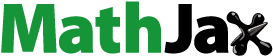
ABSTRACT
The use–make framework is employed to explain functional forms in production theory, including Cobb–Douglas and Leontief. Productivity and efficiency are interrelated by augmenting the framework with a linear program that determines the frontier output.
1. Introduction
In this paper, I present the concepts of productivity and efficiency in the use–make framework of input–output analysis. This framework is very flexible. It accommodates different numbers of inputs, outputs, and production units. Perhaps surprisingly, it even has the capacity to explain parametric production functions, not only Leontief functions, but also functions featuring substitution. The different results, particularly the derivation of the Cobb–Douglas and Leontief functions and the decomposition of productivity growth in technical change and efficiency, are not new, but are now interrelated in a unifying framework, the use–make framework. This has not only pedagogical appeal, but I hope also stimulates cross fertilization, such as the introduction of efficiency measures in input–output analysis and, vice versa, the use of a general equilibrium input–output framework in productivity analysis.
I have taken a leave of absence from input–output research and studied the concepts of efficiency and productivity in the fundamental framework of Debreu (Citation1951); see ten Raa (Citation2008). This paper is a coming home. I show how the stuff I learned is useful in input–output analysis and, perhaps more significant, how use–make tables are an organizing framework not only for national accounting (Beutel, Citation2017) but also for economic theory (Rueda-Cantuche, Citation2017, ten Raa, Citation2017, and Shestalova, Citation2017, and the references given there).
The use–make framework is presented in the next section. To connect with traditional input–output analysis, I review the case where the number of products is equal to the number of industries. Section 3 determines the frontier output of the economy. To connect with traditional productivity analysis, I also consider the case of a small, open economy. In such an economy, all products are priced by the international terms of trade and, therefore, all industries are machines transforming factor inputs into value added. In other words, industries have multiple (factor) inputs, but essentially a single ‘output,’ namely value added. This single output case facilitates the construction of the Cobb–Douglas production function in Section 4. General productivity and efficiency accounting follow in Section 5, including the decomposition of the Solow residual in technical change and efficiency change terms. In Section 6, I consider the opposite extreme case, an economy with a single ‘input,’ say labor, and multiple products. This case shows the emergence of the Leontief function between output and input. The final section concludes.
2. The use–make framework
An economy has endowments, activities, which make products, and consumers. The endowments are used as factor inputs. The activities are called decision-making units in operations research, firms in economics, and industries in input–output analysis. In this journal, I opt for the latter terminology. The products are used by the industries and the consumers. The industries thus use factor inputs and intermediate (produced) inputs. Products not used by the industries feed the consumers. This is final demand. The economy is a machine that transforms factor inputs into final demand. Productivity is final demand per unit of factor input. The trouble is that both the numerator and the denominator are multidimensional. After presenting the use–make framework, the cases with a single output and a single input will be dealt with first.
Presently the main reference for the use–make framework is Beutel (Citation2017). I focus on what is necessary for the development of production functions and productivity measures. I begin with some notation. The economy has l factor inputs, m industries, n products. The factor use table is l×m- dimensional matrix L. The first row depicts the distribution of say labor by industry. The first column depicts the factor inputs of industry 1. And so on for the other rows and columns. The use table is n×m-dimensional matrix U. The first column depicts the intermediate inputs of industry 1. The first row depicts the distribution of the first intermediate input by industry. The make table is n×m-dimensional matrix V. The first column depicts the products of industry 1. The first row depicts the distribution of the first product by industry. In the System of National Accounts, the make table is of opposite dimension, but for this paper, it is handy to have it as the same dimension as of the use table. The unit vector, with all entries equal to 1, is denoted by e. It acts as summation vector. For example, Ve is the vector of row totals of the make table, i.e. the gross output product vector, denoted x:
(1)
(1)
Subtraction of the intermediate input vector, Ue, yields the final demand vector, denoted y:
(2)
(2)
The economy is a machine that transforms factor inputs L into final demand y. An economy is more productive if it produces more y for less L. The framework is very general. Activities, of which there are m, may well be production establishments. Establishments producing the same outputs, even in the same proportions, may have disproportionate inputs. If so, one establishment is more efficient than another. Efficient establishments determine the production possibility frontier of the economy. The further away an establishment is from the frontier, the less efficient it is.
