaptive beliefs. Following Daniel Williams (Citation2021), we can think of socially adaptive beliefs as beliefs whose formation is sensitive to social reward and punishment. Socially adaptive beliefs are a variant of motivated cognition, and they tend to occur when their possession leads to practical success. The odds of practical success, in turn, depend on the ratio between (1) social benefits accrued from the relevant beliefs and (2) risks incurred from believing false or unsupported beliefs. Although Williams does not discuss CTs, they seem to fit the bill. There is low-risk associated with believing false claims and there are putative social benefits (even if CT believers do not admit that their beliefs are motivated by social benefits). These characteristics of CTs are all conducive to self-sealing and incorrigibility.
There is psychological research suggesting that CT believers are as biased toward confirmatory evidence (and against disconfirmatory evidence) as patients suffering from clinical delusions (Georgiou et al., Citation2021). CT believers typically respond by invoking “counter-evidence” from their own “experts” or by attempting to discredit those producing the evidence. In the case of COVID-19 vaccines, for example, some CT believers claim that one of the architects of mRNA vaccines is skeptical of the COVID-19 vaccine. Some CT believers claim that the NIH director is part of the deep state, and so on. At the same time, mainstream research findings are subjected to intense skepticism and interpreted with heavy bias. For example, some CT believers claim that a Danish cohort study (Hansen et al., Citation2021) uploaded to medRxiv shows that vaccines damage the immune system. This is because the study indicates that the number of participants who received two vaccine shots and tested positive is larger than the number of unvaccinated participants who tested positive three months after vaccination.Footnote1 I shall return to this psychological aspect of confirmation bias in the next section.
According to Quassim Cassam (Citation2019), distinguishing properties of CTs are that they are esoteric and premodern.Footnote2 A CT is esoteric if it puts forward large-scale secrets and mysteries. Although this can be true, there are issues with Cassam’s description. There is surely a difference in scale between a sheer piece of misinformation (e.g., “mRNA vaccines are more harmful than beneficial”) and a full-scale CT (e.g., “pharmaceutical companies are colluding with the deep state to reduce the world population”).Footnote3 It is not always true that CT beliefs connect to a full-blown large-scale background theory. It is possible for CT beliefs to be both piece-meal and local.
According to Cassam, a CT is premodern because a CT believer is likely to assign everything with causal significance to malicious intent. This disallows the possibility that bad outcomes can arise from other sources. In principle, a CT believer does not acknowledge the variety of causal systems underlying events in the world (varying from intentional systems like us to intention-free brute physical systems). On the CT worldview, “things always happen for a reason”, a malicious human-determined reason. If there are no evident “villains” to pin the blame on, then they must be hiding, and so on.
Also important are the psycho-social features of a typical CT believer. It has been reported that CT believers are typically in socially adverse situations, they distrust society, and have negative emotions (related to anxiety or depression, for example) (Freeman & Bentall, Citation2017; Miller et al., Citation2016). Daniel Freeman and Richard Bentall state that CT believers tend to have
lower levels of physical and psychological well-being, higher levels of suicidal ideation, weaker social networks, less secure attachment style, difficult childhood family experiences, and were more likely to meet criteria for a psychiatric disorder
The psycho-social aspect is apparent in the misinformation upon which COVID-19-related CTs are built. Eastern Europe, where citizens often have high degrees of distrust in government, tend to have very low vaccination rates (Ghodsee & Orenstein, Citation2021). A recent survey showed that people are more susceptible to CTs in totalitarian countries (like Russia) or in polarized countries (like the US) (De Coninck et al., Citation2021). Interestingly, there is an ethnicity gap in US vaccination rates. Although this gap is decreasing, African Americans have the lowest vaccination rate among ethnic groups in the US (57% as of March, 2022) (Ndugga et al., Citation2022). Mistrust of government is likely a contributing factor given its history of mistreating African Americans (the notorious Tuskegee experiments come to mind).
Let us take stock of the relevant explananda before I flesh out my FEP account of CTs.
(CTs neutral definition) CTs are explanations that (1) refer to plots and (2) are not supported by established epistemic authorities.
Properties of CTs and CT believers:
Procedural insulation.
Esoteric and premodern characteristics.
Confirmation bias.
Negative emotions.
Socially adverse situations and distrust of authorities.
Employing the free-energy principle, I now draw on these properties to explain why and how a CT believer takes up a CT.
4. An FEP Account
In this section, I propose an FEP account of CTs. FEP is notoriously difficult to understand. It is a formal theory, and a full description is therefore not possible here (See Clark, Citation2016; Hohwy, Citation2013 for comprehensive philosophical introductions). I shall, however, introduce the core of the framework and its corollaries to the extent that is needed for our purposes. I shall also discuss FEP’s connection to associated frameworks or theories. These include Bayesian inference, predictive coding, and hierarchical prediction error minimization.
