ABSTRACT
Domain adaption has attracted quite a lot of interest in object detection. It is hoped that domain adaption can mitigate the discrepancy between the source domain with label-rich and the target domain with no label or label-scarce. In this paper, we address the domain transfer issue from three aspects: (1) We design a synthetic image generator with automatically labelled based on 3d scenes. (2) A structured domain adaptation framework was designed and two domain adapters on global-local levels, respectively, were constructed to mitigate the cross-domain gap. (3) By the virtue of synthetic data, different strategies are adopted to deal with sample transferability in the global and local levels, respectively, to eliminate the performance bottleneck. Recently, adversarial-based methods were used to make alignment with distributions for source and target objects that have been proven effective when adapting object classifiers. We reinforce the two domain adapters to learn a domain classifier with synthetic data and a consistency regularisation is applied to boost the performance. We conduct extensive experiments to demonstrate that the proposed model achieves large performance margins, which show significant advances over the other state-of-the-art models, performing a promotion of 10% −15% mAP on various domain adaptation scenarios.
Disclosure statement
No potential conflict of interest was reported by the author(s).
Additional information
Notes on contributors
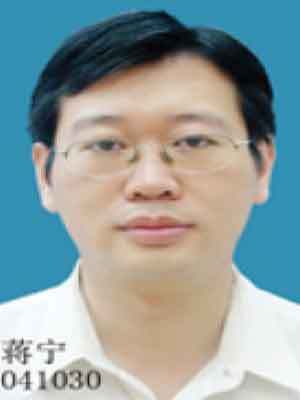
Ning Jiang
Jiang Ning is currently working toward the Ph.D. degree at school of computer science and technology, Hangzhou Dianzi University, Hangzhou, China. His research interests include domain adaptation systems , deep learning and its application.
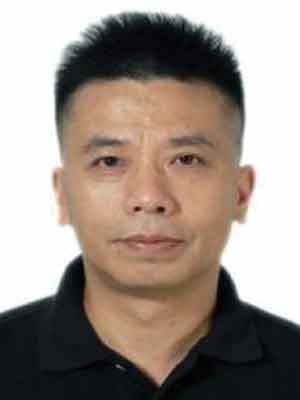
Jinglong Fang
Jinglong Fang received his Ph.D. degrees in Zhejiang University of Technology, China. Now he is a professor and deputy head of School of Computer Science at Hangzhou Dianzi University, China. He has wide research interests, mainly including machine learning, data mining, pattern recognition and artificial intelligence.
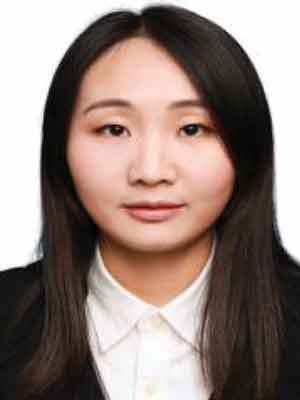
Yanli Shao
Yanli Shao received her Ph.D. degree in Zhejiang University, China, in 2016. She used to work at the Institute of Automation, Chinese Academy of Sciences from 2017 to 2018. Currently, she is an associate researcher at the School of Computer Science, Hangzhou Dianzi University, China. Her research interests include computer aided design, software engineering, and artificial intelligence.