In traditional input–output analysis, however, the number of activities is equal to the number of products. All industries determine the production possibility frontier. None are inefficient. This is why, historically, there is little attention for efficiency analysis in the input–output community. In traditional input–output analysis m = n and, therefore, make table V is square. If it has a dominant diagonal (meaning that the majority of any product is produced in the corresponding industry, the V is invertible and
(3)
(3)
is the matrix of input–output coefficients (following the commodity technology model, Rueda-Cantuche, Citation2017). Input–output coefficients are inputs divided by outputs. Equations 1–3 imply that final demand
or
(4)
(4)
Equation 4 is the basic equation of traditional input–output analysis. It connects final demand to the factor inputs. The vector of factor inputs is obtained by summation of the factor use table over industries: Le. Analogous to Equation 3, the matrix of factor input coefficients is
(5)
(5)
By Equations 5 and 1, the vector of factor inputs is
. Substituting the Leontief inverse of Equation 4,
(6)
(6)
where
is the matrix of factor contents. If products have low factor contents, then the factor inputs produce much net output, the economy is productive.
3. Frontier output
The economy maps factor inputs Le into net output (V − U)e. Could it produce more? Not in the traditional case, with as many industries as products. However, if there are more activities than products, m > n, there will be room for improvement by reallocation of factor inputs to the efficient activities. The way to model this is by setting up a linear program that maximizes the level of net output by reallocating activity. The implicit assumption is that the consumers have Leontief preferences. This assumption has been shown to free the analysis from unobserved consumer data and to yield conservative inefficiency estimates (ten Raa, Citation2008). In the observed situation, the activity level vector is e. The reallocation vector will be denoted by s. If, for example, s1 = 1.1, then industry 1 will produce 10% more. The level of final consumption will be denoted by c. If, for example, c = 1.25, then the economy will produce 25% more. The actual level of net output, normalized at 1, is lower than potential net output, 1.25. The economy, in this example, is 80% efficient. In general, efficiency is measured by 1/c.
In maximizing the level of final demand by reallocation, the economy is constrained by the material balance and the factor endowments. The linear program is as follows:
(7)
(7)
The objective function is final demand level c. I have included a multiplicative scalar,
the national product. (The prime denote transposition, making summation vector e a row vector.) Program (7) has n product constraints and l factor input constraints. Denote the Lagrange multipliers of the product constraints by p and of the factor input constraints by w. By the theory of linear programing, the Lagrange multipliers equal the marginal products of the constraining entities,
and the total value of the bounds (0 and Le) equals the value of the program: wLe = yec. National income equals the national potential product.
If the economy is small and open, net output (V − U)s can be distributed between domestic final demand yc and net exports z. Net exports are constrained below by the balance of payments, πz ≥ 0, where π is the row vector of the world terms of trade. In this case, the analysis of the linear program simplifies. This is demonstrated by invoking the dual program. On the suggestion of a referee, I present the concepts of primal and dual programs in full (ten Raa, Citation2005).
A linear program is a triplet (a b C) where a is an n-dimensional row vector of objective function coefficients, b an m-dimensional column vector of bounds, and C an m×n-dimensional matrix of constraint coefficients. The primal program reads
(8)
(8)
In problem (8), x is an n-dimensional column vector of variables. The first-order condition of problem (8) is that the gradient of the objective function, a, is proportional to the gradient of the constraint function, a = λC, where λ is an m-dimensional row vector of Lagrange multipliers, one for each constraint. The dual program associated with (8) reads
(9)
(9)
According to the main theorem of linear programing, the solution values of programs (8) and (9) match. This means that the value of program (8) is imputed to the binding entities according to the prices given by vector λ. For this reason, Lagrange multipliers are also called shadow prices. And, related, program (9) shows that the shadow prices equal the marginal productivities of the binding entities. They reign in perfectly competitive economies.