4.1. An Introduction to FEP
FEP is a normative principle that governs the behavior of every self-organizing system. It prescribes what a system should do to continue its existence in the face of (sometimes pernicious) environmental change. This does not mean that organisms (viz. self-organizing systems) do or should perfectly follow the principle. In reality, different organisms minimize free-energy to different degrees (Hohwy, Citation2021). Following Karl Friston (Citation2010, Citation2012) (and sacrificing some rigor) FEP can be simply stated as follows:
(FEP) Any self-organizing system that is at non-equilibrium steady-state with its fluctuating environment must minimize its free-energy.
Note that a “self-organizing system” is a system that (1) develops internal structures without the influence of external forces and (2) has the tendency to resist those forces. A “non-equilibrium steady-state” is a state in which a system persists in, but interacts with, its environment. Every life-form qualifies as a self-organizing system in a non-equilibrium steady-state.
4.1.1. Minimizing surprise
To see the need to minimize free-energy, we first need to understand what surprise is and what it means to minimize surprise. Surviving organisms are adapted to their environmental niches. This maximizes the chance that the organism can deal with changes in its environment. Thus, living organisms are motivated to remain in their adaptive environmental niches. Moving out of the niche can be an unexpected and dangerous event. It will be a surprise (e.g., a fish finding itself on dry land). According to FEP, maximizing survival involves keeping surprises to a minimum.
Surprise is formally defined as a negative log probability of sensory states given the model of the world an organism has: , where y represents sensory states and m is the model. The model is however not an objective mirror of the world. According to Thomas Parr and colleagues, the model specifies “the preferred conditions for the agent’s [the organism’s] existence” (2021, p. 46). This means that the model contains inherent optimism bias. It expects that sensations tending to co-occur with preferred states will actually occur. To minimize surprise, an organism actively seeks to acquire those preferred sensory states specified in the model, states that make the model probable. As Jakob Hohwy (Citation2016) puts it, the brain is “self-evidencing”.
4.1.2. Metaphysics of FEP
As mentioned, FEP assumes the existence of a self-organizing system that is separate from, but also interacts with, the world. There are three kinds of domains in this respect: (1) internal state, (2) external state, and (3) the boundary of the system. Under FEP, the boundary is the Markov blanket, which is defined as a state c that satisfies the following formula:
This means that the external states a and the internal states b become conditionally independent given the blanket state c. If you have information about c, information about a does not give any clues to inferring b and vice versa. Being the interface between the world and the system, the Markov blanket comprises sensory and active states. Based on sensory states, organisms infer what the external states are. They act on the world through active states to minimize surprise.
In one sense, Markov blankets can be found everywhere. We can regard the whole brain as a system enclosed by a Markov blanket. But, we can also find a blanket in many different structures if the structure satisfies the above definition. For example, we can regard a subset of a neural system as an independent system surrounded by a Markov blanket. This can even apply to a single cell, where the cell membrane is the sensory state and the actin filament in the cytoskeleton is the active state (Friston, Citation2013). In any event, unless explicitly stated, I shall assume that the whole brain is enclosed by a Markov blanket.
4.1.3. Minimizing variational free energy
As mentioned, an organism strives to minimize surprise. However, directly minimizing surprise is impossible for an actual corporeal organism. This is because calculating amounts to calculating the possibility of the occurrence of y under every possible external state x, and then summing all the values. There are simply too many external states, and marginalizing them makes the calculation intractable. Moreover, calculating the integral itself can be analytically difficult or even impossible.
This is where minimizing variational free-energy comes in. “Variational free-energy” is defined as follows:
Variational free-energy is originally a statistical thermodynamic quantity, but it works as a proxy of surprise under the FEP framework (see Kiefer & Hohwy, Citation2019 for more in-depth discussion regarding this point). This is because it consists of two functions that an organism can change via changing its internal neural state.
q(x) is the recognition density: the probability density that represents organism’s best guess about the state in the world.
p (x, y) is the generative model: the organism’s idea about how x produces y.
Applying , we get:
On the right-hand side, the first term is KL divergent between q(x) and p(x|y). This is a measure of the similarity between two probability distributions. KL divergence takes non-negative values (when it is zero, the two distributions are identical). In this case, it represents the similarity/difference between the recognition density and the true posterior. As the KL divergence gets closer to zero, free-energy becomes a tighter bound on surprise (when it becomes zero, free-energy is identical to surprise). Thus, organisms can indirectly lower KL divergence by gradually changing q(x) to lower free-energy. This amounts to more accurately recognizing the relevant external states. Alternatively, KL divergence can be minimized via action. Action can change sensory input y, and it will change p(y|x) accordingly.
But, ultimately an organism must minimize surprise. Just changing recognition density, and thereby minimizing KL divergence, is not sufficient. This is intuitive. No matter how accurate recognition becomes, it alone will not benefit the survival of the organism. Minimizing surprise via minimizing free-energy through action is crucial.
To sum up, free-energy is constituted by recognition density and a generative model that an organism can change. Free-energy minimization via changing recognition density amounts to accurate recognition. Free energy minimization via performing action can minimize surprise. This free-energy formulation nicely illustrates the surprise-minimization that emerges from the perception-action dynamic.