Let us write a frontier program (7) in the general format (8) and bring in net exports z, and the balance of payments, πz ≥ 0. The constraints are the material balance, the factor constraint, the balance of payments, and the nonnegativity of the allocation variable. (The nonnegativity of the consumption level variable, c, is automatic, since c = 1 is observed hence feasible.) These are the four rows in the constraint matrix:
(10)
(10)
Denote the Lagrange multipliers of the constraints by p, w, ϵ, and σ: the product prices, the factor input prices, an exchange rate, and nonnegativity slack variables, respectively. Then the dual constraint, in (9), reads
(11)
(11)
The first component of (11) is p(V − U) = wL − σ: value added equals factor costs minus slack. If a slack component is positive, then the associated constraint, which is a nonnegativity constraint of a component of allocation variable s, is binding and, therefore, industries with greater factor cost than value added are inactive in the allocation supporting frontier output. The second component of (11) is a price normalization constraint, . The third component, p = ϵπ, dictates the international relative prices and the level
so that
The dual program minimizes factor costs, wLe, subject to the value constraints p(V − U) ≤ wL. There are l variables (for the factor prices) and m constraints (for the industries). l constraints will be binding (ten Raa, Citation2005, p. 48) and locate the active industries. The m − l nonbinding constraints signal positive slack components of σ, hence inactivity.
I will now rewrite this in simplified value-added notation, to clarify the structure of the frontier program and to pave the way for the introduction of industry capacity constraints which may preclude the extreme specialization into only l constraints. Denote value added by v = p(V − U), a row vector with m components, one for every activity.
Frontier output is determined by maximizing By the second component of (11) this pyc. Now we are going to apply the fact that any slack in a constraint is nullified by the Lagrange multiplier to the material balance, the first component of the constraint in (10). Hence
or, substituting the balance of payments and the definition of value added, pyc = vs. In other words, the maximization of the level of final consumption is equivalent to the maximization of value added. A small, open economy can thus be considered a single output producer, of value added, which is derived by valuating product outputs and inputs at world prices and taking the differences, for each industry.
4. Multiple input–single output production functions
Identify an activity by its factor input structure per unit of value added. For example, the first activity, with value added v1 = p(V•1 − U•1), is identified by L•1/v1. This is a vector of factor intensities. In the traditional case, there are two factor inputs, namely capital and labor. Denote the two factor intensities by k and l, respectively, and the total endowments by M and N, respectively. Rescale activity levels such that activity level one corresponds to the use of k and l in the produce of one unit of value added. The primal program selects a pair of activities with minimal factor intensities. And the scales of production are now equal to value added. But now let there be capacity constraints s(k, l) ≤ S(k, l). The optimal activities are now bound, will be used to full capacity, and next best activities will also be engaged. Activities with binding capacity constraints pick up a positive shadow price of the capacity constraint (Ricardian rent) and activities run with nonbinding capacity constraints break even, as before the active industries with no capacity constraints.
Subject to the factor constraints ∫∫s(k, l)kdkdl ≤ M and ∫∫s(k, l)ldkdl ≤ N we maximize value added ∫∫s(k, l)dkdl. This is a linear program with a continuum of variables s(k, l). With a slight abuse of notation (previously w was the row vector of all factor input prices) now denote the shadow prices of the two factor constraints by scalars r and w, respectively. By the phenomenon of complementary slackness unprofitable activities, with unit cost rk + wl > 1, are not run, s(k, l) = 0. By the same argument, profitable activities, with unit cost rk + wl < 1, are run at full capacity, s(k, l) = S(k, l). Activities which break even, rk + wl = 1, have activity 0 ≤ s(k, l) ≤ S(k, l), but since the set of such activities has measure zero we may set s(k, l) = S(k, l). It follows that inputs and output are M = ∫∫rk+wl≤1 S(k, l)kdkdl, N = ∫∫rk+wl≤1 S(k, l)ldkdl, and value added Y = ∫∫rk+wl≤1 S(k, l)kdkdl, respectively. The implicit assumption is that all factor input can be fully employed. There must be activities with factor intensity k/l below endowment ratio K/L and activities with factor intensity above the endowment ratio.
The three expressions, for inputs K and L and output Y, are interrelated by the two shadow prices r and w. The idea of Houthakker (Citation1955) is to use the first to expressions to solve for r and w in terms of K and L. Substitution of the results in the third expression yields output as a function of the inputs. Houthakker (Citation1955) carries out this calculation for the capacity distribution with Pareto density function, S(k, l) = μkκ−1lλ−1, where μ, κ, and λ are positive constants. The result is Y = AKαLβ with α = κ(κ + λ + 1), β = λ(κ + λ + 1) and A a positive constant depending on μ, κ, and λ. In other words, a Pareto capacity distribution yields a Cobb–Douglas production function. This is Houthakker’s Theorem.
At the micro level, activities have fixed input–output ratios – it takes given amounts of labor to operate given machinery and equipment – but a change in resources, such as the inclusion of the East German labor force in the year 1989, is accommodated by the activation of new activities and the deactivation of some incumbent activities. Reallocations of resources across activities with different factor intensities manifest as substitutions at the macro level of the economy.
Clearly, different capacity distributions for the activity levels will generate different production functions. Houthakker (Citation1955) has generated a stream of theoretical and applied research, to day. The bulk of this literature features a lower dimension, with only one variable input, namely labor, and again one fixed output, which is now capital. In this one fixed-one variable input framework, Levhari (Citation1968) found the capital distribution for which total output is a CES function of the total fixed input (capital) and the total variable input (labor) and showed it encompasses the Cobb–Douglas function. Muysken (Citation1983) has consolidated the Cobb–Douglas, CES, and VES functions by showing they are all generated by beta distributions, with alternative parametrizations. Two books on the distribution approach to production are Johansen (Citation1972) and Sato (Citation1975). In this literature, activities have fixed input–output proportions and capacity constraints explain the existence of inefficient activities. Increases in levels of inputs prompt the activation of less-efficient activities, in Ricardian style. The law of one price yields rents to the more-efficient activities. The activation of different activities prompts different proportions between the input totals and the output.
5. Productivity and efficiency
The continuum of activities was a theoretical device to explain the emergence of the Cobb–Douglas function in the use–make framework. Having completed that business, let us return to the case of a finite number of activities, in fact, two suffices, with only one factor input. So firm 1 has factor input intensity l1 and firm 2 l2. We organize them in row vector l. The (labor) endowments are N1 and N2. Let the first industry be more productive than the second: l1 < l2. (This is an innocent assumption, because we are free to relabel the industries.) Then industry 1 can produce no more than it produces, at least under the assumption that the data represent all conceivable practices of production. Industry 2, however, could perform better by adopting the technique of industry 1.
Frontier output is determined by a maximization program. The program can be applied to a firm (1 or 2) or to the industry (the duopoly) and the results can be combined to determine firm and industry efficiencies (ten Raa, Citation2011). The simplest program features constant returns to scale and is applied to the economy:
(12)
(12)
The dual program minimizes the value of the bound, w(N1 + N2), subject to the dual constraint. The matrix of constraint coefficients, including of the nonnegativity constraints, is
. The dual constraint is
. As the solution is the economy-wide adoption of technique 1, s1 > 0, hence s1 ≥ 0 is nonbinding, hence its Lagrange multiplier is zero, σ1 = 0, hence the first component of the dual constraint reads wl1 = 1 or w = 1/l1. Since l1 is the amount of labor per unit of output in the relatively productive industry, the shadow wage equals output per unit of labor, i.e. productivity, in the relatively productive industry. If this shadow wage would prevail, industry 1 would break even and industry 2 would be unprofitable. This confirms that shadow prices are perfectly competitive prices, would induce profit maximizers to select the activities that yield frontier output.