Free-energy minimization is achieved by calculating the relevant values of recognition density. This is done by changing the default values by some small margin. Value-modification stops when changing the values does not decrease the free-energy value. The relevant iterative first-order optimization algorithm is called gradient descent. The character of free-energy minimization is important in this context. This is because the free-energy principle only assures local free-energy minimization (given the organism and its environment). Following Friston et al. (Citation2013), we can then consider free-energy minimizing organisms to be bounded-rational agents.
Another important formulation of free-energy is the following:
The former term is another KL divergence between q(x) and p(x). It is called the complexity term. This measures how much a model must learn to incorporate a new guess q(x). It is called “the complexity term” because incorporating new data into a model usually increases the complexity of the model.
The latter term is the expected value of the log posterior possibility. It is called the accuracy term. If the posterior becomes on-average higher, the model becomes more “accurate”. Pursuing accuracy of the model decreases free-energy. Yet, it can also force the model to incorporate new data and it increases the complexity term. This shows how the delicate balance between complexity and accuracy is important when choosing what to believe.
4.1.4. Minimizing FEP as approximate Bayesian inference
Minimizing KL divergence through sequential changes of recognition density q(x) or sensation y through action is tantamount to performing an approximate Bayesian inference. Following Bayes theorem is one of the most reasonable ways to make a decision based on limited evidence. Bayes theorem states:
However, as explained above, p(y) is often computationally intractable. This makes finding posterior possibility p(x|y) analytically difficult. The alternative is to minimize KL divergence between q(x) and p(x|y) by making q(x) as close as possible to p(x|y).
4.1.5. Minimizing FEP and prediction coding
Recognition density is assumed to be a Gaussian under Laplace approximation (see Parr et al., Citation2022, p. 81 for mathematical detail). The form of a Gaussian distribution is univocal given its mode and variance. As such, approximate Bayesian inference can only be performed by finding the appropriate mode and variance. This is what predictive coding does (Friston & Kiebel, Citation2009; Rao & Ballard, Citation1999). The basic idea proceeds as follows: predict what a sensory input would be given a prior belief about an external cause, compare this with the actual sensory input, then revise the prior to minimize the difference (i.e., the prediction error).
Using the generative model p(x, y), the brain can make a prediction about what kind of sensations it will receive:
The generative model is basically a joint distribution between external state (x) and sensation (y). It can be decomposed into a prior belief about x and the likelihood of y given x. Thus, the brain can predict what sensation it is most likely to receive given a prior conception of the external cause.
The difference between the mode of prediction and the mode of actual sensation is the prediction error. The brain updates its prior belief in light of the prediction error. But, how much prediction error is incorporated into the posterior depends on how much the prediction error should be trusted. This is the precision of prediction error. “Precision” is defined as the inverse of the variance of a probability distribution. This is because the smaller the variance, the more reliable the signal becomes. Thus, highly precise prediction errors are more weighted during belief updating. This is prediction error minimization weighted by precision, which is another key component in my account of CT.
4.1.6. Predictive coding under hierarchical settings
Prediction error minimization is achieved in a hierarchical manner, at least in the case of human agents. This naturally reflects the separation of temporal causal scales in the world (Brown et al., Citation2013). This hierarchical structure also facilitates efficient learning, and is consistent with the hierarchical structure of the human neocortex Mathys (Citation2011).
The hierarchy is made up of repeating mechanisms, each of which make predictions, calculate the error between the prediction and the input, and update their beliefs based on the error and precisions. The main differences resulting from the hierarchical setting are: (1) predictions are sent to a level below, (2) the input is the prediction error from the lower level (except for the lowest level that receives sensations directly), and (3) mechanisms are organized into levels to reflect the causal order of the world. The levels are organized based on the spatiotemporal order of causality in the world: higher levels deal with larger spatiotemporal orders. Two key relationships between levels ensure efficient minimization of prediction errors at all levels. First, top-down predictions provide parameters to lower levels. For instance, if you believe that this is the face of a cat (a higher-level belief), then you infer that you should find hair nearby (a higher-level belief). Second, the higher-level belief can modulate the precision at lower levels. As discussed above, it is not wise to update priors if the prediction error is not reliable. The higher-level belief can provide information about whether the prediction error should be trusted. To take another example, if you are having a conversation with your friend in a noisy bar, your auditory input is not trustworthy. In this case, the precision of the auditory prediction error is estimated to be low, so the brain relies more on prior beliefs.
In the setting of the hierarchical Bayesian inference model, it is commonly assumed that the distinction between perception and cognition is a graded one. A cognitive representation – a belief – is a representation located at a higher-level in the hierarchy. Perceptual representation is located at a lower-level (Clark, Citation2016; Hohwy, Citation2013). Lower levels are dealing with inputs coming from specific sensory modalities. These different sources of information are however integrated at higher-levels. The interplay between levels in the hierarchy and sensory integration also plays an important role in my account.