The frontier program can also be applied to individual industries. For industry 2 it reads
(13)
(13)
The solution is s = (N2/l1 0): industry 2 would adopt the technique of industry 1. Actual output is N2/l2 and frontier output is N2/l1. As in Section 3, the ratio of actual output to frontier output defines efficiency. Here, efficiency is equal to
i.e. the ratio of actual productivity to optimal productivity.
Now we return to the general use–make framework, (7), with the factor input shadow prices arranged in row vector w. Productivity growth of factor input i is where the dot stands for the derivative with respect to time. This is sensitive with respect to the unit of measurement. However, aggregating across factor inputs, weighing by the units of inputs,
the sensitivity gets lost, because
in which the ratio is a growth rate while the subsequent product is insensitive with respect to the unit of measurement. It is also customary to express the change in the output/input ratio as a growth rate, by dividing by the level,
and to use real wages by subtracting the growth rate of the final demand prices. In short, the output/input ratio grows at the rate
(14)
(14)
Expression (14) is called technical change. This Lagrange multiplier approach to productivity can be related to the Solow residual approach. Recall that the value of the primal program, (10), is imputed to the bounds by the shadow prices
or, using the second component of (11), pyc = wLe. Differentiating totally,
and, therefore, expression (14) equals
or
(15)
(15)
The left hand side of expression (15) is the Solow (Citation1957) residual between net output growth and factor input growth. On the right hand side, the first term is technical change and the second term is efficiency change indeed the growth rate of efficiency term 1/c of Section 3.
6. Single input–multiple output production
In Section 4, substitution was considered a symptom of the change in the range of active activities (run with positive intensity), from labor intensive to capital intensive. Not surprisingly, this mechanism disappears when there is only one input. Perhaps surprisingly though, the disappearance of the substitution mechanism holds true even when there are multiple outputs, as the next section will review in detail.
In classical economics labor is the only factor input; all other inputs are produced commodities, also called intermediate inputs. Production output is used to fulfill intermediate demand and final demand, where the latter is defined residually, as the difference between output and intermediate input. Production output is also called gross output; similarly, final demand is also called net output. In standard input–output analysis each output has a single technique to produce it. Assuming constant returns to scale, the input of commodity j, j = 1, … , n, per unit of output, is denoted by the input vector (a1j, … , anj, lj), of which the components represent the n intermediate inputs and the factor input (labor), respectively. If these unit input requirements are fixed, they are necessarily efficient, because actual and optimal production coincide. If, however, there is a set of input vectors for each product j, there is room to reallocate labor between alternative techniques, which may save labor or, alternatively, increase output. This would increase the output/input ratio from actual productivity to optimal productivity. The ratio of the two would be efficiency. A deep result states that the optimal input vectors, one for each product, are independent of the composition of final demand. This is the substitution theorem, but for an obvious reason also called non-substitution theorem, which goes back to Samuelson (Citation1951). The proof of the theorem has a long history, in which details have been worked out and minor flaws eliminated. This culminated in a proof based on the efficiency program of maximizing the expansion factor of a some net output vector, determining the optimal input vectors, one for each product, and showing that this combination of input vectors remains optimal when the net output vector is replaced by another one (ten Raa, Citation1995).
The substitution theorem yields an all-purpose frontier technology, featuring one technique for each product. Given any net output vector, one can compare the optimal labor input to the actual labor input. The ratio is the efficiency of the economy. The extension of the analysis to multiple factor inputs is the subject of the next section.
7. Conclusion
When the number of products is equal to the number of industries, the use–make framework specializes in traditional input–output analysis. At the reporting level use–make data are not consolidated, but firm specific. The raw use–make tables are rectangular, with more ‘industries’ than products. The consequent scope for reallocations is exploited to determine the frontier output of the economy by linear programing. Moreover, if the economy is small and open, all industries are machines transforming factor inputs into value added. This essentially single output case facilitates the construction of the Cobb–Douglas and related production functions. The ratio between actual and potential output measures efficiency and productivity may grow due to efficiency and technical changes, as is shown in the general multiple input and multiple output case. In the opposite polar case, an economy with a single ‘input,’ say labor, and multiple products, generates the Leontief function between output and input.
Opposite functional forms of production functions, such as Cobb–Douglas and Leontief, are thus generated by the general use–make framework, which is the basis of modern input–output analysis. In this sense, nonparametric analysis, including input–output and linear programing, is more fundamental than parametric analysis.
Disclosure statement
No potential conflict of interest was reported by the authors.
References
- Beutel, J. (2017) The Supply and Use Framework of National Accounts. In: Thijs ten Raa (ed.) Handbook of Input-Output Analysis. Cheltenham, Edward Elgar, 41–132.
- Debreu, G. (1951) The Coefficient of Resource Utilization. Econometrica, 19(3), 273–292. doi: 10.2307/1906814
- Houthakker, H.S. (1955) The Pareto Distribution and the Cobb-Douglas Production Function in Activity Analysis. The Review of Economic Studies, 23(1), 27–31. doi: 10.2307/2296148
- Johansen, L. (1972) Production Functions: An Integration of Micro and Macro, Short run and Long run Aspects. Amsterdam, North-Holland.
- Levhari, D. (1968) A Note on Houthakker’s Aggregate Production Function in a Multifirm Industry. Econometrica, 36(1), 151–154. doi: 10.2307/1909609
- Muysken, J. (1983) Transformed Beta-capacity Distributions of Production Units. Economics Letters, 11(3), 217–221. doi: 10.1016/0165-1765(83)90138-6
- Samuelson, P.A. (1951) Abstract of a Theorem Concerning Substitutability in Open Leontief Models. Activity analysis of production and allocation 13, 142–6.
- Sato, K. (1975) Production Functions and Aggregation. Amsterdam, North-Holland.
- Solow, R.M. (1957) Technical Change and the Aggregate Production Function. The Review of Economics and Statistics, 39(3), 312–320. doi: 10.2307/1926047
- ten Raa, T. (1995) The Substitution Theorem. Journal of Economic Theory, 66(2), 632–636. doi: 10.1006/jeth.1995.1062
- ten Raa, T. (2005) The Economics of Input-Output Analysis. Cambridge, Cambridge University Press.
- ten Raa, T. (2008) Debreu’s Coefficient of Resource Utilization, the Solow Residual, and TFP: The Connection by Leontief Preferences. Journal of Productivity Analysis, 30(3), 191–199. doi: 10.1007/s11123-008-0112-8
- ten Raa, T. (2011). Benchmarking and Industry Performance. Journal of Productivity Analysis, 36(3), 285–292. doi: 10.1007/s11123-011-0214-6
- ten Raa, T. (2017). Multipliers, Factor Contents, and Productivity. In: Thijs ten Raa (ed.) Handbook of Input-Output Analysis. Cheltenham, Edward Elgar, 223–244.
- Rueda-Cantuche, J.M. (2017). The Construction of Input-Output Coefficients. In: Thijs ten Raa (ed.) Handbook of Input-Output Analysis. Cheltenham, Edward Elgar, 133–174.
- Shestalova, V. (2017). Input-Output Based General Equilibrium Analysis of Efficiency. In: Thijs ten Raa (ed.) Handbook of Input-Output Analysis. Cheltenham, Edward Elgar, 372–406.