4.1.7. Active inference
Both in the ordinary language discourses and in the philosophical traditions, actions are considered as the consequences of a desire or an intention. We look for any leftovers in the fridge because we desire for food, for example. But, FEP explains actions in terms of expectation. The food search case would be described in the following way. When we are hungry, we expect the hunger is cured. (Since we are hungry,) this is prediction error. Consequently, action is initiated to revolve this error. We know the fridge tends to contain something edible, thus we get a counterfactual belief that visiting the fridge would cure the hunger. Given this counterfactual belief and prediction error, action to reach the fridge and search inside is performed. In other words, under FEP, desires are optimistic expectations that are fulfilled by actions. This treatment might sound unnatural but it offers the advantage of explaining perception and action in the same framework: cognition changes expectation (or prediction) to minimize prediction error, action changes the world to minimize the prediction error. In turn, this implies confirmation bias is inherent in our action as our action is carried out to make our hypotheses more probable.
4.1.8. Minimizing expected free energy
A final feature of free-energy minimization to touch on is minimizing expected free-energy. An organism that can perform future-oriented goal-directed actions involving multiple steps must evaluate which policy – which action plan – is worth pursuing. This means that the organism must be able to evaluate which policy (on average) minimizes free-energy the most. That it, the organism chooses the action that minimizes expected free-energy (Friston et al., Citation2013, Citation2014).
Expected free-energy is a function of the relevant action plan. And, the lower the expected free-energy, the more the action plan is actually adopted by an organism (see Friston et al., Citation2013 for mathematical details and further discussion). As with free-energy, expected free-energy reveals interesting features of action planning. One such feature is that expected free-energy provides a solution to the classic dilemma between exploration and exploitation (should I be satisfied with what I got or should I keep looking?). Minimizing expected free-energy depends on reducing the uncertainty between action sequences (policy implementations) and goals (prior preferences). The degree of reduction of uncertainty is the epistemic value. The degree of satisfaction of prior preferences is the utility value. The latter includes whatever the organism prefers (safety, resources, reproductive partners, allies, etc.). If uncertainty is high, the organism will choose exploratory behavior that does not guarantee any utilities. But, if uncertainty is low, the organism will switch to exploiting things it prefers (Friston et al., Citation2015).
We have looked at FEP in some detail. I have already intimated at how FEP might apply to CTs. I now flesh out the details.
4.2. Applying FEP to CTs
As we have seen, FEP is a normative principle that different organisms satisfy to different degrees. As such, my goal is not to show that the behavior of all CT believers is explained by FEP and that all CT believers are therefore rational. Rather, if someone is in a certain emotional state or has gone through certain experiences, then they can end up holding CT beliefs (even when they follow FEP).
I have suggested that distrust of authorities (whether political, scientific, or the like) plays a significant role in the dynamics of CTs. I now contend that there are two kinds of distrust at play. Each is associated with different aspects of the mind: one is emotionally entrenched and the other is a cognitive or doxastic phenomenon. Let us call these low-level distrust and high-level distrust, respectively. I shall explicate both in terms the FEP framework in this section.
My central thesis is that (1) procedural and agential insulation and (2) different kinds of distrust of authorities can create dynamics in which it becomes very difficult for an agent to escape CT beliefs. This is the rabbit hole referred to in my title. Nonetheless, believing in CTs is a bounded-rational choice given the psycho-social position of some CT believers.
4.2.1. Low-level distrust and high-level distrust
There are two ways in which distrust is entrenched in CT believers. Low-level distrust obtains when a person is in an emotionally distressed state. High-level distrust obtains when distrust forms gradually through past experiences or through communication of shared stories by the members of the communities at the level of beliefs (this does not necessarily entail occurrent negative emotional states though). I shall begin with a detailed account of the dynamics of CT beliefs emerging from low-level distrust.
In the case of low-level distrust, an emotional state is the key starting point. The exact nature of emotion is a contentious issue that goes beyond the scope of this paper. Nonetheless, an FEP-congenial account suggests that felt emotions arise from actively-inferred generative models of the causes of interoceptive sensations (Seth, Citation2013). Although there is not universal agreement, there does appear to be a consensus among scholars that emotion and interoceptive sensations are closely linked (see Craig, Citation2009; Damasio, Citation1999; James, Citation1894).
Through the lens of FEP, we can see that emotion and interoceptive sensation play fundamental roles in cognition and decision-making. When we think about what kind of belief someone in a negative emotional state might settle into, we need to take interoceptive prediction errors into account (along with what the person hears, reads, etc.). This is because the relevant belief would explain the person’s interoceptive sensations (along with other sensations) within the prediction error minimization framework. When put this way, it seems likely that someone in an emotionally negative state will take up a CT. To press this point, imagine the following scenario (this is similar to one used by Giovanni Pezzulo, Citation2014).
Jojo is in a negative emotional state, such as a state of anxiety. Suppose also that Jojo has read some anecdotes about people who died immediately after getting the COVID-19 vaccine. What is the most probable explanation for this anecdotal evidence? Consider two candidates: Official Story and CT.
Official Story states that there are more benefits than harms involved in getting vaccinated. Although pharmaceutical companies developed the vaccines with good intentions, people can suffer serious side-effects in rare cases.
CT states that pharmaceutical companies (big pharma) are attempting to cull the human population through vaccinations, and that the “side-effects” are intended. Because of procedural and agential insulation, either Official Story or CT can explain the anecdotal evidence. That said, Official Story cannot explain why Jojo is feeling anxious. However, CT can explain both the anecdotal evidence and Jojo’s emotional state in one sweep. Indeed, Jojo should feel anxiety if there is a plot to kill people on a large scale. Looked at this way, CT is, in fact, a more probable hypothesis than Official Story. FEP stresses that our cognitive inferences are not emotion-free. Rather, they are embodied and situated.
Official Story can gain the same explanatory power if it is combined with an auxiliary story (perhaps, Jojo has just been laid-off). But, this also makes Official Story more complicated than the CT (there are more degrees of freedom). As we have seen, complexity increases free-energy. Simple CT is then preferable to complex Official Story, even if they have the same explanatory power (this is akin to Bayesian model-choice). Consequently, CT is the winning hypothesis.
4.2.2. Combating interoceptive prediction errors
However, this only amounts to explaining why someone may be in a negative emotional state. It does not dispel that state. Negative emotion increases prediction errors (and surprise). It is related to interceptive sensations (e.g., increased heart rate and excessive sweating) that signal an organism’s discomfort (Jojo’s discomfort, in this case). As such, negative emotion violates the organism’s expectation that it is in an environment conducive to survival.
The premodern narratives that CTs put forward seem to offer emotionally supportive accounts. They may boost someone’s sense of control over whatever (uncomfortable) situation they find themselves in. Such narratives purport to go beyond a purely factual description. In an effort to make sense of the situation, CTs outwardly explain why bad things happen, where the blame lies, and how to redress related injustices. The explanatory “aura” of CTs can support a person’s psychological well-being, precisely by producing explanatory reasons and causes. Official Story, in contrast, does not (at least, not intellectually accessible ones anyway).
Highly precise interoceptive prediction errors caused by negative emotions can encourage someone to explain away those prediction errors (Solms & Friston, Citation2018). In such cases, action will be undertaken based on a CT. As I introduced in 4.1.7, our action is carried out to fulfill our expectation. This can result in the CT believer only looking for information congenial to the CT (usually online). This explains how a rather domain-specific unorthodox account (e.g., skeptical thoughts about vaccines) can develop into a full-blown CT about big pharma and the deep state. CT believers start to see the world through the lens of their CTs. Their view of the world is informed by their CT beliefs, and this can result in CT beliefs spreading to other belief-conducive domains.
CT believers may also begin to find confirmatory evidence for their CT everywhere. This makes them more confident in their CT, and can lead them to seek further information affirming it. In terms of free-energy minimization or Bayesian inference, finding similar evidence from separate sources makes the posterior probability higher, and therefore more credible (Friston et al., Citation2010).
In addition, online fellowship with other CT believers can result in emotional fulfillment. Adherence-seeking can engender a sense of purpose, direction, or meaning (however corrupt or factitious) into a life that may otherwise lack such existential resources. On the whole, CT explanations lack proper grounds and are superficial in nature. Nonetheless, CT beliefs can be adaptive because they secure the multiple, and indeed essential, benefits of sociality. This is concordant with the notion of minimizing expected free-energy.
4.2.3. High-level distrust
The above low-level distrust account illustrates how CT beliefs can obtain in someone in a negative emotional state. It is however possible that someone distrusts authorities only at the level of beliefs (without associated negative emotions). This is what I am calling high-level distrust. High-level distrust can happen when someone (or the community they belong to) has been subjected to systematic mistreatment. In such cases, a person’s experiences can gradually form the belief that established authorities are not trustworthy.
The distrust at level of belief is situated at the higher-level of hierarchy. The higher-level belief can influence the overall prediction error minimization in the ways introduced in 4. 1. 6. First. top-down predictions from high-level distrust provide parameters to lower levels. In the case of CT, for example, one can reason as follows: “Since the government is not trustworthy, it is likely that some malicious intentions are involved in the promotion of COVID-19 vaccines.” Second, the higher-level belief can modulate the precision at lower levels. The high-level distrust predicts that information from established authorities is imprecise and discourage the person from updating her belief in light of it. In addition, just as in the case of low-level distrust, the person would actively sample the CT-congenial evidence if the probability of CT becomes high enough. It results in solidification of the belief in CT.
So far, I have illustrated low-level distrust and high-level distrust as separate phenomena. But, they are, most likely, inter-related, and can occur simultaneously. CT believers can learn to distrust authorities if exposed to a pattern of historical betrayal or mistreatment. This naturally fosters emotions like anger, frustration, and disappointment. Emotion-related low-level distrust can then gradually lead to distrust at the higher level of beliefs.
In this section, I explicated how someone might take up a CT, and how the associated beliefs are consolidated from the viewpoint of FEP. A CT can emerge as a most reasonable hypothesis from the normal functioning of approximate Bayesian inference given someone’s psychological and social situation.
5. Implications
In this section, I discuss two important implications that follow from my account. First, I examine the relationship between CTs and rationality. Second, I discuss possible directions of interventions.
5.1. (Ir)rationalioty of CTs from the perspective of FEP
I have argued that a CT believer in an environment where authorities are not trusted can be bounded-rational. CT believers appear irrational from the perspective of those in different (arguably, better) social settings. Yet, as a situated agent, a CT believer is as rational as a non-CT believer (in a better social setting).
Some might think that this “rationalization” of CT believers simply displays an inherent problem with Bayesian cognitive science (including FEP): it makes every behavior or decision rational. Proponents of Bayesian approaches might consider clinical delusions to be the result of faulty, yet Bayes-optimal, inferences (Colombo & Fabry, Citation2021). Brown et al. (Citation2013), for example, have developed a simulation of aberrant precision-weighting that reproduces the behavior of schizophrenic patients. Brown et al. state: “it should be noted that – from the point of view of the subject – its inferences are Bayes optimal. It is only our attribution of the inference as false that gives it an illusory or delusionary aspect” (2013, p. 423).
Thus, if Bayes-optimality implies some kind of rationality, then what is supposed to be irrational under Bayesian schemes? This absence of criterion to distinguish rational from irrational (or optimal from suboptimal) is a thorny problem for Bayesian cognitive science. My claim that a CT believer can be bounded-rational might then sound uninteresting. This is a topic of ongoing debate, and I cannot hope for a full resolution here. Nonetheless, I shall propose one way in which FEP might avoid the problem. Recall that the crucial observation of FEP is that an organism has to minimize surprise for survival. That is why the model of the world it harbors also includes the preferred conditions for the organism’s existence. This means that the best possible guess about the external states in the world is neither necessary nor sufficient for free energy minimization in the long run. Unless the organism succeeds in remaining in the suitable econiches and keeping its homeostatic states within a certain range, there is a degree of surprise (or free energy). In contrast, most Bayesian schemes are only concerned with cognitive decisions about external states in the face of exteroceptive and interoceptive sensations. From this limited perspective, the delusory experience that one’s movements are controlled by aliens can be described as the result from a normally functioning Bayesian mechanism that has been fed abnormal inputs. However, we think about the minimization of free energy in the long run for the system as a whole, the patients of delusory experiences presumably fail to minimize free energy (or surprise.) It is because someone who believes that they are a puppet under alien control would presumably have a difficult life. They would be emotionally distressed. This implies that, in the long run, there will be a high degree of prediction error (especially in the interoceptive domain), which in turn implies an elevated level of surprise.
At the level of a whole system, we can see that CT believers are different from delusory patients. Both suffer negative emotions, but, for CT believers, this is not a consequence of their CT beliefs. On the contrary, my account suggests that negative emotions are the cause of CT beliefs, and CTs are adopted and maintained in an effort to reduce negative emotions. Moreover, CT believers can gain social cohesion with their fellows, and thereby even improve their psychological well-being. My claim is that, given the socio-psychological situation of CT believers, they may be better off believing a CT, and it is in this sense that their choice is bounded-rational. This is not to say CT believers are overall better off than non-CT believers. Nonetheless, CT belief can be an optimal decision from the perspective of situated and embodied inference.
5.2. Possible directions of intervention
The conclusion that a CT believer can be bounded-rational may imply that intervention on them is rather difficult. What would the consequences of interventions be? I do not think that existing suggestions – like cognitive infiltration (Vermeule & Sunstein, Citation2009) or appeals to moral emotions (Cassam, Citation2019) – are likely to be effective in breaking the “hold” CTs have on their believers.
On my account, the CT believer rejects Official Story either because Official Story does not explain their emotional state or because they assign lower credence to evidence from established authorities. It seems unlikely that they will be persuaded by “infiltrators” carrying out debunking work in their online spheres. It is more likely that CT believers will collectively oust infiltrators. Regarding appeals to moral emotions, CT believers in a negative emotional state seek an explanation for their state, and this leads them to adopt a CT. This is partly because a CT generates a narrative that depicts its believers as victims. Emotive and cognitive interventions might work for those on the fence or those just tilting toward a CT, but fervent believers are unlikely to be persuaded.
What then would be a better way to intervene? Proposals for concrete forms of intervention go beyond the scope of this paper. A more detailed implementation agenda should be the topic of further research. Nonetheless, my analysis does suggest that the most effective forms of intervention will focus on the two kinds of distrust (low-level and high-level distrust). Low-level distrust starts from negative emotions, and providing emotional support to people suffering from negative emotions may then be effective. This can be a preventive measure as well as an early intervention for people tilting toward CTs. A CT should lose its position as the superior hypothesis if negative emotions are alleviated.
High-level distrust entrenched from past experiences would be harder to correct. It can be deeply rooted in the history of a person or community. A possible intervention is to acknowledge CT believers rather than alienating them as social outcasts. Acknowledging CTs does not, of course, amount to believing the content of those CTs. Understanding why people believe in CTs might result in amendments to the inequalities affecting their lives. The range of concrete measures will vary according to context. These may include redressing inequality, redistributing resources from the rich to the poor, formal recognition, restitution for the historical mistreatment of minorities, and effective social welfare.
These measures may seem indirect because they approach the problem of CTs by focusing on social and historical context. But, it seems to me that this is the most, and probably one of the few, effective ways to combat CTs.
6. Conclusion
In this paper, I focused on the special epistemic properties of CTs and an agential description of those who subscribe to them. I argued that the negative emotional states of CT believers and a deeper lack of trust in established authorities are key in both the prevalence and intractability of CTs. By appealing to the principle of free-energy, I showed how someone might take up a CT, and how their belief in the CT become reinforced over time. I also showed how believing in CTs can be regarded as bounded rational.
This FEP account not only illuminates the dynamics surrounding CTs, but also provides FEP with more theoretical credence as a unifying theory of cognition. It turns out that FEP is nicely suited to explaining complex social phenomena such as CTs. It has ample theoretical resources to accommodate embodied and situated cognition. CTs do not emerge in a vacuum. They are the result of specific historical, social, and emotional contexts. This is where intervention should take place.
Acknowledgements
I thank two anonymous reviewers for their highly helpful and constructive comments for the earlier version of this paper. I also would to thank Ryo Uehara, Yoshiyuki Hayashi, and Masaya Chiba for their comments and suggestions. This work was supported by JSPS KAKENHI Grant Number JP19K12942.
Disclosure statement
No potential conflict of interest was reported by the author.
Additional information
Funding
Notes
1. The Reuters fact checking team has rejected this interpretation. See https://www.reuters.com/article/factcheck-immunesystem-covid19-vaccines-idUSL1N2TE17B.
2. Cassam (Citation2019, p. 28) identifies three other characteristics of CTs: speculative, contrarian, and amateurish. I take it that these three characteristics are already implied by my neutral definition (section 2), despite the negative connotations of the wording.
3. Both are nonetheless CT beliefs (or, at least, will be handled as such in this paper).
References
- Bortolotti, L. (2009). Delusions and other irrational beliefs. Oxford University Press.
- Brown, H., Adams, R. A., Parees, I., Edwards, M., & Friston, K. (2013). Active inference, sensory attenuation and illusions. Cognitive Processing, 14(4), 411–427. https://doi.org/10.1007/s10339-013-0571-3
- Bruineberg, J., Dołęga, K., Dewhurst, J., & Baltieri, M. (2022). The Emperor’s new Markov Blankets. The Behavioral and Brain Sciences, 45, E183. https://doi.org/10.1017/S0140525X21002351
- Cassam, Q. (2019). Conspiracy theories. Polity Press.
- Clark, A. (2016). Surfing uncertainty: Prediction, action, and the embodied mind. Oxford University Press.
- Coady, D. (2003). Conspiracy theories and official stories. The International Journal of Applied Philosophy, 17(2), 197–209. https://doi.org/10.5840/ijap200317210
- Colombo, M., & Fabry, R. E. (2021). Underlying delusion: Predictive processing, looping effects, and the personal/sub-personal distinction. Philosophical Psychology, 34(6), 829–855. https://doi.org/10.1080/09515089.2021.1914828
- Craig, A. D. (2009). How do you feel – now? The anterior insula and human awareness. Nature Reviews Neuroscience, 10(1), 59–70. https://doi.org/10.1038/nrn2555
- Damasio, A. R. (1999). The feeling of what happens: Body and emotion in the making of consciousness. Houghton Mifflin Harcourt.
- De Coninck, D., Frissen, T., Matthijs, K., d’Haenens, L., Lits, G., Champagne-Poirier, O., Carignan, M., David, M., Pignard-Cheynel, N., Salerno, S., & Généreux, M. (2021). Beliefs in conspiracy theories and misinformation about COVID-19: Comparative perspectives on the role of anxiety, depression and exposure to and trust in information sources. Frontiers in Psychology, 12, 646394. https://doi.org/10.3389/fpsyg.2021.646394
- Freeman, D., & Bentall, R. P. (2017). The concomitants of conspiracy concerns. Social Psychiatry and Psychiatric Epidemiology, 52(5), 595–604. https://doi.org/10.1007/s00127-017-1354-4
- Friston, K. (2010). The free-energy principle: A unified brain theory? Nature Reviews Neuroscience, 11(2), 127–138. https://doi.org/10.1038/nrn2787
- Friston, K. (2012). Prediction, perception and agency. International Journal of Psychophysiology, 83(2), 248–252. https://doi.org/10.1016/j.ijpsycho.2011.11.014
- Friston, K. (2013). Life as we know it. Journal of the Royal Society Interface, 10(86), 20130475. https://doi.org/10.1098/rsif.2013.0475
- Friston, K., Daunizeau, J., Kilner, J., & Kiebel, S. J. (2010). Action and behavior: A free-energy formulation. Biological Cybernetics, 102(3), 227–260. https://doi.org/10.1007/s00422-010-0364-z
- Friston, K., FitzGerald, T., Rigoli, F., Schwartenbeck, P., & Pezzulo, G. (2016). Active inference and learning. Neuroscience & Biobehavioral Reviews, 68(1), 862–879. https://doi.org/10.1016/j.neubiorev.2016.06.022
- Friston, K., & Kiebel, S. (2009). Predictive coding under the free-energy principle. Philosophical Transactions of the Royal Society B: Biological Sciences, 364(1521), 1211–1221. https://doi.org/10.1098/rstb.2008.0300
- Friston, K., Rigoli, F., Ognibene, D., Mathys, C., Fitzgerald, T., & Pezzulo, G. (2015). Active inference and epistemic value. Cognitive Neuroscience, 6(4), 187–214. https://doi.org/10.1080/17588928.2015.1020053
- Friston, K., Schwartenbeck, P., Fitzgerald, T., Moutoussis, M., Behrens, T., & Dolan, R. (2013). The anatomy of choice: Active inference and agency. Frontiers in Human Neuroscience, 7, 598. https://doi.org/10.3389/fnhum.2013.00598
- Friston, K., Schwartenbeck, P., FitzGerald, T., Moutoussis, M., Behrens, T., & Dolan, R. J. (2014). The anatomy of choice: Dopamine and decision-making. Philosophical Transactions of the Royal Society B: Biological Sciences, 369(1655), 20130481. https://doi.org/10.1098/rstb.2013.0481
- Georgiou, N., Delfabbro, P., & Balzan, R. (2021). Conspiracy-beliefs and receptivity to disconfirmatory information: A study using the BADE task. SAGE Open, 11(1), 21582440211006131. https://doi.org/10.1177/21582440211006131
- Ghodsee, K., & Orenstein, M. (2021). Why won’t Eastern Europeans get vaccinated? The Strategist. Retrieved from https://www.aspistrategist.org.au/why-wont-eastern-europeans-get-vaccinated/.
- Hansen, C. H., Schelde, A. B., Moustsen-Helm, I. R., Emborg, H., Krause, T. G., Mølbak, K., & Valentiner-Branth, P. (2021). Vaccine effectiveness against SARS-CoV-2 infection with the omicron or delta variants following a two-dose or booster BNT162b2 or mRNA-1273 vaccination series: A Danish cohort study. medRxiv. Retrieved from https://www.medrxiv.org/content/10.1101/2021.12.20.21267966v2.
- Hohwy, J. (2013). The predictive mind. Oxford University Press.
- Hohwy, J. (2016). The self-evidencing brain. Noûs, 50(2), 259–285. https://doi.org/10.1111/nous.12062
- Hohwy, J. (2021). Self-supervision, normativity and the free energy principle. Synthese, 199(1–2), 29–53. https://doi.org/10.1007/s11229-020-02622-2
- James, W. (1894). The physical basis of emotion. Psychological Review, 1(5), 516–529. https://doi.org/10.1037/h0065078
- Kiefer, A., & Hohwy, J. (2019). Representation in the prediction error minimization framework. In S. Robins, J. Symons, & P. Calvo (Eds.), The Routledge companion to philosophy of psychology (pp. 384–409). Routledge.
- Levy, N. (2007). Radically socialized knowledge and conspiracy theories. Episteme, 4(2), 181–192. https://doi.org/10.3366/epi.2007.4.2.181
- Mathys, C. (2011). A Bayesian foundation for individual learning under uncertainty. Frontiers in Human Neuroscience, 5, 39. https://doi.org/10.3389/fnhum.2011.00039
- Miller, J. M., Saunders, K. L., & Farhart, C. E. (2016). Conspiracy endorsement as motivated reasoning: The moderating roles of political knowledge and trust. American Journal of Political Science, 60(4), 824–844. https://doi.org/10.1111/ajps.12234
- Ndugga, N., Hill, L., Artiga, S., & Haldar, S. (2022). Latest data on COVID-19 vaccinations by race/ethnicity. KFF. Retrieved from https://www.kff.org/coronavirus-covid-19/issue-brief/latest-data-on-covid-19-vaccinations-by-race-ethnicity/.
- Parr, T., Pezzulo, G., & Friston, K. J. (2022). Active inference: The free-energy principle in mind, brain, and behavior. MIT Press.
- Pezzulo, G. (2014). Why do you fear the bogeyman? An embodied predictive coding model of perceptual inference. Cognitive, Affective, & Behavioral Neuroscience, 14(3), 902–911. https://doi.org/10.3758/s13415-013-0227-x
- Rao, R. P. N., & Ballard, D. H. (1999). Predictive coding in the visual cortex: A functional interpretation of some extra-classical receptive-field effects. Nature Neuroscience, 2(1), 79–87. https://doi.org/10.1038/4580
- Seth, A. K. (2013). Interoceptive inference, emotion, and the embodied self. Trends in Cognitive Sciences, 17(11), 565–573. https://doi.org/10.1016/j.tics.2013.09.007
- Simon, H. A. (1956). Rational choice and the structure of the environment. Psychological Review, 63(2), 129–138. https://doi.org/10.1037/h0042769
- Simon, H. A. (2000). Bounded rationality in social science: Today and tomorrow. Mind & Society, 1(1), 25–39. https://doi.org/10.1007/BF02512227
- Solms, M., & Friston, K. (2018). How and why consciousness arises: Some considerations from physics and physiology. Journal of Consciousness Studies, 25(5–6), 202–238.
- Vermeule, C. A., & Sunstein, C. R. (2009). Conspiracy theories: Causes and cures. The Journal of Political Philosophy, 17(2), 202–227. https://doi.org/10.1111/j.1467-9760.2008.00325.x
- Williams, D. (2021). Socially adaptive belief. Mind & Language, 36(3), 333–354. https://doi.org/10.1111/mila.12294